Zero-shot Prompting Explained
Summary
TLDRفي هذا الفيديو، يناقش المتحدث مفهوم التوجيه دون أمثلة مسبقة (Zero Shot Prompting) عند استخدام نماذج اللغة الكبيرة مثل GPT-4. يوضح كيفية عمل هذه النماذج في تحليل المشاعر وتصنيف النصوص دون الحاجة إلى تقديم أمثلة سابقة. يشرح أيضًا أهمية هذا النوع من التوجيه في التطبيقات العملية، ويشير إلى تقنيات أخرى مثل التوجيه مع أمثلة مسبقة (Few Shot Prompting) والتي سيتم مناقشتها في فيديوهات قادمة. الفيديو موجه للمطورين والباحثين المهتمين بفهم كيفية تحسين أداء النماذج في مهام محددة.
Takeaways
- 🤖 اللغة المستخدمة للنماذج اللغوية الكبيرة مثل GPT-4 يمكن تنفيذ المهام بدون تدريب مسبق باستخدام تقنيات مثل التحفيز الصفري.
- 📊 التحفيز الصفري يعني إعطاء النموذج تعليمات دون تقديم أمثلة على المهمة، ومع ذلك يمكن للنموذج فهم المهمة وأدائها بناءً على تدريبه المسبق.
- 📝 في حالة التصنيف العاطفي، يمكن للنموذج تحديد ما إذا كانت النصوص تحمل مشاعر إيجابية أو سلبية أو محايدة بدون الحاجة إلى أمثلة.
- 🎯 يمكن تعديل التحفيز لجعل النموذج يفهم المهمة بشكل أفضل عن طريق إضافة مؤشرات خاصة بالمخرجات.
- 🧠 النموذج يفهم المهام لأنه تم تدريبه على بيانات واسعة النطاق تتضمن أمثلة على هذه المهام.
- 🔧 التحفيز الصفري قد لا يكون كافيًا في جميع الحالات، وخاصة في التطبيقات الواقعية التي تتطلب تحسين النتائج باستخدام أمثلة إضافية.
- 🔍 التحفيز المدعوم بأمثلة، المعروف باسم التحفيز في السياق، قد يكون ضروريًا لتحسين أداء النموذج في بعض التطبيقات.
- 📚 التحفيز التعليمي هو عملية تدريب النموذج على الاستجابة لتعليمات معينة بناءً على المدخلات.
- 🎥 القناة ستنشر المزيد من الفيديوهات التي تستعرض تقنيات التحفيز المختلفة وكيفية مقارنة نتائجها مع طرق مثل التعديل الدقيق.
- 💬 دعوة للمشاهدين للمشاركة في التعليقات واقتراح مواضيع أخرى للفيديوهات المستقبلية التي تتناول تقنيات التحفيز.
Q & A
ما هو المقصود بـ "zero shot prompting"؟
-"Zero shot prompting" هو أسلوب يتم فيه تقديم مهمة معينة للنموذج دون إعطائه أمثلة مسبقة على كيفية أداء هذه المهمة. يتم الاعتماد على المعرفة التي تم تدريب النموذج عليها مسبقًا لأداء المهمة مباشرة.
كيف يمكن للنموذج أن يعرف كيفية تصنيف النصوص في مهام مثل تحليل المشاعر؟
-النموذج يتم تدريبه على بيانات ضخمة تحتوي على أمثلة مختلفة لمهام مثل تحليل المشاعر. هذا يعني أنه يمكن للنموذج فهم كيفية تصنيف النصوص إلى فئات مثل إيجابي، سلبي، أو محايد دون الحاجة إلى أمثلة إضافية.
لماذا لا يتطلب النموذج أحيانًا إعادة تدريب أو ضبط (fine-tuning) لأداء مهام محددة؟
-النموذج غالبًا ما يحتوي على المعرفة الكافية من البيانات التي تم تدريبه عليها، مما يسمح له بأداء بعض المهام بشكل جيد دون الحاجة إلى إعادة تدريب أو ضبط.
ما هي أهمية استخدام مؤشرات الخروج (output indicators) في التحفيز الصفري (zero shot prompting)؟
-مؤشرات الخروج تساعد النموذج على فهم نوع المهمة المطلوب منه أداؤها بناءً على طريقة تصميم الجملة التحفيزية (prompt).
ما هي الخطوات التي يمكن اتباعها للتحقق من أداء النموذج في مهام تصنيف النصوص؟
-يمكن للمطورين أو الباحثين استخدام مجموعات بيانات كبيرة لتقييم دقة النموذج في تصنيف النصوص، مع تجربة نماذج تحفيزية مختلفة لتوجيه النموذج نحو النتائج المطلوبة.
ما هي العلاقة بين التحفيز الصفري (zero shot prompting) والتحفيز القليل (few-shot prompting)؟
-التحفيز الصفري لا يتطلب تقديم أي أمثلة مسبقة للنموذج، بينما التحفيز القليل يتطلب تقديم عدد قليل من الأمثلة لتوجيه النموذج بشكل أفضل نحو النتيجة المرغوبة.
ما هو الدور الذي تلعبه "instruction tuning" في تحسين أداء النماذج؟
-"Instruction tuning" هي عملية يتم فيها تدريب النموذج على مجموعة من التعليمات المرتبطة بالمخرجات المتوقعة، مما يساعد النموذج على تقديم استجابات دقيقة عندما يواجه مدخلات مشابهة.
كيف يمكن للنموذج أداء مهام مثل تلخيص النصوص واستخراج المعلومات في سياق التحفيز الصفري؟
-النموذج يعتمد على المعرفة المكتسبة من التدريب على بيانات ضخمة ليتمكن من تلخيص النصوص أو استخراج المعلومات دون الحاجة إلى أمثلة أو تعليمات إضافية.
ما هي التحديات التي قد تواجه استخدام التحفيز الصفري في التطبيقات الواقعية؟
-في بعض الحالات الواقعية، قد لا يكون أداء النموذج في التحفيز الصفري كافياً للحصول على النتائج المطلوبة، مما يتطلب تقديم أمثلة أو إعادة تدريب النموذج للحصول على دقة أفضل.
كيف يمكن للباحثين والمطورين تحسين أداء النموذج في التحفيز الصفري؟
-يمكن تحسين الأداء من خلال استخدام تقنيات مثل التحفيز القليل (few-shot prompting) أو إعادة تدريب النموذج على مجموعات بيانات مخصصة لتوجيه النموذج بشكل أفضل نحو المهمة المحددة.
Outlines
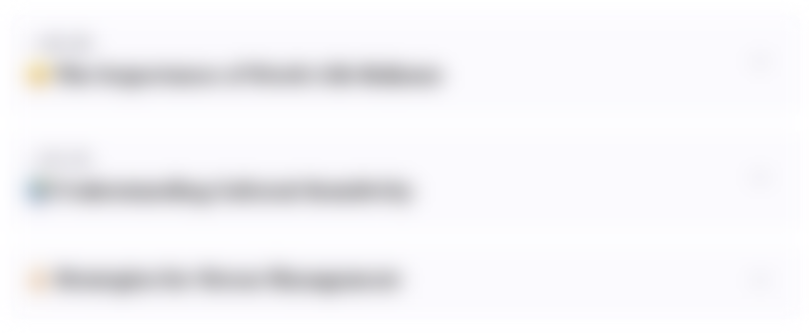
This section is available to paid users only. Please upgrade to access this part.
Upgrade NowMindmap
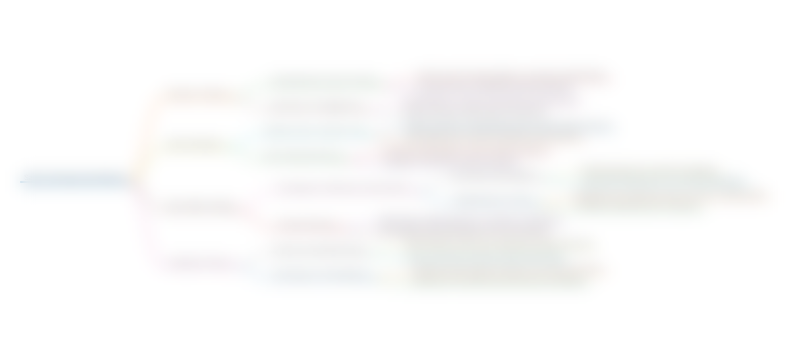
This section is available to paid users only. Please upgrade to access this part.
Upgrade NowKeywords
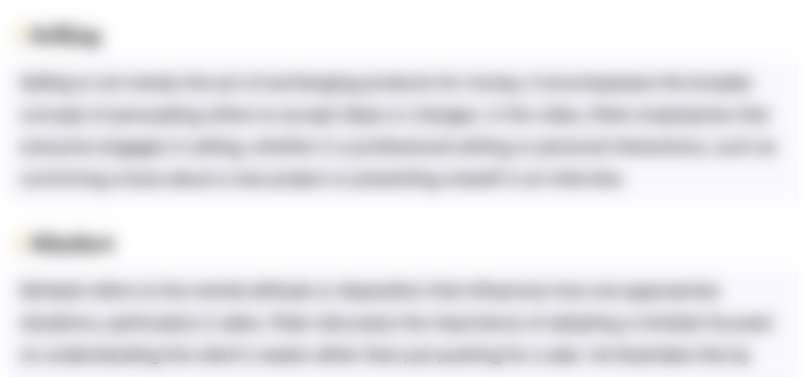
This section is available to paid users only. Please upgrade to access this part.
Upgrade NowHighlights
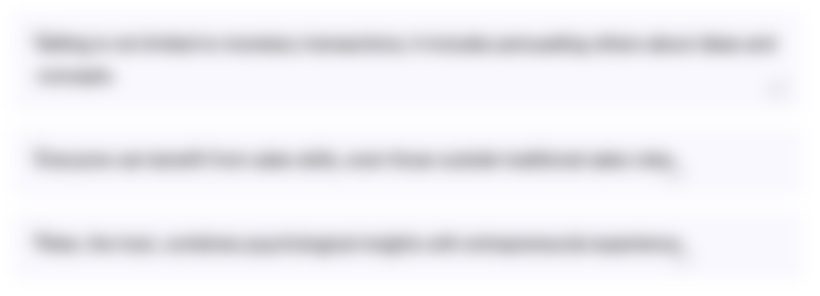
This section is available to paid users only. Please upgrade to access this part.
Upgrade NowTranscripts
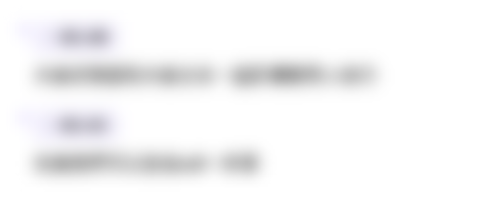
This section is available to paid users only. Please upgrade to access this part.
Upgrade Now5.0 / 5 (0 votes)