Demis Hassabis - Scaling, Superhuman AIs, AlphaZero atop LLMs, Rogue Nations Threat
Summary
TLDR在这次播客中,DeepMind的CEO Demis Hassabis分享了他对人工智能和神经科学的看法。他讨论了智能的本质,大型语言模型(LLMs)的学习方式,以及人工智能在科学和健康领域的应用。Hassabis强调了负责任地开发AI的重要性,并探讨了未来AI技术的潜在影响,包括对人类工作的影响和多模态交互的可能性。他还提到了DeepMind在确保AI安全方面的努力,以及他们如何通过内部检查和平衡来管理风险。
Takeaways
- 🧠 智能定义:Demis认为智能是一系列高层次通用算法主题,尽管大脑有专门化的部分。
- 📈 学习转移:大型语言模型(LLMs)在特定领域数据丰富时,会在该领域表现出不对称的改进。
- 🤖 人工智能学习:人工智能通过经验学习,类似于人类在特定领域(如棋类、创意写作)的专业化学习。
- 🧬 神经科学启示:神经科学为人工智能提供了灵感,如强化学习和注意力机制,尽管并非直接的算法映射。
- 🔍 智能分析:需要更多研究来理解这些系统构建的表示,类似于对真实大脑的fMRI或单细胞记录分析。
- 🌳 人工智能发展:尽管人工智能取得了显著进展,但在规划和构建正确世界模型方面仍有待改进。
- 🚀 计算能力:通过提高模型的准确性和可靠性,可以更有效地进行搜索,减少所需的计算资源。
- 🏆 奖励函数:在现实世界系统中定义正确的目标函数和奖励函数是一个挑战,但科学问题通常可以指定目标。
- 💡 创新与扩展:结合旧的算法思想和新的大规模模型是人工智能发展的一个重要方向。
- 🔒 安全与开放源代码:在确保安全性的同时,开放源代码可以促进科学进步,但需要平衡以防止滥用。
- 🤔 未来展望:人工智能的未来将由多个利益相关者共同决定,包括民间社会、学术界和政府等。
Q & A
Demis Hassabis认为智能的核心算法主题是什么?
-Demis Hassabis认为智能的核心算法主题是大脑如何处理我们周围的世界,尽管大脑有专门做特定事情的部分,但可能有一些基本原则支撑着所有这些。
在特定领域给予大型语言模型大量数据时,它们在该领域的性能会不对称地提升,这是否意味着我们应该在所有不同领域都看到普遍的改进?
-Demis Hassabis提到,有时在特定领域改进时,确实会在其他领域看到出人意料的改进。例如,当这些大型模型在编码方面改进时,实际上可以提高它们的一般推理能力。
Demis Hassabis认为人工智能系统中的哪种转移学习最令人惊讶?
-Demis Hassabis希望看到更多这类转移学习,例如在编码和数学方面变得更好,然后普遍提高推理能力。他认为这与人类学习者的工作方式相似。
Demis Hassabis如何看待神经科学对人工智能的启示?
-Demis Hassabis认为神经科学在过去的10-20年中为人工智能提供了很多有趣的方向性线索,例如强化学习和深度学习的结合,以及体验重放和注意力机制等概念。
Demis Hassabis如何看待从大型语言模型到具有树状搜索功能的系统的转变?
-Demis Hassabis认为这是一个非常有前途的方向。我们需要不断提高大型模型的准确性,使它们成为更可靠的世界模型,并在此基础上研究类似AlphaZero的规划机制。
Demis Hassabis如何看待计算资源的高效利用?
-Demis Hassabis认为摩尔定律有所帮助,每年都会有更多的计算资源。但他强调重点是样本高效的方法和重用现有数据,例如体验重放,以及寻找更高效的搜索方式。
Demis Hassabis如何看待人工智能的目标函数和奖励函数的设定?
-Demis Hassabis指出,为现实世界系统定义正确的目标函数和奖励函数是非常困难的。他认为这是一个挑战,需要以一种既一般又足够具体的方式来指定它们,以便正确指引系统的方向。
Demis Hassabis如何看待人类智能与人工智能的规划和搜索能力?
-Demis Hassabis认为人类大脑并不适合进行蒙特卡洛树搜索,而是使用直觉、知识和经验来构建非常准确的物理模型,包括心理模拟。他认为这与AlphaGo等人工智能系统规划下一步的方式不同。
Demis Hassabis对于强化学习在解决数据瓶颈问题上的乐观态度基于什么?
-Demis Hassabis认为还有更多的数据可以使用,尤其是如果我们将多模态和视频等视为数据来源。他看到了通过模拟、自我对弈等方法创造合成数据的空间,这在AlphaGo和AlphaZero中已经得到了很好的应用。
Demis Hassabis如何看待人工智能的未来发展和可能的时间线?
-Demis Hassabis没有给出具体的时间预测,但他表示,当他在2010年创办DeepMind时,他们将其视为一个20年的项目,并且他们现在仍在正确的轨道上。他不会对在未来十年内看到类似AGI的系统感到惊讶。
Demis Hassabis如何看待人工智能系统的安全和控制问题?
-Demis Hassabis认为,为了确保这些系统是可理解且可控的,我们需要更多的理解。他提到了一些可能的解决方案,如更严格的评估系统、使用专门的AI工具来帮助分析和总结更广泛系统的活动,并创建硬化的沙箱或模拟环境来进行实验。
Outlines
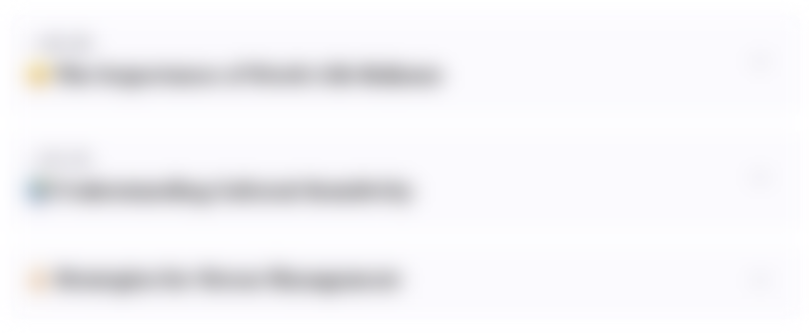
This section is available to paid users only. Please upgrade to access this part.
Upgrade NowMindmap
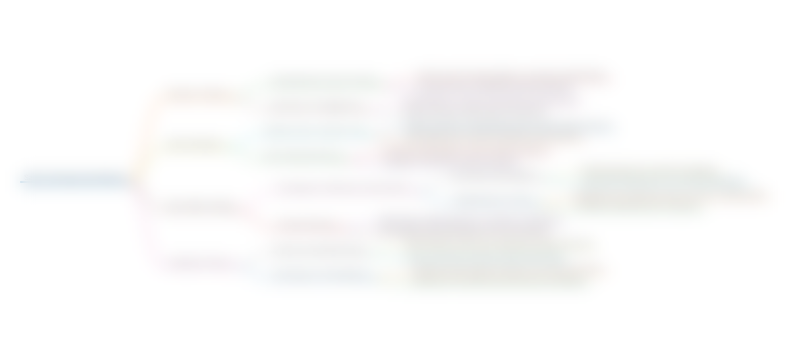
This section is available to paid users only. Please upgrade to access this part.
Upgrade NowKeywords
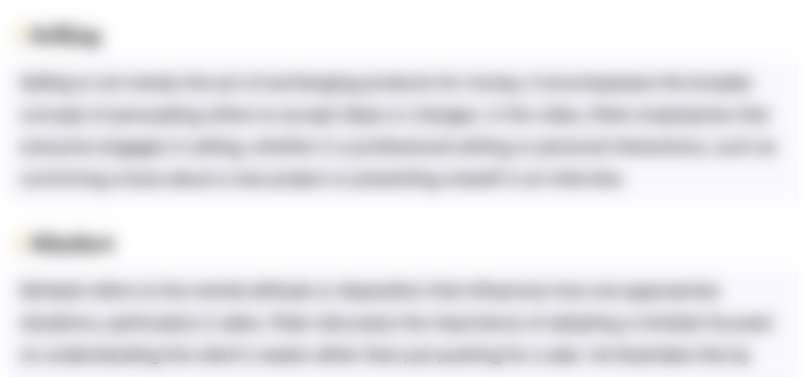
This section is available to paid users only. Please upgrade to access this part.
Upgrade NowHighlights
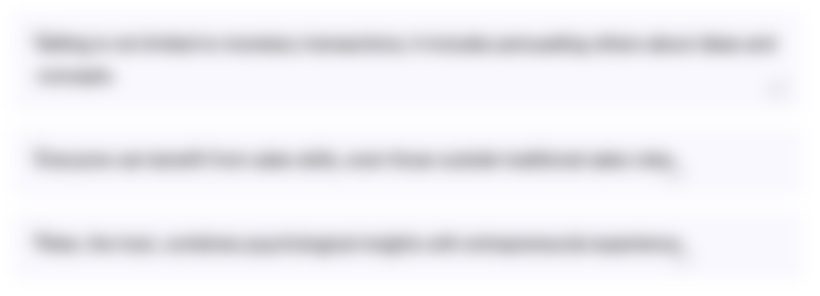
This section is available to paid users only. Please upgrade to access this part.
Upgrade NowTranscripts
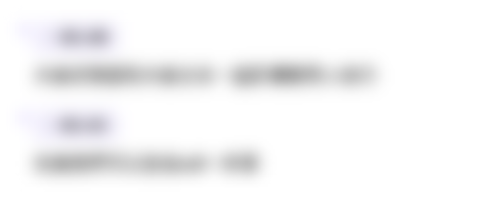
This section is available to paid users only. Please upgrade to access this part.
Upgrade NowBrowse More Related Video
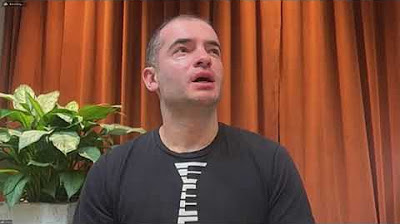
Interview with Dr. Ilya Sutskever, co-founder of OPEN AI - at the Open University studios - English
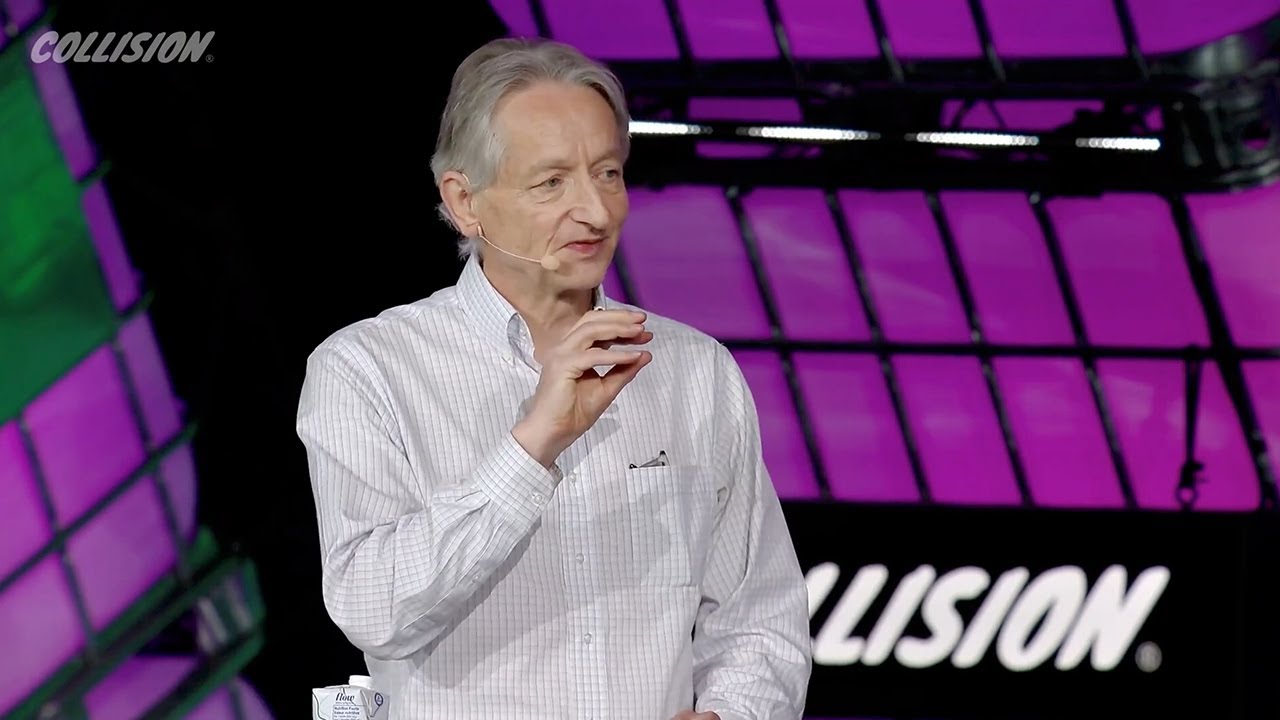
In conversation with the Godfather of AI
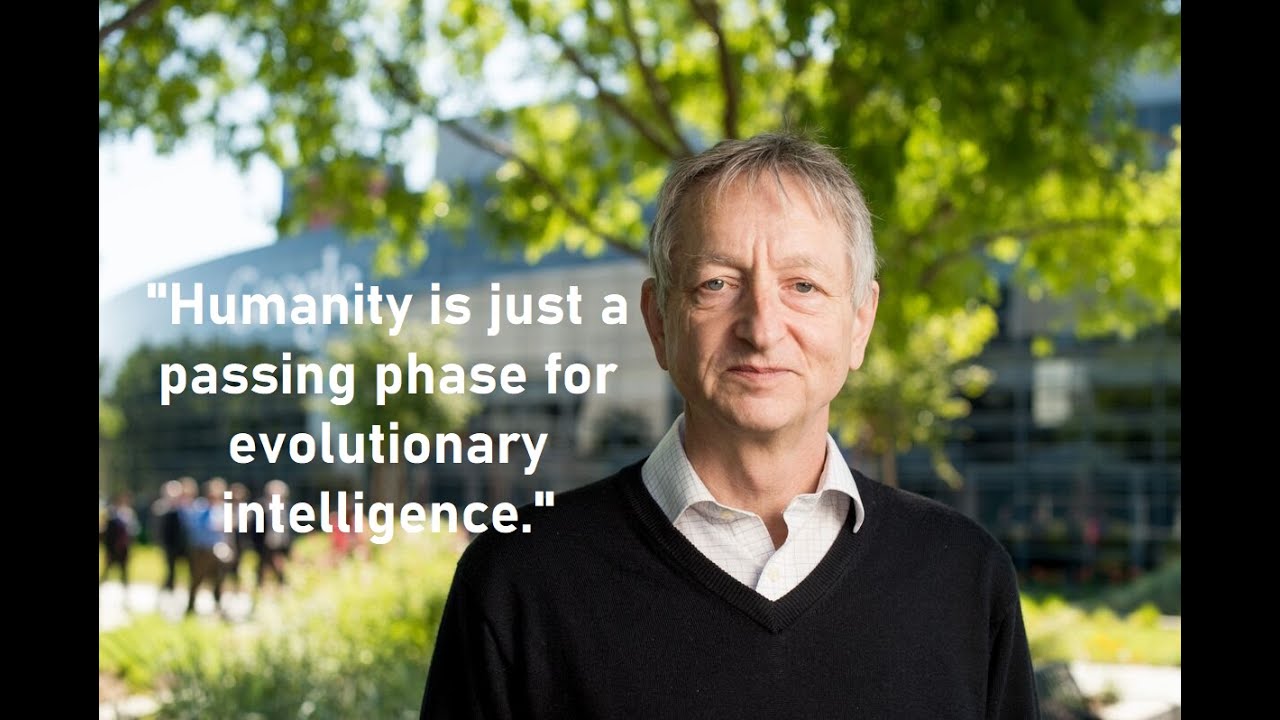
Possible End of Humanity from AI? Geoffrey Hinton at MIT Technology Review's EmTech Digital
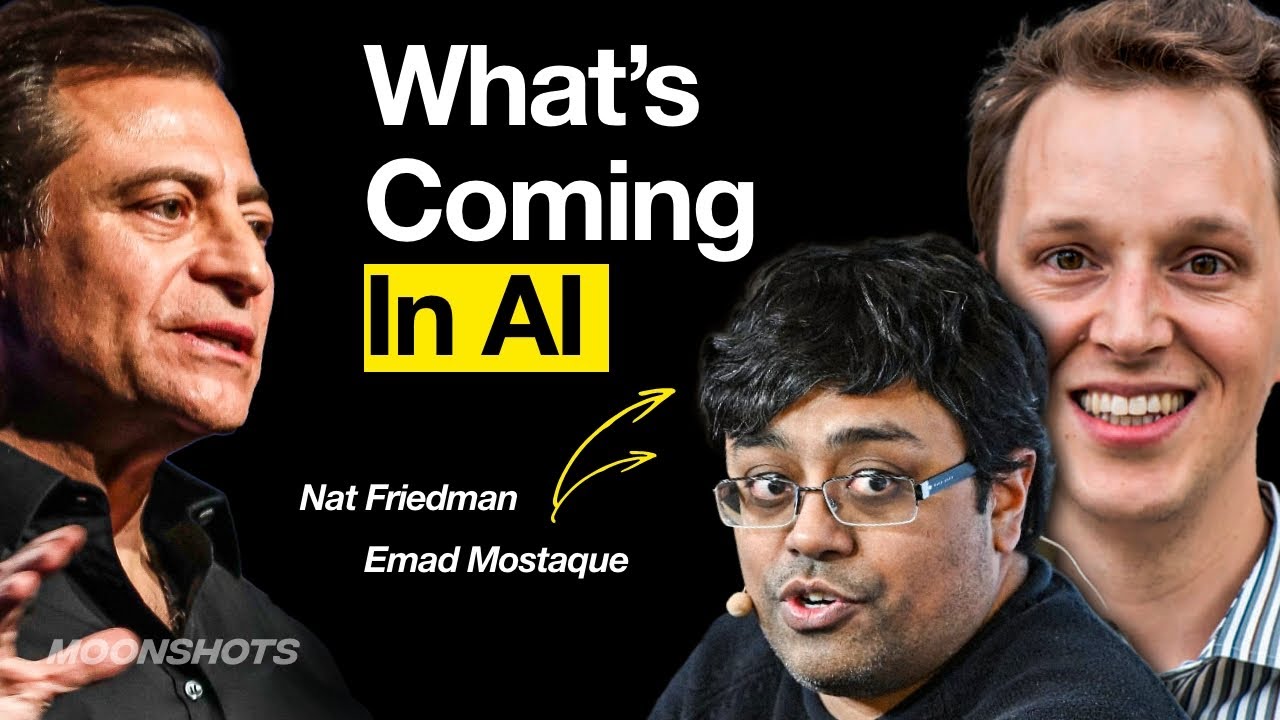
2 Ex-AI CEOs Debate the Future of AI w/ Emad Mostaque & Nat Friedman | EP #98
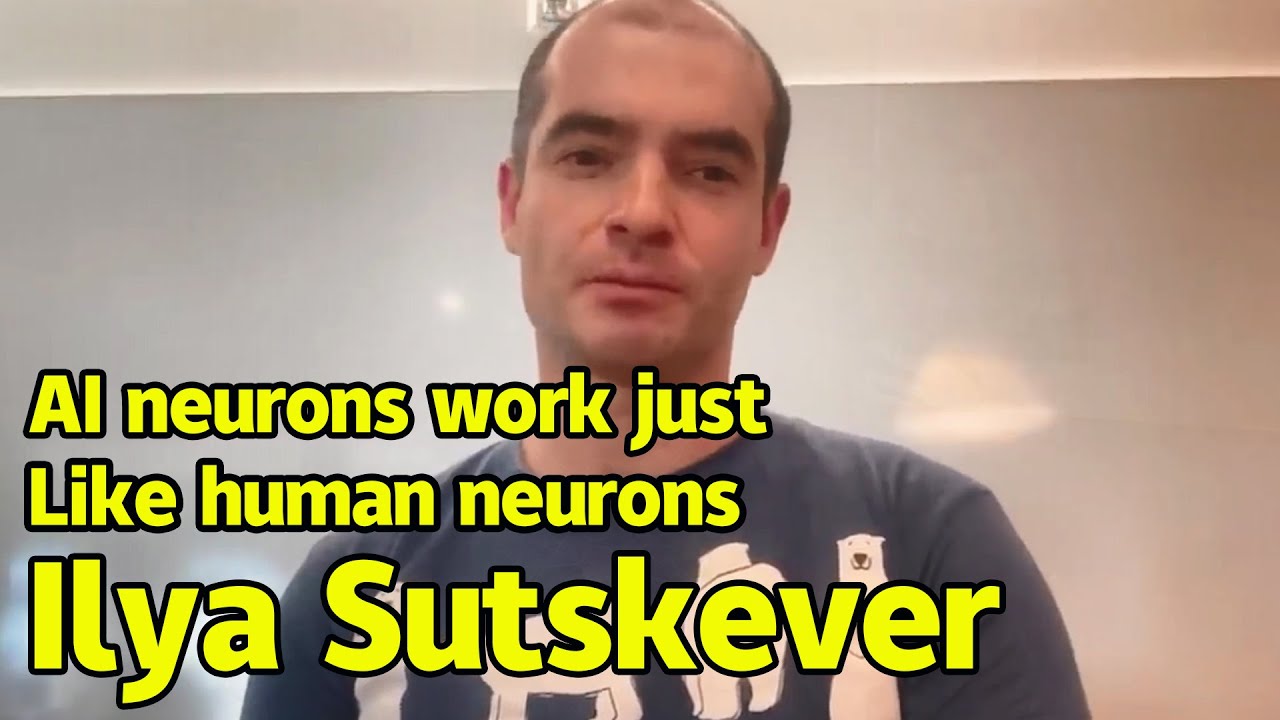
Ilya Sutskever | AI neurons work just like human neurons | AGI will be conscious like humans
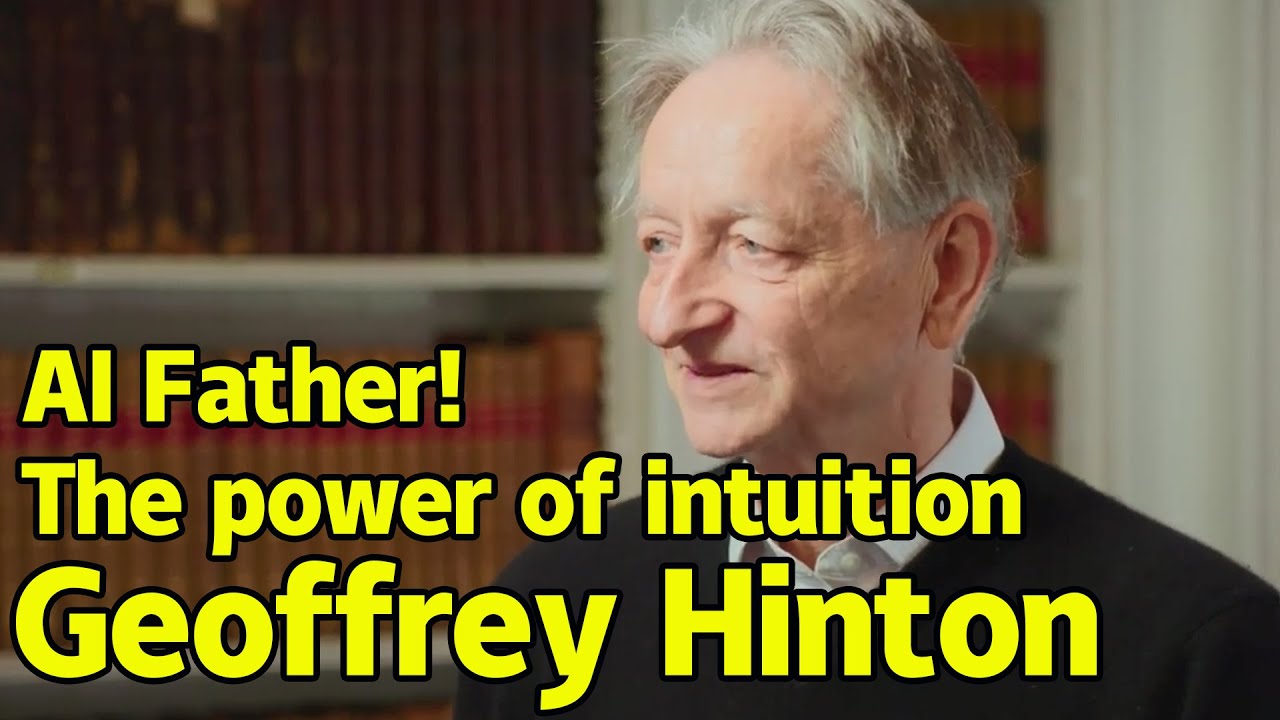
Geoffrey Hinton | Ilya's AI tutor Talent and intuition have already led to today’s large AI models
5.0 / 5 (0 votes)