Mixture-of-Agents Enhances Large Language Model Capabilities
Summary
TLDRこのビデオスクリプトでは、大規模言語モデル(LLM)の力を結集し、より強力なモデルを作ることができる「エージェントの混合(MOA)」フレームワークを紹介しています。MOAは、異なるLLMを層状に配置し、応答を生成して洗練することで、個々のモデルの限界を克服し、応答の品質を向上させます。様々なベンチマークで、MOAはGPT-4などのトップモデルを上回るパフォーマンスを発揮し、オープンソースモデルのみで高い品質とコスト効率を証明しています。
Takeaways
- 🧠 大型语言模型(LLMs)通过大量数据训练,展现出了卓越的自然语言理解和生成能力。
- 📈 尽管LLMs表现出色,但在规模和训练数据方面仍存在限制,扩展模型成本高昂。
- 🤝 研究发现,当不同的LLMs协同工作时,它们的性能会显著提高,即使辅助模型的输出质量低于单个LLM。
- 🔄 我们提出了一种名为“混合代理(MOA)”的方法,通过迭代利用多个LLMs来提高响应质量。
- 🛠️ MOA结构包含多个代理层,每层包含多个LLMs,这些模型可以在层内或跨层重复使用。
- 🔑 通过选择基于性能指标和输出多样性的LLMs,MOA旨在克服个别模型的限制,并通过协作综合提高整体响应质量。
- 🏆 我们的评估使用各种基准测试显示,MOA在诸如Alpaca、Aval 2.0等上取得了显著改进,实现了最先进的胜率。
- 🌟 我们提出了一个新的框架MOA,通过利用多个LLMs来增强推理和语言生成能力。
- 🔍 我们强调了LLMs的协作性,表明它们在共同工作时表现更好,并在竞争性基准测试中通过MOA框架实现了最先进的性能。
- 📊 我们还开发了MOA的不同变体,如使用GPT-4作为聚合器的MOA with GPT-4,以及注重成本效益的MOA light。
- 📈 通过详细的实验和预算分析,我们展示了不同实现的MOA可以达到与GPT-4 Turbo相当的性能,同时更具成本效益。
Q & A
大規模言語モデル(LLM)とは何で、どのように自然言語の理解と生成を革新的に進化させましたか?
-大規模言語モデル(LLM)は、膨大なデータ量に学習され、人間の好みに合わせて調整された高度な言語モデルです。これらのモデルは自然言語の理解と生成において、人間の能力を超える能力を示しており、自然言語の処理をより正確かつ効果的に行うことが可能です。
LLMの限界はどのようなものがありますか?
-LLMの限界は主にサイズとトレーニングデータのスケーリングに関連しています。モデルを拡大するほどコストが高くなり、各モデルには独自の強みと特長があります。
複数のLLMを組み合わせることでどのようにモデルのパフォーマンスが向上するのか説明してください。
-複数のLLMを組み合わせることで、モデル同士が出力から相互に参照することができる「協調性」という現象が生じます。これは個々のモデルが単独で提供できるものよりも低い品質の応答でも、パフォーマンスが向上することがわかりました。
Mixture of Agents(MOA)フレームワークとは何であり、どのように応答の品質を向上させるのでしょうか?
-MOAフレームワークは、複数のLLMを利用して応答の品質を繰り返し向上させる方法論です。このフレームワークは、応答を生成し、洗練むエージェントのレイヤーを含み、最終的には強固で包括的な出力を生成することを目指しています。
MOAフレームワークにおける「proposers」と「aggregators」の役割とは何ですか?
-「proposers」は多様な視点を提供する役割を持ち、「aggregators」はそれらの応答を高品質な出力を形成する役割を果たします。MOAフレームワークでは、これらの役割を通じてモデルの多様性と強みを活用して応答を洗練化します。
MOAフレームワークの評価で、どのような基準に基づいてパフォーマンスが測定されますか?
-MOAフレームワークの評価では、様々な基準に基づいてパフォーマンスが測定されます。これには、人間の好みへのモデルのアライメントや、詳細なパフォーマンスのスコアリングが含まれます。
MOAフレームワークがオープンソースモデルを使用してどのようにパフォーマンスを達成しているのか教えてください。
-MOAフレームワークは、オープンソースモデルを使用して強力なパフォーマンスを達成しています。これは、モデルの選択と多様性に基づいて、さまざまなベンチマークで重要な改善を示しています。
MOAフレームワークの異なる実装では、どのようにコスト効率を達成しているのか説明してください。
-MOAフレームワークの異なる実装では、コスト効率を達成するために、モデルの選択、層の数、および最終層でのアグリゲーターの使用を最適化しています。たとえば、MOA lightはコスト効率を重視し、より少ないレイヤーとアグリゲーターを使用しています。
モデルの多様性とproposersの数が増えることで、最終的な出力をどのように改善するのか説明してください。
-モデルの多様性とproposersの数を増やすことで、より多くの補助情報を得ることができます。これは、最終的な出力を改善し、多様なLLMエージェントを持つMOAレイヤーがパフォーマンスを向上させることを示しています。
MOAフレームワークがどのようにして、計算効率とスケーラビリティを確保しているのか教えてください。
-MOAフレームワークは、プロンプトインターフェースを通じてLLMを操作することで、計算効率とスケーラビリティを確保しています。これにより、内部の活性化や重みを変更することなく、モデルの強みを最大限に活用して一貫性のある出力を生成することができます。
MOAフレームワークが示す「協調性」の効果は、どのような内部メカニズムに基づいているのか?
-MOAフレームワークが示す「協調性」の効果は、アグリゲーターがすべての提案された出力を洗練的に集約する能力に基づいています。これは、単一のLLMの選択よりも高度な集約プロセスが行われていることを示唆しています。
Outlines
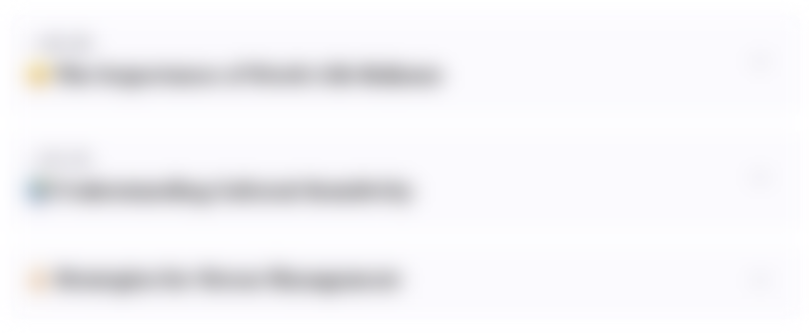
このセクションは有料ユーザー限定です。 アクセスするには、アップグレードをお願いします。
今すぐアップグレードMindmap
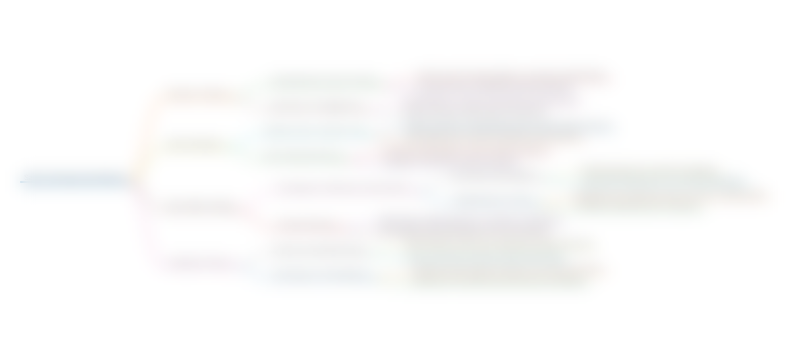
このセクションは有料ユーザー限定です。 アクセスするには、アップグレードをお願いします。
今すぐアップグレードKeywords
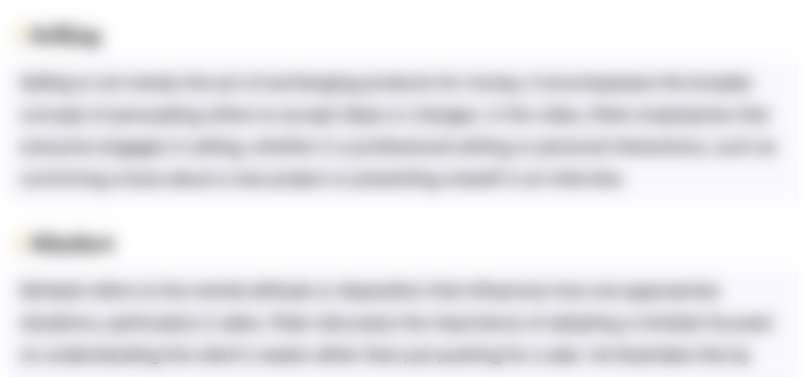
このセクションは有料ユーザー限定です。 アクセスするには、アップグレードをお願いします。
今すぐアップグレードHighlights
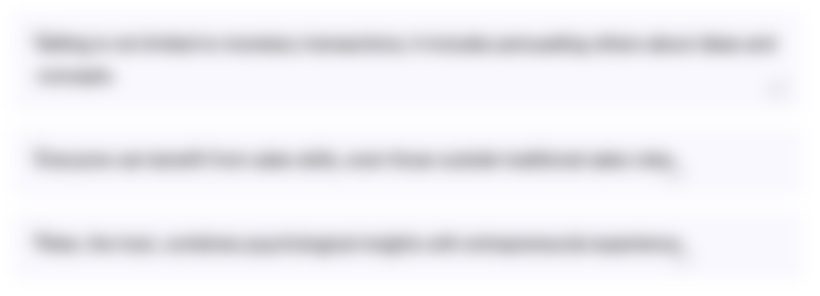
このセクションは有料ユーザー限定です。 アクセスするには、アップグレードをお願いします。
今すぐアップグレードTranscripts
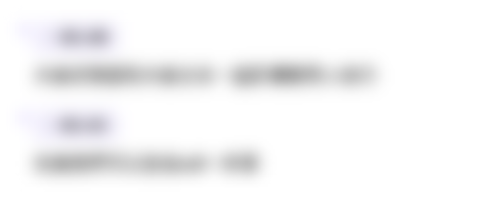
このセクションは有料ユーザー限定です。 アクセスするには、アップグレードをお願いします。
今すぐアップグレード5.0 / 5 (0 votes)