Learn to deploy AI models on edge devices like smartphones
Summary
TLDRこのレッスンでは、オンデバイスAIが人気のある理由とその利点を学びます。低遅延、効率の向上、コスト削減、プライバシー保護などがあります。リアルタイム音声検出、意味分割、物体検出、身体活動検出など、現実世界でのオンデバイスAIの様々な応用についても学びます。さらに、モバイル、PC、産業IoT、自動車業界など、オンデバイスAIが適用される産業も紹介されています。モデルをデバイス上で実行する4つの主な理由と、クラウドでトレーニングしたモデルを約5分でデバイスで動作するように展開する新しい方法も説明されています。AIubを使用して自動化されたモデルのキャプチャ、コンパイル、検証、パフォーマンス測定のプロセスも紹介されています。
Takeaways
- 🤖 オンデバイスAIは、遅延を減らし、効率を高め、コストを削減し、プライバシーを保護する多くの利点をもたらします。
- 📱 スマートホンでの写真撮影には20以上のAIモデルが、数百ミリ秒以内に完璧なショットをキャプチャします。
- 🚗 産業IoTセグメントでは、オンデバイスAIの経済的な影響は約3兆ドルと評価されています。
- 🔍 高度な運転支援システムを持つ車は、オンデバイスAIに基づいて動作します。
- 🗣️ スマートスピーカーとの会話は、デバイス上で動作するテキスト読み上げ機能によって可能になっています。
- 🤖 配送や組み立てを行うロボット、産業や農業の用途で地形をスキャンするドローンなど、オンデバイスAIは様々な場面で使われています。
- 🎨 スマートホンやラップトップでの写真編集は、オンデバイスAIによってパワーされています。
- 🔄 オンデバイスAIは、音声、画像、ビデオ、音声、およびセンサーを組み合わせてマルチモダリティAIモデルを作成することができます。
- 📈 モバイルスマートホン、PC、産業IoT、自動車業界など、オンデバイスAIが適用される産業は多岐にわたります。
- 💡 オンデバイスでモデルを実行する4つの主な理由は、コスト効果、効率、プライバシー保護、そしてパーソナライズされた体験の提供です。
- 🛠️ QualcommのAIUBを使用すると、モデルをデバイスに展開するプロセスを自動化し、わずか5分でクラウドでトレーニングしたモデルをデバイスで動作させることができます。
- 🎨 ライブ翻訳、ライブトランスクリプション、写真生成、AIベースの写真編集、セマンティックサーチ、テキスト要約、各種の仮想アシスタント、ライティングアシスタント、および画像生成など、オンデバイスAIは創造的なAIアプリケーションにも非常に人気があります。
Q & A
オンデバイスAIが人気のある理由は何ですか?
-オンデバイスAIは遅延が少なく、効率が向上し、コストが削減され、プライバシーが保護されるなどの利点があります。
オンデバイスAIが持つ主な利点は何ですか?
-オンデバイスAIの主な利点は、遅延の低減、効率の向上、コストの削減、プライバシーの保護です。
オンデバイスAIがどのようなリアルタイムアプリケーションを提供できますか?
-オンデバイスAIはリアルタイム音声検出、リアルタイム意味分割、リアルタイム物体検出、および身体活動検出などのアプリケーションを提供できます。
スマートスピーカーとの会話がどのようにオンデバイスAIと関連しているか説明してください。
-スマートスピーカーとの会話は、デバイス上で実行されるテキスト読み上げ機能によってオンデバイスAIによってパワーされています。
オンデバイスAIが産業界でどのような経済的影響を与えると予想されていますか?
-産業IoTセグメントでは、オンデバイスAIの経済的影響は約3兆ドルと予想されています。
デバイス上でモデルを実行する4つの主な理由は何ですか?
-デバイス上でモデルを実行する4つの主な理由は、コスト効果、効率、プライバシー、そしてパーソナライズされた体験の提供です。
モデルをデバイスに展開する新しい方法とは何ですか?
-モデルをデバイスに展開する新しい方法は、クラウドでトレーニングしたモデルを約5分でデバイスで動作するようにすることです。
デバイスにモデルを展開するプロセスにはどのようなステップが含まれますか?
-デバイスにモデルを展開するプロセスには、モデルを計算グラフとしてキャプチャし、ターゲットデバイス用にコンパイルし、デバイス上でモデルの数値を検証し、デバイス上でパフォーマンスを測定するという4つのステップが含まれます。
QualcommのAIUBとは何ですか?
-QualcommのAIUBは、デバイスへのモデルの展開プロセスを自動化するツールであり、キャプチャ、コンパイル、検証、パフォーマンス測定の4つのステップを簡素化します。
オンデバイスAIが適用される最も人気のある産業はどれですか?
-オンデバイスAIが適用される最も人気のある産業は、モバイルスマートホン産業、PC産業、産業IoT産業、および自動車産業です。
オンデバイスAIが提供するジェネラティブAIアプリケーションには何が含まれますか?
-オンデバイスAIが提供するジェネラティブAIアプリケーションには、ライブ翻訳、ライブトランスクリプション、写真生成、AIベースの写真編集、意味検索、テキスト要約、各種の仮想アシスタンス、ライティングアシスタンス、および画像生成が含まれます。
デバイス上で展開可能なモデルの例をいくつか挙げてください。
-デバイス上で展開可能なモデルの例には、言語モデルのLlama、音声モデルのWhisper、顔検出モデルのMediaPipe、安定化拡散などがあります。
Outlines
🤖 デバイス上でAIを実行する理由と利点
この段落では、デバイス上でAIを実行する人気とその理由について学びます。デバイス上でAIを実行することには、レイテンシの低減、効率の向上、コストの削減、プライバシーの保護という様々な利点があります。リアルタイム音声検出、セマンティックセグメンテーション、オブジェクト検出、身体活動検出など、実際の世界でのデバイス上でAIの様々な応用についても学びます。さらに、デバイス上でAIがどのように機能し、産業IoTセグメントでの経済的影響、スマートスピーカーやロボット、ドローンなどの応用例についても紹介されています。
📱 デバイス上でAIを実行する主要な理由と手順
デバイス上でモデルを実行する4つの主な理由について説明されています。それはコスト効率、効率性、プライバシー保護、そしてパーソナライズされた体験の提供です。また、デバイス上でモデルを展開する新しい方法についても紹介されており、クラウドでトレーニングしたモデルをデバイス上で動作させるプロセスは5分以内で完了できます。このプロセスには、モデルのキャプチャ、コンパイル、数値の検証、そしてデバイスでのパフォーマンスの測定という4つのステップが含まれています。QualcommのAIUBを使用してこれらのステップを自動化し、デバイス上でAIを展開するプロセスを非常にスムーズにしています。
🛠️ デバイス上で展開可能なAIモデルの種類と応用
デバイス上で展開可能なAIモデルの種類とその応用について説明されています。スマートフォンやラップトップ上で実際に展開されているAIの応用例が挙げられています。言語モデル、音声モデル、顔検出モデル、テキスト読み上げ、音声認識、QRコード検出、バーチャルバックグラウンドセグメンテーションなど、デバイス上で簡単に展開できる100以上のモデルが存在することを紹介しています。
Mindmap
Keywords
💡オンデバイスAI
💡低遅延
💡効率性
💡コスト削減
💡プライバシー保護
💡パーソナライズ
💡リアルタイム処理
💡マルチモダリティ
💡生成的AI
💡 Qualcomm AI Ub
Highlights
On-device AI is popular due to benefits like reduced latency, improved efficiency, reduced cost, and privacy preservation.
Applications of on-device AI include real-time speech detection, semantic segmentation, object detection, and physical activity detection.
Over 20 AI models run every time a picture is taken with a smartphone to capture the perfect photo.
The estimated economic impact of on-device AI in the industrial IoT segment is about 3 trillion.
Advanced Driver Assistance systems in cars are entirely based on on-device AI.
On-device AI is used in various scenarios like typing on a keyboard, interacting with smart speakers, and editing pictures.
Multimodal on-device AI models can combine audio, image, video, speech, and sensors for enhanced capabilities.
Key industries for on-device AI include mobile, PC, industrial IoT, and automotive.
Running models on-device is cost-effective as it utilizes local computational resources without cloud computing.
Local data processing in on-device AI improves computational efficiency.
Data privacy is enhanced with on-device AI as data never leaves the device.
On-device AI allows for personalized experiences through locally customized models.
A new method for deploying models on-device simplifies the process to under 5 minutes.
The deployment process involves capturing the model, compiling it, validating numerics, and measuring performance.
Qualcomm's AIUB automates the deployment process for on-device AI.
On-device AI is popular for generative AI applications like live translation, transcription, and photo editing.
A collection of various models like Lama, Ban, Whisper, Media Pipe, and Stable Diffusion are deployable on-device today.
Transcripts
in this lesson you will learn about why
on device AI is so popular you will
learn about various benefits such as
reduced latency improved efficiency
reduced cost and privacy
preservation you will also learn about
various applications of on device AI in
the real world today including realtime
speech detection real-time semantic
segmentation realtime object detection
as well as physical activity detection
let's Dive Right
In let's start with a few fun facts
about on device AI did you know that
every time you take a picture with your
smartphone over 20 AI models run to
capture the perfect picture within a few
hundred milliseconds in the industrial
iot segment the estimated economic
impact of on device AI is about3
trillion and every time you drive a car
that's equipped with Advanced Driver
assistance
it's entirely based on on device Ai and
on device AI is everywhere when you type
on a keyboard on a laptop it's powered
by a language model that runs on device
when you talk to a Smart speaker the
text to speech is entirely powered on
device robots that deliver and assemble
have a lot of on device AI drones that
can scan landscapes for industrial and
agricultural use cases use on device AI
every time you edit a picture on a
smartphone or a laptop that's powered by
on device Ai and every time you drive a
car that's also powered by on device
Ai and here are a few of the applicable
use cases with audio with image as well
as sensors uh the popular audio and
speech applications include text to
speech speech recognition machine
translation audio noise removal when you
work with images and videos you have
photo classification QR code detection
virtual background segmentation and when
you work with sensors your physical
activity detection keyboard models
digital handwriting recognition are all
powered by on device Ai and what's
fascinating about this is you can even
mix multiple modalities with audio with
image with video and speech and sensors
and produce multimodel on device AI
models and the most popular Industries
where on device AI is applicable include
clude the Mobile smartphone industry
your PC industry uh your industrial iot
industry as well as the automotive
industry now let's look at why you would
want to run models on device there are
four main reasons the first one is that
it's cost effective because you can
utilize all the computational resources
that are available locally without any
additional cloud computing resources the
second one is efficiency because you can
process process data locally and not
need to send it to the cloud receive the
results and then process it again
locally this whole process becomes
computationally much more efficient the
third is about privacy because your data
will remain on your device and never
leave it and that ties into our fourth
reason which is personalization because
having models being customized locally
on your device without any external data
can create uniquely personalized
experience
in this lesson we're going to walk
through a new way to deploy models on
device that makes it extremely easy to
take a model that you've trained in the
cloud and make it work on the device
within about 5 minutes and there are
four main steps as part of this process
in the first one you will capture the
model as a computational graph then you
will take that computational graph and
compile it for your Target device you
will then validate the numerics of that
model on the device that you're trying
to deploy to and then finally you will
measure performance on the device now
all of this requires an actual device in
the loop that's why you will be using a
device in the loop to go through each of
these four steps and finally when these
four steps are done you will have an
artifact that you can deploy on a device
and integrate it in your application to
make this extremely seamless you will be
using qualcomm's aiub that automates all
of these for four steps the capture the
compilation the validation and the
performance measurement along with the
device that's provided to you so you can
go through this process in about 5
minutes now on device AI is also
extremely popular for generative AI
applications this includes things like
live translation live transcription
photo generation AI based photo editing
semantic search text
summarization various virtual assistance
writing assistance as well as image
Generation all of these are commercially
deployed applications of on device AI on
your smartphone or
laptop and these are a collection of all
the various models that are all
Deployable today on a smartphone a
laptop an iot device or a car this
includes language models like uh Lama
and ban speech models like whisper face
detection models like like media pipe uh
stable diffusion and over 100 plus
models that are all very easy to deploy
on device today
Browse More Related Video
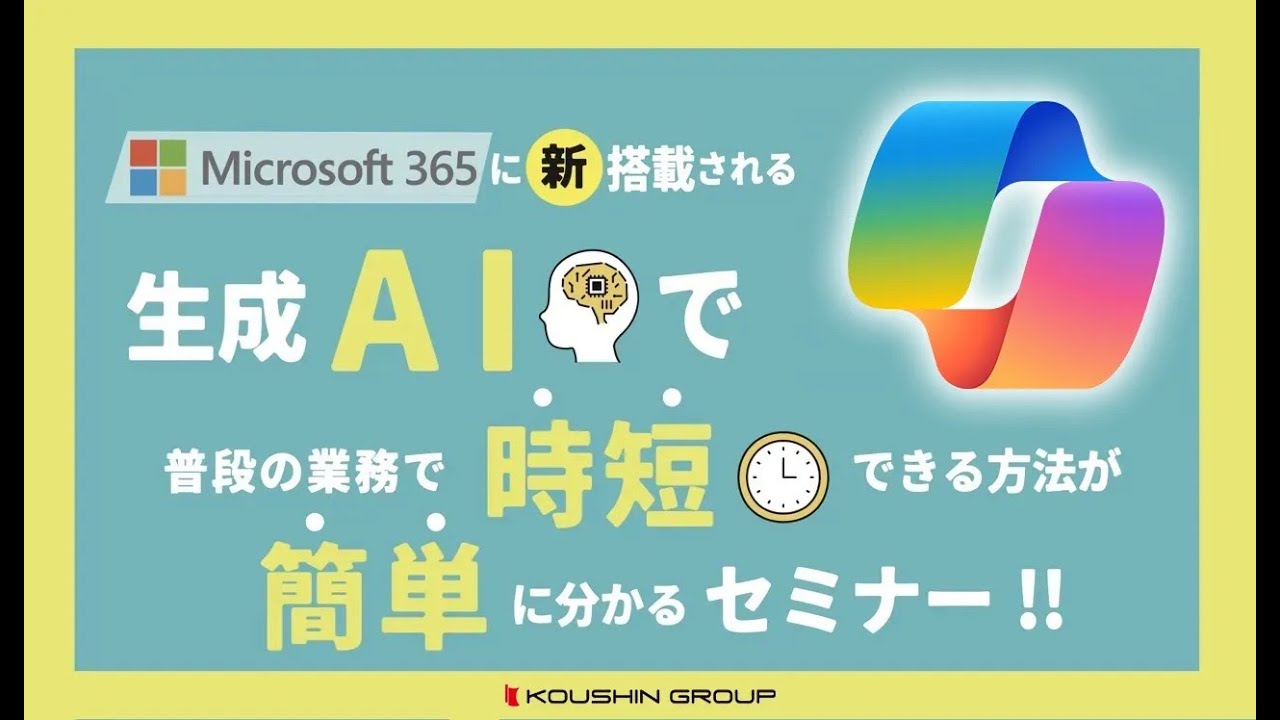
Copilot for Microsoft365 のAIが業務を簡単に時短できる方法 ウェビナー
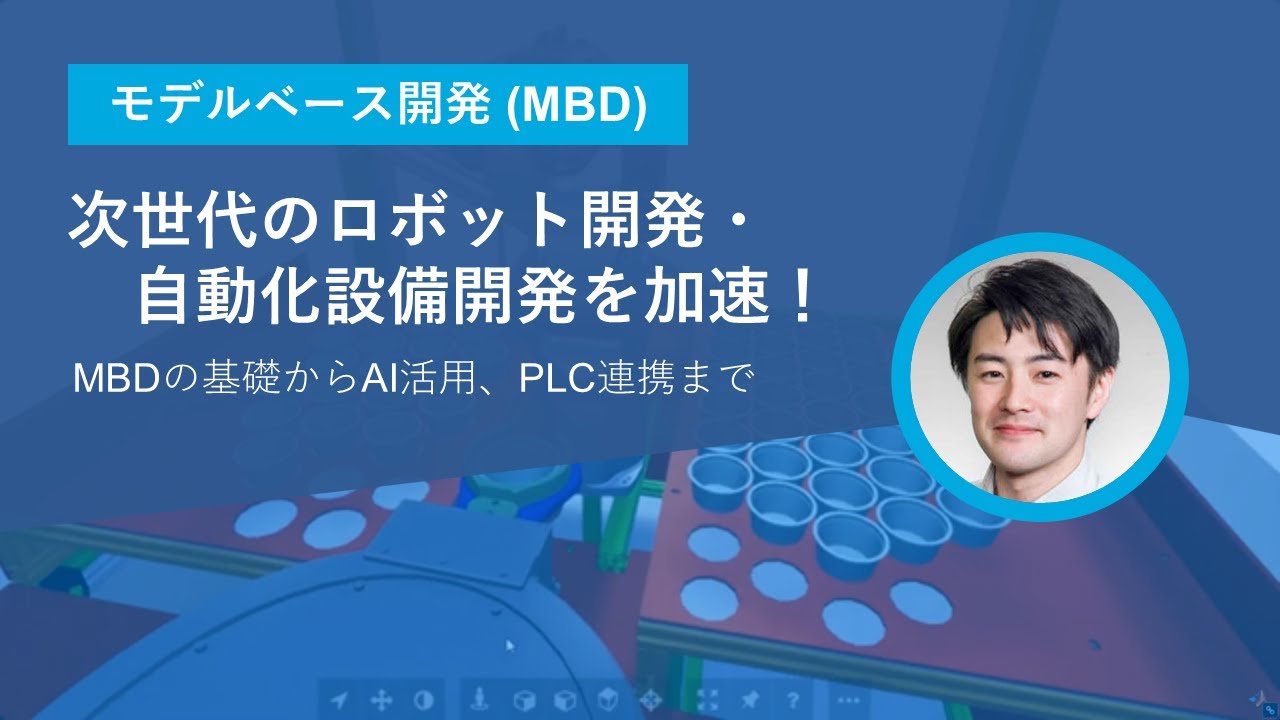
モデルベースデザインで次世代のロボット・自動化設備開発を加速! ~モデルベースの基礎からAI活用、PLC連携まで~
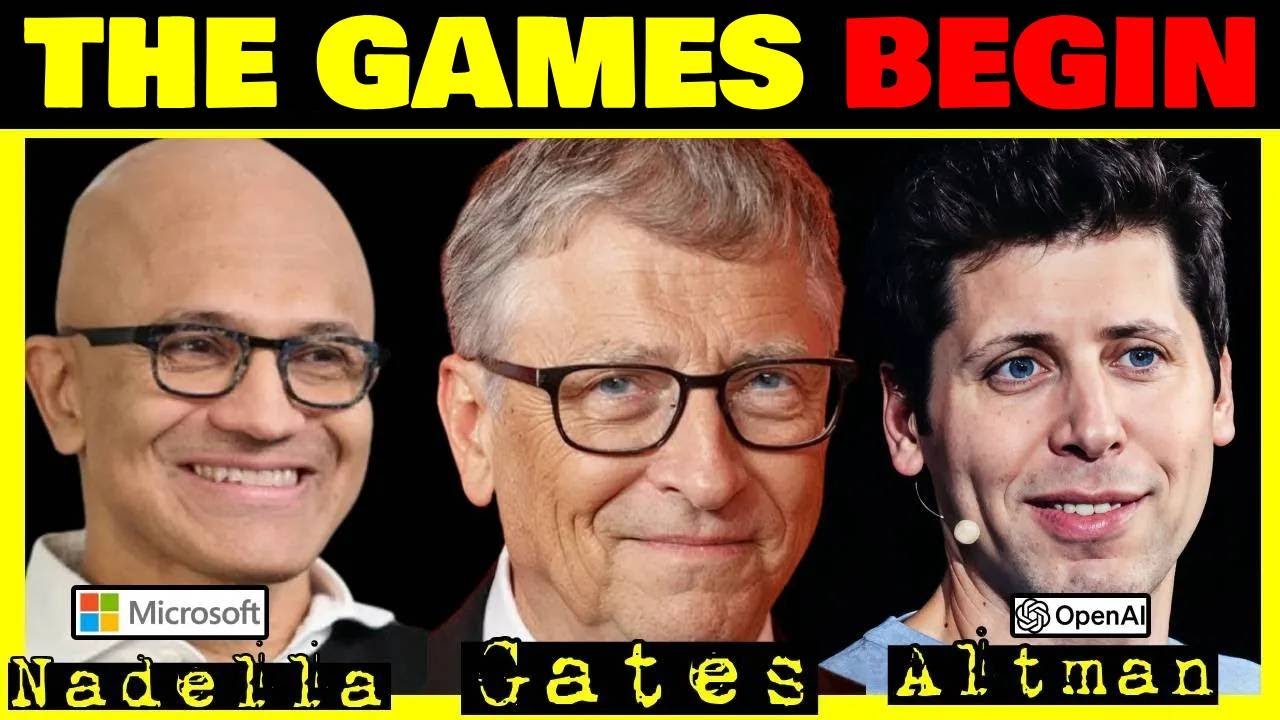
Microsoft's new "Embodied AI" SHOCKS the Entire Industry! | Microsoft's Robots, Gaussian Splat & EMO

【就活】仕事の将来性を語る:AIエンジニアやデータサイエンティスト【転職】
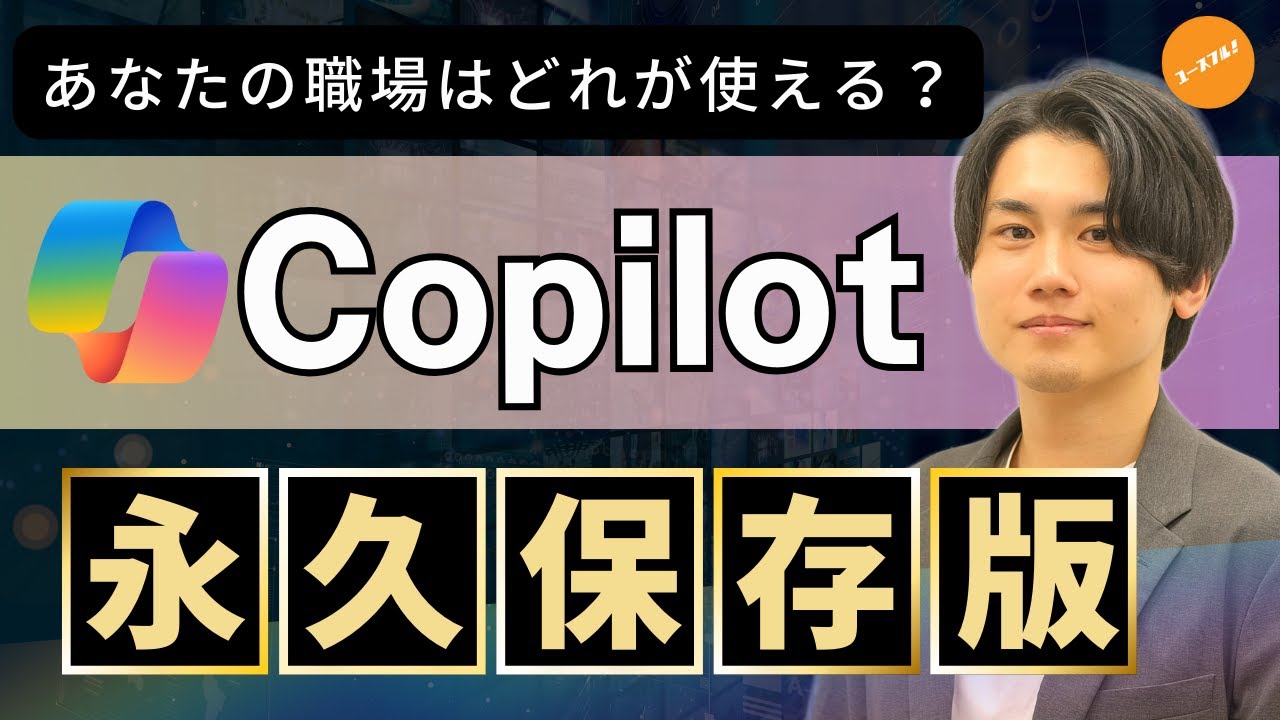
【Copilot】2024年の最新トレンドを先取り!Microsoft Copilot全ラインアップを徹底解剖【無料で使える生成AI】
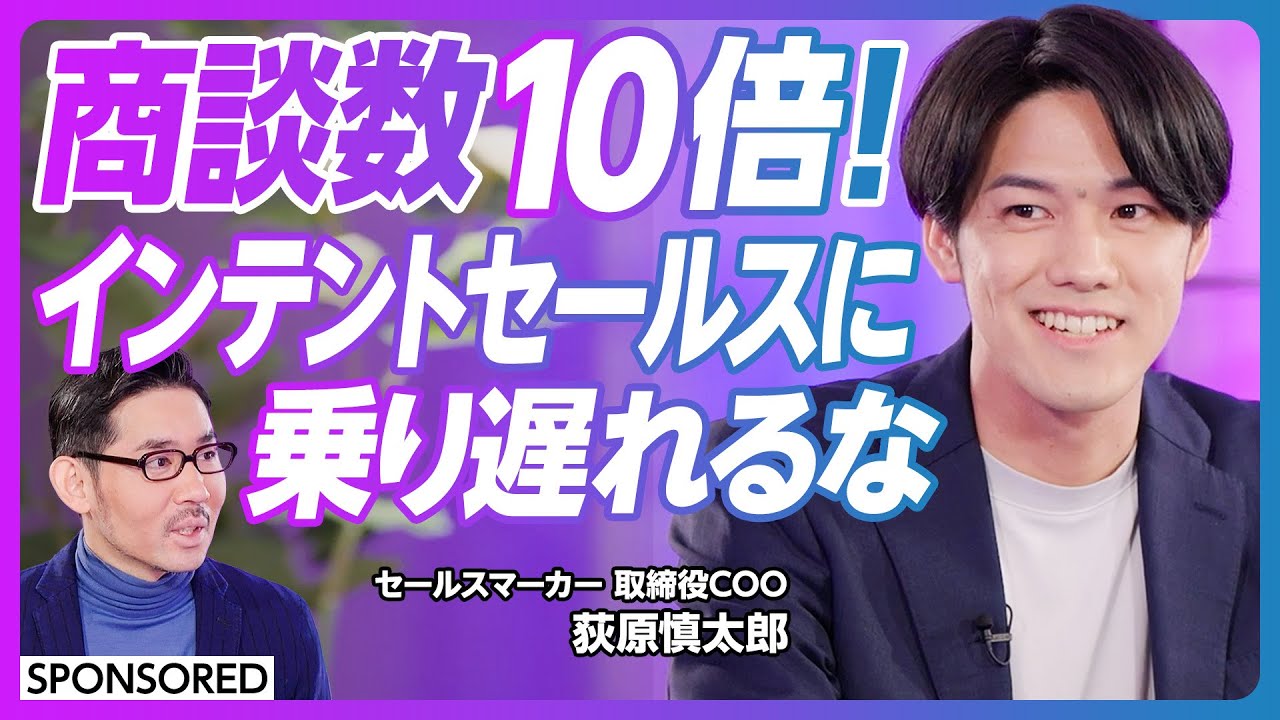
【インテントセールスに乗り遅れるな】導入成果は商談数10倍、アポイント取得率3倍/現場に愛されるSaaS/顧客のインテントを起点としたインテントホイール/ニーズが高い企業にAIが自動でアプローチ
5.0 / 5 (0 votes)