Tsuzumi
Summary
TLDRこのスクリプトでは、NTTが開発した愛言語モデル「スズミ」について紹介されています。スズミは、7億のパラメータを持つ比較的小型ながらも強力なモデルで、英語と日本語の両方に対応しています。また、持続可能な社会のコンセプトを導入し、大規模なモデルに頼ることなく、小規模で効率的なAIの集積知能を活用して社会課題に対処しています。スズミは、高速なテキスト生成能力と日本語の専門知識を持ち合わせており、日本の言語モデルのベンチマークで優れた結果を出しています。さらに、2020年以降、視覚理解機能を強化し、文書を視覚的に理解できるようにしました。将来的には、人間と自然に共存できるAGI(人工一般知能)を目指し、人間のように幅広い多様性と物理的タスクを実行できるソフトウェアロボットを開発し、人類の意志を実現するというビジョンを持っています。
Takeaways
- 📚 Tumiは、NTTによって開発された言語モデルで、7億のパラメータを持つ比較的小型ながらも強力なモデルです。
- 🗣️ Tumiは英語と日本語の両方に対応し、特に日本語の理解と生成において優れています。
- 💡 Tumiは伝統的な日本の楽器からインスパイアされており、小型ながらも大きな影響力を持つと比喩的に述べています。
- 🌐 Tumiは持続可能な社会のためのコンソーシアムの概念を導入し、大規模なモデルに頼ることを避け、小規模で効率的なAIを利用しています。
- ⚙️ Tumiは軽量モデルとして2つのタイプがあり、パラメータ数が小さく、1つのGPUで動作可能です。
- 🔍 Tumiのトークン化プロセスは、日本語に特化しており、効率的で自然な日本語構造を反映しています。
- 📈 TumiはLudaという日本語言語モデルのベンチマークでGPT 3.5を上回る性能を発揮しました。
- 🇯🇵 Tumiは日本語での質問に答えることもできますが、英語での応答も可能です。
- 👀 Tumiは視覚理解コンポーネントを強化し、文書を視覚的に理解することができます。
- 📈 TumiはAIの未来について意見を述べ、絵文字を使って表現することができ、多様なデータタイプを効果的に処理できます。
- 🌟 Tumiの将来のビジョンは、人間と自然に共存し、様々な環境で協働できるAGI(人工的な一般知能)の開発です。
Q & A
スズミはどのような言語モデルをベースにしていますか?
-スズミは、NTTが開発した愛言語モデルをベースにしています。
スズミのパラメータ数はいくつですか?
-スズミのパラメータ数は70億です。他のモデルに比べて比較的小さく、1つのGPUで動作可能です。
スズミは日本語と英語のどちらの言語に対応していますか?
-スズミは日本語と英語の両方の言語に対応しています。
スズミが持つ特徴として、持続可能な社会のためのコンセプトとは何ですか?
-スズミは、大規模なモンティックモデルに頼ることなく、小規模で効率的なAIの集団知能を活用して社会課題を解決するコンセプトを導入しています。
スズミのトークン化プロセスはどのようにして日本語の効率性を向上させていますか?
-スズミのトークン化プロセスは、日本語の文法知識を活用して冗長なトークンを最小限に抑えることで、自然な日本語構造を反映しています。
スズミは日本語の言語モデルのベンチマークでどのようにパフォーマンスしていますか?
-スズミは、他の日本語モデルやGPT 3.5と比較して、30%以上の勝率増加を示しており、1兆トークンでの事前トレーニングと高品質な指示チューニングコーパスで調整されています。
スズミは英語での質問にどのように応答していますか?
-スズミは英語での質問にも日本語と同じように応答することができます。具体的な応答例がスクリプトに示されています。
スズミのマルチモダリティの能力とは何ですか?
-スズミは、2020年頃から視覚理解コンポーネントを強化し、文書を視覚的に理解することができます。画像から情報を抽出したり、視覚コンテンツに基づいて質問に答える機能があります。
スズミの将来のビジョンは何ですか?
-スズミの将来のビジョンは、人間に自然に共存できるAGI(人工的な一般知能)の創造であり、人間と協力してコンピュータ上で働くソフトウェアロボットの開発を目指しています。
スズミが持つ視覚ドキュメント理解モデルの構成要素は何ですか?
-スズミの視覚ドキュメント理解モデルは、画像エンコーダー、言語モデル、そして視覚ドキュメント理解のためのアダプターから構成されます。
スズミがデジタル変換トランスフォーメーションにどのように役立つか説明してください。
-スズミは、画像から情報を抽出し、それをJson形式に変換することで、デジタル変換トランスフォーメーションに役立ちます。これにより、文書のデジタル化が促進されます。
スズミの開発チームは今後どのようなモダリティを強化する予定ですか?
-スズミの開発チームは、今後も聴覚や触覚など、様々なモダリティを強化し続ける予定です。これにより、より包括的な人間の能力にマッチする入力出力インターフェースを開発することが可能です。
Outlines
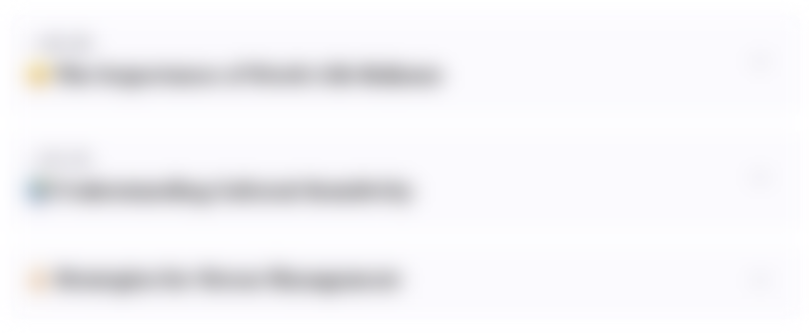
This section is available to paid users only. Please upgrade to access this part.
Upgrade NowMindmap
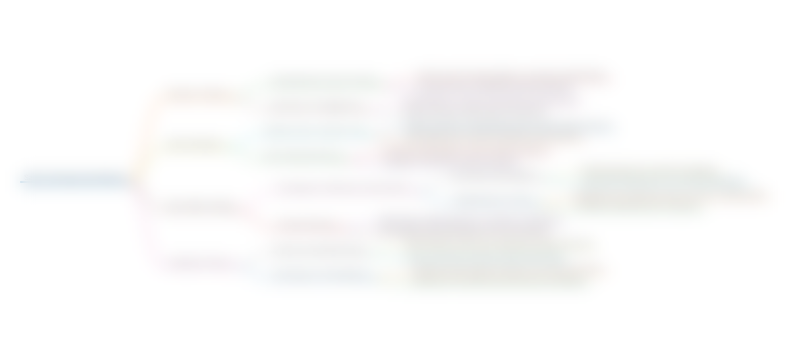
This section is available to paid users only. Please upgrade to access this part.
Upgrade NowKeywords
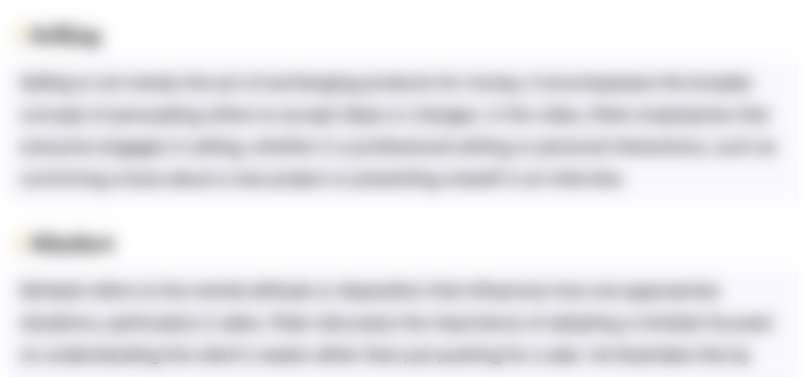
This section is available to paid users only. Please upgrade to access this part.
Upgrade NowHighlights
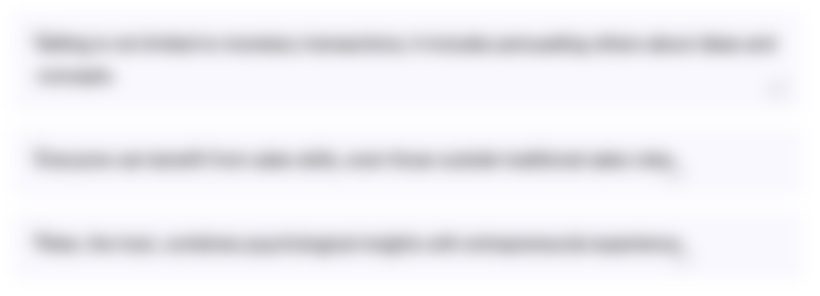
This section is available to paid users only. Please upgrade to access this part.
Upgrade NowTranscripts
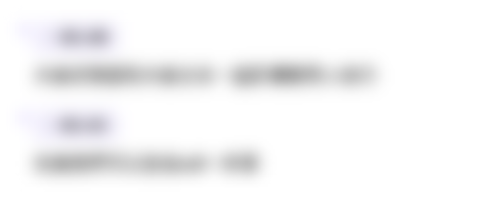
This section is available to paid users only. Please upgrade to access this part.
Upgrade Now5.0 / 5 (0 votes)