How to Tally, Encode, and Analyze your Data using Microsoft Excel (Chapter 4: Quantitative Research)
Summary
TLDRThis lesson introduces quantitative data analysis, explaining how to prepare, organize, and analyze data using statistical tools. It covers key concepts such as coding data, using software like SPSS and Excel, and presenting data through graphs and charts. The video also explores different statistical techniques, including descriptive statistics (mean, median, mode, and standard deviation) and advanced methods like correlation, regression, and ANOVA. Practical examples show how to apply these methods to research data, interpret results, and determine significant relationships or differences between variables, making complex data analysis accessible for beginners.
Takeaways
- 📊 Quantitative data analysis simplifies the interpretation of data through statistical tools like SPSS or Excel.
- 📋 The first step in quantitative data analysis is data preparation using a coding system to convert words, images, or pictures into numerical values.
- 🖥️ Microsoft Excel or SPSS is used to input and process data from surveys, with Excel being appropriate for smaller datasets.
- 📉 Different types of graphs are used based on the data type: bar graphs and pie charts for nominal data, histograms or frequency polygons for interval or ratio data.
- 🔢 Data tabulation involves organizing data into categories and calculating percentages, such as the gender distribution in a survey.
- 📈 Descriptive statistical techniques include frequency distribution, measures of central tendency (mean, median, mode), and standard deviation.
- 🔬 Advanced analytical methods include correlation, ANOVA, and regression to determine relationships and predictions between variables.
- 📊 Coding data into Excel involves assigning numerical values to variables (e.g., 1 for male, 2 for female) and analyzing responses using Likert scales or other metrics.
- 📑 Descriptive statistics can help rank and interpret items based on their mean, median, and standard deviation, providing insights for research papers.
- 🧪 Statistical tests like t-tests are used to determine if there is a significant difference between variables, with interpretation based on the t-stat value compared to a standard alpha value.
Q & A
What is the first step in quantitative data analysis?
-The first step in quantitative data analysis is preparing your data using a coding system, where words, images, or pictures are converted into numbers to make them suitable for analytical procedures.
What tools are commonly used for data entry in large and small studies?
-For large, complex studies, SPSS (Statistical Package for the Social Sciences) is commonly used, while for smaller datasets, Microsoft Excel is often sufficient.
What types of graphs are appropriate for different data types?
-For nominal data, bar graphs and pie charts are used. Ordinal data also uses bar graphs, while interval and ratio data are often represented with histograms or frequency polygons.
What are the basic methods of analyzing quantitative data?
-Quantitative data can be analyzed using descriptive statistical techniques such as frequency distribution, measures of central tendency (mean, median, mode), and standard deviation. Advanced methods include correlation, ANOVA (analysis of variance), and regression analysis.
What is the role of standard deviation in quantitative analysis?
-Standard deviation measures the extent of variation or dispersion in a set of data values from the mean, helping to understand how spread out the data points are.
What is the difference between correlation and regression in data analysis?
-Correlation measures the strength and direction of a relationship between two variables, while regression goes further to describe the nature of that relationship and whether one variable can predict another.
When would you use parametric vs. non-parametric tests?
-Parametric tests are used for interval and ratio data, where assumptions about the data's distribution are made. Non-parametric tests are used for nominal or ordinal data that do not meet these assumptions.
What is the Pearson’s r coefficient used for?
-Pearson’s r coefficient is used to measure the strength and direction of the relationship between two variables, where values closer to 1 or -1 indicate a stronger relationship.
How do you interpret a correlation coefficient value of 0.57?
-A correlation coefficient of 0.57 suggests a moderate positive relationship between the two variables, as it is closer to 1 than to 0.
How can you determine if there is a significant difference between two variables using a t-test?
-To determine if there is a significant difference, compare the t-test result (t-stat) to the alpha value (0.05). If the t-stat is greater than 0.05, there is a significant difference. Otherwise, there is no significant difference.
Outlines
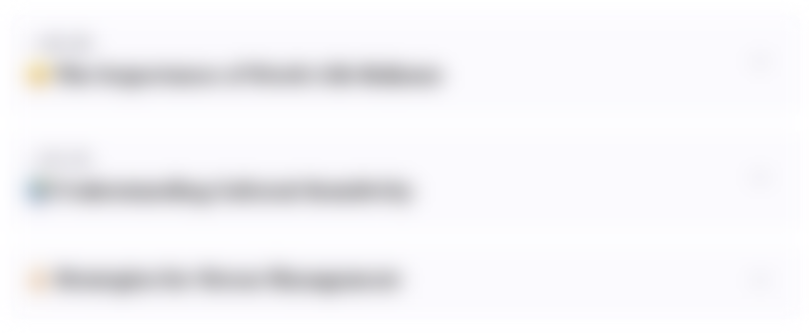
Этот раздел доступен только подписчикам платных тарифов. Пожалуйста, перейдите на платный тариф для доступа.
Перейти на платный тарифMindmap
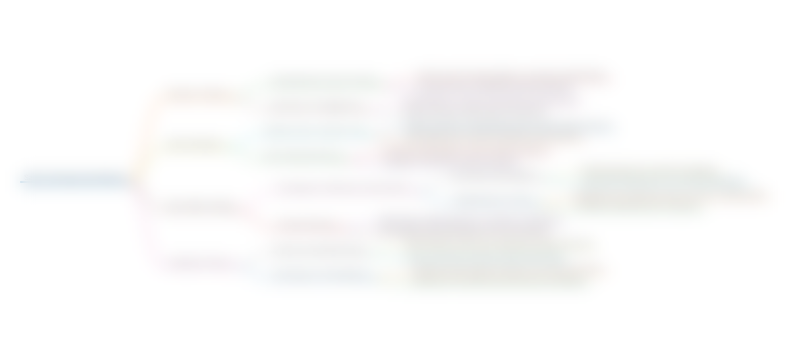
Этот раздел доступен только подписчикам платных тарифов. Пожалуйста, перейдите на платный тариф для доступа.
Перейти на платный тарифKeywords
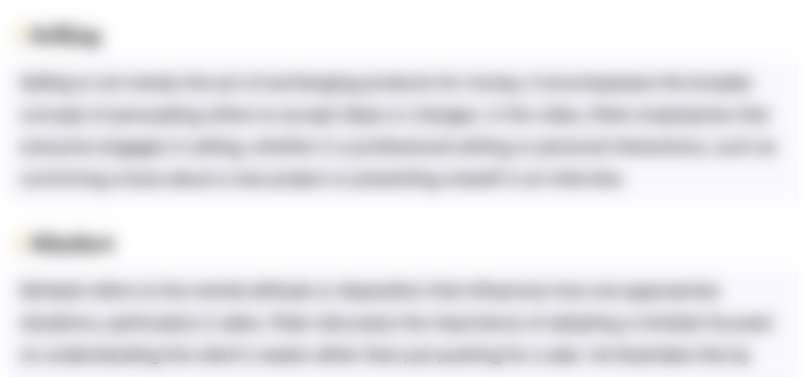
Этот раздел доступен только подписчикам платных тарифов. Пожалуйста, перейдите на платный тариф для доступа.
Перейти на платный тарифHighlights
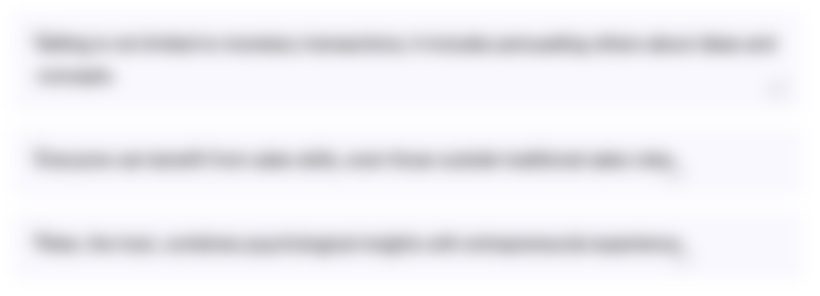
Этот раздел доступен только подписчикам платных тарифов. Пожалуйста, перейдите на платный тариф для доступа.
Перейти на платный тарифTranscripts
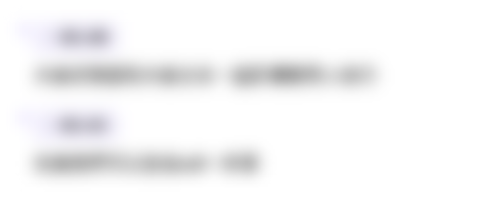
Этот раздел доступен только подписчикам платных тарифов. Пожалуйста, перейдите на платный тариф для доступа.
Перейти на платный тарифПосмотреть больше похожих видео
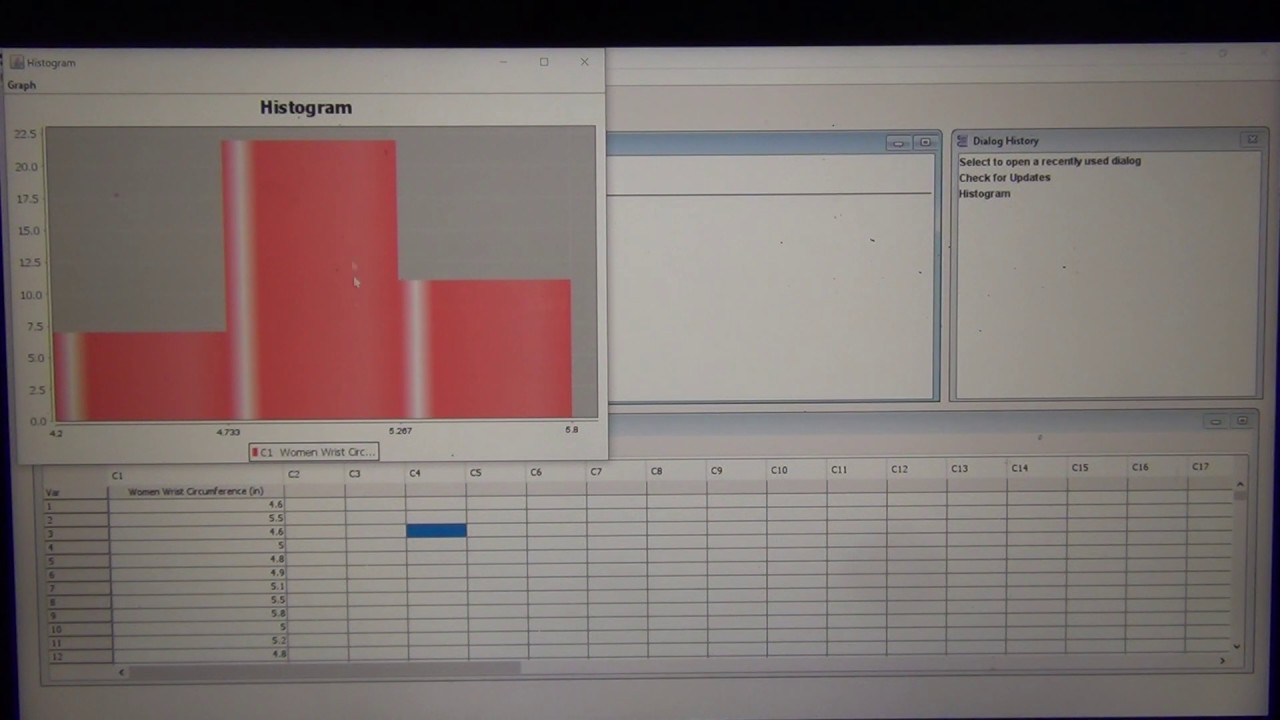
Normal Data Analysis with Software Part 1
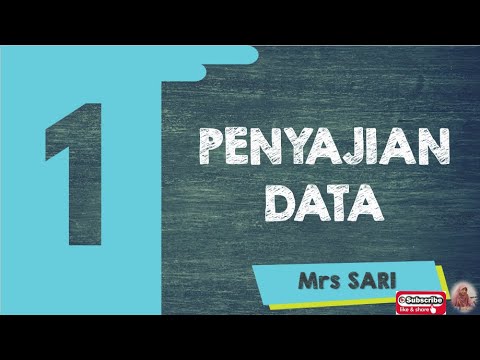
Penyajian Data (Part-1) ~ Tabel dan Diagram (Materi PJJ Kelas VII / 7 SMP)

Ringkasan Materi Statistika kelas 8 Genap
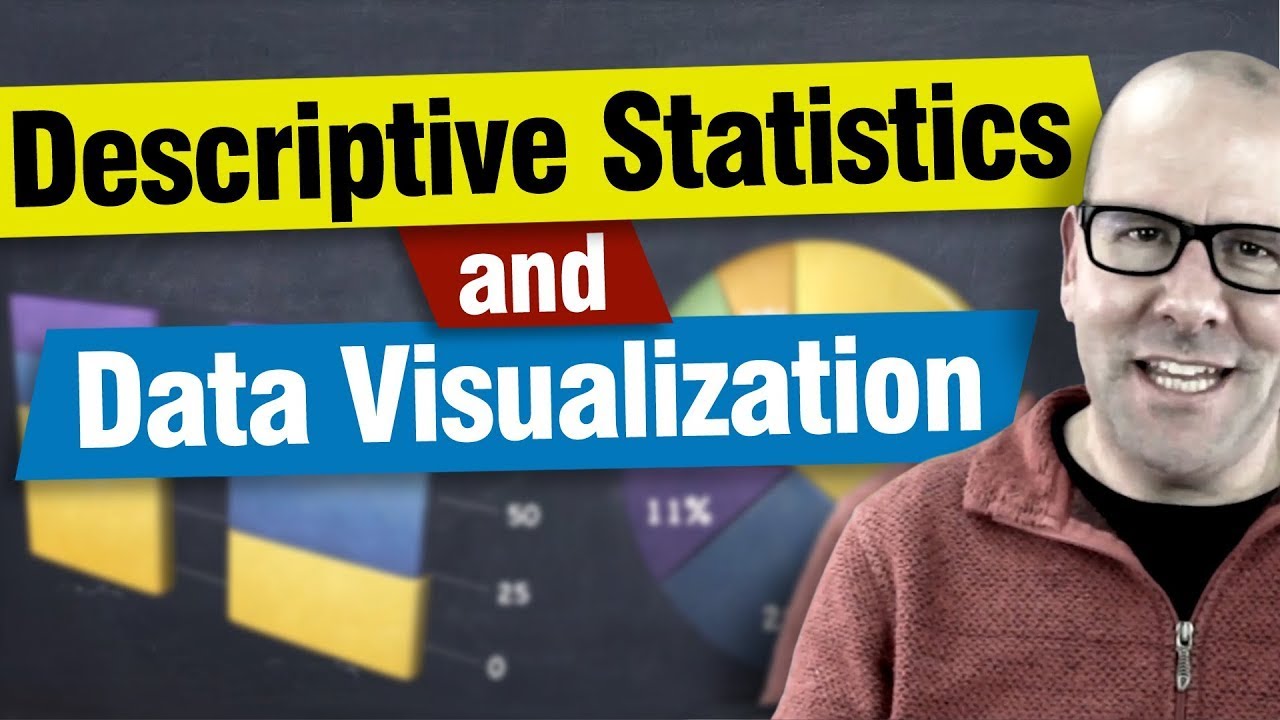
Descriptive statistics and data visualisation. An introduction to statistics and working with data
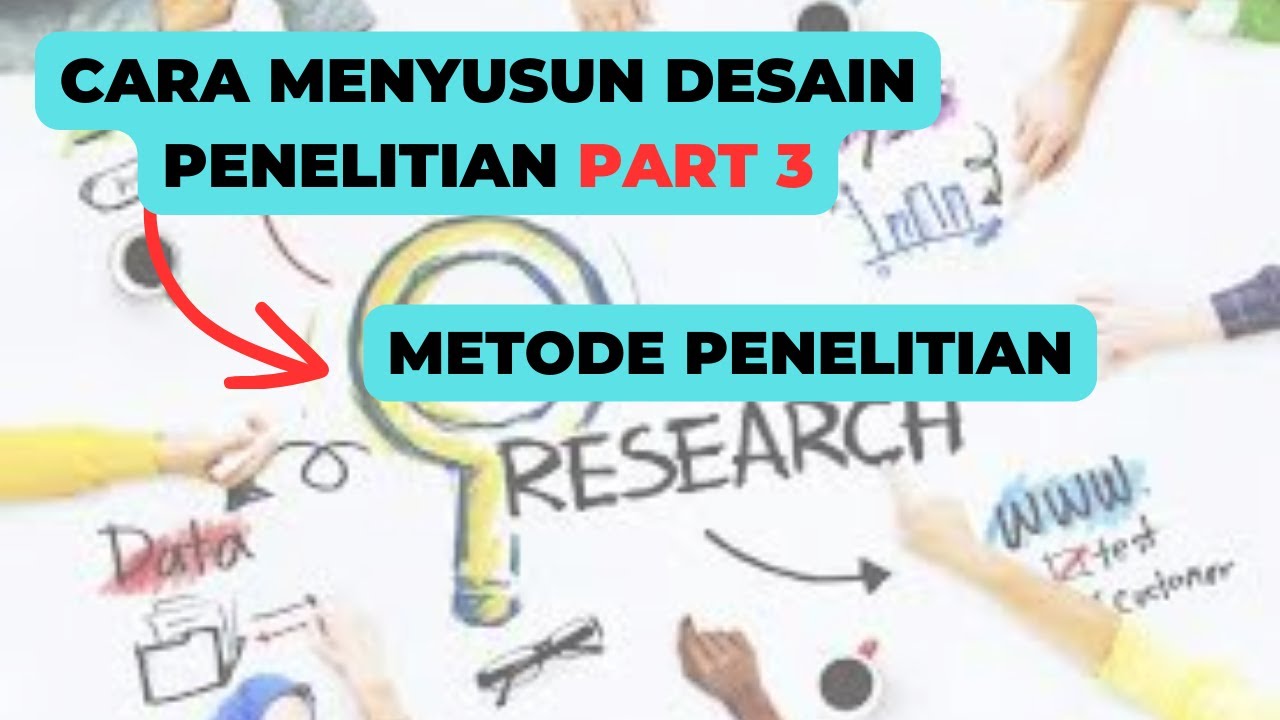
Cara Menyusun Desain Penelitian - Metode Penelitian Survey Part 3

Konsep Dasar Statistik Pendidikan
5.0 / 5 (0 votes)