SPSS Factor Analysis for Beginners 1 - Basic Ideas
Summary
TLDRIn this SPSS tutorial, Ruben introduces the concept of factor analysis through a case study on client satisfaction with the Dutch unemployment agency. The agency uses 16 survey questions to assess their performance, with responses scored on a 7-point scale. Ruben explains the limitations of calculating mean scores and introduces factor analysis as a method to identify underlying factors from the survey data. He discusses exploratory factor analysis (EFA) in SPSS, which aims to determine the number of factors, which items measure them, and what real-world characteristics they represent. The tutorial also covers the use of eigenvalues to assess factor quality and the interpretation of factor loadings.
Takeaways
- ๐ **Factor Analysis Basics**: The video introduces the basic concept of factor analysis, which is a statistical method used to describe variability among observed variables in terms of fewer unobserved variables called factors.
- ๐ณ๐ฑ **Case Study Context**: The case study revolves around a Dutch unemployment agency aiming to assess client satisfaction through a survey, highlighting the practical application of factor analysis.
- ๐ **Survey Design**: The agency formulated 16 survey questions to measure client satisfaction, each scored on a 7-point Likert scale, demonstrating a common research methodology.
- ๐ค **Philosophical Questioning**: The video raises questions about whether client satisfaction is made up of separate aspects and if some statements might measure the same underlying sentiments.
- ๐ **Factor Analysis Diagram**: A diagram is used to illustrate how nine out of 16 statements might measure only three underlying factors, simplifying the data into more manageable components.
- ๐ **Exploratory vs Confirmatory Factor Analysis**: The tutorial differentiates between exploratory factor analysis (EFA) and confirmatory factor analysis (CFA), with EFA being more about discovering the factor structure within the data.
- ๐ข **Correlation Analysis**: The video explains how correlations among variables can indicate the presence of underlying factors, with high correlations suggesting shared factors.
- ๐ข **Conditional Formatting**: A practical tip is given on using conditional formatting in Excel to visualize correlations, making it easier to identify patterns.
- ๐งฎ **Eigenvalues**: The concept of eigenvalues is introduced as a method to determine the number of factors to retain, with factors having eigenvalues over one considered significant.
- ๐ **Factor Loadings**: Factor loadings are mentioned as a way to interpret how strongly each item is related to a factor, which is crucial for understanding what each factor represents.
- ๐ **Dimension Reduction**: Factor analysis is positioned as a method of dimension reduction, simplifying data sets from multiple variables to a few underlying factors for easier analysis and reporting.
Q & A
What is the main purpose of the unemployment agency in the Netherlands mentioned in the script?
-The main purpose of the unemployment agency in the Netherlands is twofold: first, to provide unemployed people with monthly income known as 'doll', and second, to assist unemployed individuals in finding employment.
What is the significance of the 16 survey questions formulated by the unemployment agency?
-The 16 survey questions are significant as they were designed to measure client satisfaction, which helps the unemployment agency assess their performance in assisting and providing income to unemployed people.
What is the likert scale mentioned in the script, and how is it used?
-The likert scale is a 7-point scale used to measure responses to statements, with each point representing a level of agreement or disagreement. In this case, it is used to score the 16 statements regarding client satisfaction.
Why might computing the mean scores for each statement not be the best method to evaluate performance according to the script?
-Computing the mean scores might not be the best method because it doesn't account for the different aspects of client satisfaction that each statement might represent, nor does it consider the possibility that some statements might measure the same underlying sentiment.
What is the basic idea behind factor analysis as introduced in the script?
-The basic idea behind factor analysis is to identify the underlying factors or dimensions that explain the correlations among a set of observed variables. In the context of the script, it is used to simplify the 16 satisfaction statements into a smaller number of factors.
What is the difference between exploratory factor analysis (EFA) and confirmatory factor analysis (CFA)?
-Exploratory Factor Analysis (EFA) is used to identify the underlying structure of a set of variables, whereas Confirmatory Factor Analysis (CFA) is used to test whether observed data fit a proposed factor model.
What are the three basic questions EFA tries to answer according to the script?
-The three basic questions EFA tries to answer are: 1) How many underlying factors are measured by the variables? 2) Which items measure which factors? 3) What real-world characteristics do the factors represent?
What is a Pieron correlation and how is it used in factor analysis?
-A Pieron correlation is a numerical value between -1 and +1 that indicates the extent to which two quantitative variables are linearly related. In factor analysis, it is used to identify patterns of correlations among variables, which can suggest the underlying factors.
What is the significance of the eigenvalues in factor analysis?
-Eigenvalues in factor analysis are used to determine the number of factors to retain. It is common practice to retain factors with eigenvalues greater than one, as they are considered to represent significant variance in the data.
How can factor loadings help in interpreting factors in factor analysis?
-Factor loadings indicate the correlation between each item and the factors. They help in interpreting factors by showing which items have a stronger relationship with each factor, thus providing insight into what each factor represents.
What is the practical application of factor analysis mentioned in the script?
-The practical application of factor analysis mentioned in the script is dimension reduction, where the original variables (statements) are reduced to a smaller number of factors, which can then be used for reporting or further analysis.
Outlines
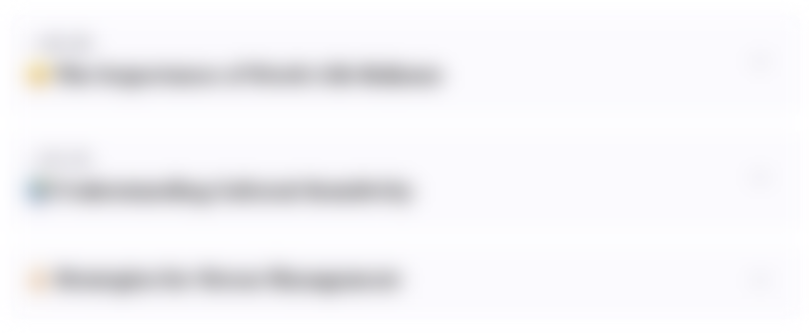
This section is available to paid users only. Please upgrade to access this part.
Upgrade NowMindmap
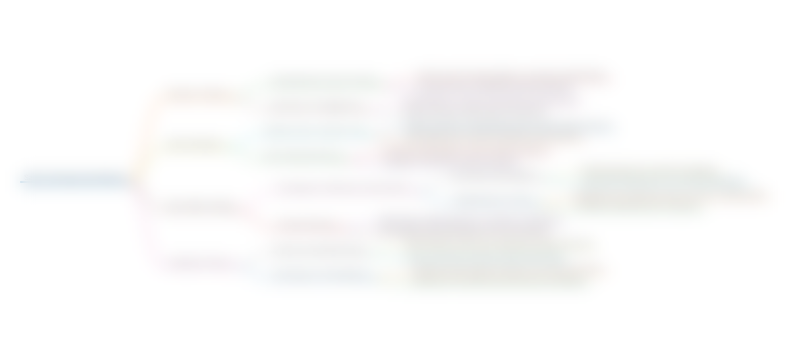
This section is available to paid users only. Please upgrade to access this part.
Upgrade NowKeywords
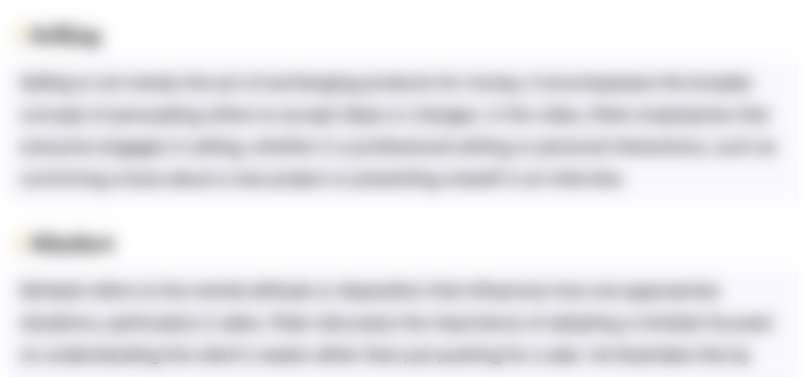
This section is available to paid users only. Please upgrade to access this part.
Upgrade NowHighlights
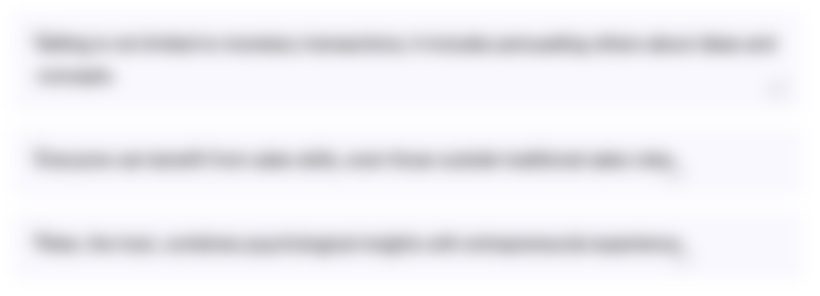
This section is available to paid users only. Please upgrade to access this part.
Upgrade NowTranscripts
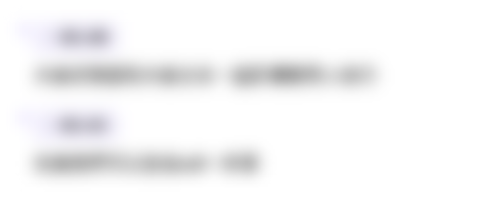
This section is available to paid users only. Please upgrade to access this part.
Upgrade NowBrowse More Related Video
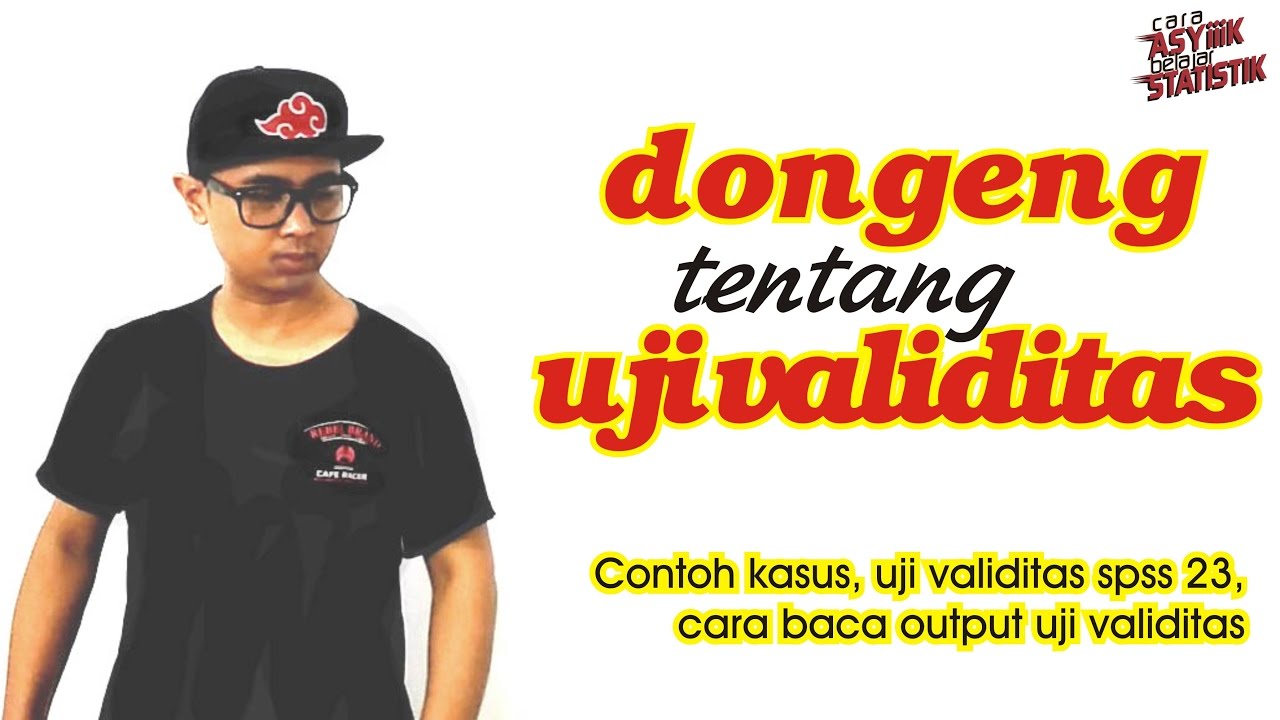
Dongeng tentang uji validitas Cara uji validitas spss 23, cara baca output
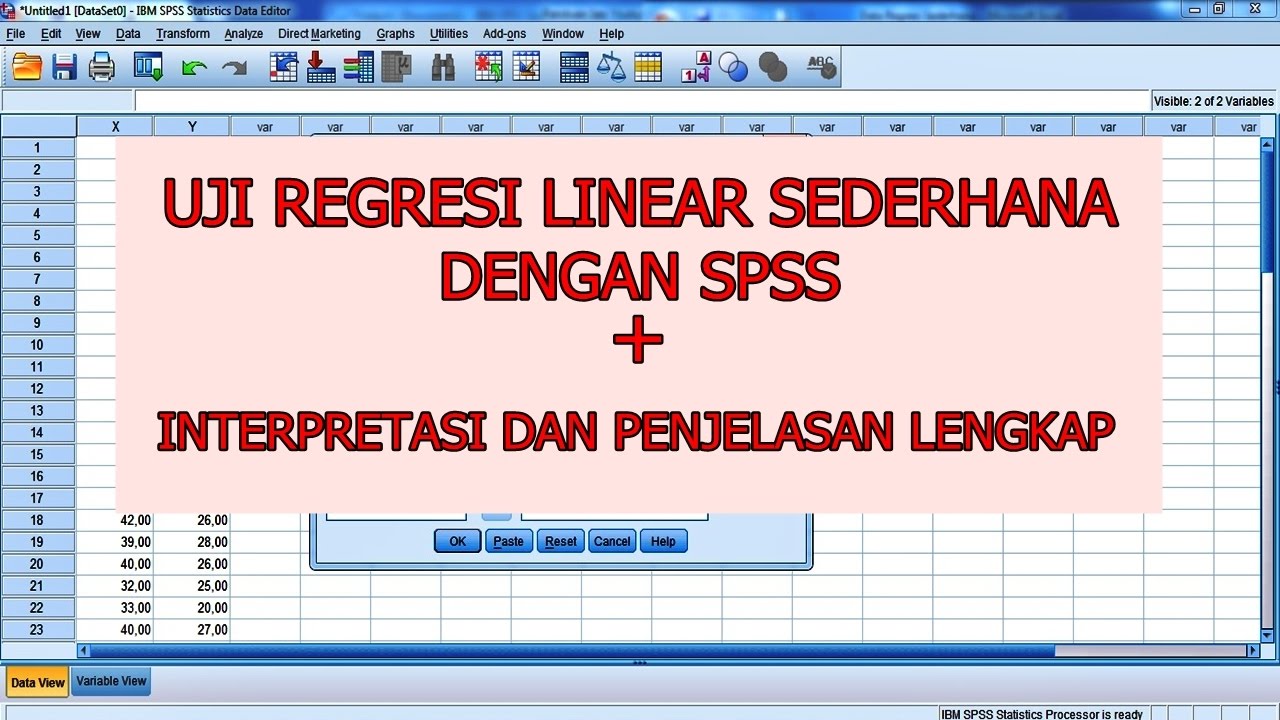
Uji Regresi Linear Sederhana dengan SPSS Sangat Detail
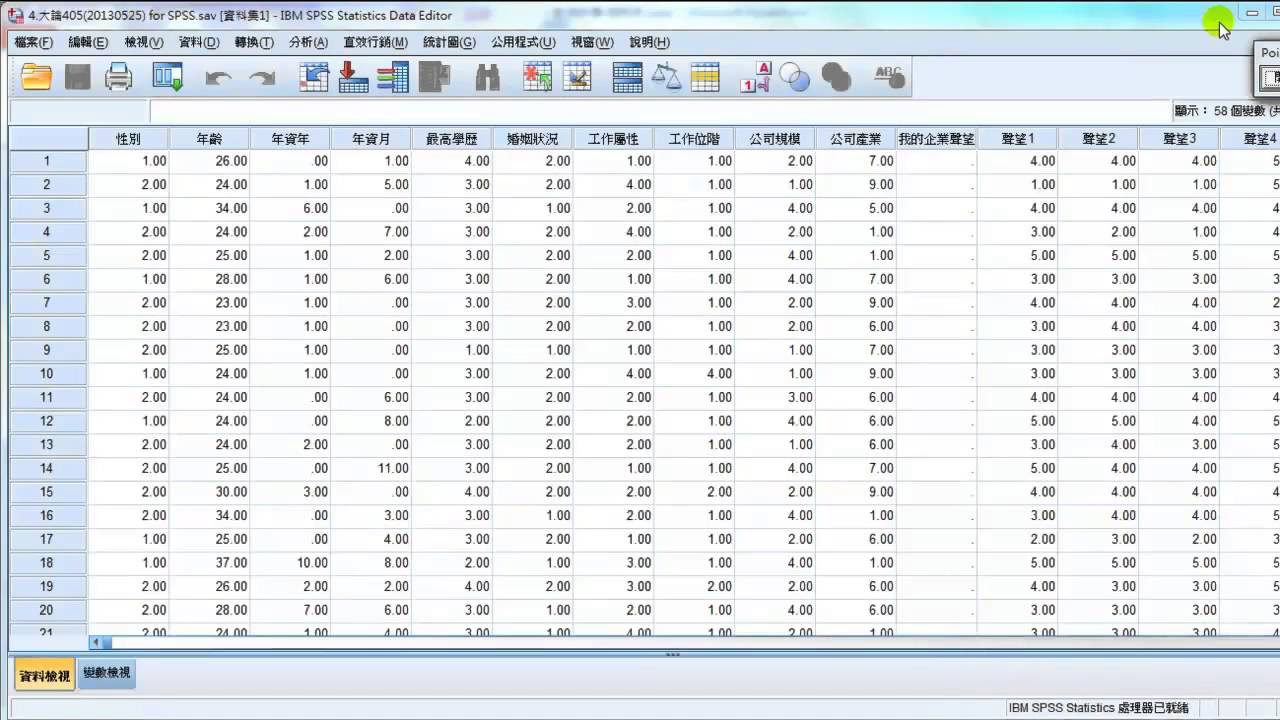
ไธๅคใ็ตฑ่จๅญธ๏ผไฟกๅบฆๅๆ
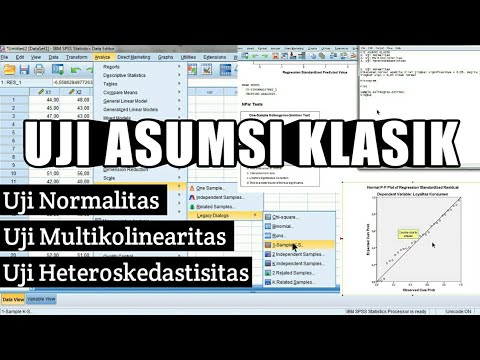
Tutorial SPSS Uji Asumsi Klasik : Uji Normalitas, Uji Multikolinearitas dan Uji Heteroskedastisitas
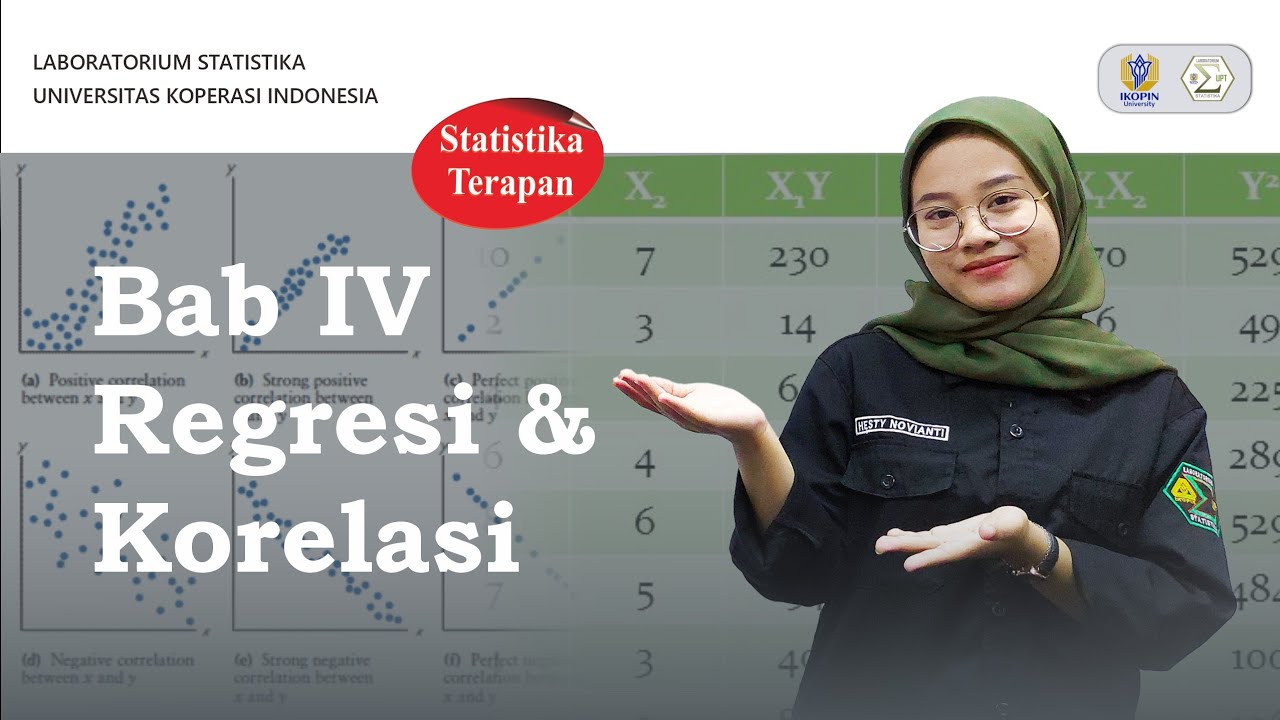
BAB 4 REGRESI KORELASI - Statistika Terapan | Lab Statistika Universitas Koperasi Indonesia
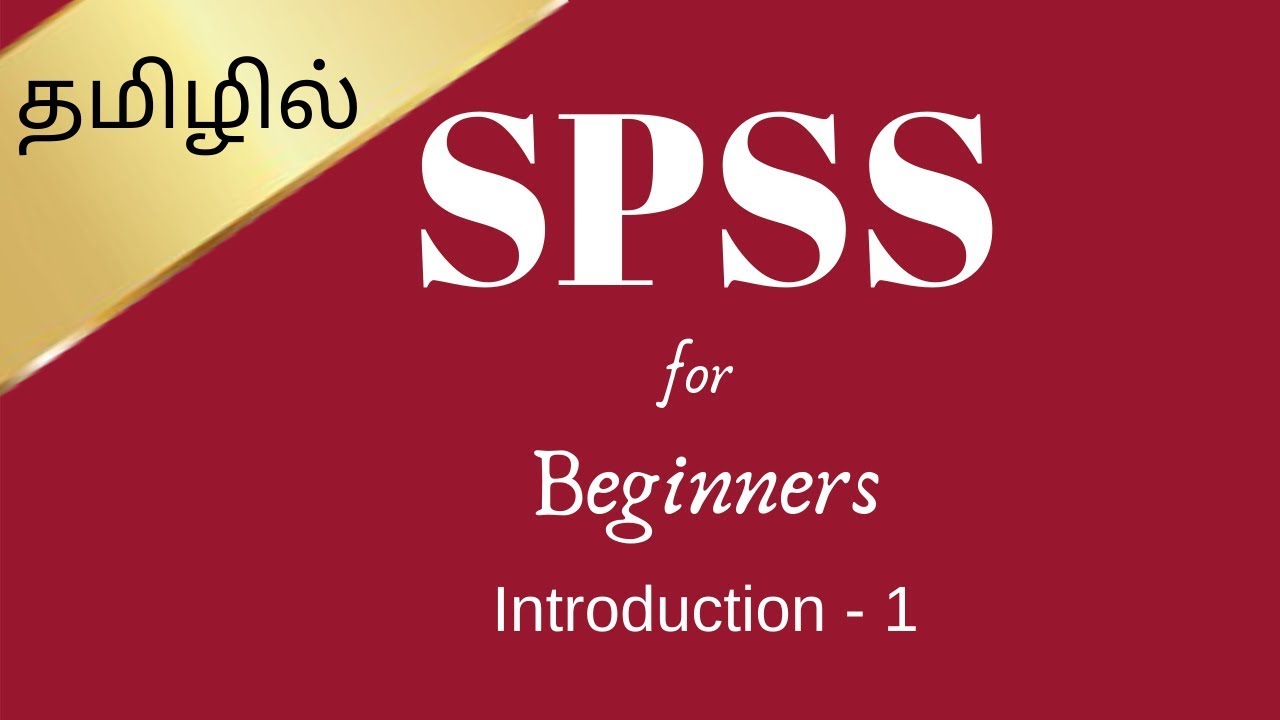
SPSS tutorial in tamil for beginners part -1 | Introduction
5.0 / 5 (0 votes)