Prof. Chris Bishop's NEW Deep Learning Textbook!
Summary
TLDR本次访谈中,我们有幸与人工智能和机器学习领域的杰出人物克里斯·毕晓普教授进行对话。克里斯是微软研究院的技术院士和剑桥科学人工智能主任,同时担任爱丁堡大学计算机科学的名誉教授和达尔文学院的院士。他还是《深度学习基础与概念》一书的合著者,该书是他与儿子休一起出版的。克里斯分享了他在深度学习领域的研究心得,包括他对不变性的看法以及如何从大量论文中提炼出核心概念。此外,克里斯还讨论了他在量子场理论方面的博士研究,以及他如何从理论物理学家转变为全职神经网络研究者的经历。他强调了概率理论在机器学习中的基础性作用,并分享了他对于神经网络和深度学习未来发展的看法。
Takeaways
- 📚 教授Chris Bishop是人工智能和机器学习领域的杰出人物,微软研究院的技术研究员和剑桥AI for Science的主任。
- 🎓 Chris Bishop与他的儿子Hugh合著了一本关于深度学习基础和概念的新书。
- 🌟 Chris在2004年被选为英国皇家工程院院士,并在2007年和2017年分别被选为爱丁堡皇家学会和英国皇家学会的院士。
- 📈 他在微软研究院负责全球工业研究和开发组合,特别关注机器学习和自然科学。
- 🔍 Chris解释了在写书时如何选择包含哪些内容,强调了从核心概念中提炼的重要性。
- 💡 他讨论了深度学习领域的重要概念,如概率、梯度方法等,并强调了这些概念的持久价值。
- 🌐 Chris提到了他对于神经网络和机器学习对自然科学,包括物理学的影响的看法。
- 📖 他分享了自己从理论物理学家转变为神经网络研究者的个人经历。
- 🤖 他讨论了人工智能的未来,特别是神经网络和机器学习如何增强人类的创造力和认知能力。
- 🔑 他强调了机器学习中的贝叶斯框架的重要性,并讨论了实践中的近似方法。
- 🚀 Chris对于人工智能技术的未来发展持乐观态度,认为我们正处于一个新时代的开始。
Q & A
Chris Bishop教授在人工智能和机器学习领域的主要贡献是什么?
-Chris Bishop教授是机器学习领域的杰出人物,他是微软研究院的技术研究员和主任,专注于AI在科学研究中的应用。他还是爱丁堡大学计算机科学的名誉教授和达尔文学院的研究员。他的主要贡献包括编写了机器学习领域的重要教材《Pattern Recognition and Machine Learning》(简称PRML),并与其子Hugh共同撰写了新书《Deep Learning Foundations and Concepts》。
Chris Bishop教授的学术背景和研究领域有哪些?
-Chris Bishop教授在牛津大学获得了物理学学士学位,并在爱丁堡大学获得了理论物理学博士学位,博士论文主题是量子场论。他的研究领域包括机器学习和自然科学研究,特别是深度学习的基础和概念。
Chris Bishop教授在微软研究院的职责是什么?
-在微软研究院,Chris Bishop教授负责监督全球范围内的工业研究和开发工作,特别关注机器学习和自然科学。他领导的团队致力于将机器学习技术应用于科学研究,推动科学发现的进程。
Chris Bishop教授对于深度学习模型的哪些特性感到自豪?
-Chris Bishop教授对于他与儿子共同编写的新书《Deep Learning Foundations and Concepts》中的生产价值感到自豪。他们与出版商紧密合作,确保书籍的物理质量高,特别是采用了称为缝合签名的印刷技术,使得书籍可以平整地打开,便于阅读且耐用。
Chris Bishop教授如何看待深度学习模型在科学发现中的应用?
-Chris Bishop教授认为深度学习模型在科学发现中的应用是最令人兴奋的前沿领域。他认为,将机器学习和人工智能应用于科学研究是最重要的应用之一,因为这将极大地加速我们进行科学发现的能力。
Chris Bishop教授对于深度学习模型的未来发展有何看法?
-Chris Bishop教授认为深度学习模型的未来发展前景广阔,他强调了Transformer架构的重要性,并表示深度学习模型的成功部分归功于其能够从大量数据中提取规则和模式。他预测,尽管Transformer架构非常成功,但未来还会有新的架构出现。
Chris Bishop教授如何看待人工智能的创造性?
-Chris Bishop教授认为人工智能系统具有创造性,尽管它们是由人类创造和设计的。他指出,人工智能系统的创造力是建立在人类知识和经验之上的,通过学习和实践,人工智能可以发展出新的思考方式和创新能力。
Chris Bishop教授对于人工智能的安全性和道德问题有何看法?
-Chris Bishop教授强调,我们需要创造对人类有益的技术,并确保人工智能系统的安全性和道德性。他提到,虽然在发展过程中可能会出现一些挑战和错误,但总体上,我们正在朝着正确的方向发展,越来越多的关注和努力正在投入到理解人工智能的潜在风险,并采取措施来减轻这些风险。
Chris Bishop教授如何看待神经网络在模式识别中的作用?
-Chris Bishop教授认为神经网络在模式识别中扮演了强大的工具角色。他的第一本书《Neural Networks for Pattern Recognition》就强调了神经网络在这一领域的重要作用,并推动了神经网络作为机器学习的强大工具的普及。
Chris Bishop教授在科学研究中使用深度学习的一个具体例子是什么?
-Chris Bishop教授在科学研究中使用深度学习的一个具体例子是他在聚变研究中的工作。他和他的团队使用神经网络实现了对高温等离子体形状的实时反馈控制,这是通过训练一个神经网络模拟器来完成的,该模拟器能够根据磁场测量预测等离子体的形状。
Chris Bishop教授对于深度学习模型的泛化能力有何看法?
-Chris Bishop教授认为深度学习模型的泛化能力非常出色,但他同时也指出这是一个开放的问题,我们还需要进一步研究为什么这些看似过参数化的模型能够如此良好地泛化。他提到,尽管我们可以描述模型并对其有很多了解,但为什么它们能够如此有效地工作仍是一个需要深入研究的问题。
Outlines
📚 与人工智能领域的杰出人物Chris Bishop教授的对话
本段落介绍了与人工智能领域著名学者Chris Bishop教授的对话。Chris是微软研究院AI for Science in Cambridge的技术研究员和主任,同时担任爱丁堡大学计算机科学的名誉教授和达尔文学院剑桥的研究员。他与儿子Hugh合著了一本关于深度学习基础和概念的新书,并讨论了他们在书中特别强调的生产价值和书籍的物理质量。Chris分享了他对于机器学习和人工智能领域的重大贡献,包括他所著的《Pattern Recognition and Machine Learning》(PRML)教科书,以及他对神经网络和机器学习的热情。
🌟 Chris Bishop教授的职业转变与研究领域
在这一段中,Chris Bishop教授回顾了他的职业生涯,从量子场理论的理论研究转向对核聚变的兴趣,并最终投身于神经网络和机器学习领域。他分享了自己是如何被Geoff Hinton的反向传播论文所启发,并开始使用神经网络处理来自聚变项目的数据。Chris还讨论了他对于深度学习在自然科学研究中潜在影响的兴奋,包括物理学。此外,他提到了与GPT-4相关的个人经历,以及他对人工智能未来发展的看法。
🤖 人工智能与符号主义的融合
在这一部分中,讨论了人工智能领域中连接主义和符号主义的融合。Chris提到了自2012年以来深度学习的显著成功,以及人们对于结合这两种方法的讨论。他指出,像GPT-4这样的模型已经能够进行更高级别的推理,显示出神经网络的能力正在扩展。Chris认为,神经网络的发展类似于人类大脑,能够处理各种不同类型的推理和智能。他还讨论了对于神经网络未来可能性的看法,强调了神经网络的能力和进步速度。
📖 创作《模式识别与机器学习》的动机
Chris Bishop分享了他创作《模式识别与机器学习》(PRML)这本书的动机和思考过程。他提到,这本书的初衷是为了提供一个全面且易于理解的机器学习领域的学习资源。Chris强调了概率理论在机器学习中的基础性作用,并讨论了在实践中应用贝叶斯框架的局限性。他还提到了大规模神经网络相对于贝叶斯方法的优势,以及如何通过训练更大的网络来提高效率。Chris认为,尽管贝叶斯方法在某些领域仍有其应用,但在主流机器学习中,重点是规模和使用如随机梯度下降等方法。
🧠 深度学习模型的本质与能力
在这一段中,Chris探讨了深度学习模型的本质,特别是大型语言模型如GPT-4。他强调了这些模型的多功能性和它们如何超越特定任务的能力。Chris提到了模型的通用性,以及它们如何在输入敏感的方式下激活不同的部分。他还讨论了大型语言模型是否应该被视为单一模型或多个模型的集合,并分享了他对于模型作为推理引擎的看法。Chris提出了关于模型能力和专业化的问题,以及大型语言模型在科学发现中的潜在作用。
💡 人工智能的创造性与未来
Chris Bishop讨论了人工智能的创造性,以及它们是否能够创造出新的东西。他提出了一个观点,即尽管人工智能系统是由人类创造的,但这并不意味着它们本质上不具有创造性。Chris强调了人工智能系统如何依赖人类的创造力和以往的工作,并认为这些系统增加了人类创造力的总和。他还讨论了人工智能系统如何作为辅助工具增强人类的创造力,并提出了关于人工智能未来发展的一些思考。
📖 创作新书《深度学习基础与概念》
在这一段中,Chris谈到了他与儿子Hugh合著的新书《深度学习基础与概念》的创作过程。他分享了决定包含和省略书中内容的思考过程,以及他们如何专注于提炼出对理解深度学习领域至关重要的核心概念。Chris还提到了他们在创作过程中遇到的挑战,以及他们如何努力在保持书籍紧凑的同时,确保其内容的质量和深度。
🌐 AI for Science的愿景与实践
Chris介绍了他在微软研究院领导的AI for Science倡议。他强调了科学发现对人类进步的重要性,并讨论了深度学习和人工智能如何加速这一过程。Chris提到了机器学习模型作为模拟器的潜力,以及它们如何能够显著加快复杂数值模拟的速度。他还提到了AI for Science团队的多元化和多学科背景,以及他们对于利用人工智能推动科学发现的热情。
🧬 深度学习在药物发现中的应用
Chris讨论了深度学习在药物发现中的应用,特别是在寻找针对特定疾病的目标和候选药物分子方面。他描述了药物发现过程的挑战,包括如何从巨大的分子空间中筛选出具有潜在治疗效果的候选药物。Chris提到了利用深度学习模型来生成和筛选候选药物分子的方法,并分享了他们在结核病治疗方面的具体工作案例。他还强调了与领域专家合作的重要性,以及如何将深度学习模型与实验数据结合起来以推进药物发现。
🌟 深度学习的未来与挑战
在这段对话的最后部分,Chris和主持人探讨了深度学习的未来,包括对于模型架构的持续研究和改进,以及深度学习在控制和规划问题中的潜在应用。Chris提到了深度学习领域的一些开放性问题,例如为什么深度学习模型能够如此有效地泛化,以及如何更好地理解这些模型的工作原理。他还表达了对于人工智能技术未来发展的乐观态度,并强调了在这个令人兴奋的领域工作的重要性。
Mindmap
Keywords
💡人工智能
💡深度学习
💡机器学习
💡神经网络
💡模式识别
💡科学发现
💡概率理论
💡自然语言处理
💡核聚变
💡GPT
Highlights
Chris Bishop 教授讨论了深度学习的基础和概念,这是他与儿子 Hugh 合著的新书。
Chris 讨论了在写书时如何决定包含哪些内容,以及如何从数千篇每月发表的论文中筛选出核心概念。
Chris 提到了他们如何通过查看关键论文和专注于经得住时间考验的技术与理念,来确定书中的内容。
Chris 强调了他最喜欢的书中的图表,特别是由他的儿子 Hugh 制作的图表,包括 GPT 的 transformer 架构图。
Chris 讨论了他如何通过研究来准备书籍,包括研究关键论文和识别领域中的近期思想。
Chris 回顾了他的职业生涯,从对人工智能的兴趣出发,到深入研究量子场理论,再到完全投入到神经网络领域。
Chris 讨论了他对神经网络和机器学习对自然科学,包括物理学的影响的看法。
Chris 探讨了神经网络和深度学习技术的未来,以及它们如何推动科学发现。
Chris 讨论了他对人工智能的看法,以及他认为我们已经迈出了真正的人工智能的第一步。
Chris 讨论了他对神经网络的看法,以及他认为它们如何类似于人类大脑的工作方式。
Chris 讨论了他对于神经网络和深度学习未来发展的看法,以及他认为我们还没有达到这些技术的潜力极限。
Chris 讨论了他对于人工智能和机器学习领域的安全性、公平性和道德性的看法,以及我们如何确保这些技术对人类有益。
Chris 讨论了他对于深度学习模型的可解释性和我们如何理解它们工作方式的看法。
Chris 讨论了他对于神经网络在控制和预测方面应用的看法,以及这些技术如何被用于实际问题的解决。
Chris 讨论了他对于深度学习技术在未来的应用和发展方向的看法,以及他认为这些技术将如何继续改变我们的世界。
Transcripts
Today, we have the privilege of speaking with Professor Chris Bishop,
a luminary in the field of artificial intelligence and machine learning.
Chris is a technical fellow and director at Microsoft Research
AI for Science in Cambridge. He's also honorary professor of
computer Science at the University of Edinburgh and fellow of
Darwin College, Cambridge. Hi. Nice to meet you, Tim.
This is the new book on deep learning foundations and concepts
published with my son Hugh. What prop have you got? Ethanol.
I don't know whether I'll use it, but we're going to talk about
invariance and that's wonderful. That's wonderful because you
ought to get a little bit techie at some point, don't you?
Oh yeah. Our audience loves that. In 2004, he was elected fellow of
the Royal Academy of Engineering. In 2007, he was elected fellow of
the Royal Society of Edinburgh, and in 2017 he was elected
fellow of the Royal Society. Chris was a founding member of the
UK AI Council, and in 2019 he was appointed to the Prime Minister's
Council for Science and Technology. At Microsoft Research,
Chris oversees a global portfolio of industrial research and development
with a strong focus on machine learning and the natural sciences.
Chris obtained a BA in physics from Oxford and a PhD in
Theoretical Physics from the University of Edinburgh, with the
thesis on quantum field theory. Chris's contributions to the
field of machine learning have been truly remarkable.
He's authored one of the main textbooks in the field,
which is Pattern Recognition and Machine Learning, or Prml,
and it has served as an essential reference for countless students
and researchers around the world. Chris explained in the interview
how it steered the field towards a more probabilistic perspective at
the time, and he also mentioned his first textbook, Neural Networks for
Pattern Recognition and its role in promoting neural networks as a
powerful tool for machine learning. So this is the new textbook, Deep
Learning Foundations and Concepts. And one of the things that we
were proud of with this book is the production values.
We really worked with the publisher to to ensure the book would be
produced to a high physical quality. And in particular, it's produced with
what are called stitch signatures. So if you look down the edge there,
you'll see the pages are not simply glued in.
Instead, this uses an offset printing technique where 16 pages
are printed on a big sheet of paper on both sides, so some of
the pages are turned upside down, and then the the page of the paper
is folded and then folded and then folded again and trimmed.
And the resulting set is called a signature.
And they're actually stitched in with cord.
And the point about that is it allows the book to open flat.
So it means that the book is easy to read and it means it
should should last a long time. What are your favorite figures
in the book? Chris. Well, the ones produced by my son,
of course, are the best. I mean, here's a nice picture of
the transformer architecture, which is this is GPT, so you could
say it's one of the most important figures in the book, I suppose.
And I just love the way the way he's done this.
How did you do the research for this? So that's a great question.
I think one of the big challenges with writing a book
like this is knowing what to include and what not to include,
and with literally thousands of papers being published every month,
it can be overwhelming for the authors, never mind the readers.
So I think the value we add in the book is trying to distill out what
we think of as the core concept. So part of this was really looking at
key papers in the field, seeing what relatively recent ideas there are,
but also trying to focus down on techniques and ideas that we believe
will actually stand the test of time. We don't want this book to go
out of date in a year or two. We want it to have have lasting
value. And of course, it's quite possible
there'll be a breakthrough next week and that it will turn out to be a
very important new architecture. But for the most part,
many of the core concepts actually go back a long way.
And so what we've really done is taken some some of the foundations
of the field and brought them into the modern deep learning era.
But the idea of probability is the idea of gradient based
methods and so on. Those have been around for decades,
and they're just as applicable today as they as they ever were. Yes.
One of the things I really like, actually, is the chapter on
convolutional networks. My son Hugh did a, um,
did a lot of this chapter. He works on using, um,
techniques like convolutional neural nets as part of his work
on autonomous vehicles. And, and I think there's a
really nice, uh, description here of convolutional networks,
really from the ground up, explaining the basic concepts and,
but also motivating them, not just saying this is how a
convolutional network is built, but why is it built this way?
How do we actually motivate it? So that's one of my favorite
chapters as well. Yeah,
it's been a very interesting career. And this stage of the career,
I can now finally look back and make sense of it.
But at the time it felt like a bit of a random walk.
So actually when I was a teenager, I went to see, um,
2001 A Space Odyssey. It was actually very inspired by
that rather abstract concept of an artificial intelligence,
very different from the usual sort of Hollywood portrayal of robots.
So I was very interested in the idea of artificial intelligence from a
young age, but I was very uninspired by the field of AI at the time,
which was very much sort of rule based and, and didn't seem to be
on a path to intelligence. And then I did a PhD in quantum
field theory, which is a very hot field at the time.
Gauge field theory at Edinburgh University had a wonderful time.
At the end of my PhD, though, I wanted to do something a bit
more practical, a bit more useful. And so I entered into the fusion
program. I'm a big fan of nuclear fusion.
Um, it was sort of 30 years away then, and it's kind of still 30
years away now, but I'm still a big believer.
But I went to work on, uh, on tokamak physics, essentially
theoretical physics of, of plasmas, trying to trying to understand the
instabilities and control them. So I was working very happily as
a theoretical physicist, having a, having a great time.
And after about ten years or so as a theoretical physicist, uh,
Geoff Hinton published the backprop paper, and it came to my attention.
And I found that very inspiring, because there I saw a very,
very different approach to towards intelligence.
And so I started by applying neural networks to data from the
fusion program, because it was big data in its day.
I was I was working near to the the Jet tokamak, and they had many,
many high resolution diagnostics. So I had lots of data to play with.
And I became more and more fascinated by neural networks.
And then I did a sort of completely crazy thing.
I walked away from a very respectable career as a theoretical physicist
and went full time into the field of neural nets, which at the time
was not really a respectable field. I would say it's not wasn't
mainstream computer science. It certainly wasn't physics.
It wasn't really anything. But I just found it very inspiring.
And I was particularly inspired by the work of Geoff Hinton.
And so I've been in that field for, you know,
three and a half decades now. And of course,
recent history suggests that was probably a good career move.
Um, and now most recently, I've brought the two ends of my
career together because I'm now very excited about the impact that
neural nets and machine learning are having on the natural sciences,
including physics. Hinton is a famous connectionist,
so he believes that knowledge is subsymbolic.
And I was speaking with Nick Chaytor the other week.
He had a book called The Mind Is Flat, which is talking about the
inscrutability of of our brains. How do you feel that things have
changed? I mean,
you were talking about a convergence of these different ideas in AI.
I think one thing that's very interesting is that there has been
a lot of discussion, let's say, from 2012 onwards, when deep learning
was clearly being very successful, a lot of discussion that it was
missing the sort of symbolic approach that we somehow had to find a way
to combine this connectionist approach and to use that sort of
probably rather dated terms now. But but that sort of, you know,
that neural net approach with the more traditional symbolic approach.
And I think what we've seen with with models like GPT four, for example,
that it's perfectly capable of reasoning at a more symbolic level,
not at the level of a human being, of course, but it can do that,
that kind of more abstract, higher level reasoning.
And so I think what we what we're seeing with neural nets is
rather like the human brain. The human brain doesn't have a
connectionist neural net piece. Then some other machinery that
does symbolic reasoning that that same substrate is capable
of all of these different kinds of reasoning and these different
kinds of intelligence. And we're starting to see that
emerge now with neural nets. So I think, I think for me,
the discussion of should we somehow combine symbolic.
Reasoning with, with connectionism, know that that
to me that's a piece of history. It's about how can we how can we
expand on the capabilities of neural nets. Yeah. That's so interesting.
I remember there was a paper by Pylyshyn.
I think it was the connectionist critique in 1988,
and I was quite sold on this idea of, you know, systematicity and
productivity and so on. And even now, folks from that school
of thought think that our brains are Turing machines, this ability
to address potential infinity. And I guess what I'm what I'm
getting from, from what you're saying is that the distinction
isn't really there anymore. You can do that kind of
reasoning with neural networks. Well, I take a very simple view,
which is that neural nets in since 2012, in particular,
have been shown to be spectacularly capable and there's no end in sight.
The rate of progress is faster now than ever, so it seems very straight.
Nobody imagines that that machine learning and deep learning has
suddenly ended at, you know, whatever the time is today.
You know, this is this is this is the beginning of an S curve.
So the idea that we would worry so much about the limitations of neural
networks and what they can't do, I think we just put the word.
Yet at the end of it, neural nets can't do x, Y and Z yet,
but I don't think any sense. We've hit the buffers of of what
neural nets can do. And it's by far the most
successful or the most rapidly advancing technology we have.
So to me, you should look for the keys under the lamppost.
We have this powerful technology that's getting better by the week.
Why would we not see how far we can push it rather than worry
about its limitations? Absolutely. Now, um, Professor Bishop,
you are incredibly famous, um, for your book, Prml.
But of course, it wasn't your first book as you were just speaking to.
But what was I mean, could could you just tell us about your your
motivations and just the thought process behind that book? Yes.
So as you said, it wasn't my first book.
My first book was published in 1995, Neural Networks for Pattern
Recognition. And that book had a very specific
motivation, which is that I was a newcomer to the field.
I mentioned earlier that I got excited about backprop and and sort
of transitioned from theoretical physics into machine learning.
That was my way of learning about the field.
You know, if you're a university professor, a great way to learn about
something is to teach a course on it, because it forces you to think
about it very carefully. You're going to get tricky questions
from smart students, and you're very motivated to to really understand it.
And so for me, the analog of that was was writing a book.
Um, PML was rather different by by the time we got to what's
published in 2006, and by then the field was much larger in a sense,
it was much more mature as a much more established and respected field.
There are many courses on machine learning.
The goal there was very different. I simply wanted to write the,
as it were, the book that everybody would use to learn about the field.
So it was trying to be comprehensive, but trying to be to explain the
concepts as clearly as possible. And so really that was the goal.
The goal was to in a sense, you know, replace the earlier neural nets
for pattern recognition book, which was which served an important
role in its day, I think, but really tried to produce a single
coherent text where people could learn about the different topics in,
you know, with a shared notation and hopefully trying to explain
things as clearly as I could. We know in theoretical physics,
you know, you can you can write down an equation, but solving it
may be extremely difficult. You have to resort to approximations,
but it's still nice to have that that North star,
that compass that guides you. And so for me, I try to think of
machine learning in similar terms. There are some some foundations
that that really don't change much over time that are that are
very good guiding principles. And we're dealing with data,
we're dealing with uncertainty. We want to be quantitative.
So you're led very naturally indeed uniquely into probability theory.
And if you apply probability theory consistently that is the
Bayesian framework. So for me the Bayesian framework
is a very natural bedrock on which you can build and think
about machine learning. Now, just as with theoretical
physics, you can't often just solve things. Exactly.
And certainly the Bayesian paradigm calls for integration or
marginalization of all possible values of the parameters in your
neural network. Well, you always operate with a
fixed computational budget, right? It may be a huge one,
but it you're always constrained by by computational budget.
And should you spend that budget doing a very thorough Bayesian
marginalization over a small neural network, or should you take the
same number of compute cycles and train a very much larger network?
And if you have plenty of data to train the larger network,
then the latter seems to be much more effective in a practical sense.
So while from a practical point of view, the Bayesian approach
still has certain applications in in various domains,
for the most part, it's not the framework we'd want to use in, in
sort of mainstream machine learning. Today, we're much more interested
in scale and making point estimates and using stochastic
gradient descent and so on. So I still think that students
should learn the basic ideas of of Bayesian inference,
because really they have to learn. You have to learn about probability.
I don't think you can be a machine learning and not
understand probability. And then once you understand
probability and you apply it uniformly that, that that really
is the Bayesian framework. So I think it's the foundation.
But then you're led to make approximations.
And in particular you make point estimates.
So in practice you don't actually execute the full Bayesian paradigm.
Yeah I agree that um, Bayesian reasoning is it's beautiful and it's
the continuation even of sort of propositional logic in the domain of,
of uncertainty. It's fundamental. But there is this question of, um,
the world is a very gnarly place, and folks argue that the brain is a
kind of Bayesian inference machine, but it can't it can't possibly
be solving the intractable Bayesian problem.
And therein lies the question. So there are many hybrids or even
deep learning approaches could be seen as some kind of a continuation
or somewhere on the spectrum between maximum likelihood point
estimation and Bayesian, um, models. I mean,
how do you think about that spectrum? I think that's a great that's a
great question. I think you're spot on there.
If you look back to a time when there were a lot of competitions,
here's a data set. We're going to hold out the test set.
You've got a score as high as you can on the test set.
And what approach should you use. The winner always is an ensemble.
You should try ten different things, preferably diverse, and then combine
them suitably, maybe taking an average or some smarter combination.
And that ensemble will always outperform any one single model.
So if you're not constrained by compute and in some of those
competitions you weren't, then the ensemble always wins.
And you can think about that ensemble as like as you say, a sort
of rough and ready approximation to a full marginalization over
all of the uncertainty in the predictions that you might make.
And so I think there's a little glimmer of sort of Bayesian
approaches coming through there. But again, you know, in the modern
era, you're probably better off training one single large model than
ten smaller ones than averaging. So it's um, so I think knowing
about the Bayesian paradigm and understanding where you can learn
from it is still valuable today. But nevertheless, um, it's unlikely
in most applications that you're going to want to apply the full
Bayesian machinery because it's just so computationally expensive.
Fascinating. I mean, just one more thing on this.
Um, do you think of large, you know, let's say large language models,
but large deep learning models, do you think of them as one model,
or do you think of them as an inscrutable bundle of models?
Because we're kind of getting into the no free lunch theorem here.
Um, coming from the Bayesian world, we design models, you know, using
principles and with neural networks we just train these big black boxes.
So do you think of them as one model or lots of models?
I certainly I always think of them as a single model.
I've never thought that thought of them as separate models,
unless you unless you explicitly construct a mixture of experts or
something like that where you have an internal and internal structure.
Um, I guess everything is sort of very distributed and somehow sort
of holographic and overlapping. And, you know, a remarkable thing
about GPT four is that, um, you know, you often see people
when they first they first use it, they'll ask, ask some questions.
How tall is the Eiffel Tower? And it probably gets the right
answer, you know, and say, oh, that's kind of interesting.
And you sort of a little bit disappointed in this technology,
but it's like being given the keys to a very expensive sports car.
And you notice the cup holders and you notice that it can can
support a cup rather nicely. You don't realize you need to start
the engine and drive off in it to really get the full experience.
And so until you realize that actually you can you can have a
conversation, it can, it can write poetry, it can explain jokes,
it can write code, it can do so many, many different things.
And all those capabilities are embedded in the same model.
And and what is, I think a really interesting lesson of the last few
years is that models like GPT four outperform the specialist models.
So for example, in my lab, we had a project for many years which
essentially said the following. It said, well, you know, this is
Microsoft World's biggest software company. We have lots of source code.
We could use source code as training data for machine learning.
We'd be able to do all sorts of things.
You know, spot bugs, do autocomplete, you know, all kinds of things you
could do if you had a good model of source code and the project was
reasonably successful, it was, you know, it worked reasonably well.
But what we've learned is that when you build one gigantic model that
that yes, it sees source code, it sees scientific papers,
it sees Wikipedia, it sees many, many different things in some way.
It becomes better at writing source code than a model specifically
for writing source code. And there are even even been ablation
studies where people have have a model that's trained to solve maths
problems, and it does reasonably well, and now you give it some
apparently irrelevant information, let's say from Wikipedia.
But with anything to do with maths stripped out and you find it
actually does better at the maths. So I think there are things here
that we don't really understand. But the general lesson, I think,
is fairly clear that when you have a larger, very general model,
it can outperform a specific model, which I think is very interesting.
I guess the reason I was talking about the no free lunch theorem
is it feels to me, as you say, that models behave quite differently
in an input sensitive way. Uh, so you ask them about this
particular thing, and it's almost like it's a
different model because different parts of the model get activated.
And then there's this question of, well, is the no free lunch
theorem violated? Can there be such a thing as a
general foundational agent that could, in robotics, just do really
well in any game or any environment? Or do you think, do you think there's
still some need for specialization? Another great question.
So I think, um, uh, these are really open research questions.
Honestly, I'm not sure anybody really knows, but I think one of the
lessons is that the general can be more powerful than the specific.
So clearly, one of the research frontiers we
should push on is greater and greater generality and see. So.
GPT four can't ride a bicycle. But if we have models that can
can do robotics, should they be separate and distinct models,
or if we somehow combined everything into a single model,
would it be more powerful? And there's a decent chance that
the latter would be true, that it would be more powerful.
So certainly that's one research frontier we should push on.
An area I'm very interested in these days is is deep learning for for
science, for scientific discovery and science, amongst other things,
involves very precise, detailed numerical calculations.
Now, if you want to multiply some numbers together, GPT four would
be a terrible way of doing it. It might give you the wrong answer,
and even if it gets the right answer, you're burning a tremendous
amount of compute cycles to do something you could do with far
fewer compute cycles. So there will still, as far as I
can see in certain domains, be a role for specialist models.
But even then I can see them being integrated with things like large
language models, partly to provide, um, human interface,
because one of the wonderful things about language models is they
they're so easy to interact with. You don't have to be a computer
programmer. You just have a natural
conversation with them. But also so, um,
the other remarkable thing about the large language models, I think
there are two remarkable things. The first of all is that they're
so good at human language. Maybe that's not too surprising
because they're sort of designed to do that,
but by virtue of being forced to effectively compress human language,
they become reasoning engines. And that's a remarkable discovery.
Right? That is a big surprise. Certainly to me.
I think to many people, perhaps to everybody in the field, that they
can function as reasoning engines. And so even if you're, let's say,
doing some specialist scientific calculations, you might still think
about large language model as, as a kind of a copilot for the scientist,
helping the scientist reason over what increasingly consists of
massive, massively complex spaces, very high dimensionality,
many different modalities of data, it's harder and harder for humans to
sort of wrap their head around this. And this is where I think a
large language model can can, can be valuable.
But I still see it calling on specialist tools in the foreseeable
future because you were talking about statistical generalization,
but you could argue that language models can't do let's say they can't
compute the nth digit of pi because they don't have an expandable memory.
They're not Turing machines. So that that's a computational
limitation. But but they might be able to do
this statistical generalization, as we were talking about,
even though it might in fact be a weird form of specialization in terms
of an ensemble of methods and models inside, um, a large language model,
but on the on the language thing and the reasoning, this is fascinating.
So I think that language is a bunch of memetically embedded programs.
So we we play the language game and we establish cognitive categories.
We embed them and share them socially.
And it's like there's a little simulation out there and I'm
using that to think. But the question always is to what
extent, um, is that that's a bunch of processing that previous humans
have done, and we can use it. But can the language model
create new programs like that? This is, I think, part of a
fascinating and broader discussion. So I do hear a lot of, oh, it can't
do X, Y, and Z often. That's true. And I always put the word yet at the
end of it, because I don't know any law of physics that it can't do,
there are some things which perhaps the current
architectures provably can't do, but but there's lots of exploration
in different architectures. There's a lot of scope for, for,
for expanding and generalizing neural nets.
So I always think of it can't do a certain thing yet.
But a lot of the questions, a lot of the comments about the
limitations of models, um, I have a have a hypothesis on this.
I mean, let me test this out on you. I maybe I may be way short of the
mark on this one, but a lot of the, a lot of the critique of what models,
um, seemingly can't do, especially when it's, oh,
they will never be able to do this. They cannot be creative or they
cannot reason or they cannot whatever.
I wonder if a lot of this comes to, um, to a much more fundamental point
that's not actually a technical one. It's really to do with the human,
the human journey over the last few thousand years because we've,
you know, a few thousand years ago, I guess, most humans would have
perceived humanity as the center of the universe.
The Earth was the center of the universe.
The universe was created for the benefit of humanity.
We had this very arrogant view of our own importance and what we've learned
over the centuries, especially from fields like astronomy, is.
Of course, you know, the entirety of humanity's existence is a brief blink
of the eye compared to the existence of the whole universe and our and
our physical place in the universe. In terms of length, scale, we're on a
little speck of dust orbiting an insignificant star in a rather boring
galaxy in this colossal universe. And and so I think it's natural
for us as humans to sort of continue to cling to the things
that we feel make us special. And we're certainly not the
fastest creatures on Earth. We're not the strongest, but it's our
brains that seem to make us unique. We are the most intelligent creatures
by far on Earth, and so we think of our of our intelligence as
being the very special thing. Yes. Okay, we get it that we're just
living in a boring corner of the universe, but nevertheless,
it's our brains that make us special. So let me tell you a little story,
which is, uh, because I work for Microsoft, I was very privileged
to have early access to GPT four when it was still a highly tested,
highly secret project. And so I was exposed to GPT four
at a time when I could only discuss it with a very small.
Small number of very specific colleagues.
And for everybody else, I couldn't couldn't even talk about it.
And it was quite, a quite a shocking moment.
The the ability to, to understand and generate language
sort of didn't come as so much of a surprise, because of course,
I'd been following GPT two and GPT three and, you know, knew this
technology was getting better. But this ability to reason there was
a a sort of visceral reaction I had, which took me right back to that
film, 2001, that sense of I was engaging with
something which my colleague Sebastian Bubeck called it the
sparks of artificial intelligence. So nobody, nobody's claiming GPT
four is anywhere close to human intelligence or anything like that.
But there was just the first glimpse of something.
It was the first time in my life that I'd interacted with something
that wasn't a human being, that had a glimmer of this,
this high level of intelligence. And, um, and realizing this may
be the dawn of a, of a new era that may be even more
significant than the 2012 moment of the dawn of deep learning.
There was something very special going on, and I wonder if part
of the reaction that we have to these models is a little bit of
that sense of that threat to the specialness that we feel as humans.
I may be completely wrong. This is purely speculation, but,
you know, it's interesting that we talk about people use phrases
like stochastic parrot. It's just regurgitating stuff
that that it's seen before some people claim or, you know,
of course it hallucinates. Sometimes it comes up with stuff
that's just wrong or doesn't make sense.
But but think about the following. Imagine there was a very,
very smart physics student. Went to went to a top university,
worked really hard for four years. What would they do?
They would they would read books. They would read papers, listen to
lectures, have discussions with their professors and with other students,
and then they sit their final exam and they get 95% in their final exam,
and they come top of the year. We don't say, huh.
Well, 95% of the time there are stochastic parrot regurgitating
Einstein and Maxwell, and the other 5% of the time they're hallucinating.
No, we say congratulations, you have a first class honors degree.
You've graduated with honors. This is this is a, you know,
a wonderful achievement. So it's interesting that we do seem
to view the capabilities of, of neural nets with almost a different
ruler to, to that of humans. And while nobody suggesting that
current models are anywhere close to humans on many axes of intelligence,
nevertheless I see the first sparks of of of artificial intelligence.
And just one final comment. Um, the Terme AI artificial
intelligence has been very popular for many years. I used to hate it.
I used to always say, that's machine learning.
None of these systems are intelligent.
They're very good at recognizing cats in images.
There's nothing really intelligent about this in one sense.
Um, and yet now I find for the first time I feel comfortable talking
about artificial intelligence, because I think we've taken the first
baby steps towards what I think of as true artificial intelligence.
I still think that agency and creativity are the distinguishing
feature, not necessarily that we are biological beings.
It's more to do with we are independent agents and we are
sampling random things from our local worlds, and we're combining them
together in in interesting ways. And in doing so, intelligence is
about the process of building models and sharing models and
embedding models in our culture. So it feels to me that GPT was
building models at the time it was trained.
And and that's all it's doing. I can imagine a world where
there were lots of gpts. We all had GPT in our pockets,
and maybe then it would be much more like biomimetic intelligence.
I think there are lots of interesting points that you touched on there,
Tim. So, um, I think one thing is in
terms of creativity, you know, are these systems creative?
It's certainly true. They only exist because of humans.
They are created by humans. And and we should acknowledge that.
But I don't think it means they're intrinsically not creative.
If I asked an artist to, um, paint me a picture of some people
walking on the beach with a sunset or whatever, and they came
back a few days later with some beautiful picture, I might hate it.
They may have used very vivid colors. I might like pale pastel colors,
but that's a matter of opinion. But I wouldn't deny that there
was creativity there. But their expertise came because,
well, they went. They had some intrinsic ability
in some sense. But they went to art school.
They studied the work of other artists.
They practiced, they got better. And, and, and that creativity
owes a lot to what went before. But I don't think it diminishes that
in the same way a physics student who can explain the theory of relativity,
you have to say, well, you didn't invent the theory of relativity.
No, Einstein invented that. You only learned it from Einstein.
But it doesn't diminish, um, the fact that they have understanding,
the fact that they convey it, and the fact they can potentially
think in new ways and be creative. So I'm, I'm less convinced about
discussions about the limitations of, of, of the technology in general
and where it can go. I don't particularly see any
limitations. The brain is a machine. It uses this tum we used earlier
connectionist approach. It uses these fine grained
neural nets. And and so there are similarities to
the technology that we have now. There are also huge differences.
Some of those differences point to the artificial neural nets
being much more powerful than biological neural nets.
And Hinton has made a strong point of this lately.
And I think it's a very interesting perspective.
So I would be the first to say, yes, the technologies we have on many
axes are a long way short of humans on many axes. They're much better.
GPT four can create text much better than any human.
I mean, to produce a page of coherent text that's correctly
punctuated and good grammar and so on in a few seconds.
There aren't many people that can do that, I think.
So on an increasing number of axes systems clearly outperform humans,
and on others there's still a very long way to go.
But I think one of the nice things about technologies like this,
generative AI technologies, whether it's, you know,
Sora for creating videos or GPT four or whatever it might be,
is they do rely on a prompt. There is a clear role.
They are copilots, as we say they they sit there and do nothing,
and you use them as a sort of a a cognitive amplifier.
You have an idea sort of half baked, and now you can engage in a
conversation and sure enough, it can come up with a different
way of thinking and say, hey, that's really good. I like that idea.
Now let's take that, work that back in and try again.
And so it becomes now a companion, a copilot, something that enhances
your your cognitive ability. But the human is still very much in
the loop and playing a key part and actually initiating the process.
And then of course, finally, at the end of the day,
you're the one that selects the, you know, the ten video clips,
you pick the one that you like. And so the human is very much
involved in the loop throughout. So I think that's a very nice
feature of this technology. I completely agree with that.
So at the moment AI's are embedded in the cognitive nexus of humans.
So we have the agency and we drive these things and they help us think.
And also, I agree with you that it doesn't
make sense to think of these things as limited forms of computation.
We should think of the collective intelligence.
So we are Turing machines and we are driving these things and we
are sharing information. So when you look at the entire
system, it is a new type of memetic intelligence.
In fact, you know, to to a certain extent, GPT four
isn't running on Microsoft's servers. It's in all of us. Right?
And that's that's a wonderful way, um, to think about it.
But to me, the extent to which it is constraining our agency and
creativity is what I'm fascinated by. So GPT says,
unraveling the mysteries. And, you know, the the intricate
dance of X, Y, Z and all of these weird motifs and constructions.
And maybe that's just the way that our life has constrained the model.
Or maybe it's it speaks to the constraining forces in general of
having these low entropy models that kind of, you know, snip off a
lot of the interesting pathways. So we are very creative.
GPT four resists creativity a little bit. Is it a problem?
Well, I think there's some design choices there.
So you talked about reinforcement learning through human feedback as
part of that alignment process. We want to create this
technology in a way that does good to minimize harm.
And so naturally we do constrain it. So for sure it's true that a
constrained GPT four behaving you might say less creative ways
but perhaps more helpful and beneficial ways.
And it's appropriate that we should do that.
And perhaps we lose a little bit of the creativity, um, in the process.
And so there's a balance. There's a, there's a,
there's a choice to be made, a design choice in how we want
to create the technology. And we should be very deliberate
about that and not not apologetic for that.
I think it's good that we are that we are making those design choices,
but people sometimes have an intuition that it's not creative and
contrast that to I'm using DaVinci resolve and I'm using all of these
nodes and I have all of these filters and, and processing transforms.
The difference seems to be that I'm designing the architecture.
So I'm using cognitive primitives, and I'm composing them together
in a new way. And by tweaking the parameters
on the filters, I'm going off piste a little bit.
I'm doing I'm creating the structure myself.
Whereas in neural networks the structure is implicit.
I don't know what the structure is. Well, I think you're talking
about your contrasting two different kinds of tools there.
So the video editing tool, um, is designed so that it follows
your instructions very precisely. And you prefer one tool over another,
perhaps because the interface is easier to use, you get the
results faster, but you have in your you've done the creativity.
You've designed this to video edit that you want, that you want to have.
And now the tool is to try to get you to that as fast as possible,
as accurately as possible. But sometimes we need more than that.
Sometimes, you know, if you've got writer's block and you don't know
where to begin, having a tool like GPT four could be very powerful.
You're not you're not delegating the entire process to the technology.
You're working with it as a, as a as a copilot,
as an assistant that can can for sure help you with that creative process.
It will come up with with crazy things.
And most of them you may not like, but maybe one of them you don't
like it either, but it causes you to think about something that you
would otherwise not have thought of. And so the two working together
can surely be more creative. So I think certainly as a as a
working in unison with humans, it's certainly enhances creativity.
So that's certainly my experience I think there's no doubt about that.
But also if you think about, let's say, a simple example,
I think most people relate to which is which is image generation.
You're giving a talk and you want some image to illustrate the talk.
And, you know, you could go to stock images and you know,
it's a fixed set and you know, you can't easily adjust it or you or you
go to editing the images yourself. That's a sort of slow and
painful process. But now you can just with a
simple prompt, you know, you can get a bunch of examples.
And if one of them isn't quite what you like, you can alter the
prompt and fine tune it. And it now becomes that that process,
which is a creative process. Um, and you can sort of say the
human is in the driving seat, but the overall creativity is
certainly enhanced. And when you take a text prompt and,
and the machine produces this beautiful photorealistic image,
I mean, how many of us weren't absolutely
blown away by the incredible advances in generative AI over the last,
you know, the last decade? Um, why would you not call that
creative of a human being? Did it you would call it creative.
Why? Why are we not allowing the machine
to be described as creative? That's the piece that I don't
that I don't quite understand. So you could argue that creativity is
just pure novelty of the artifact. So it's just how much entropy is
in the artifact. But, um,
you could you could think of GPT four prose as being a kind of category.
So there's a lot of variance in there.
Um, but there are also certain motifs.
And now when people see the motifs, they say, oh, I've seen that
a million times before. So I did think it was novel and
interesting, and now I don't. And, but but this is the thing.
So now when I'm writing blog posts and stuff like that,
I'm deliberately trying to do something genuinely creative.
You know, it's almost like the intrinsic creativity isn't important.
I don't want people to think that I use GPT four, so that's driving it.
Do you see what I mean? Yeah. So I mean, clearly creativity is
about novelty and novelties. Um, you know, what we desire here,
but whether that novelty has value or not, that's a subjective opinion.
In your case, it's whether it's achieving the goals that you desire.
So I think there is no doubt that even if you say we're just taking
existing ideas and combining them in new ways, everything that humans do,
I think builds on the work of their own previous experience
and on the work of others. And and I think that's
absolutely fine. That's a wonderful thing about
humanity is that we from generation to generation, we build upon the
work of what's gone before and the machines that we build now are
heavily dependent on the creativity and the work of humans before,
because they learn from humans and they're designed by humans.
And I think that's absolutely fine. It's a wonderful thing.
And they add to the sum total of human creativity.
And that's a wonderful thing. Chris, you've written a really
beautiful book and you wrote it with your son Hugh.
And there was a picture of Hugh, and I think in the introduction of,
of Prml. And I guess part of what I want
to understand is, is deep learning is is a huge field.
Um, I mean, what was the thought process and how did you decide what
to tackle and what not to tackle? Great questions.
There's an interesting story behind the new deep Learning book, which
is that Prml was written in 2006. It predates the deep learning
revolution, and what is constantly surprised me is just how popular it's
remained, in spite of the fact that, in one sense, it's massively out
of date because it has no mention of the most important thing in
the field of machine learning. So I've long felt it was time to
update the book, produced the second edition,
add some material and deep learning. Um, but life is busy, and you know,
anybody who's ever written a book will tell you that it takes
way more effort than you can possibly imagine if you've not
actually had that experience. And so I never really got around
to to doing it. And then along came the Covid
pandemic and we all went into lockdown.
And I feel like it was one of the very privileged people in
that lockdown. We were we were locked down together
as a family, um, in Cambridge. And you know, when you're locked
down at home for several months, you kind of need a project.
And, and I thought this would be a great time to think about a
second edition of the Prml book, because, you know, what else are
you going to do in lockdown? And it became a project with my
son because he was he was with me by this time.
He he'd, uh, um, gained a lot of experience,
master's degree in machine learning. And he'd been working in
autonomous vehicle technology. And in a sense,
he had a lot more practical, hands on experience with deep learning
than than I did at that point. And so we started this as a
joint project. But we very quickly realized that
what was needed was not a couple of extra chapters on Prml, but rather
the whole field had changed so much. And also, we didn't want to
write a book that we were just accumulated more and more material.
It would just become a huge, a huge tome.
The value of a book, I think is, um, is in the distillation is in
the way it draws your attention to a subset of specific things.
This is the small set of things that you really need to understand,
and then you're equipped to go off into the field.
So what we omitted was almost as important as what we what we added.
And we very quickly realized this was a this was a new book.
So we, we, we we called the book Deep Learning Foundations and Concepts.
And we made a lot of progress. But then of course,
the lockdowns ended. Um, I started a new team called
AI for Science at Microsoft. Uh, Hugh started at Wave
Technologies, building the the core machine learning technology
for their autonomous vehicles. And we were all just far too busy.
And then the next thing that happened was the ChatGPT moment where,
you know, in a space of a few weeks, 100 million people were using this
and suddenly AI machine learning was in the in the consciousness
of the general public. And we realized that if ever there
was a time to finish this book, it had to be now.
And so we had just a really big push to to get the book finished and
available for, for NeurIPS in 2023. And we made it just, you know,
at the last minute as you do. And the book was on display at
NeurIPS there, and Hugh and I spent the week going
around the conference together. Talking to folks at posters and
and just had a great time. So it was actually a huge privilege
to to be able to write the book with my son. Yeah. That's fantastic.
Um, what was your favorite chapter? And I mean, are there any, um,
things that you felt were omissions that you would have liked to do,
but you just had to draw a line under it? Yeah.
In terms of favorite chapters, I mean, of course, the things the
the more recent architectures were particularly interesting.
I very much enjoyed writing the diffusion chapter, and Hugh had a
lot of input into that chapter, and of course, Transformers as well,
and just understanding how to how to integrate the sort of the
different generative frameworks, how to bring think about Gans and
how to think about variational autoencoders and how to think
about normalizing flows and so on, and how to think about those
under one umbrella and present them in a more coherent way.
So that was that was part of the interesting for me,
the learning experience. I always enjoy learning new things,
and I learned things writing that book, and I think Hugh did as well.
And so in a sense, that was that was the favorite part of the book.
The things where I where I learned new things or new ways of looking at
things I already knew about the real decision process was what to put in
and what not to put in, while keeping the size of the book under control.
Because I think it's something like it's thousands of papers a month
now published in Machine Learning. It's overwhelming for the beginner.
So really the goal of the book is to distill out those few core concepts,
which means there are always things, oh, should we have added this?
Should we have added that? What we wanted to do was to
avoid adding the latest sort of architecture that might be very hot
at the minute, that could easily disappear three months down the line.
So I hope we resisted that, that temptation.
Um, but there are areas where, you know, perhaps when we at some point,
if we get around to a second edition, we might think about including
reinforcement learning is something which is of growing
importance and would be lovely to have a chapter on reinforcement
learning that integrates well with the rest of the book.
There are books on reinforcement learning. There are review articles.
There's plenty of place to go learn about them, but something that
sort of integrated with the book, I think could be could be valuable.
So that is something we might we might visit in the future.
But for the moment, we've just focused on what we think are the
the core principles that any, any newcomer to the field,
whether a master's student, whether they're somebody who's
self-taught, a practitioner coming into the field wanting to
understand the basics of the field. And so the goal was to try to
keep the book, as it were, as short as possible, but no shorter.
Looking back on on your last couple of books as well, in retrospect,
um, which bits are you? Are you most kind of proud of,
and which bits do you do you kind of feel that when you did make
the decision at the time, perhaps you mispredicted how successful
something might be? Very interesting. So the thing I'm most proud of,
actually, is the very first book called Neural
Networks for Pattern Recognition. And the reason is because I think
the book was quite influential in steering the field towards a more
probabilistic, more statistical perspective of machine learning.
It perhaps hard for people to appreciate today,
but it wasn't always that way. When I first went into machine
learning, a lot of it was inspired by neurobiology,
which is which is fine, but it lacked sort of mathematical rigor.
It lacked any mathematical foundation.
And so there was a lot of trying to learn a bit more about the
brain and then try to copy that in the algorithms and see if
that worked better or not. And there was a lot of trial and
error. Still a lot of empirical, uh,
trial and error in machine learning, of course, but at least we have that,
that sort of bedrock of probability theory.
And so I think the book was the first one to really address
machine learning and neural networks from a statistical from
a probabilistic perspective. I think in that respect,
the book was very influential. The field was much smaller then today
we take we take that as obvious. But I think in terms of the
thing I'm most proud of, it's probably the influence of that.
That first book, back in back in 1995, in terms of things I look back
on that I might do differently. I suppose when I look at if I look
at Prml, for example, and I look at the trajectory of the field,
we've seen that neural networks were were all the rage in the
mid mid 1980s to mid 1990s, and then they kind of got
overtaken by other techniques. And then we had this sort of
Cambrian explosion of, you know, support vector machines and
Gaussian process and Bayesian methods and graphical models and,
and all the rest of it. And, and I think one thing,
that one thing that I think Geoff Hinton really got right is he really
understood that neural networks were the way, the way forward.
And he really stuck to that perspective sort of through
thick and thin. Um, I got kind of distracted
particularly. We talked earlier about Bayesian
methods and how beautiful and how elegant they are.
And to a theoretical physicist, it's very appealing to think of everything
from a Bayesian perspective. But really, what we've seen today
is that the the practical tool that's giving us these extraordinary
advances is neural networks. And most of those ideas go back to
the to the mid 1980s to the idea of gradient descent and, and so on,
a few new, a few new tweaks. You know, we have GPUs,
we have Relu's, we have a few, but essentially most of the ideas
were were were still were around back in the back in the late 1980s.
We didn't really understand the incredible scale at which you need
to use them, but they only really work when you have this gargantuan
scale of data and compute. And of course,
we didn't really have GPUs or know how to use them back then.
So there were there were some key developments that have unlocked.
This had made it possible, but I think perhaps if I did
something differently with the amazing benefit of hindsight,
other than sort of investing in certain stocks and whatever,
and all the other things you could do if you had perfect hindsight,
I think the other thing I would do is probably just stay really
focused on neural networks, because eventually that's the
technology that came good. But I always come back to probability
theory is very much a unifying idea. So let me just give you a
specific example from Prml. Actually, there were two different
technologies, one called Hidden Markov Models, that were all the rage
in speech recognition back then. Another technique called Kalman
filters that have been used for many years to to guide spacecraft,
track aircraft on radar and all sorts of things.
Um, it turns out they're essentially the same algorithm,
and not only are the same algorithm, but they can be derived from the
most beautifully simple principle. You just take the sum and
product rule of probabilities, and then you take the idea that
a joint probability distribution has a factorization described by a
directed graph, and if you want to. So when I was preparing Prml,
I looked over a bunch of books called Kalman Filters An
Introduction to Kalman Filters, and they would go on chapter
after chapter at the forward and then chapter after chapter about
the reverse equations and so on. It's very, very complex and very,
very heavy going. But you can derive the Kalman filter
and get the hidden Markov model for free in almost a few lines of,
of algebra, just starting from probability theory.
And this idea of factorization, a sort of deep mathematical principle
that operates there, and you discover the message passing algorithm.
And if it's a tree structure graph, it's exact and you have two passes.
It's very beautiful and very elegant. So I so I love the fact we're
exploring all these many different frontiers, but I love
the fact we have some at least some compass to guide us as we as
we engage in the exploration of this combinatorially vast space.
Yeah, it's so interesting. My co-host, Doctor Keith Dugger,
he always says that he doesn't need to remember all of the different
statistical quantities because he can read rederive them from
first principles. It's that nice. But we should move on to AI for
science. So you're leading this initiative
at Microsoft Research. Can you tell us about that? Yes.
So at a personal level, of course, this brings back my my earlier
interest in theoretical physics and and chemistry and biology
and brings it together with, with machine learning.
And what many people realized, uh, a few years ago was that of the
many areas that machine learning would impact the scientific,
the area of scientific discovery would be, I think, in my view,
the most important, the reason I say that is because
it's actually scientific discovery that really has allowed
humans to go on that trajectory of the last few thousand years,
not just understanding our place in the universe, but to be much
more in control of our own destiny, to double our lifespan,
to cure many diseases, to give us much higher standards of living, uh,
to give us a sense, a much brighter outlook for the future than human
humans have traditionally enjoyed. And and that's come through
scientific discovery. And then the application of that
knowledge and understanding of the world in the form of technologies to
agriculture, industrial and so on. And so I can't think of any more
important application for AI. But what's really interesting is
it's very clear that many areas of scientific discovery are
being disrupted. And when I say disrupted, I'll
just give you one simple example, the ability of neural nets,
machine learning models to act as emulators for what previously
were very expensive numerical simulators very often gives you
a factor of 1000 acceleration. You know, we can forecast the
weather a thousand times faster with the same accuracy than we
could a few years ago, prior to the use of deep learning.
Now, if that were the only thing that was happening,
that alone would be a disruption. That alone would be worth setting
up a team on AI for science. I think actually it's only scratching
the surface, but any time something that's very core, very important,
gets a thousand times faster, it means you can do things that would
take years in a few tens of hours. That really is a disruption.
It really is transformational. So, um, a couple of years ago,
I pitched to to our chief technology officer to say, look,
this is a really important field. I'm happy to step down from my role
as the lab director of MSR in Europe. And instead, um, I'd like to lead a
new team focusing on AI for science. And it met with enormous enthusiasm.
And so we've been growing and building that team.
It's very interesting team. It's very multinational.
We have people on on many different continents and different countries.
We've opened new labs in Amsterdam and in Berlin.
We have teams in, in Beijing and, and in Shanghai and folks in,
in Seattle as well. And um, so very,
very multidisciplinary, very multinational, but with,
with one thing in common, this real excitement and passion
for what machine learning and AI is going to do to really transform
and accelerate our ability to do scientific discovery.
You were talking about Inductive priors just a second ago, and I
guess I first learned about this, the art of, you know, designing
inductive priors and machine learning from Max Wellings group.
They were saying that, you know, the remarkable thing is that you can,
um, using, um, principles, let's say from physics, we can design
these inductive priors and we can reduce the size of the hypothesis
class that that we're. Approximating. And because we know the target
function is inside that class, we are not introducing any
approximation error. And we're we are kind of overcoming
some of the curses in machine learning by making the problem
tractable, which which is amazing. But that's speaking to this kind
of principled approach of imbuing domain knowledge into these systems.
It's really interesting, actually. Max and I have a similar trajectory.
You both did PhDs in theoretical physics and then moved into
machine learning, and I think we both feel there's a
very important role for inductive bias to play in the use of machine
learning in the scientific domain. I think I'm sure everybody is
familiar with the the blog called The Bitter Lesson by Rich
Sutton and if any, if anybody watching this is not familiar,
they should immediately after this video go and read that blog.
It's a very short blog, and without giving too much of a spoiler,
he essentially says that every attempt by people to improve the
performance of machine learning by building in prior knowledge building,
in what we call inductive biases into the models,
it produces some improvement. And then but very quickly,
it's overtaken by somebody else who just has more data.
And and that indeed is a bitter lesson.
And, and it's a wonderful blog and people should I've read it many times
and I think people should, you know, probably read that once a month.
And it's very inspiring. But I think there may be exceptions.
And I think the scientific domain is one where inductive biases for
the foreseeable future will be extremely important, sort of almost
contrary to the bitter lesson. And a couple of reasons for this.
One is that the inductive biases we have are not not of the kind.
Let's say let's take linguistics or something, which is or any
domain where which is based on human expertise acquired through
experience, because a person who's had a lot of experience over a number
of years and formulated some sort of rules of thumb that guide them,
that's exactly what machine learning is very good at processing very
large amounts of data and and inducing the the rules, as it were,
the patterns within that data. Um, so I think that kind of inductive
bias is, is typically harmful. And, and I think the bitter lesson
will certainly apply there. But in the scientific domain
it's rather different. First of all, the inductive
biases we have are very rigorous. We have the idea of conservation of
of energy, conservation of momentum. We have symmetries.
If I have a molecule in a vacuum, it has a certain energy.
If I rotate the molecule, the representation of the
coordinates of all the atoms changes wildly in the computer,
but the energy is the same. So we have this very rigorous
inductive bias. We also know that the world at the
atomic level is described exquisitely well by by Schrodinger's equation.
And sprinkle in a few relativistic effects.
And you've got an amazingly accurate description of the world, but it's
way too complex to just solve it directly, or it's exponentially
costly in the number of electrons. But nevertheless, we have this
bedrock of of really understanding the laws that govern the universe.
And so and so I think that's the first the first thing,
we have very rigorous priors that we believe in deeply.
It's not that we think conservation of energy doesn't work.
We we know that it's true. The second thing is that we're
operating in a data scarce regime. So large language models are able
to use very large quantities of internet scale quantities of
human created data, whether it's in the form of, you know,
whether it's Wikipedia or whether it's just scientific papers or, uh,
any of the output of humans almost is potentially material on which,
which large models can feed their in a very data rich regime and can
go to scale and and so the bitter lesson I think really kicks in there
in the scientific domain, the data might come from simulations which
are computational and expensive, or it might come from lab experiments
which are which are expensive. And the data is is limited.
So we're operating usually in a data scarce regime.
So we have relatively limited data and we have very rigorous
prior knowledge. And so the balance between the
data and the inductive bias, um, is very different because of course,
the no free lunch theorem says you can't learn purely from data.
You have to have some form of inductive bias.
And in the case of a transformer, it's a very lightweight form of
inductive bias. We believe there's a there's a
deep hierarchy. There's some, you know,
data dependent, uh, self-attention, but but really, that's it.
Uh, and the rest is determined from the data.
In science, there's much more scope for
bringing in these inductive biases. There's much more need to bring
in the inductive biases. And that also, incidentally, again,
in my personal very biased opinion, makes the application of machine
learning and AI to the sciences the most exciting frontier of AI
and machine learning, because it's the one that's richest
in terms of the creativity, and also in terms of the need to
bring in some of that beautiful mathematics that that underpins the
universe. Yeah, so, so fascinating. I mean, could we just linger
just just for a second on that? So Rich Sutton, in his Bitter Lesson
essay, he explicitly called out symmetries as being, you know,
he was warning against human designed artifacts in these models.
And I mean, Max welling, as you say, famously, um, built these gauge
equivariant neural networks, bringing in his physics knowledge.
Um, so I'm just trying to understand the spectrum between
high resolution physical priors and the kind of macro.
For human knowledge that that we learn, which is presumably brittle.
Is it just that we think that these physical priors are
fundamental and that's that's a that's a perfectly acceptable way
to constrain the search space. But these high level priors are
brittle. Yes. I think I think the prior knowledge
that comes from human experience, um, is, is, is more of that
brittle kind because the machine can see far more examples than
any human can in a lifetime, and can can do a more systematic job
of looking across all of that data. We're not not subject to, say,
recency bias and those sorts of things.
So I think that kind of prior knowledge is, is one where where
scale and data will, will will win, whereas the, the prior knowledge
that we have from the physical laws in a sense, is much more rigorous.
And symmetry is, is, is very powerful.
It's sometimes said that physics more or less is symmetry.
That's almost right. I mean, right. So conservation conservation
laws arise from symmetry. You know, translation invariance in
space time gives you conservation of energy and momentum.
And, you know, gauge symmetry of the electromagnetic field gives
you charge conservation and so on. And so these are very, very rigorous
laws that apply from symmetry. But, you know, even if you take
a data driven approach, people often use data augmentation.
If you know that an object doesn't depend is identity,
doesn't depend on where it is in the image, you might, you know,
make lots of random translations of your data to augment your data.
So data augmentation can be a data driven way of building in
those symmetries. But now when we have very rich
prior knowledge, I'll come back to Schrodinger's equation.
It describes the world with exquisite precision at the atomic level.
But solving it is very, very expensive.
And so what we can do is we can cache those computations.
We call it the fifth paradigm of scientific discovery,
which is a rather fancy turn. But the idea is very simple,
is that instead of taking a conventional numerical solver and
using it to solve something like Schrodinger's equation or something
called density functional theory, instead of solving that directly
to solve your problem. Instead you use that simulator
to generate training data, and then use that training data to
train a machine learning emulator. And then that machine learning
emulator can now emulate the simulator, but typically 3 or 4
orders of magnitude faster. So provided you use it a lot and
you amortize the one off cost of generating the training data and
doing the training, if you're going to use it many, many times overall,
it becomes dramatically faster, dramatically more efficient than
using the simulator. And that that's just one of the
breakthroughs we're seeing in this space.
So first of all, um, there's a spectrum, as you say,
of we could just train on lots of data or we could augment the data,
or we could make a simulator for the data, and then we can train
a machine learning model. And as we were just speaking to these
inductive priors, they are so high resolution that we are not, um,
restricting the target function that, that, that we want to learn.
And we can make quite a principled argument about that.
But the one question to me is there's a kind of I don't know
whether it's best to frame it as exploration versus exploitation,
but there needs to be some amount of going off piste.
So we define the structure and we we essentially build a generative
model and we can generate a whole bunch of trajectories.
But could it ever be the case that we wouldn't have enough variance
to find something interesting? There's a very interesting question
about the the overall scientific method of formulating hypotheses,
running tests, evaluating those hypotheses, refining the hypotheses,
running more experiments, and so on that, that that scientific loop.
I think machine learning will have an important role to play there
because data is becoming very high dimensional, very high throughput.
Humans can't analyze this data anymore.
A human can't directly look at the output of the Large Hadron Collider
with its, you know, petabytes a second or whatever it is pouring off.
We need machines to help us. But again, I think the human, um,
rises to the level of the conductor of the orchestra, as it were.
They no longer have to do things by hand.
Machines are helping to accelerate that.
And and I think the machines can help accelerate the creative process,
potentially by pointing to anomalies or highlighting patterns in the
data and so on, but very much with the human scientist in the loop.
But but even coming down from those sort of lofty, more sort
of philosophical considerations just to the the practicalities,
when we talk about discovery, we're also interested in just
the very practical method of how do we discover a new drug,
or how do we discover a new material. So scientific discovery also means
that, that, that, that, that very pragmatic near-tum approach.
And there we're seeing really dramatic acceleration through the
concept of this emulator, um, in our ability to explore the
combinatorially vast space of new molecules and new materials,
exploring those spaces efficiently to find potential candidates that
might be new drugs or new, um, uh, new materials for batteries or
other other forms of green energy. So that that alone is a very
exciting frontier. I think it's so interesting.
Um, so searching the space, I mean, drug discovery is an interesting one.
I think you spoke about sustainability as well as another
application you can speak to, but how do you identify?
Find an interesting drug. So the drug discovery process starts
first of all with the disease. And trying to, first of all,
deciding we want to go tackle a particular disease and then
finding a suitable target. So the standard so-called small
molecule paradigm, which is where most drugs are today, they're small
synthetic organic molecules that bind with a particular protein.
So pharma companies will will spend a lot of time identifying targets.
So say a protein that has a particular region with a
molecule combined and can bind to and therefore can influence
the behavior of that protein, switching on or switching off some
part of that disease pathway and breaking the chain of disease.
And so the challenge then is to find a small molecule that first of all,
has the property that it binds with the target protein.
That's the first step. But there are many other things
that it has to do. It has to be absorbed into the body.
It has to be metabolized and excreted.
It mustn't and particularly mustn't be toxic.
It mustn't bind to anything, many other proteins in the body
and cause bad things to happen. So what you have is a very large
space of molecules, usually estimated around ten to the power
60 potential drug like molecules. And out of that enormous space
of ten to the 60 are trying to find an example that meets all
of these many, many criteria. And so one approach is to
generate a lot of candidates, but in computationally and then
screen them one by one for different properties, that screening process,
the more that can be done in silico rather than in a wet lab,
the faster it can be done. And the the larger the search
space can be, and therefore the bigger the fraction of that space
of possibilities you can explore, hopefully thereby increasing the
chances of finding a good candidate. Because many attempts to find a drug
for a disease simply fail. Nothing. Nothing eventually comes of it.
So increasing the probability of success, increasing the speed of
that discovery process. So in all of that,
there are many places where machine learning could could be disruptive.
So on that process of, um, I guess you're describing,
you generate candidates and then you almost discriminate interesting ones,
and then you rinse and repeat in a kind of iterative process.
Let me give you a concrete example. So we've done some work looking
at tuberculosis. So tuberculosis kills something
like 1.3 million people very sadly back in 2022, which is the
last year we have we have data. And it might seem surprising because
we have we have antibiotics, we have drugs for tuberculosis.
Why are so many people dying? And one core reason is that the
bacterium is evolving to develop drug resistance.
And so there's a search on for new for new drugs.
So maybe I'll just take a moment to explain some of the architecture and
get into a little bit of the sort of the techie details of, of this.
So we wanted a way of finding we know what the target is.
We've been told what the target protein is.
The target has a region called a pocket, and we're looking for
molecules that will bind tightly with that pocket region on the protein.
And and so the way the way we approached this was, first of all,
build a language model, but not a language model for human language,
but for the language of molecules. So we first of all take there's
a representation representation called smiles.
It's a way of taking a it's an acronym.
It's just a way of taking a molecule and describing it as a
one dimensional string. And so you first of all,
take a large database of, I don't know, 10 million molecules
represented as smile strings, and you treat them like the tokens
for a, for a transformer model. And by getting it to predict the
next token, the next element of the smile string, you build a transformer
based language model that can speak the language of molecules.
So it can it can run generatively and it can create new create new
molecules as output. So you can think of that as kind of
like a foundation language model. But speaking the language of
molecules. Now we want to generate molecules,
but not just any molecules. We want molecules which bind with
a particular target protein. So we have the target protein in
particular. It's the pocket region that
we're interested in. So we can give it the amino acid
sequence of the protein as input. But we need more than that.
We need the geometry of the of the pocket.
And this is where some of those inductive biases come in.
So we we need to have representations of the geometry of
the atoms that form that pocket. But a way that represents these
equivariances. And so they're encoded as input
to a transformer model that learns a representation for the
protein pocket. And the final piece we need, as you
said, we want to do this iteratively. We want to take a good molecule and
make it a better molecule, rather than just searching blindly across a
space of ten to the 60 possibilities. And so the other thing we want to
provide is input is a molecule a descriptor of a of a of a of a
known small molecule that does bind with the pocket already.
And but we want to do this in a way that creates variability.
And we actually use a variational autoencoder to
create that representation. And and that's the an encoder
that translates the molecule into a latent space.
And we can sample from that latent space.
And then the this um language model, the Smiles language model can
attend to both the output of the variational auto encoder and the
output of the protein encoded using Cross-attention.
And so what we've done there is, I think, rather tastefully combined
some elements from, you know, state of the art modern deep learning.
The result then can be can be trained end to end using a
database of known other protein. Teens that are known to bind
efficiently to to small molecules. And once the system is trained,
we can now provide as input the known target for tuberculosis and some
known molecules that bind with this. And then we can iteratively refine
those molecules at the output. We get molecules that have
better binding efficiency. And we're able to increase the
binding effectiveness by two orders of magnitude.
And so we now have state of the art molecules in terms of
binding efficiency to this, um, to this, uh, target protein.
Of course, we can't do the wet lab experiments ourselves.
We partner with an organization called Gedi, the Global Health
Drug Discovery Institute. They've synthesized the
molecules that we've we've generated and measured their
their binding efficacy. And so we're very,
very excited about this. And of course, the next stage now
is to take that as a starting point and further refine and
optimize those molecules and, and try to address all those other
requirements that we have for, for a drug can actually be
tested on humans in terms of its toxicity and metabolism and all
and all the other things. But I think it's just a very,
a very nice example of almost like a first step in using modern deep
learning architecture to accelerate the process of drug discovery.
And already we have, I think, really quite a spectacular success,
given that we're we're kind of newcomers to this, to this field,
partnering with experts, domain experts, with the wet lab experience
and the wet lab capability. Um, to me, this is the beginning
of a very exciting journey. That sounds incredible.
Is there any kind of representational transfer between the models?
So, for example, um, you were talking about this, um,
this geometric prayad model and in a generating tokens to go
into the language model. Because just using language models,
by the way, is a fascinating approach.
I spoke with Christian Szigeti and he was doing mathematical conjecturing,
just using language models, you know, just just taking
mathematical constructions and putting them into language.
And they used to use graph neural networks for this.
And so I guess the question is, could you kind of bootstrap it with a,
you know, with an inductive, um, principled model and then kind of,
um, just train using the language model afterwards?
I think, I think the general principle
there is a very powerful one. So the idea of borrowing strength
from other domains, and I think we're seeing this time and time again in
deep learning that that the machine learning models are able to extract
some general patterns from, even from one domain and translate them
into a completely different domain. We talked earlier about large
language models being getting better at writing code.
If they've also got exposure to to poetry or something as
seemingly quite irrelevant. There's some there's something quite
deep and subtle going on there, but perhaps in a less subtle way,
it's clear there's a sort of a language of molecules,
there's a language of materials, and that by building models that have
a broader exposure to that language, they almost invariably will become
better at the specific tasks that we want to to apply them to.
So I think there is a general principle at heart there. Yeah.
It's so interesting because I used to think that that perhaps
the drawback of these inductive prior models is that it was one
inductive prior per model, but this ability potentially to,
um, bootstrap a foundational model that can do all of the
things that's really interesting. I think the most powerful inductive
biases are the ones we focus on, are really those very general ones,
symmetries. That's just a very fundamental
properties of the universe. And we we want we want those
really baked into the models. I think the, the,
the sort of intuitions we have about more specific domains.
I think they can perhaps lead us astray because they're based on
our experience of much more limited domains.
And I think this is where the machines can be, can be much better
at processing and and interpreting large volumes of data and drawing
regularities out of that, out of data in a more systematic way. Okay, okay.
And, um, just before we leave this, this is a bit of a galaxy brain
question, and, and that's parlance that all the kids
are using these days, by the way. But, um, how fundamental is is
our physical knowledge? You know, the question is like, we
are we're designing these inductive priors as if they are fundamental.
But folks like Stephen Wolfram, for example, argue that there's
there's a deeper ontological reality. You know, it might be a graph,
cellular automaton or something like that.
And is that something you think about, the kind of the gap between
our models and what reality is. So I think, first of all, one of the
greatest scientific discoveries of all time is the fact the universe
can be described by simple laws that that is not obvious a priori.
That itself is perhaps the most profound discovery, you know,
really going back to Newton. But we found it time and time again.
What we've also found is that the our understanding of the
universe as it exists today has, has, is almost like onions were
peeling away layers of onions. You know, Newton, if you want to
navigate a spacecraft to Jupiter, you still use Newton's laws of
motion and Newton's law of gravity. It's just fine.
Um, it doesn't mean we believe it's an exact description of nature.
We've now got deeper descriptions of nature. We understand relativity.
For example, general relativity tells us that actually Newton's
second law of motion, or Newton's Newton's law of gravity,
rather, is just an approximation. The inverse square law is a pretty
good approximation, but we've got a much better description now. But.
But it's, it's it's hard to say that we've
we've found the ultimate answer. It's rather that human knowledge is
just always stands on that, that edge of what we don't understand.
And scientific discovery is always about exploring the
things we don't understand, working out whether, um, you know,
whether the laws actually do hold. And the anomaly we see in the data
is, is because of some phenomenon that we haven't yet observed.
I mean, this is how Neptune was discovered by by seeing that the
planets were not behaving as they should do according to Newton's laws.
Newton's laws were just fine. It was just another planet
perturbing them. Uh, or is the precession of the
perihelion of Mercury because. Because there's another.
No, it's because actually the Newton's
law of gravity isn't quite right. We need relativity to understand
that. So I think scientific exploration,
as far as I can tell, uh, has no particular end in sight.
It's rather that we have things that we understand and there are
new frontiers. You know, when I was, uh,
when I was a teenager getting excited by physics, I loved reading about
relativity and quantum physics, but it's kind of depressing because I
thought I was kind of born, you know, 50 years too late or whatever.
You know, all the exciting stuff happened at
the beginning of the 20th century, and it's kind of all been done.
Uh, but now we have, you know, dark matter and dark energy, and we
realize that most of the universe isn't sitting on the periodic table
that I learned about in schools. And actually, um, I needn't have
worried, you know, um, uh, I think was it Vannevar Bush who
called it The Endless Frontier? Um, the, you know,
science is an endless frontier. There is just there is always more to
explore and always more to learn. So whether the particular ideas
you alluded to have substance, I don't know.
At the end of the day, the scientific method will tell us if
they have predictive capabilities. They can predict new phenomena that
we weren't aware of before then. Um, uh, then, you know,
then they have they have credence as far as a scientist is concerned.
But ultimately, you know, we still stick to the scientific method.
It's about our ability to make predictions that are testable
experimentally. And if they stand up to the test
of experiment, then we give more weight to those, to those hypotheses,
and eventually they're elevated to the status of theory.
I often wonder about the horizon of our cognition.
You know, what we are capable of, of understanding,
and we tend to understand things using high level metaphors.
Um, information is a great example of that.
So, you know, a lot of people talk about the universe as information.
Um, this essential view is quite interesting.
So modeling everything as, as, as agents.
And it might well be possible that the universe is just so
strange and alien that we could never possibly understand it.
So there's a bit of an interplay between our kind of intelligibility
and our models and what it is. And the universe clearly is
completely unintelligible in the sense of nobody can really, um,
think about quantum physics. It completely defies our everyday
intuitions that we learn at this sort of macroscopic level.
So I think we have to accept already that the universe is
described mathematically. That's our precise description.
And then we have kind of metaphors about waves and particles and so on.
But none of them, none of them really work properly.
They're just they're just crutches to lean on.
But ultimately it's a mathematical description.
But that that is that is also very interesting, the fact that the
world is described by mathematics, by making little marks on a piece
of paper, you can discover a new planet that's quite incredible.
Shifting over to to deep learning a little bit more, more broadly.
Um, we were touching on this already, but the landscape is dominated
by transformers architectures. What are your broad thoughts
about that? Like any field,
I think machine learning has its sort of its fads and its waves.
Something works really well, and then everybody latches on to
that and makes use of that and that. That's all well and good.
Um, I'd be kind of surprised if the transformer is the last word
in deep learning. If that's the, the the, the,
the architecture will be used forevermore.
Um, but it clearly works very well. And we haven't reached the end of
its capabilities by any means. So it makes a lot of sense to
exploit the transformer architecture in applications and
see how much we can gain from that. At the same time, there's clearly
opportunities to think about the limitations of transformers,
the computational costs. Can we do the same thing we know with
better scaling if we want longer context windows and all the rest.
So there's plenty of interesting research, I think, to be done in,
in new architectures as well. So I think we need both.
So, you know, here's another galaxy brain question.
Um, why does deep learning work? You know, because on on the face
of it, it shouldn't work. It shouldn't train,
it shouldn't generalize. And they've been an absolutely
remarkable success. Why is that? So I think first of all,
at one level you could say, well, we understand why they work.
We're fitting non-linear functions. We're kind of doing curve fitting
in high dimensional space. So we need some some generalization.
And it comes back to the no free lunch theorem, some inductive biases,
perhaps its smoothness continuity. Perhaps it's something more more
constraining than that. So at one level it's sort of not
surprising. I can I can fit a polynomial to
a bunch of data points and by gradient methods,
and I can make good predictions for sort of intermediate points,
just, just generalizing that to more data and higher dimensions.
So, so at one level I say no, it's not at all surprising.
They work at a different level. Of course, the fact they work so
well is remarkable, but the way in which they work is very interesting.
So one thing which, um, if we go back to the earlier.
Years of machine learning, and certainly back to the world
of statistics. The idea that you would fit models
that have way more parameters than the number of data points would be
clearly insane to any self-respecting statistician. We never would have.
And perhaps that's why nobody really tried it very much.
Um, and yet we have these odd phenomena whereby, you know,
the training error goes to zero, and yet the test error continues
to come down, even though the training error is already at zero.
Uh, something about stochastic gradient descent.
Um, the actual training process clearly is important there.
It's not just here's a cost function. We find the global minimum.
It's a property of the global minimum.
No, there are many, many global minima that all have zero error.
Some solutions will clearly overfit. Others generalize well.
And so there's something about the training process that we
need to understand. So I think there's a lot of
research to be to be done in. Why do they work so well I think
it's an open question. We can describe the model.
We can say lots of things about the model.
We can say because it has this and this and that number of layers.
Therefore, the structure of the space has this and that properties and that
divides it up into such and such regions and so on. Those are true.
I don't know whether that gives us real insights into why it's working.
I think there are some, some very much open questions there.
It strikes me a little bit like neuroscience.
You know, we have the human brain. It does these amazing things.
And we can get more and more and richer and richer data about which
neurons are firing and when and how the firings are correlated.
We can learn something about the the underlying machinery.
This is a bit like neuroscience, except we can put a probe in every
neuron in our artificial brain and gather very, very rich information.
So again, I think there's a very interesting research frontier of
of getting better understanding of why are they able to generalize
so well and why do we have these strange phenomena with these
seemingly overparameterized models that don't overfit, but rather
have very good generalization? Lots of research to be done and
just to linger on that, that observation you made that you
can train a deep learning model. And after the training error has
converged, the test loss continues to improve.
I mean, that just seems it just doesn't make sense.
I mean, and that there's Grokking as well, which is another um,
it is almost like we were saying with physics that outside of the
the machinations of the optimization algorithm stuff is
happening that we don't understand. Well, you can tell stories, right?
You can say there's a there's a big space.
Each point in the space is a setting for all the parameters of the model.
So the sort of the weight space of the model, and maybe you started
off somewhere near the origin with some little random initialization.
Then you follow some trajectory that's defined by stochastic
gradient descent. And there are lots and lots of
places in this space, all of which have zero training error.
So and they're connected. So there's some sort of manifold
of zero training error. And you're starting off at the
origin. And stochastic gradient descent
is somehow not taking you in a random way.
Maybe it's taking you to something like, you know, the nearest point
on this manifold or something, and that maybe that's some kind
of regularization and maybe that, that that place has certain
smoothness properties that lead to good generalization.
So you can kind of tell these stories.
I think the challenge is to take the stories and make them predictive.
So I think when we have a theory, when we have a theory of what's going
on, we'll know we have a theory because it can predict new things,
not just tell stories about what we've already discovered empirically,
but really become predictive. I think that's still very much
an open question. So, um, what do you think about
the the intelligibility of, of neural networks in terms of things
like bias and fairness and safety? Um, because you could just think
of these things as inscrutable, um, bags of neurons.
And, but we need to have some guardrails, don't we?
Well, we absolutely need to create technology that's
beneficial to humanity. There's no question about that.
And there are mechanisms for doing that to to align the systems,
whether it's to through, you know, human feedback, whether it's external
guardrails that are providing more conventional sort of checks
on on how things are being used. That's,
that's that's clearly necessary. And I find it very encouraging
that so much energy and effort is going into this.
And yes, there will be there'll be bumps in the road and
missteps on the way for sure. Um, but overall, we seem to be
heading in a very good direction. But I think the fact that there is a
lot of attention being paid to the potential risks associated with this
very powerful and very general new technology gives me hope that we will
avoid most of the the biggest risks. Can you give me a specific example
of an emulator? Yes I can. So one very nice example.
Actually it was the final project I worked on, uh, when I was
working in the fusion program. So I was using fusion as a sort of
springboard to get into machine learning, and we wanted to do real
time feedback control of a fusion experiment, a thing called a tokamak,
very high temperature plasma. We wanted to use neural nets to
do nonlinear feedback. So the challenge there was to
take a plasma. It's like a donut shaped ring of
hot plasma. And it was known that if you could
change the cross sectional shape, you could improve its performance.
So there's an experiment called a Compass Compact assembly at
Culham in Oxfordshire. And the experiment is designed
to produce very interesting, exotic cross sectional shapes to
to explore the performance. So we want to use a neural net
to do that. Feedback control. Now the good news is we had a great
piece of inductive bias, a thing called the grad Shafranov equation.
It's a second order elliptic partial differential equation.
But the point is it. Describes the boundary of the
plasma very accurately. Right? So you make a bunch of measurements
from hundreds of little pickup coils around the plasma.
And those are boundary conditions. You solve the grashoff runoff
equation. You know, the shape of the plasma.
And the goal was to decide ahead of the time that you wanted to
create a circular plasma and then change its shape and, and,
and then make corrections. If the shape wasn't quite the
one you wanted, you would change the the big control
coil currents and alter it shape. The problem was the Grad Trifonov
equation on a state of the art workstation of the day would
take 2 or 3 minutes to solve, whereas we had to do feedback on a
sort of 20kHz frequency or something. It was about something like six
orders of magnitude too slow. So what we did instead was we we
solved the Grad Trifonov equation many times on the, on the
workstation, um, over a period of, you know, days and weeks until
we built up a large database of, of known solutions along with
their magnetic measurements. And then we trained a neural network,
just a simple two layer neural network back in the day, um,
with probably only a few thousand parameters.
I mean minuscule by modern standards, but it was trained to take the
magnetic measurements and predict the shape, and we could put that
into a standard feedback loop. And and we're in a bit of a race
with another organization that was doing a similar thing,
a different fusion lab that was working on the same project.
And so that was very motivating. And I'm pleased to say we got
there first, and we did the world's first ever real time
feedback control of a tokamak plasma using a neural network.
But it was a beautiful example of a of a of an emulator.
We could get 5 or 6 orders of magnitude speed up,
not by solving the equation directly to do feedback control,
but by using the numerical solver to generate training data and using
the training data to train the emulator and then the emulator.
And even then it was still quite demanding for the silicon of the day.
Uh, there was no process of fast enough.
So we actually built a physical implementation of the neural net,
believe it or not. So it was a hybrid, um,
analog digital system. It had an analog signal pathway
with analog sigmoidal units, but the weights were set using
digitally set resistors. So we could take the numerical
output of the the emulator, download it into this bespoke
hardware physical neural network and do real time feedback control.
So pretty pretty excited about that project. That's fascinating.
What do you think about, um, control now?
Do you have any opinions on, you know, model predictive control.
And control is a super important area different.
Both both the both the control problem and the overall planning
problem? I think, um, despite all the
remarkable advances in GPT four, the world of instantiated AI and
robotics and so on is still a very, very wide open frontier.
Um, we don't we don't really have robots that can even yet drive a
car through central London. That's still a major challenge
that we're seeing some very remarkable progress recently. Yeah.
I mean, more broadly, um, I've been speaking with some
neuroscientists and they say that we have the matrix in our heads,
so we're always running simulations. And presumably in the future
this will be a principled way of building agents.
So the agents will run counterfactual simulations and select trajectories
which look like good ones. And then the process will will
iterate. I think this is very powerful.
I mean, the idea of sort of type one and type two, fast learning,
slow learning, the idea that we simulate the world
and we compare the simulation with the reality and we can learn from
our own simulators and so on. We don't we don't quite know
what best to do with that, but it feels such a powerful and
compelling concept. And we we think something like that
is going on in the brain that, again, that feels like an area
that's ripe for exploration. And I think in some form, some kind
of, you know, model prediction and simulation of the world feels like
it will be increasingly a part of AI systems as we go forward.
I mean, for me, the takeaway in all of this is just what an
amazing time to be in this field. There are so many fascinating
things to work on. Professor Bishop, it's been an
honor to have you on MLS. Thank you so much.
Well thank you I've enjoyed it. Thank you. Amazing.
Browse More Related Video

Heroes of Deep Learning: Andrew Ng interviews Geoffrey Hinton
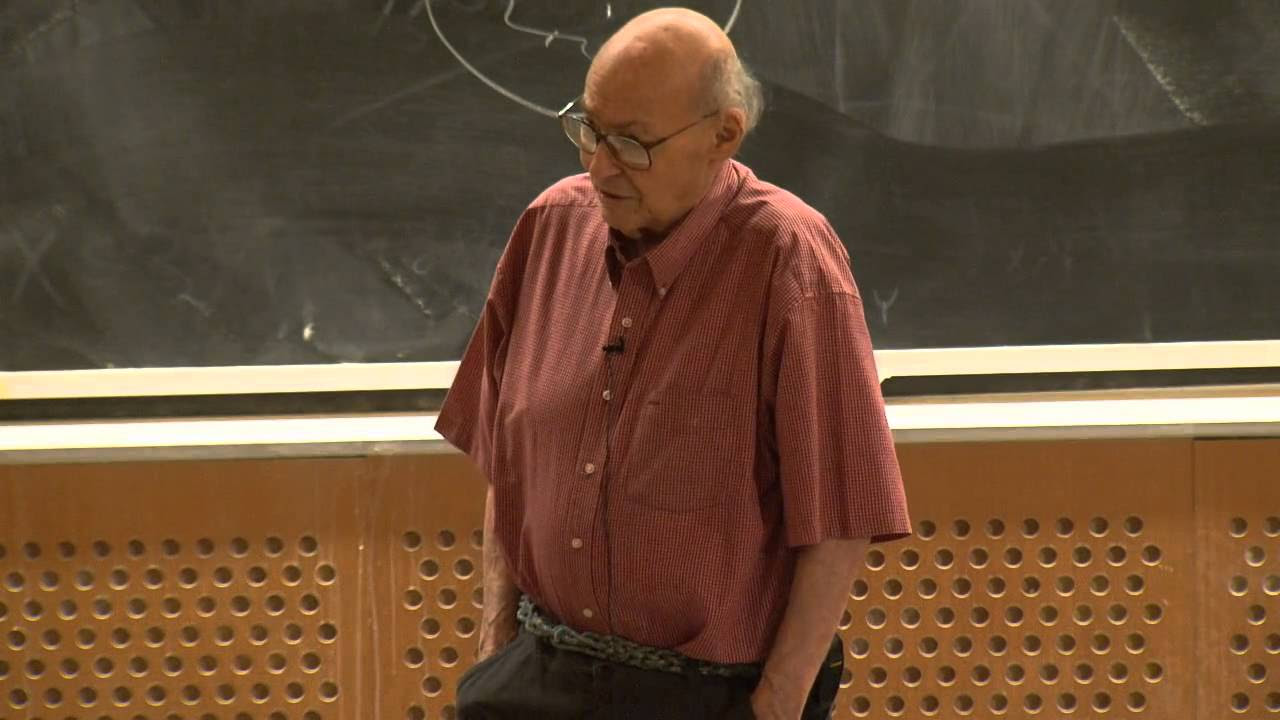
4. Question and Answer Session 1
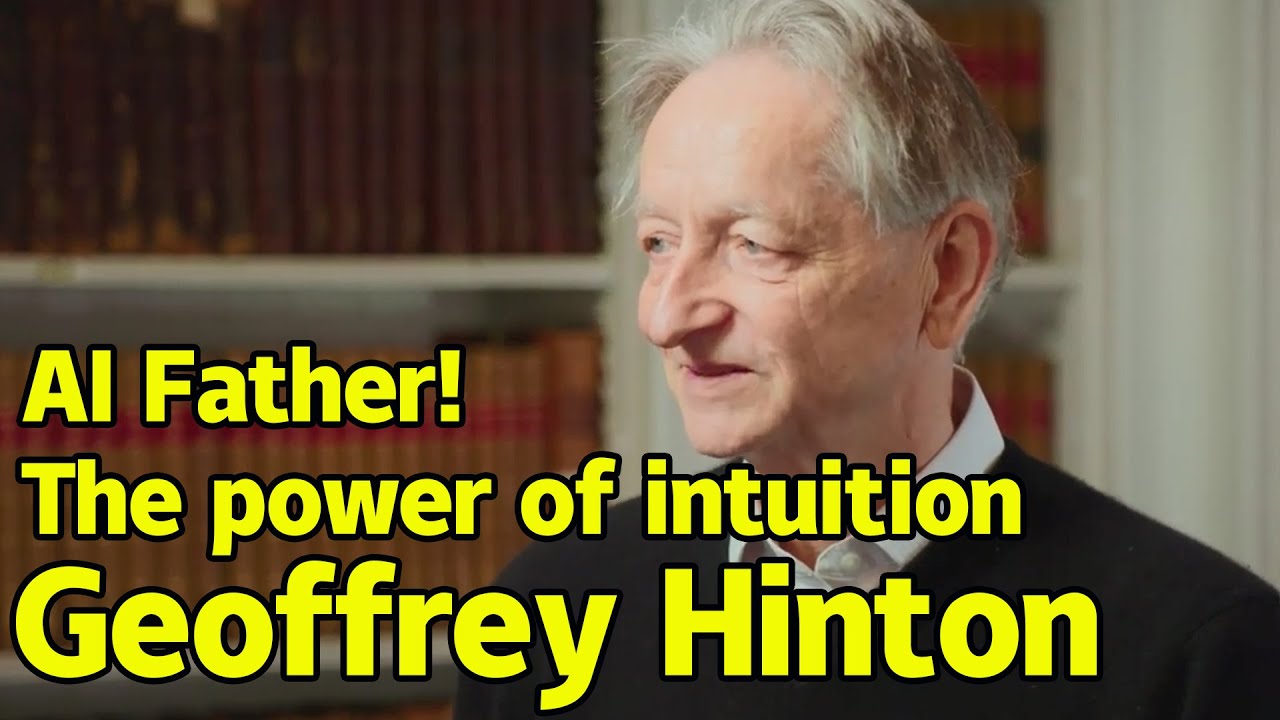
Geoffrey Hinton | Ilya's AI tutor Talent and intuition have already led to today’s large AI models
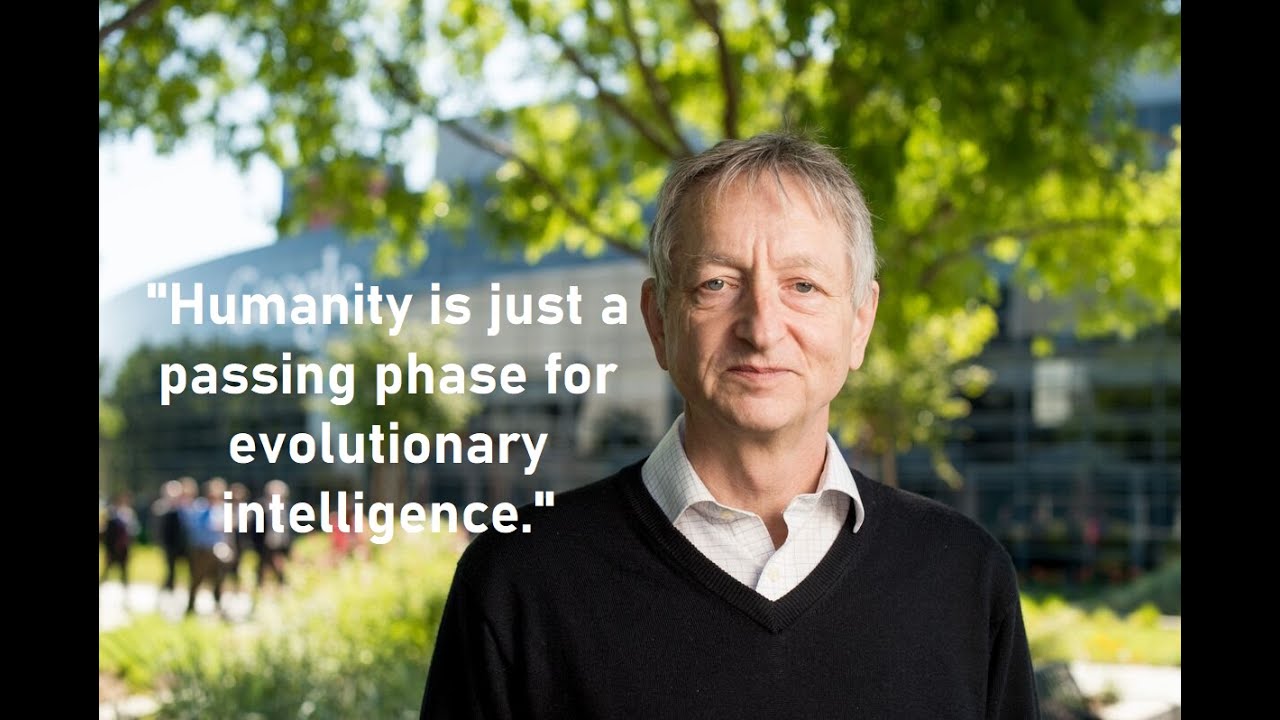
Possible End of Humanity from AI? Geoffrey Hinton at MIT Technology Review's EmTech Digital
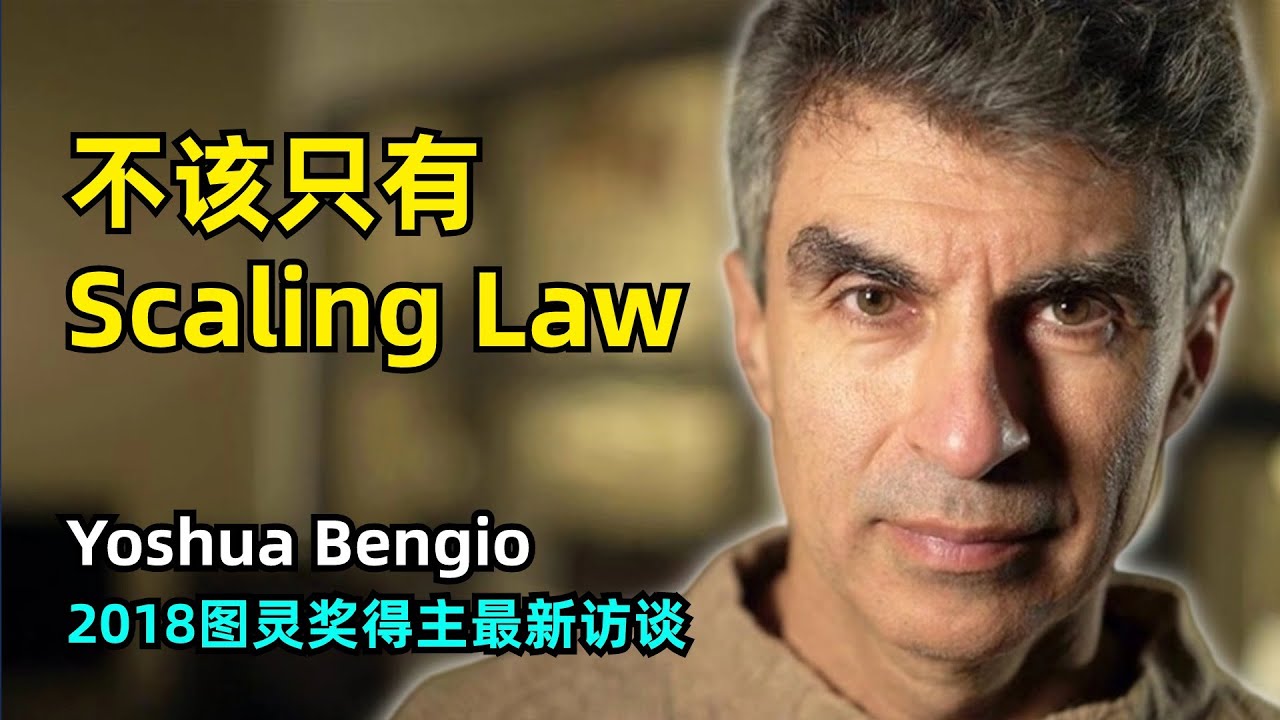
【人工智能】图灵奖得主Yoshua Bengio最新访谈 | 不应该只有Scaling Law | 深度学习三巨头 | 学术生涯 | 神经网络 | 系统2 | AI safety | 如何科学研究
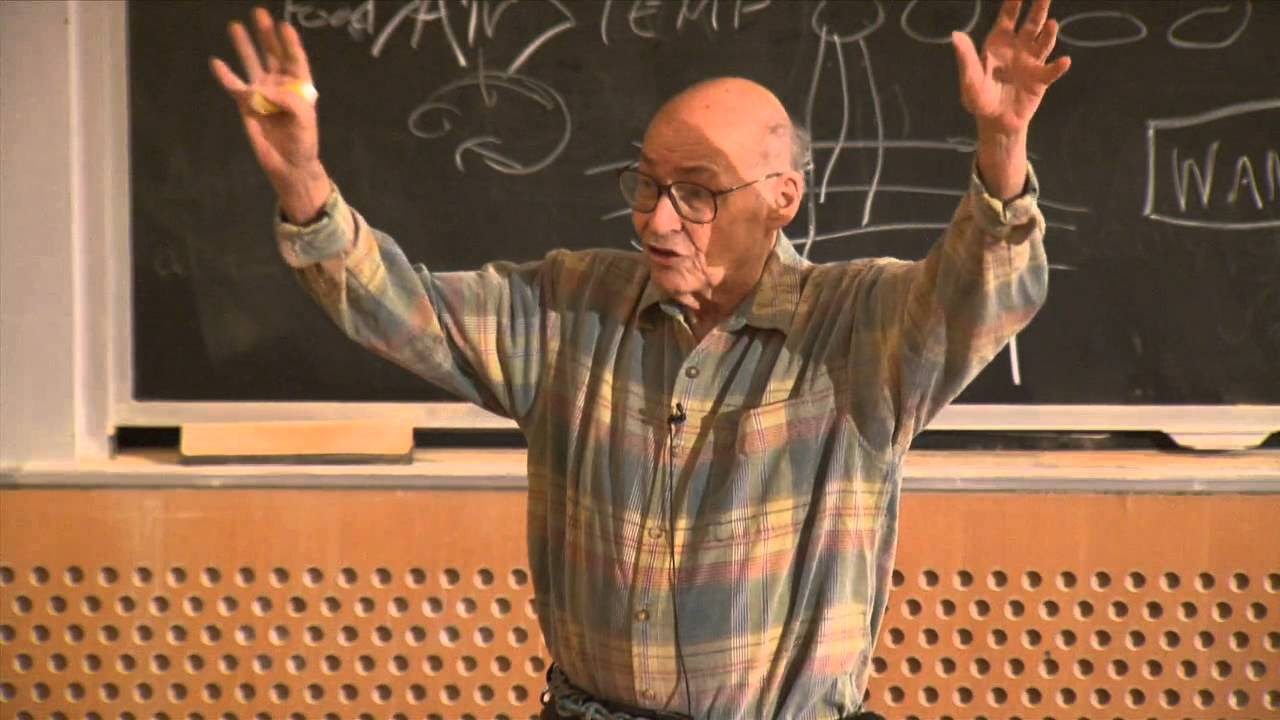
7. Layered Knowledge Representations
5.0 / 5 (0 votes)