What is exploratory data analysis
Summary
TLDRExploratory Data Analysis (EDA) is a vital approach in statistics that involves visual methods and basic statistics to uncover trends, outliers, and patterns within data sets. Unlike classical analysis, EDA does not impose models but allows data to suggest the most fitting model. It is one of three main data analysis approaches, along with classical and Bayesian analysis, each with its sequence and focus. EDA employs techniques like scatterplots and boxplots to maximize insights and inform subsequent modeling steps.
Takeaways
- 📊 Exploratory Data Analysis (EDA) is a method used to analyze datasets to summarize main characteristics, often with visual methods.
- 🔍 EDA uses graphics and basic sample statistics to get a feel for the information that might be obtainable from a dataset.
- 📈 The goal of EDA is to generate theories that can later be tested in a formal modeling step.
- 🔑 EDA involves techniques that allow for quick examination of data for trends, outliers, and patterns.
- 🌟 EDA maximizes insight into a dataset, uncovers underlying structures, and helps in detecting outliers and anomalies.
- 🛠️ EDA does not impose deterministic or probabilistic models on the data but allows the data to suggest the best fitting models.
- 📚 There are three popular data analysis approaches: classical, exploratory (EDA), and Bayesian.
- 🔄 The classical approach follows data collection with model imposition and focuses on the parameters of that model.
- 🚀 Unlike classical analysis, EDA does not start with model imposition but with analysis to infer an appropriate model.
- 🎯 Bayesian analysis uses probability statements based on prior data and updates these with new information to determine posterior probabilities.
- 📈 EDA techniques are primarily graphical, including scatterplots, boxplots, histograms, and more.
- 🧩 In practice, data analysts often mix elements from classical, EDA, Bayesian, and other approaches.
Q & A
What is Exploratory Data Analysis (EDA)?
-Exploratory Data Analysis (EDA) is an approach to analyzing data sets to summarize their main characteristics, often using visual methods. It involves using graphics and basic sample statistics to get a feeling for the information that might be obtainable from a data set.
Is a statistical model necessary for EDA?
-A statistical model can be used in EDA, but it is not a requirement. EDA primarily focuses on seeing what the data can tell us beyond formal modeling or hypothesis testing.
What are the main goals of EDA?
-The main goals of EDA are to uncover underlying structures, extract important variables, detect outliers and anomalies, test underlying assumptions, develop parsimonious models, and determine optimal factor settings.
How does EDA differ from classical data analysis?
-Classical data analysis follows data collection with the imposition of a model and focuses on the parameters of that model. In contrast, EDA does not impose models on the data but allows the data to suggest admissible models that best fit it.
What is the purpose of Bayesian analysis?
-The purpose of Bayesian analysis is to determine posterior probabilities based on prior probabilities and new information. It uses probability statements to answer research questions about unknown parameters, incorporating domain knowledge and expertise as new information is obtained.
What is the difference between prior and posterior probabilities in Bayesian analysis?
-Prior probability is the probability an event will happen before new evidence is taken into account. Posterior probability is the probability an event will happen after all evidence or background information has been considered.
What types of graphical techniques are commonly used in EDA?
-EDA techniques include scatterplots, boxplots, histograms, and other graphical methods that help to visualize data and identify trends, outliers, and patterns.
How does EDA contribute to the development of theories?
-EDA contributes to the development of theories by allowing analysts to quickly look at data for trends, outliers, and patterns, which can then be used to formulate hypotheses or theories that can later be tested in the modeling step.
Why is it important to understand the differences between the three data analysis approaches mentioned in the script?
-Understanding the differences between classical, exploratory, and Bayesian approaches is important because it helps analysts choose the most appropriate method for their specific problem, ensuring that the analysis is conducted in a way that best suits the data and the research question.
How do data analysts typically apply the different approaches in real-world scenarios?
-In the real world, data analysts often freely mix elements of classical, exploratory, and Bayesian approaches, as well as other methodologies, to best address the complexities and specific requirements of their analysis.
What is the role of sample statistics in EDA?
-Sample statistics, such as mean, median, and standard deviation, play a crucial role in EDA by providing basic numerical summaries of the data, which help analysts to get an initial sense of the data set's characteristics.
Outlines
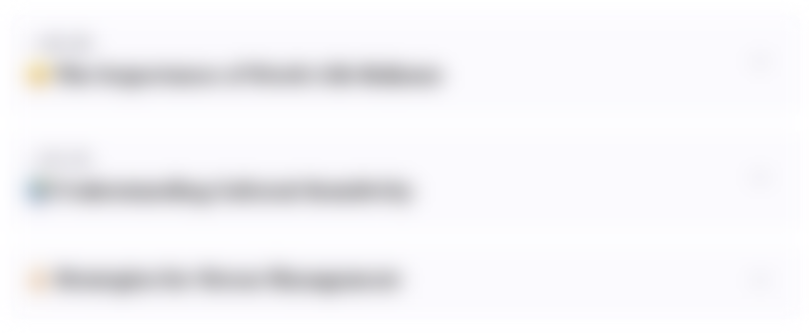
Esta sección está disponible solo para usuarios con suscripción. Por favor, mejora tu plan para acceder a esta parte.
Mejorar ahoraMindmap
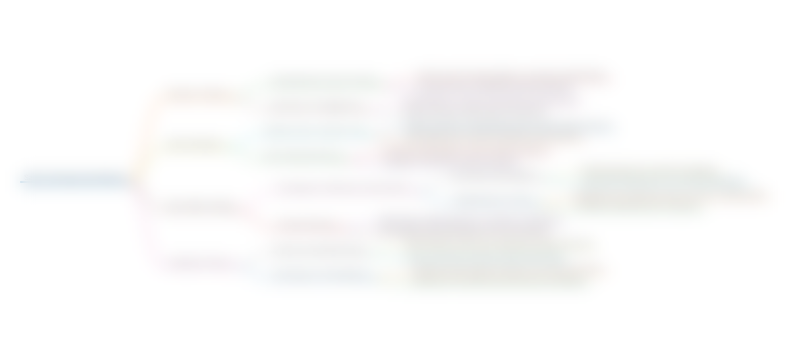
Esta sección está disponible solo para usuarios con suscripción. Por favor, mejora tu plan para acceder a esta parte.
Mejorar ahoraKeywords
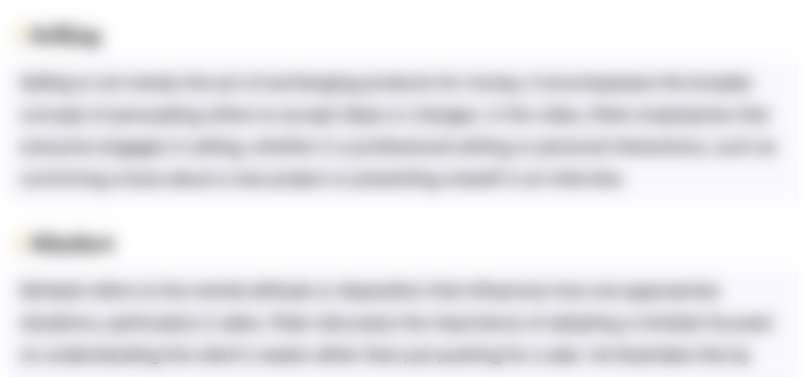
Esta sección está disponible solo para usuarios con suscripción. Por favor, mejora tu plan para acceder a esta parte.
Mejorar ahoraHighlights
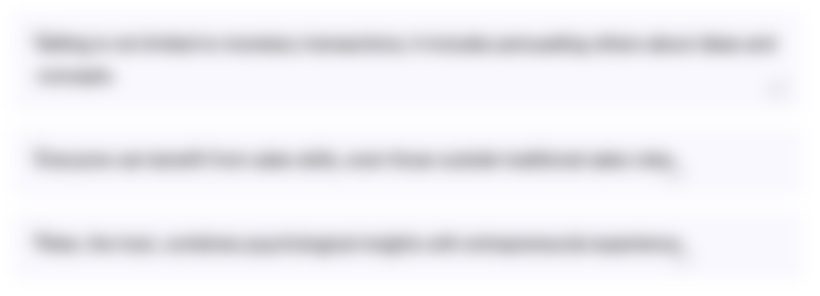
Esta sección está disponible solo para usuarios con suscripción. Por favor, mejora tu plan para acceder a esta parte.
Mejorar ahoraTranscripts
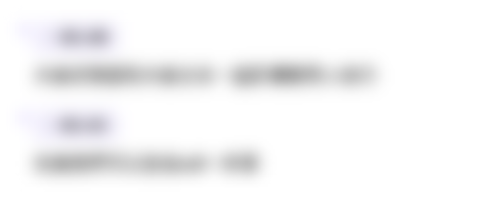
Esta sección está disponible solo para usuarios con suscripción. Por favor, mejora tu plan para acceder a esta parte.
Mejorar ahoraVer Más Videos Relacionados

EXPLORATORY ANALYSIS OF GEOLOCATIONAL DATA (MINI PROJECT)
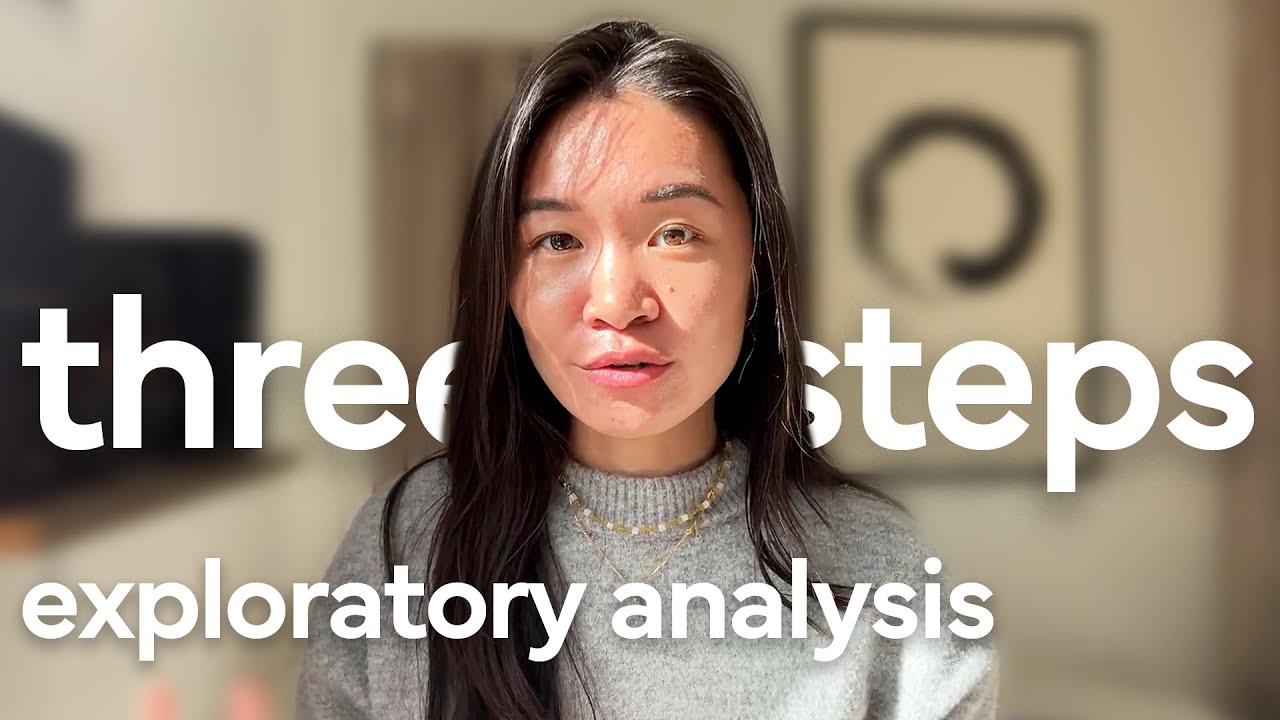
The EDA framework data hiring managers love (use it in your portfolio projects)
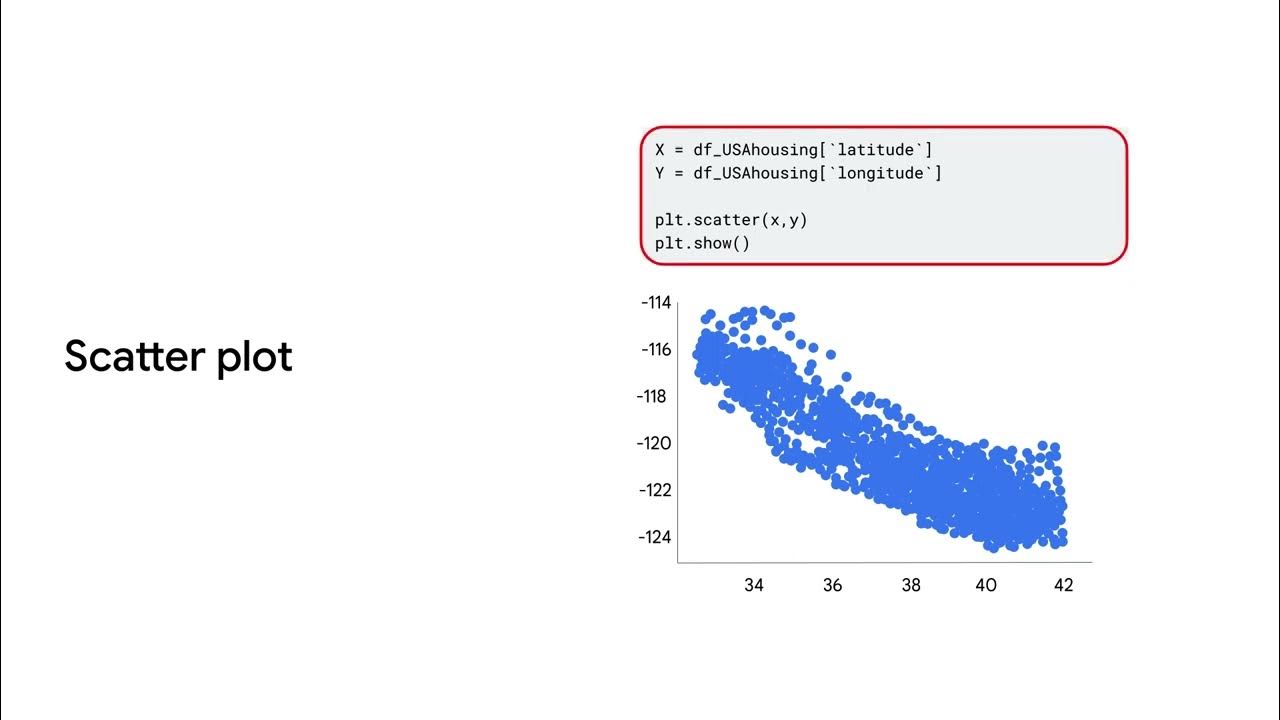
Data analysis and visualization
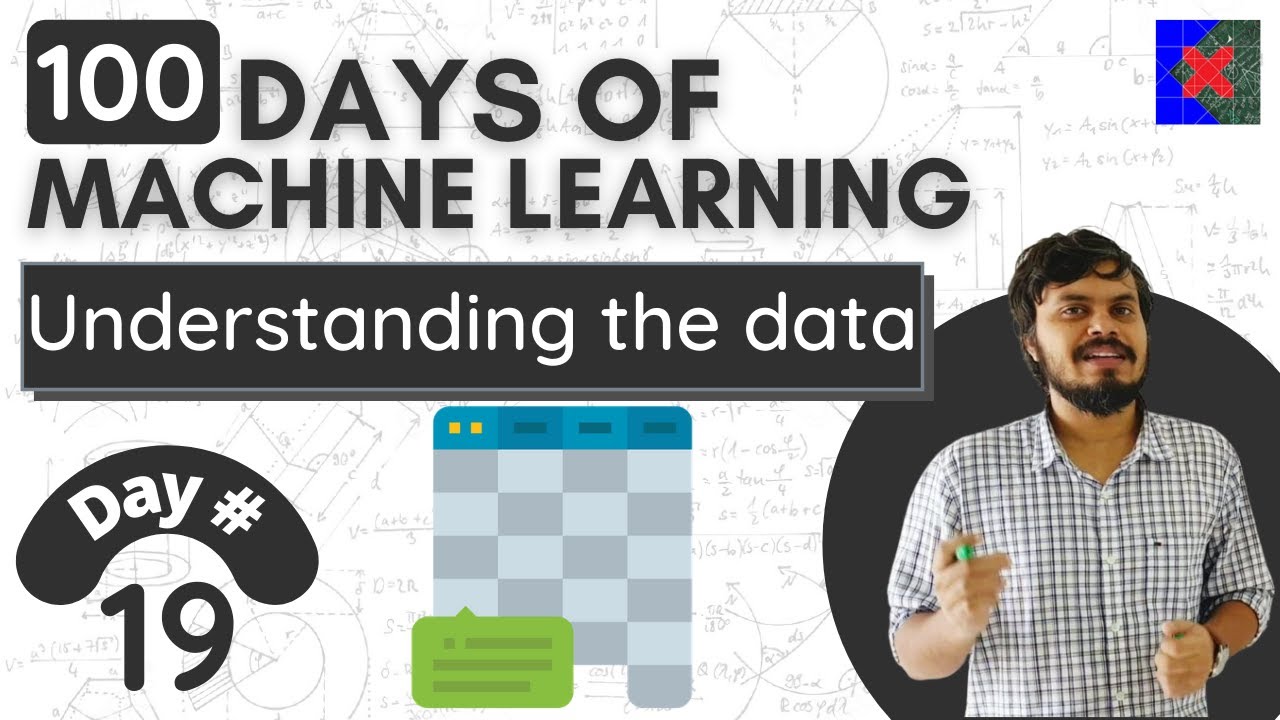
Understanding Your Data | Day 19 | 100 Days of Machine Learning
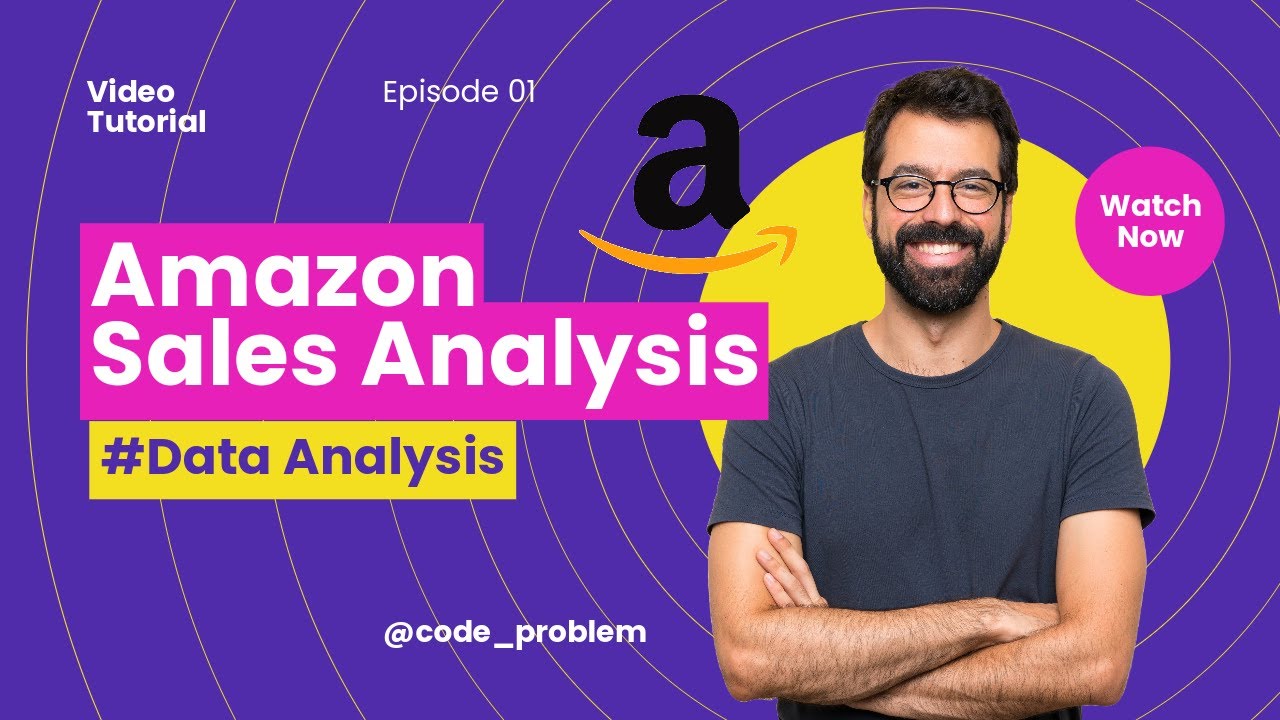
#1 Unlock The Secrets Of Data Analysis: A Comprehensive Tutorial On The Data Analysis Lifecycle
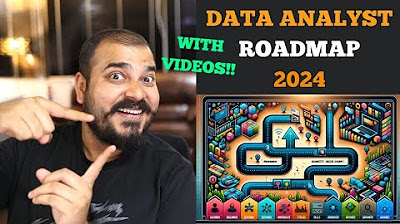
Complete Roadmap To Become Data Analyst In 2024 With Videos And Materials
5.0 / 5 (0 votes)