Statistical Power, Clearly Explained!!!
Summary
TLDRIn this StatQuest episode, Josh Starburns explains the concept of statistical power using the example of mice on different diets. Power refers to the probability of correctly rejecting the null hypothesis, which is influenced by the difference between distributions and sample size. The episode highlights how overlapping distributions and small sample sizes can lower power, while increasing sample size enhances it. Starburns also emphasizes the importance of power analysis in determining how many measurements are needed to achieve sufficient power, making statistical tests more reliable.
Takeaways
- π Power is the probability that we will correctly reject the null hypothesis.
- π A small p-value (e.g., <0.05) allows us to reject the null hypothesis and conclude that there is a significant difference between two groups.
- π When there is little overlap between two distributions, there is a high probability of correctly rejecting the null hypothesis.
- π If the two distributions overlap significantly, a higher chance exists of failing to reject the null hypothesis, leading to a larger p-value.
- π Power is influenced by sample size; larger sample sizes typically increase statistical power.
- π If we get a large p-value in an experiment, it means we failed to reject the null hypothesis, even though we might know the data comes from different distributions.
- π Power analysis helps determine the number of measurements needed to achieve a desirable level of statistical power.
- π A small sample size with overlapping distributions can result in low statistical power.
- π A power analysis can be used to plan experiments and ensure a sufficient sample size for detecting real effects.
- π Even with overlapping distributions, increasing the sample size can increase the likelihood of rejecting the null hypothesis correctly.
Q & A
What is statistical power?
-Statistical power is the probability that we will correctly reject the null hypothesis when it is false. In other words, it measures the likelihood that a statistical test will detect an effect when there actually is one.
How does overlap between distributions affect statistical power?
-When two distributions overlap a lot, the statistical power decreases. This means there is a lower probability of correctly rejecting the null hypothesis, as it becomes harder to distinguish between the two distributions.
What happens when the p-value is less than 0.05?
-When the p-value is less than 0.05, we reject the null hypothesis, suggesting that there is a statistically significant difference between the two datasets or groups being compared.
What does a large p-value indicate?
-A large p-value (greater than 0.05) indicates that there is insufficient evidence to reject the null hypothesis. This means that, despite differences between the distributions, the test fails to find statistical significance.
Why is statistical power important?
-Statistical power is important because it helps to determine the likelihood of detecting a true effect when performing an experiment. Higher power reduces the chance of making a Type II error (failing to detect a difference when one exists).
How can statistical power be increased?
-Statistical power can be increased by increasing the sample size. A larger sample size reduces variability and makes it easier to detect a true effect if one exists.
What is a power analysis?
-A power analysis is a method used to determine the sample size needed to achieve a desired level of power. It helps researchers plan their experiments so that they have enough power to detect meaningful differences.
What role does the sample size play in statistical power?
-The sample size plays a crucial role in statistical power. A larger sample size typically leads to higher power because it reduces variability and improves the precision of the statistical test.
What happens when the sample size is too small?
-When the sample size is too small, statistical power decreases. This makes it less likely to detect a true effect, even if one exists, leading to a higher probability of Type II errors.
Can we have statistical power when there is no difference between two distributions?
-No, if there is no actual difference between the two distributions, the concept of statistical power doesnβt apply because there is no effect to detect. Power is relevant only when there is a genuine difference between the groups being compared.
Outlines
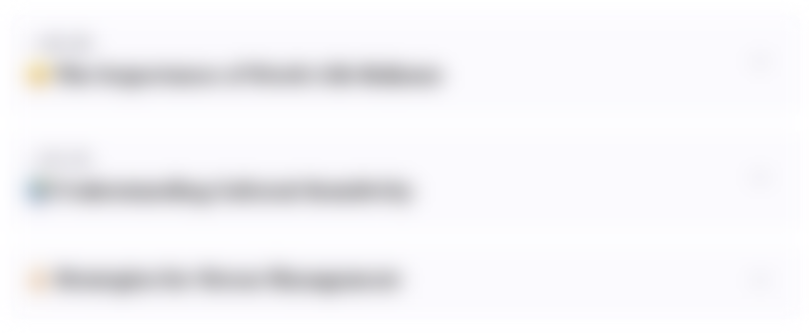
This section is available to paid users only. Please upgrade to access this part.
Upgrade NowMindmap
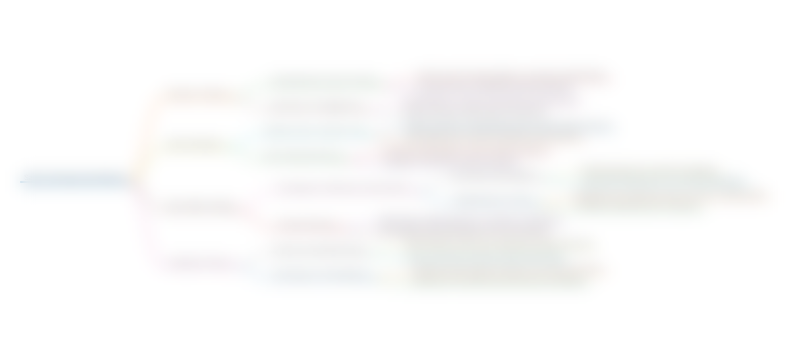
This section is available to paid users only. Please upgrade to access this part.
Upgrade NowKeywords
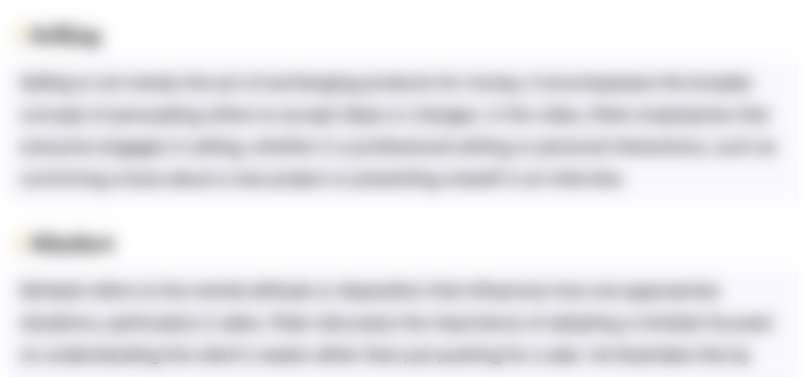
This section is available to paid users only. Please upgrade to access this part.
Upgrade NowHighlights
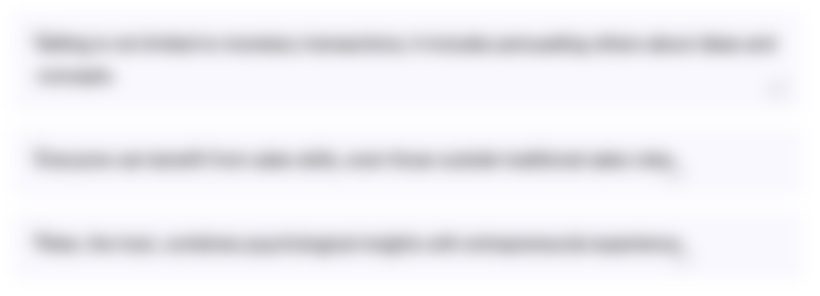
This section is available to paid users only. Please upgrade to access this part.
Upgrade NowTranscripts
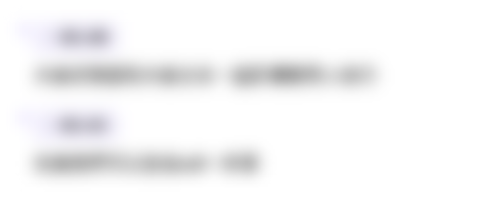
This section is available to paid users only. Please upgrade to access this part.
Upgrade Now5.0 / 5 (0 votes)