Interview with Dr. Ilya Sutskever, co-founder of OPEN AI - at the Open University studios - English
Summary
TLDR本次访谈中,OpenAI联合创始人兼首席科学家Ilya Sutskever分享了他的个人旅程和对人工智能、机器学习和开放AI的看法。他强调了开放AI的目标是确保人工智能技术的发展能够造福全人类,并讨论了大规模神经网络的发展历程,以及AI对未来教育的潜在影响。此外,他还提到了人工智能领域未来可能的发展方向,包括AI在经济中的进一步整合,以及面对超级智能时的挑战和机遇。
Takeaways
- 🎓 Shai Solomon 是美国以色列开放大学之友的董事会成员,同时也是checkpoint软件技术公司的全球网络安全人才发展总监。
- 👩🏫 Dr Ellie Shai 是开放大学以色列分校的助理教授,也是神经和生物形态工程实验室的主要调查员。
- 🤖 Ilya Sutskever 是机器学习领域的著名科学家,OpenAI的联合创始人兼首席科学家。
- 🏫 采访讨论了与以色列技术、世界以及开放大学相关的问题,强调了开放对话和听取世界领导者的第一手观点的重要性。
- 📚 Ilya Sutskever 在开放大学的学习经历,以及他对人工智能领域的兴趣起源。
- 🌐 OpenAI 的成立初衷是为了让AI技术更好地造福人类,以及对通用人工智能(AGI)的认真对待。
- 🔍 Ilya Sutskever 认为,深度学习技术的发展和信念是OpenAI成立的关键因素。
- 🧠 神经网络的发展历程,从早期的人工神经元概念到现在的大规模神经网络,如GPT-3和GPT-4。
- 📈 AI领域的未来发展趋势,包括短期内AI的能力和应用的增强,以及长期面对超智能AI的挑战。
- 🏫 教育领域的未来,特别是高等教育和AI工具在教育中的应用,以及它们对课程和教育生态系统的影响。
- 🌟 开放大学对以色列社会和创新经济的贡献,以及它在提高以色列人口技能和创新能力方面的关键作用。
Q & A
Shai Solomon 是哪个组织的董事会成员?
-Shai Solomon 是美国以色列开放大学之友(American Friends of the Open University of Israel)的董事会成员。
Ilya Sutskever 在 OpenAI 中担任什么职位?
-Ilya Sutskever 是 OpenAI 的联合创始人兼首席科学家。
Ilya Sutskever 为何对开放大学心怀感激?
-Ilya Sutskever 对开放大学心怀感激,因为他在没有高中文凭的情况下,从八年级开始就能在开放大学上课,这为他提供了学习的机会。
Ilya Sutskever 认为人工智能的未来会如何发展?
-Ilya Sutskever 认为人工智能的未来将涉及更大规模、更复杂的工程项目,需要更多的工程师合作完成,而且他预见到人工智能将朝着通用人工智能(AGI)的方向发展。
OpenAI 成立的主要目的是什么?
-OpenAI 成立的主要目的是推动人工智能的发展,并确保这项技术能够尽可能地惠及全人类。
Ilya Sutskever 是如何描述深度学习技术的发展的?
-Ilya Sutskever 描述深度学习技术的发展是一个长期的过程,从1940年代的人工神经元概念开始,经历了多次重要的技术突破,包括反向传播算法的发明,以及后来的大规模神经网络的成功应用。
Ilya Sutskever 认为未来教育会如何变化?
-Ilya Sutskever 认为未来教育将会出现非常优秀的人工智能辅导老师,他们会帮助学生解答问题并提供个性化的教学,这将对教育体系产生深远的影响。
Ilya Sutskever 对于人工智能的长期发展有何预测?
-Ilya Sutskever 预测,在长期发展中,我们将面临人工智能变得比人类更聪明的挑战,这将需要全球范围内的规则、标准和协调来确保我们能够安全地享受人工智能带来的未来。
开放大学在以色列社会中扮演着怎样的角色?
-开放大学是以色列最大的高等教育机构,它通过教育大量高技能人才,对以色列的创新经济产生了巨大的正面影响,并且通过为来自社会边缘和地理偏远地区的学生提供教育机会,帮助解决了以色列的一些人口和社会挑战。
Ilya Sutskever 认为未来的工作会有什么变化?
-Ilya Sutskever 认为未来的工作将会发生变化,因为人工智能的发展将改变许多任务和活动的性质。他认为成为一个能够快速学习新事物、具有多面性和能够舒适使用人工智能工具的通才将变得非常重要。
Ilya Sutskever 为何认为深度学习的研究在过去十年主要是关于信念而非发明新事物?
-Ilya Sutskever 认为,深度学习的研究在过去十年主要是基于对这项技术能够实现预期目标的信念,而不仅仅是发明新事物。这是因为深度学习技术已经展示出了巨大的潜力,研究者们相信通过持续的研究和开发,这项技术能够带来突破性的进展。
Outlines
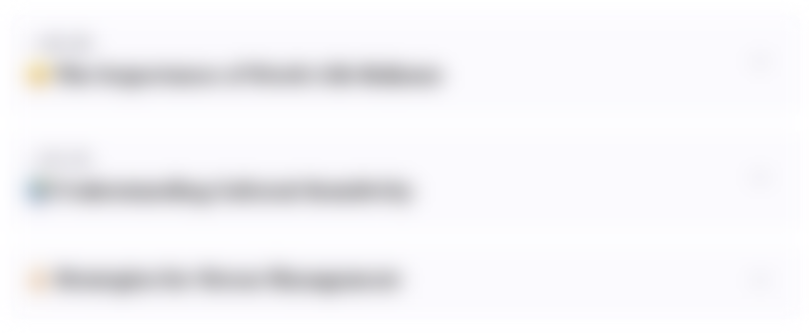
This section is available to paid users only. Please upgrade to access this part.
Upgrade NowMindmap
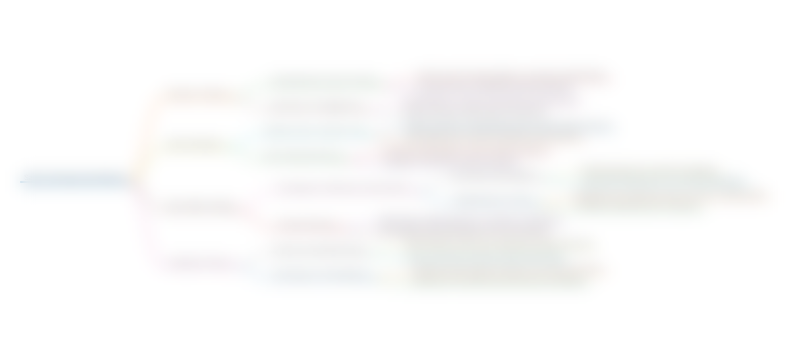
This section is available to paid users only. Please upgrade to access this part.
Upgrade NowKeywords
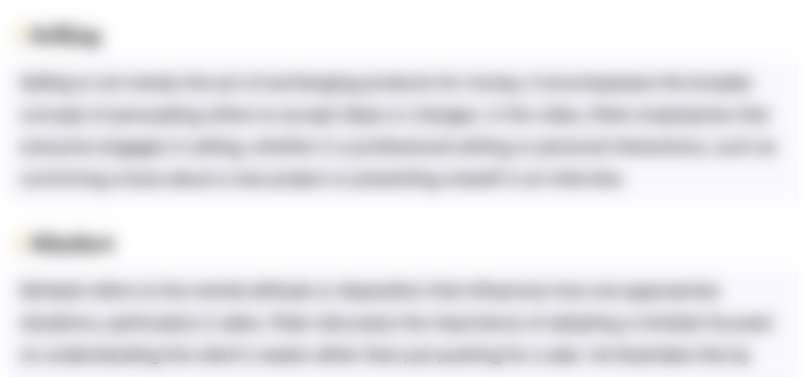
This section is available to paid users only. Please upgrade to access this part.
Upgrade NowHighlights
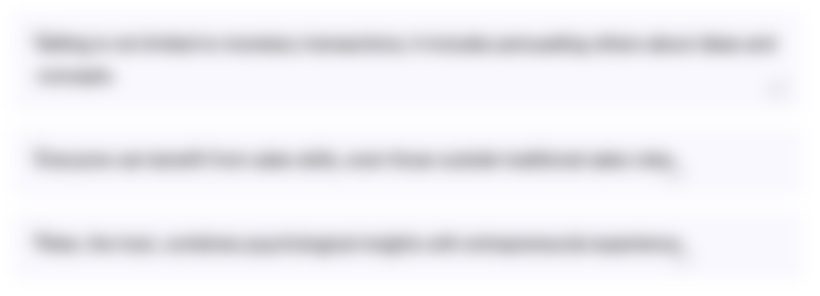
This section is available to paid users only. Please upgrade to access this part.
Upgrade NowTranscripts
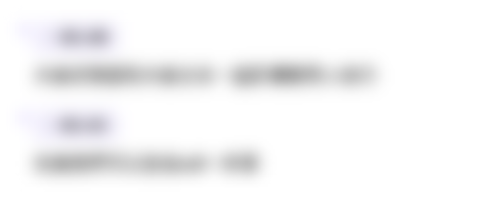
This section is available to paid users only. Please upgrade to access this part.
Upgrade NowBrowse More Related Video
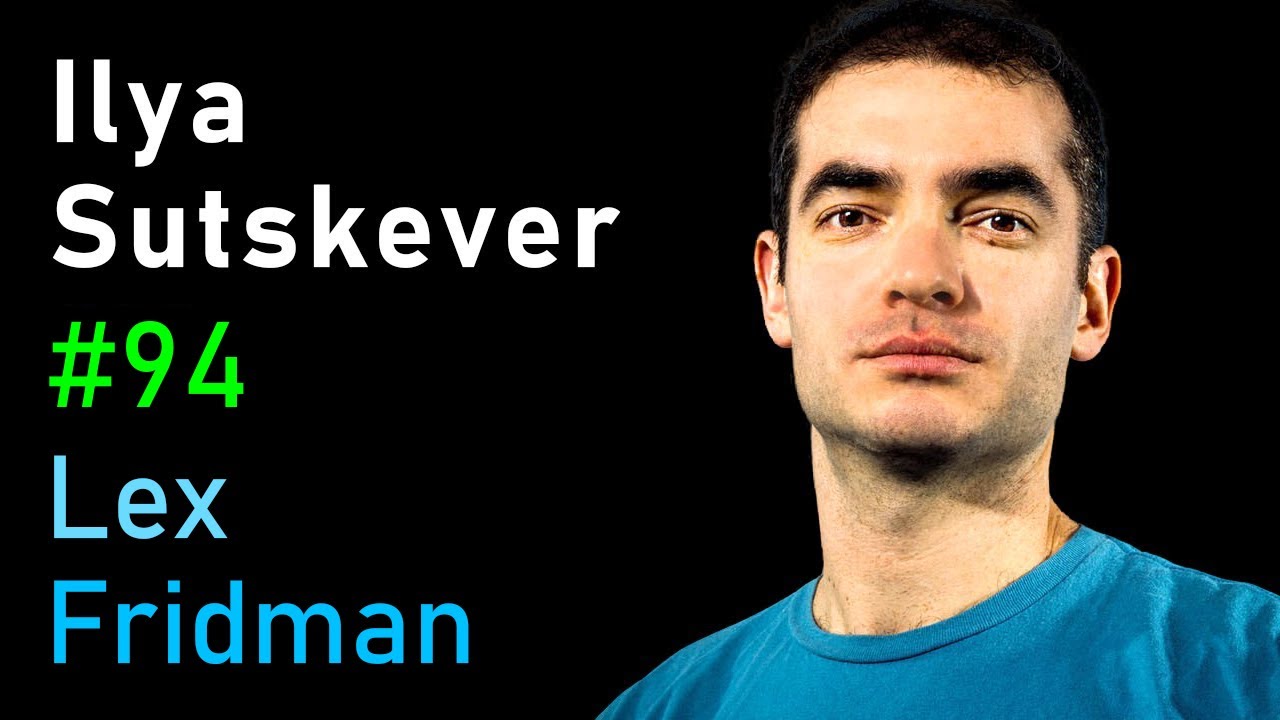
Ilya Sutskever: Deep Learning | Lex Fridman Podcast #94
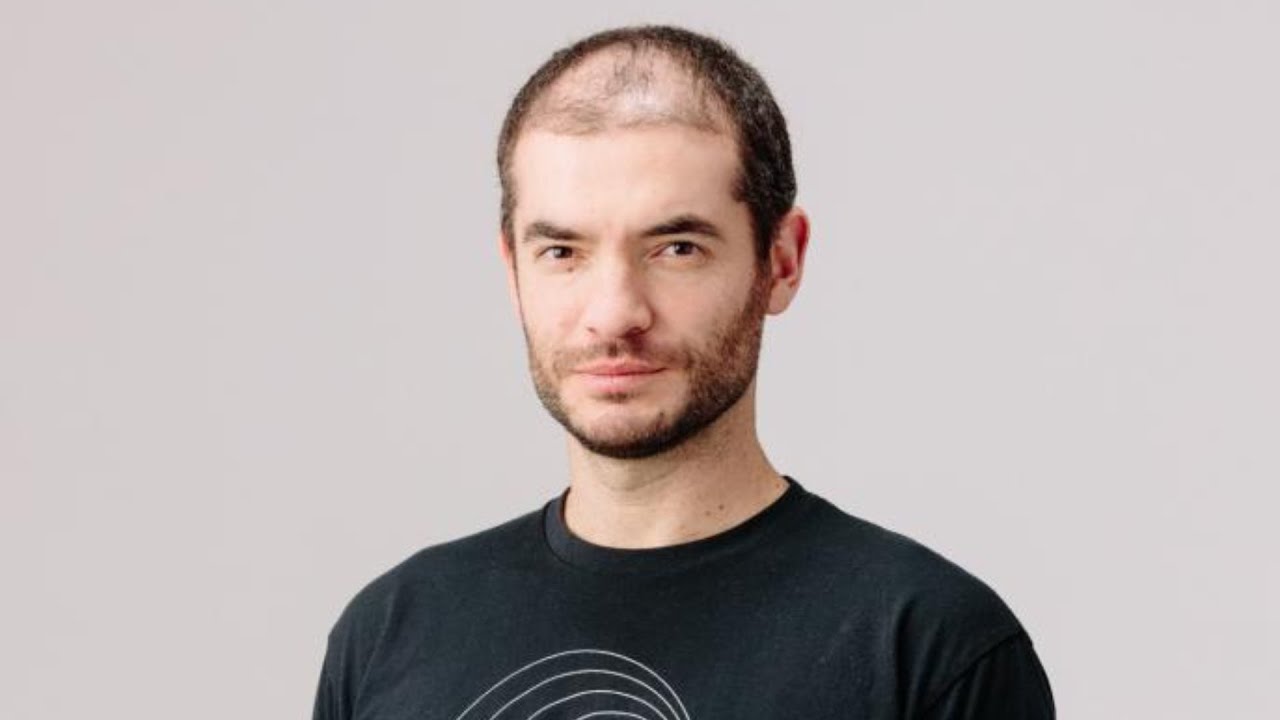
Inside OpenAI [Entire Talk]
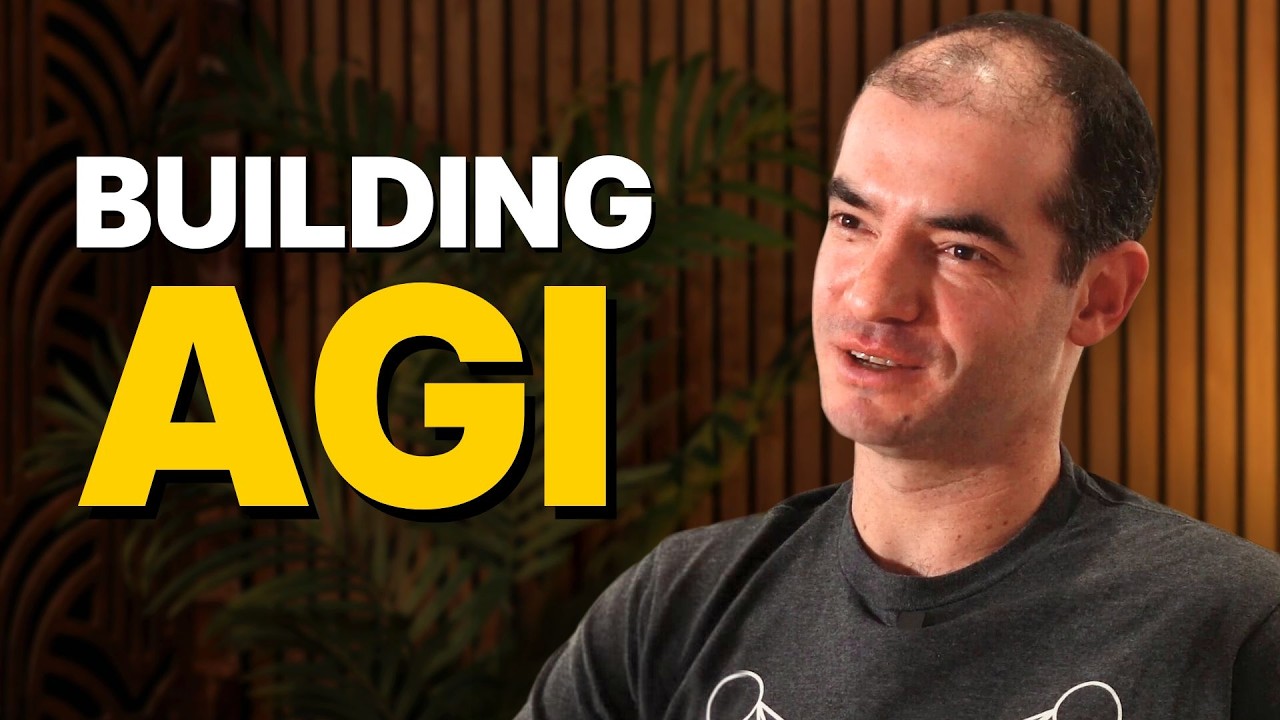
Ilya Sutskever (OpenAI Chief Scientist) - Building AGI, Alignment, Spies, Microsoft, & Enlightenment
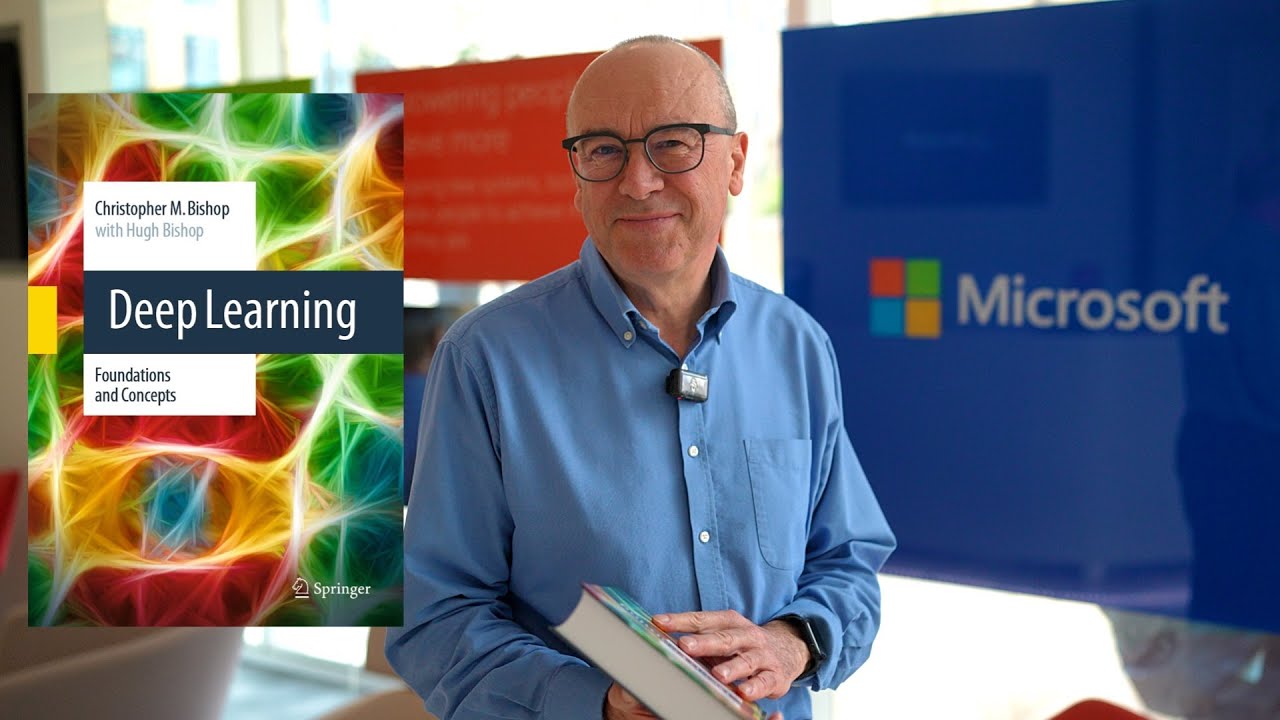
Prof. Chris Bishop's NEW Deep Learning Textbook!

Heroes of Deep Learning: Andrew Ng interviews Geoffrey Hinton
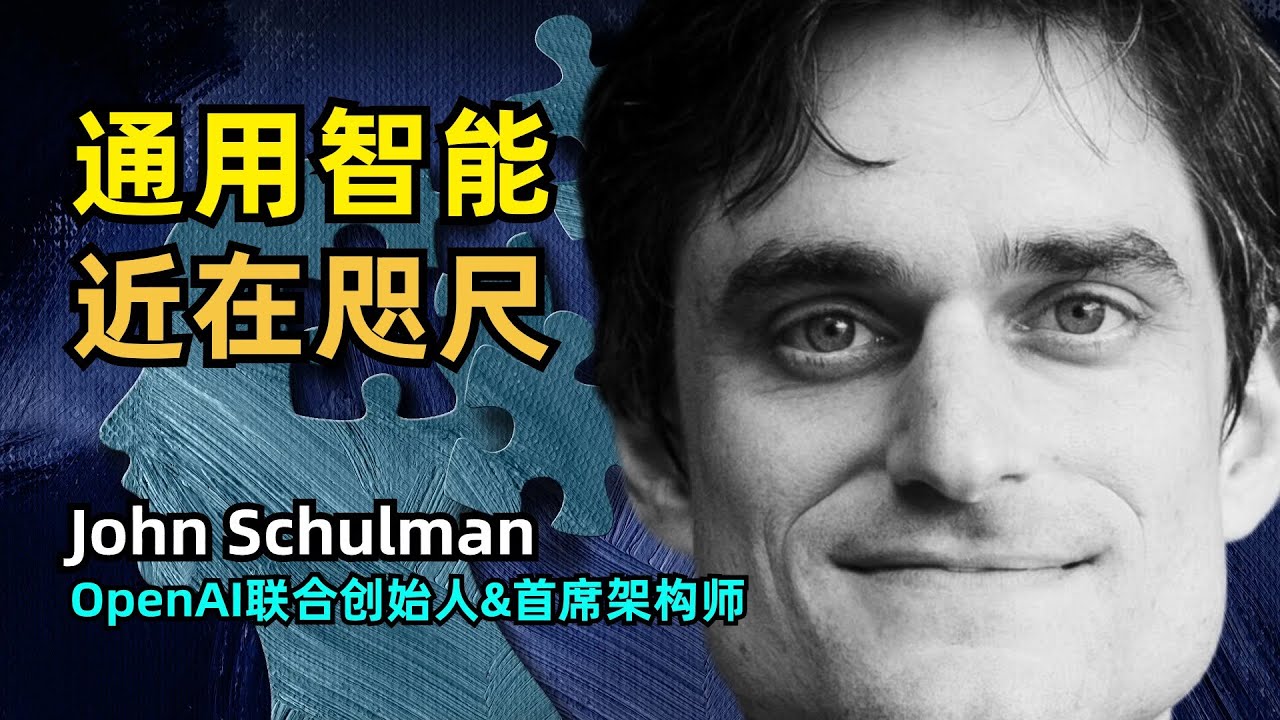
【人工智能】2027年将会实现AGI | 约翰·舒尔曼最新访谈 | OpenAI联合创始人&首席架构师 | 大模型训练 | RLHF | 技术瓶颈 | 模型蒸馏 | AI监管
5.0 / 5 (0 votes)