Sampling Distributions (7.2)
Summary
TLDRThis video explains the concepts of sample distributions and sampling distributions, illustrating their differences using the example of height measurements in a population. It details how a sample mean can vary from the population mean due to sample size and variability. The video highlights that a sampling distribution is formed from multiple sample means, demonstrating the central limit theorem's effect on normal distribution. Finally, it provides practical examples of calculating probabilities using both sampling and population distributions, emphasizing the efficiency of sampling methods in estimating population parameters.
Takeaways
- 😀 A sample is a smaller portion of a population used to draw conclusions, but its mean may differ from the population mean due to variability.
- 📊 A sample distribution refers to the distribution of data from a single sample, while a sampling distribution involves multiple samples and their statistics.
- 🔍 The process of creating a sampling distribution involves taking random samples, calculating their means, and plotting these means on a histogram.
- 📉 The central limit theorem states that with enough samples, the sampling distribution of the mean will approach a normal distribution.
- 📈 The mean of a sampling distribution (x̄) is equal to the mean of the population (μ), while its standard deviation (standard error) is smaller.
- 🔢 The standard deviation of a sampling distribution is calculated as the population standard deviation (σ) divided by the square root of the sample size (n).
- 🤔 The concept of sampling distributions helps in estimating population parameters without measuring the entire population.
- 🎯 Sampling distributions allow for calculating probabilities of certain outcomes based on sample sizes.
- 📐 To standardize a value from a sampling distribution, use the formula: (x - μ) / (σ / √n).
- 🌍 Understanding population vs. sampling distributions is crucial in statistics for making inferences about large groups based on limited data.
Q & A
What is the main difference between a sample distribution and a sampling distribution?
-A sample distribution refers to the distribution of a single sample drawn from a population, while a sampling distribution is formed by taking multiple random samples and calculating a statistic (like the mean) for each, then plotting those statistics.
Why does a sample mean not always equal the population mean?
-Because samples are smaller and have inherent variability, their means can differ from the population mean, which is based on all individuals in the population.
What does the central limit theorem state about sampling distributions?
-The central limit theorem states that with a large enough sample size, the sampling distribution of the sample mean will be approximately normally distributed, regardless of the population's distribution.
How do you calculate the standard deviation of a sampling distribution?
-The standard deviation of a sampling distribution (standard error) is calculated by dividing the population standard deviation by the square root of the sample size (n).
What is the standard error?
-The standard error is the standard deviation of a sampling distribution, indicating how much variability there is in the sample means relative to the population mean.
In the context of height, how would you find the probability that the average height of a sample is less than a specific value?
-You would standardize the sample mean using the z-score formula and then consult a z-score table to find the corresponding probability.
What does a z-score represent in this context?
-A z-score indicates how many standard deviations an observation is from the mean, helping to determine the probability of a certain outcome in a normal distribution.
Why is it more efficient to use sampling distributions instead of measuring an entire population?
-Measuring every individual in a large population is time-consuming and costly; sampling allows us to make inferences about the population from a smaller, manageable group.
What happens to the spread of the sampling distribution compared to the population distribution?
-The spread (standard deviation) of the sampling distribution is always smaller than that of the population distribution because averages (sample means) are less variable than individual observations.
How do you find the proportion of individuals above a certain height using population distribution?
-You standardize the height value to find its z-score, then use the z-score table to determine the area to the left, and subtract this from 1 to find the area to the right, representing the proportion above that height.
Outlines
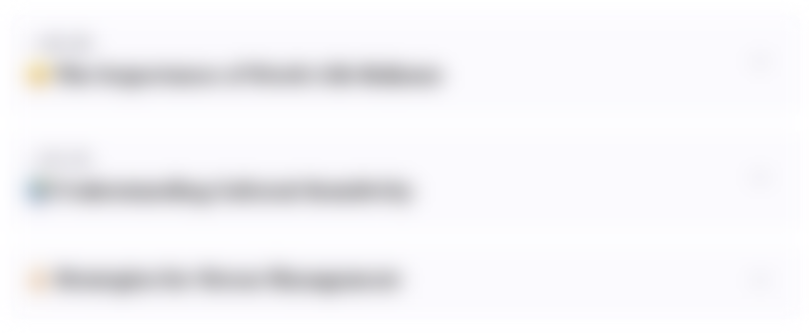
Этот раздел доступен только подписчикам платных тарифов. Пожалуйста, перейдите на платный тариф для доступа.
Перейти на платный тарифMindmap
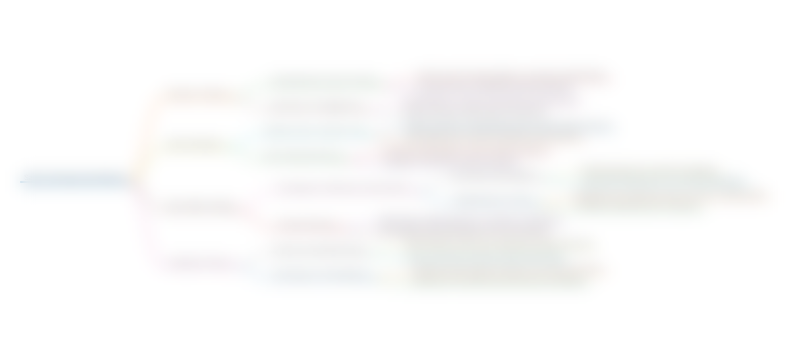
Этот раздел доступен только подписчикам платных тарифов. Пожалуйста, перейдите на платный тариф для доступа.
Перейти на платный тарифKeywords
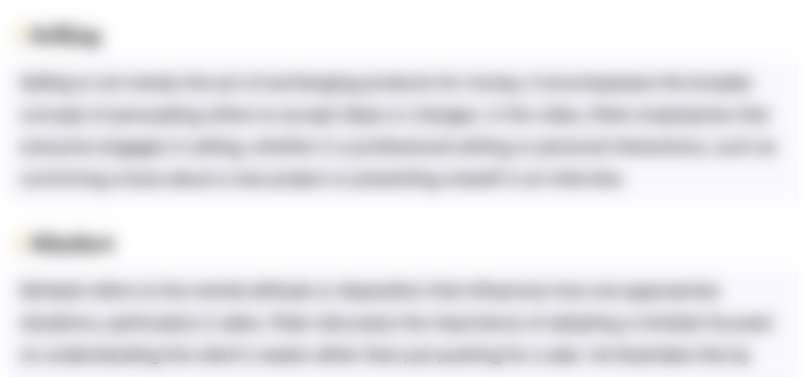
Этот раздел доступен только подписчикам платных тарифов. Пожалуйста, перейдите на платный тариф для доступа.
Перейти на платный тарифHighlights
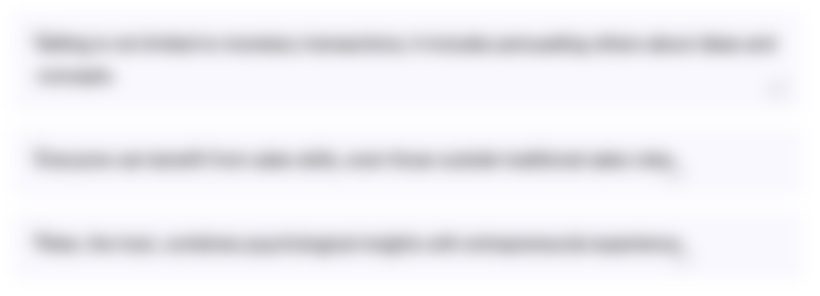
Этот раздел доступен только подписчикам платных тарифов. Пожалуйста, перейдите на платный тариф для доступа.
Перейти на платный тарифTranscripts
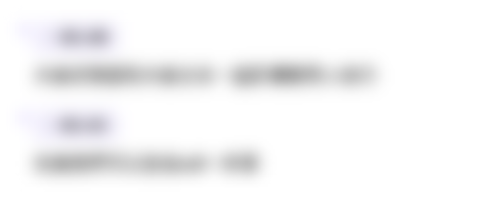
Этот раздел доступен только подписчикам платных тарифов. Пожалуйста, перейдите на платный тариф для доступа.
Перейти на платный тарифПосмотреть больше похожих видео
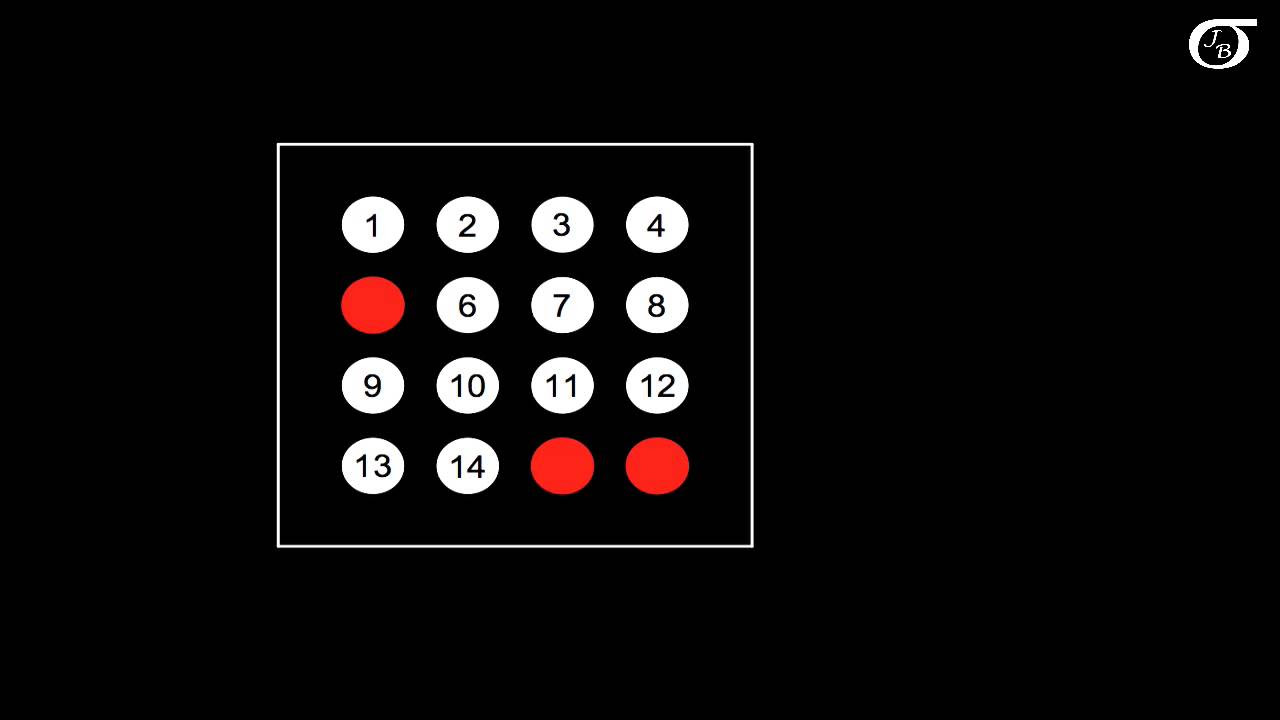
Sampling Distributions: Introduction to the Concept

Sampling distributions
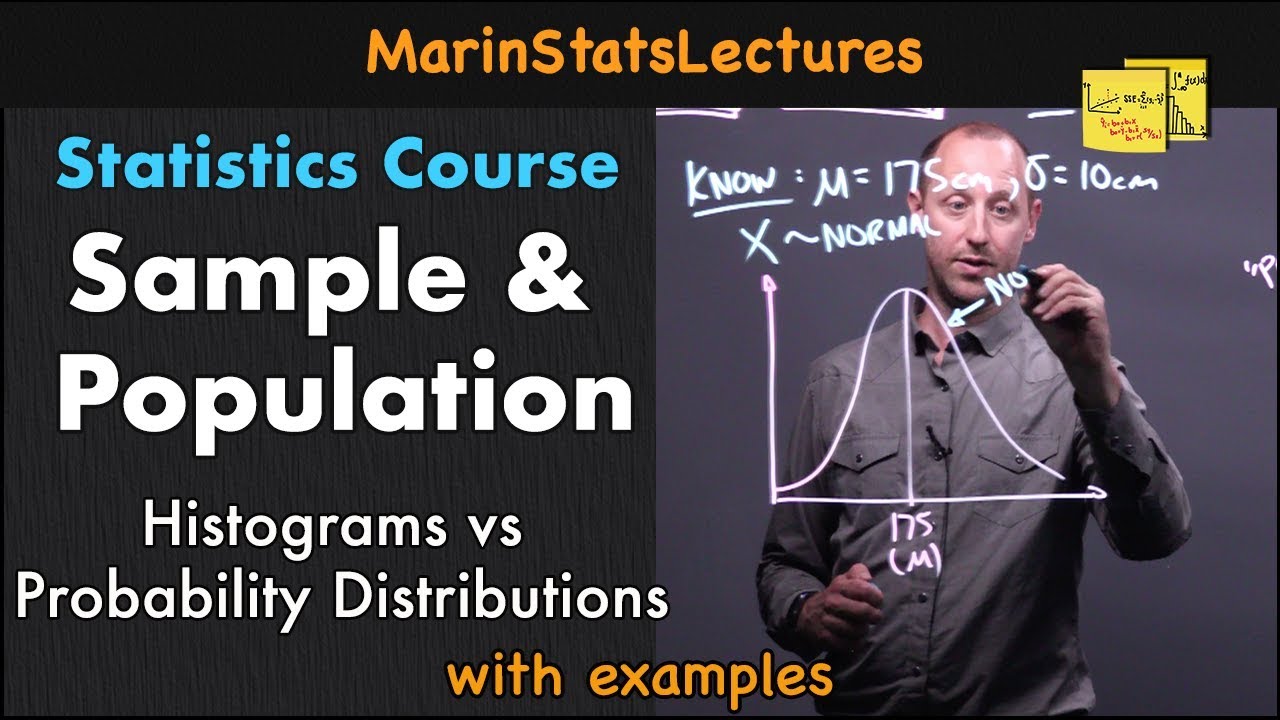
Sample and Population in Statistics | Statistics Tutorial | MarinStatsLectures
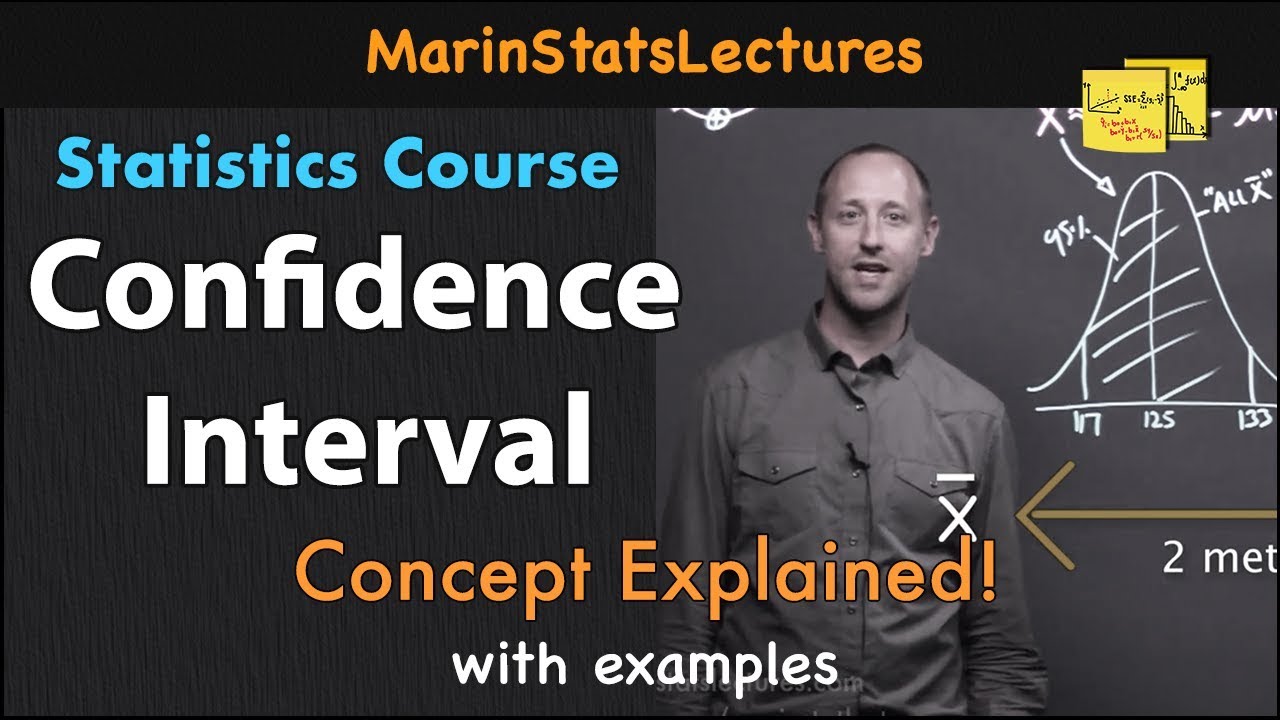
Confidence Interval Concept Explained | Statistics Tutorial #7 | MarinStatsLectures
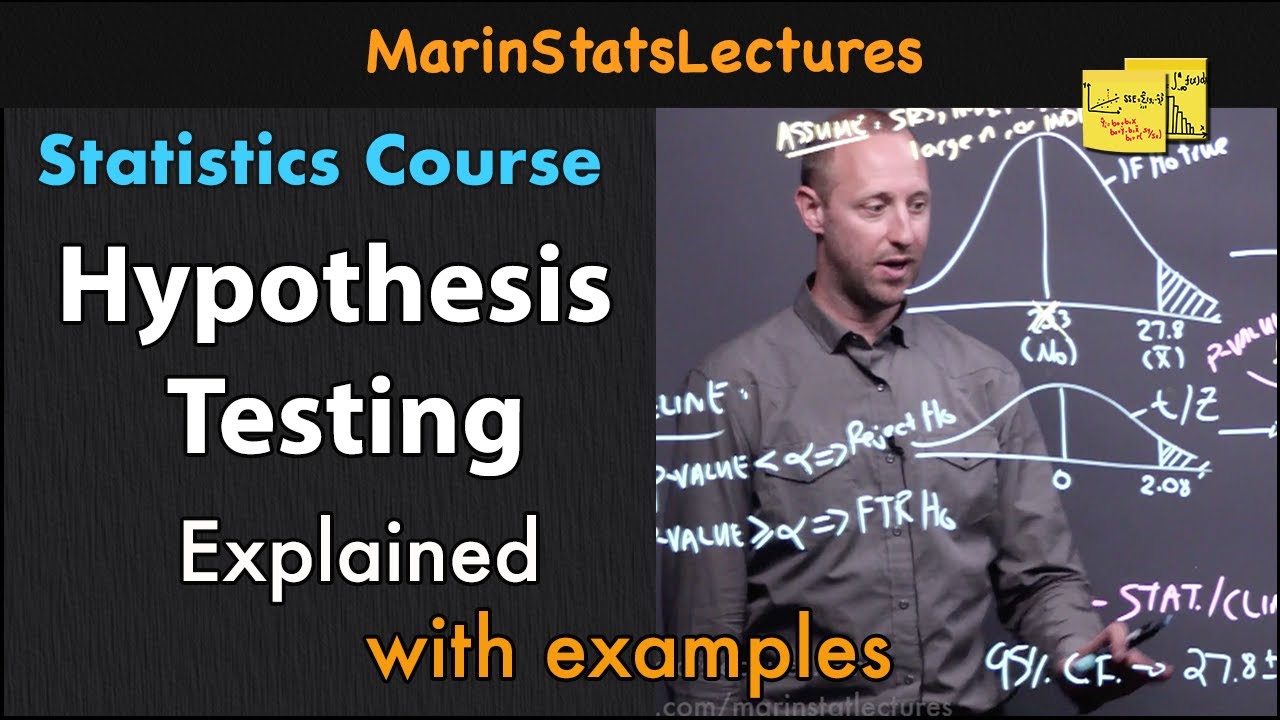
Hypothesis Testing Explained | Statistics Tutorial | MarinStatsLectures
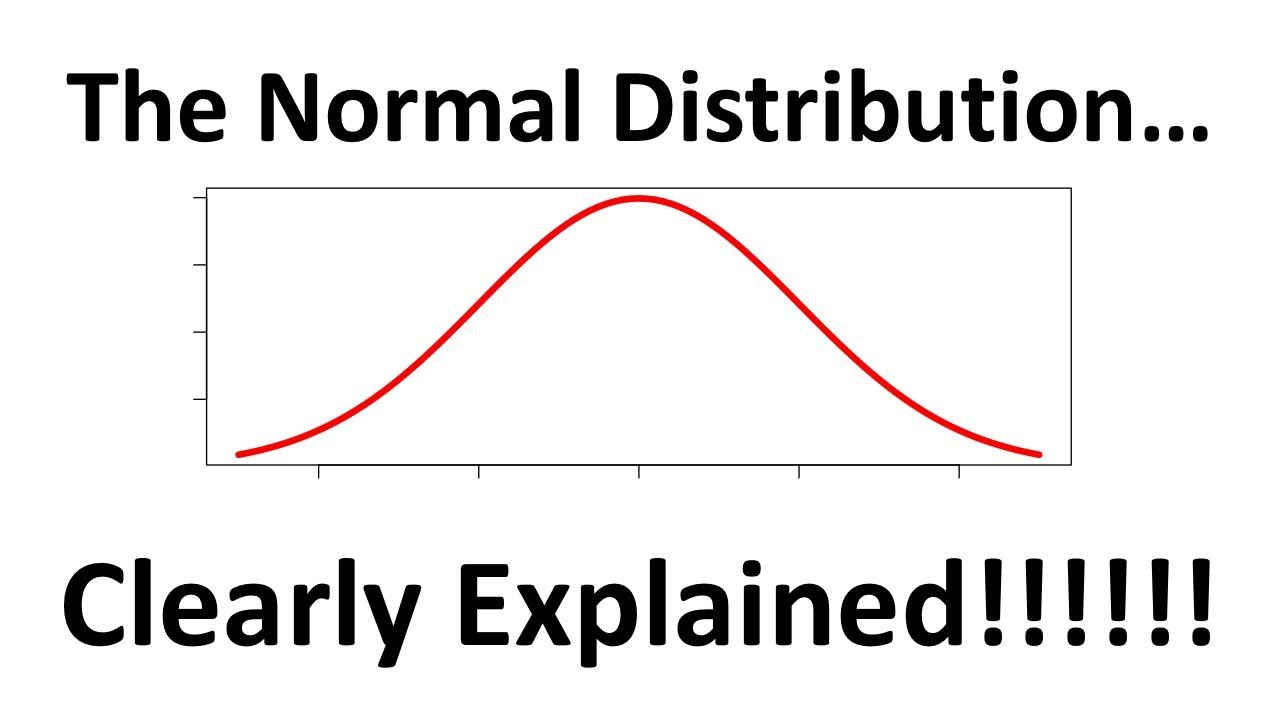
The Normal Distribution, Clearly Explained!!!
5.0 / 5 (0 votes)