Factor analysis | Statistics in Psychology
Summary
TLDRThis video covers the basics of factor analysis, a method used to identify underlying variables from observed data. It explores its history, from Spearman's g factor to Thurstone's multi-factor theory and Cattell's contributions. The video explains key assumptions such as sample size and linearity, and introduces essential terminologies like factor loadings, communality, and eigenvalues. Different methods of factor extraction, including Principal Component Analysis (PCA) and Maximum Likelihood, are discussed. The video also covers factor rotation techniques, such as Varimax and Promax, helping viewers understand how to simplify and interpret complex data sets.
Takeaways
- 😀 Factor analysis is a technique used to identify unobservable variables (factors) that explain observed variables, helping to reduce the complexity of large datasets.
- 😀 Charles Spearman introduced the concept of common factor analysis and proposed the 'g' factor, which influences cognitive performance.
- 😀 Lewis Thurstone expanded on Spearman's work, developing the multiple factor theory and introducing concepts like communality, uniqueness, and rotation in factor analysis.
- 😀 Raymond Cattell applied factor analysis to psychometrics, particularly in intelligence research, and developed methods for measuring similarity coefficients.
- 😀 Factor analysis is commonly used in psychology to study intelligence, personality, attitudes, and beliefs, simplifying complex data into manageable factors.
- 😀 Key assumptions of factor analysis include no outliers in the dataset, adequate sample size, and no perfect multi-collinearity between variables.
- 😀 Factor analysis assumes linearity in the relationships between variables, but non-linear data can be transformed to fit this assumption.
- 😀 The data used in factor analysis must be interval data, as nominal or ordinal data are not suitable for this method.
- 😀 Factor loading values indicate the strength of the relationship between variables and factors, helping to interpret how each variable contributes to a factor.
- 😀 Common methods of factor extraction include the Centroid Method, Principal Component Analysis (PCA), and Maximum Likelihood Method.
- 😀 Factor rotation, including orthogonal (independent factors) and oblique (correlated factors) rotations, is used to simplify factor interpretation and increase the clarity of the results.
Please replace the link and try again.
Outlines
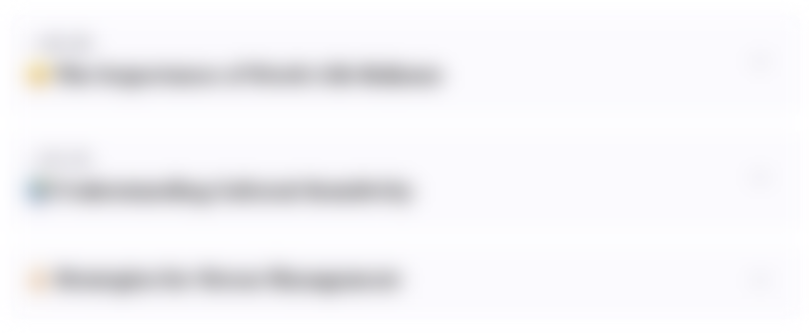
This section is available to paid users only. Please upgrade to access this part.
Upgrade NowMindmap
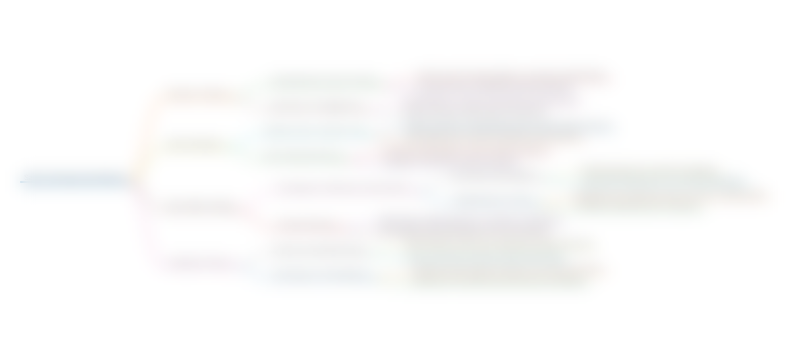
This section is available to paid users only. Please upgrade to access this part.
Upgrade NowKeywords
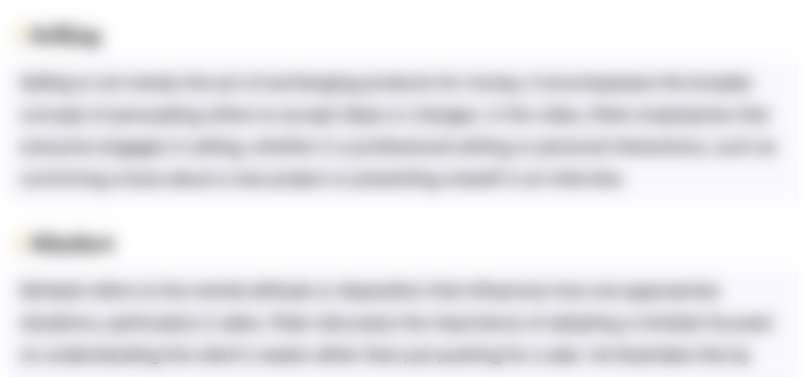
This section is available to paid users only. Please upgrade to access this part.
Upgrade NowHighlights
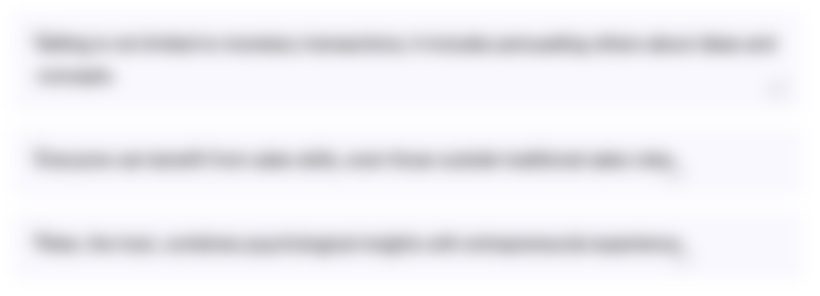
This section is available to paid users only. Please upgrade to access this part.
Upgrade NowTranscripts
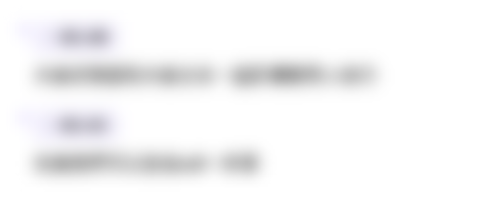
This section is available to paid users only. Please upgrade to access this part.
Upgrade NowBrowse More Related Video
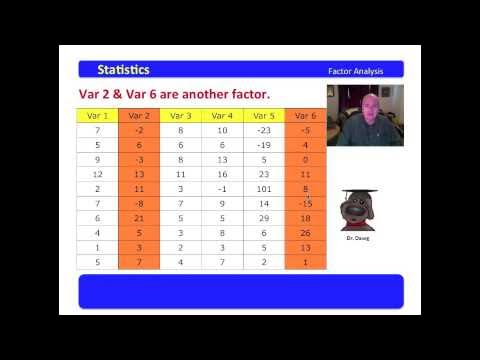
1 Factor Analysis - An Introduction

SPSS Factor Analysis for Beginners 1 - Basic Ideas
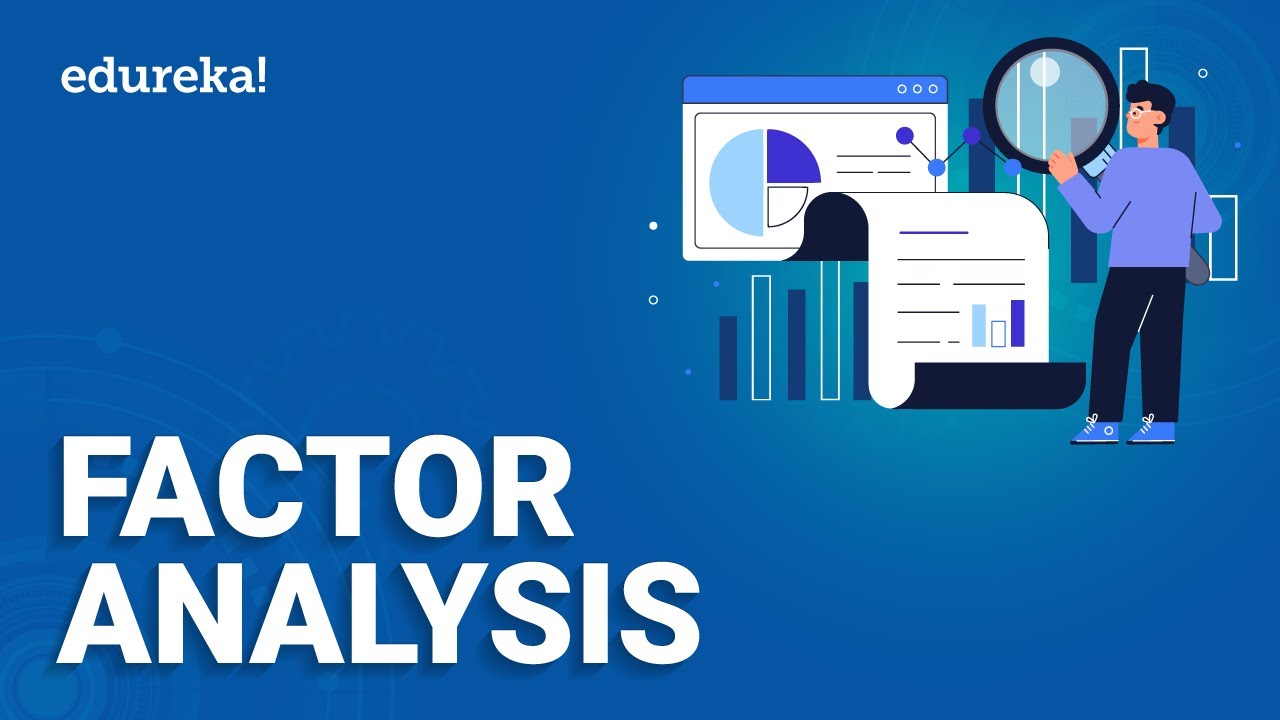
Factor Analysis | What is Factor Analysis? | Factor Analysis Explained | Machine Learning | Edureka
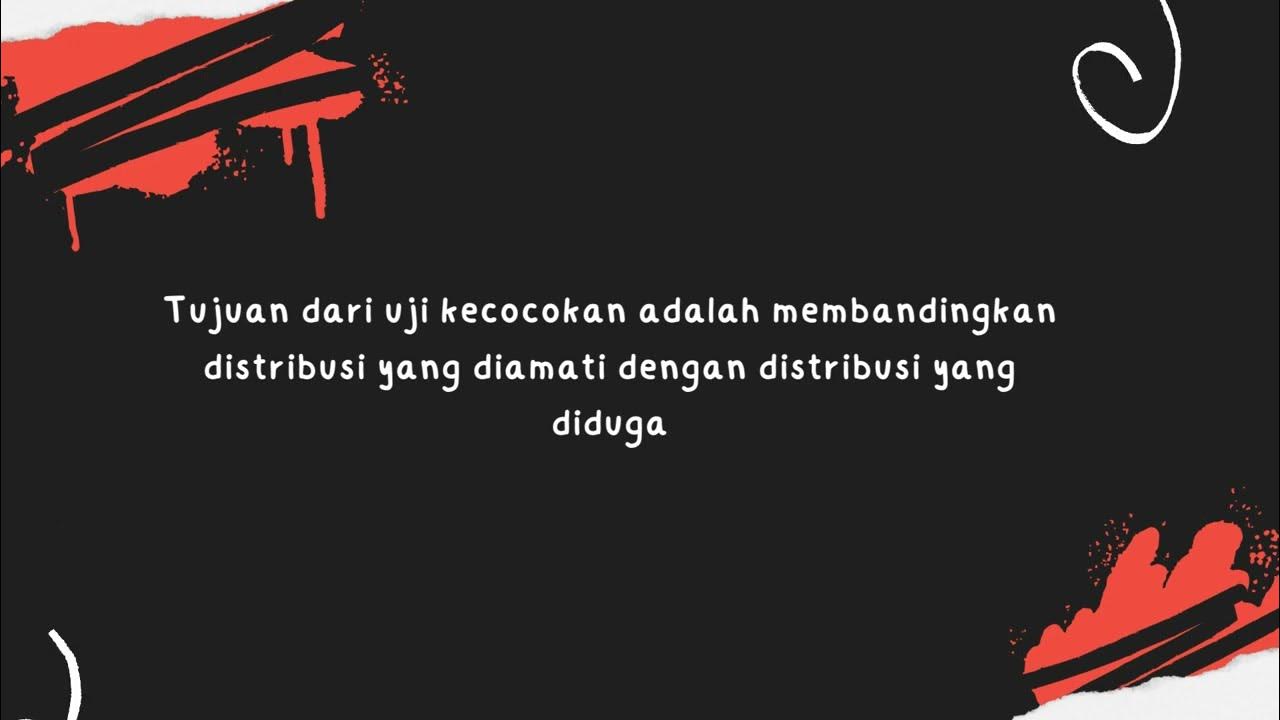
*M* Uji Kecocokan: Frekuensi yang Diduga Sama dan yang Tidak Sama dengan Microsoft Excel dan SPSS
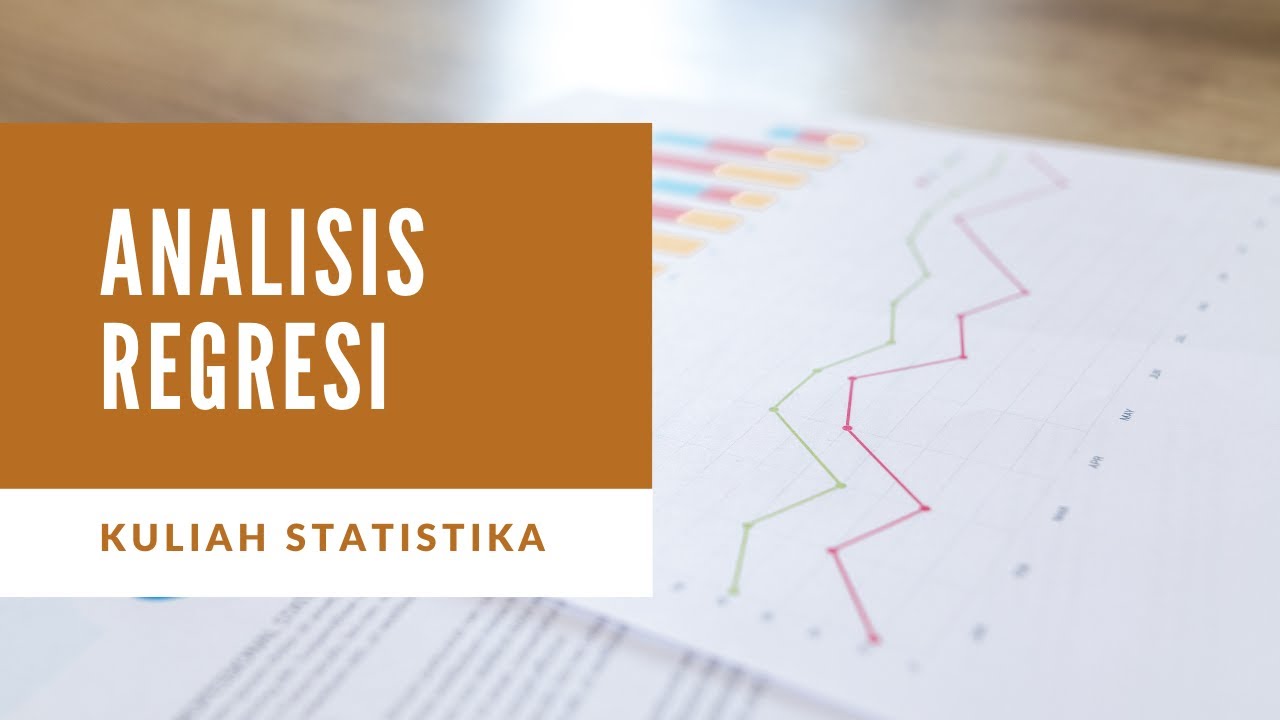
KULIAH STATISTIK - ANALISIS REGRESI
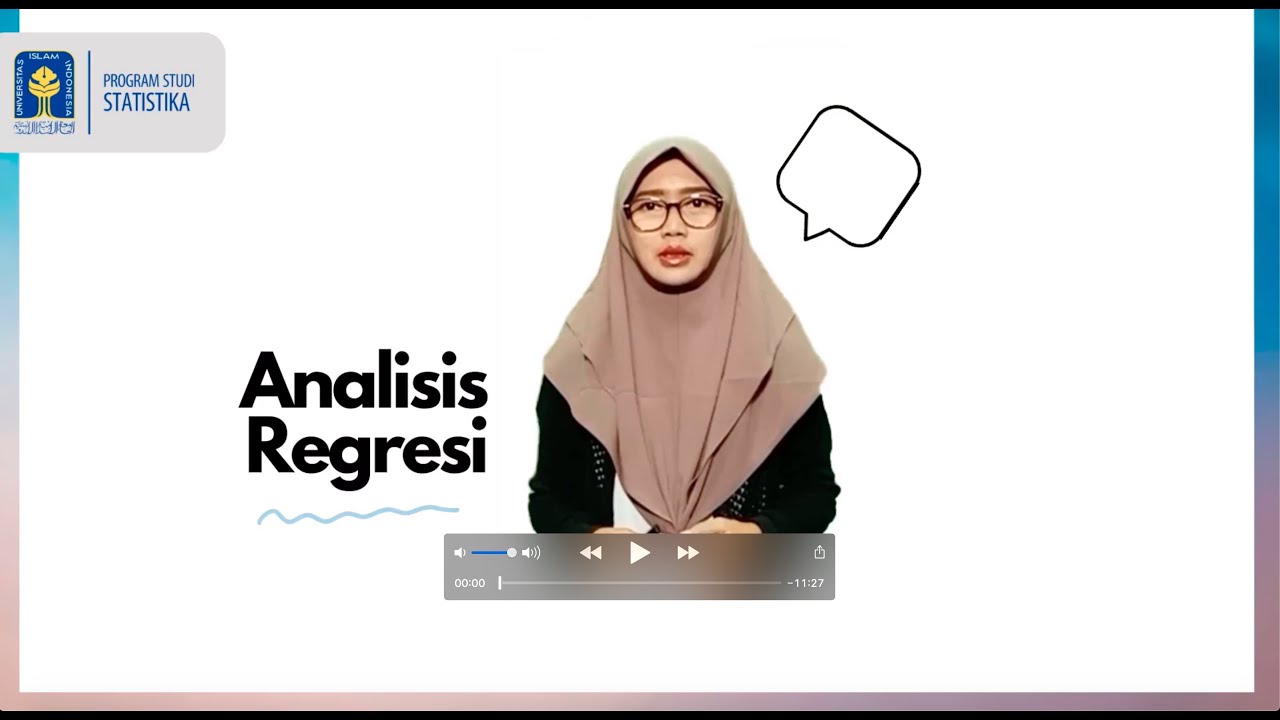
Regresi Linear Sederhana dengan Ordinary Least Square
5.0 / 5 (0 votes)