Normally distributed errors - finite sample inference
Summary
TLDRThis video discusses the assumption of normality in error terms within finite sample inference. Using two examples, one involving parental income and test scores, and the other involving wages and education, the video explains when the assumption of normally distributed errors holds and when it doesn’t. It emphasizes that in small sample sizes, normality of errors is crucial for inference. The video also contrasts exact statistical methods for normally distributed errors with approximate methods used when errors deviate from normality, highlighting the importance of the central limit theorem and sample size considerations for accurate statistical inference.
Takeaways
- 😀 The assumption of normally distributed errors is crucial for reliable statistical inference, especially in small samples.
- 😀 A graph of test scores against parental income suggests that errors might be approximately normally distributed around the regression line.
- 😀 In the case of wage vs education with a minimum wage constraint, the errors are likely non-normally distributed, particularly in regions where individuals earn the minimum wage or nothing at all.
- 😀 If errors are normally distributed, we can use exact statistical methods, such as the t-test, for hypothesis testing and inference.
- 😀 When errors are non-normally distributed, especially in small samples, we cannot apply the central limit theorem (CLT) directly, and this complicates inference.
- 😀 For small sample sizes (under 30), assuming normal errors becomes very important because the CLT doesn’t apply.
- 😀 In large samples (over 30), even if the errors are non-normal, the CLT allows for asymptotic normality, making standard inference methods more valid.
- 😀 For the wage vs education example, only after a certain threshold of education might we assume normal errors, due to the behavior of wages at lower education levels.
- 😀 The sampling distribution of beta hat (β̂) is normally distributed when errors are normally distributed, which makes inference straightforward.
- 😀 Non-normal errors may require specialized statistical techniques, but in most practical scenarios, the CLT supports inference when the sample size is large enough.
- 😀 The underlying theory of normally distributed errors can be tied to the central limit theorem, but it's often validated by practical testing rather than theory alone.
Q & A
What is the assumption of normality of errors, and why is it important in statistical inference?
-The assumption of normality of errors refers to the idea that the residuals or errors in a regression model are distributed normally. This assumption is important because many statistical methods, such as hypothesis testing and confidence intervals, rely on normality for accurate and reliable inference. When errors are normally distributed, statistical methods like t-tests and confidence intervals can be applied directly. If the assumption is violated, alternative methods may be needed.
How does the scenario with parental income and test scores demonstrate normally distributed errors?
-In the example with parental income and test scores, the assumption of normality holds because the errors (deviations from the regression line) appear to be symmetrically distributed around the line. This suggests that the distribution of errors follows a normal distribution, particularly when considering a large enough sample size, as the errors represent a sum of smaller, idiosyncratic errors that may individually be normally distributed.
Why is the assumption of normally distributed errors likely violated in the second scenario involving wages and education?
-In the scenario with wages and education, the assumption of normality is likely violated because there is a minimum wage threshold. Individuals with low levels of education are likely to earn either the minimum wage or no wage at all, leading to a non-normal distribution of errors. The distribution is truncated on the left, with many individuals clustered at the minimum wage, and the distribution becomes skewed, violating the assumption of normality.
What role does the central limit theorem play in statistical inference regarding error distributions?
-The central limit theorem (CLT) suggests that, as the sample size increases, the sampling distribution of the estimator (like beta hat) will approach a normal distribution, regardless of the distribution of the underlying errors. This is especially important in large samples where we can use approximate methods for statistical inference. However, with smaller samples, the CLT doesn't apply as strongly, and the assumption of normality of errors becomes more critical for valid inference.
How does sample size affect the importance of the normality assumption for error distributions?
-For small sample sizes (n < 30), the normality assumption is critical because the central limit theorem does not apply. This means that errors must be normally distributed for the inference to be valid. For larger sample sizes, the central limit theorem ensures that beta hat will be approximately normally distributed, even if the errors themselves are not. Thus, normality is less crucial in larger samples, but it is still important for small samples.
What is the t statistic, and how does it differ when errors are normally distributed versus non-normally distributed?
-The t statistic is used to test hypotheses about the population parameters (e.g., beta) in a regression model. When errors are normally distributed, the t statistic follows a t-distribution, which allows for precise inference about the population parameter. However, when errors are non-normally distributed, the sampling distribution of the t statistic may not resemble a t-distribution, making standard inference methods inappropriate without adjustments.
What is the main difference between exact statistical methods and asymptotic methods?
-Exact statistical methods are based on the assumption of normality of errors and are valid when the errors are indeed normally distributed. These methods produce precise results, such as exact t-distributions for the t statistic. Asymptotic methods, on the other hand, are used when errors are not normally distributed, but the sample size is large enough for the central limit theorem to apply. These methods provide approximate results and are more suitable when dealing with large samples.
What does the speaker mean by 'non-normal inference,' and why is it beyond the scope of this video?
-Non-normal inference refers to statistical methods designed for situations where the errors are not normally distributed. These methods can be complex and may involve specialized distributions or adjustments to account for the non-normality of errors. The speaker mentions that this topic is beyond the scope of the video because it requires advanced techniques and is less commonly applied compared to standard inference methods when the normality assumption holds.
Why does the speaker state that the practical approach to testing normality is more important than the theoretical justification for normal errors?
-The speaker suggests that, while there is a weak theoretical justification for why errors might be normally distributed (due to the central limit theorem), in practice, statisticians usually focus on testing for normality directly through diagnostic tests, such as Q-Q plots or statistical tests like the Shapiro-Wilk test. This practical approach allows for more reliable results in real-world data analysis, where perfect theoretical conditions may not hold.
How does the speaker conclude the video regarding how to proceed with statistical inference when errors are non-normally distributed?
-The speaker concludes that, in most cases, the sample size will be large enough to invoke the central limit theorem, which allows for approximate inference methods based on the assumption of normality. However, when the sample size is small, or if the errors are distinctly non-normal, statisticians may need to use more specialized non-normal inference methods, which were not covered in this video.
Outlines
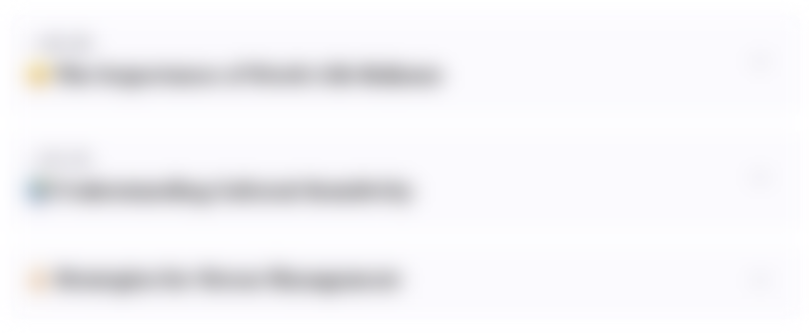
This section is available to paid users only. Please upgrade to access this part.
Upgrade NowMindmap
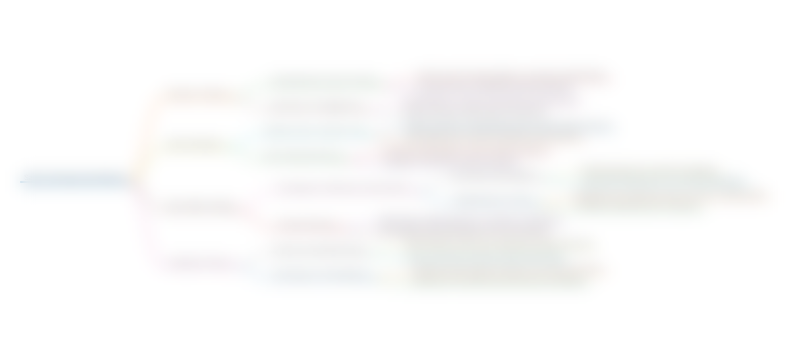
This section is available to paid users only. Please upgrade to access this part.
Upgrade NowKeywords
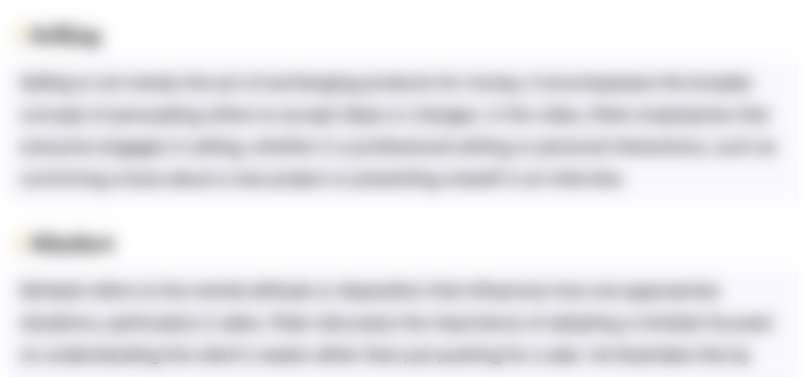
This section is available to paid users only. Please upgrade to access this part.
Upgrade NowHighlights
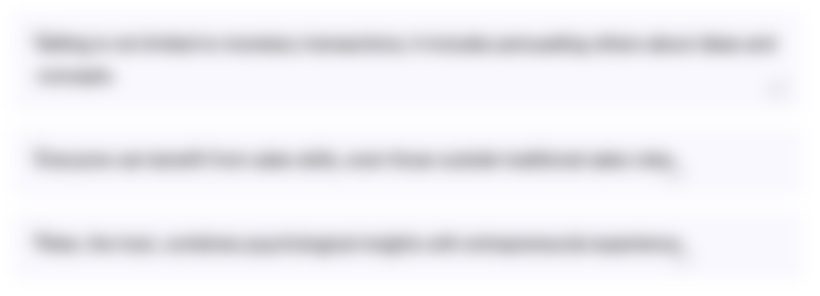
This section is available to paid users only. Please upgrade to access this part.
Upgrade NowTranscripts
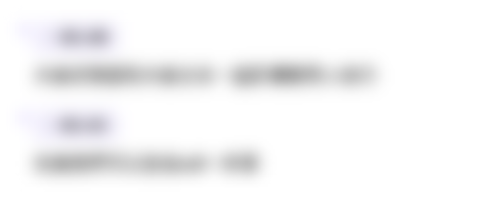
This section is available to paid users only. Please upgrade to access this part.
Upgrade NowBrowse More Related Video

Understanding Statistical Inference - statistics help

Statistical Inference: Introduction and Terminology (in Hindi)
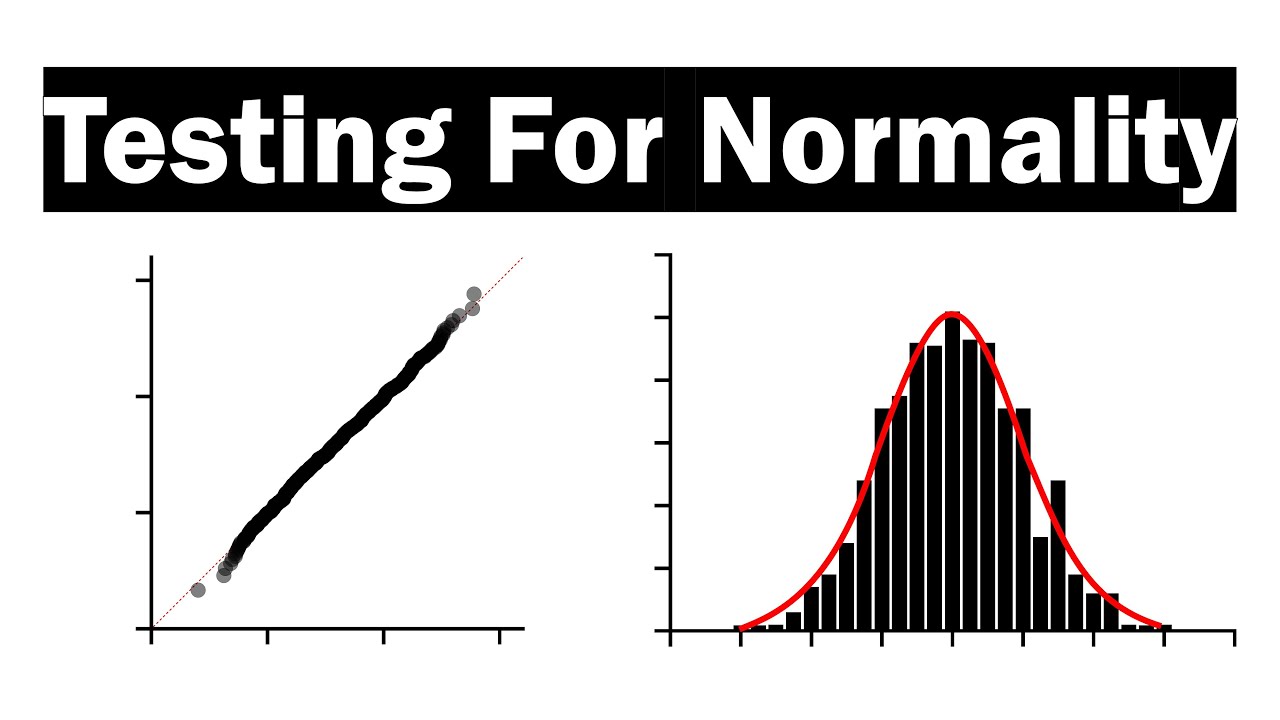
Testing For Normality - Clearly Explained
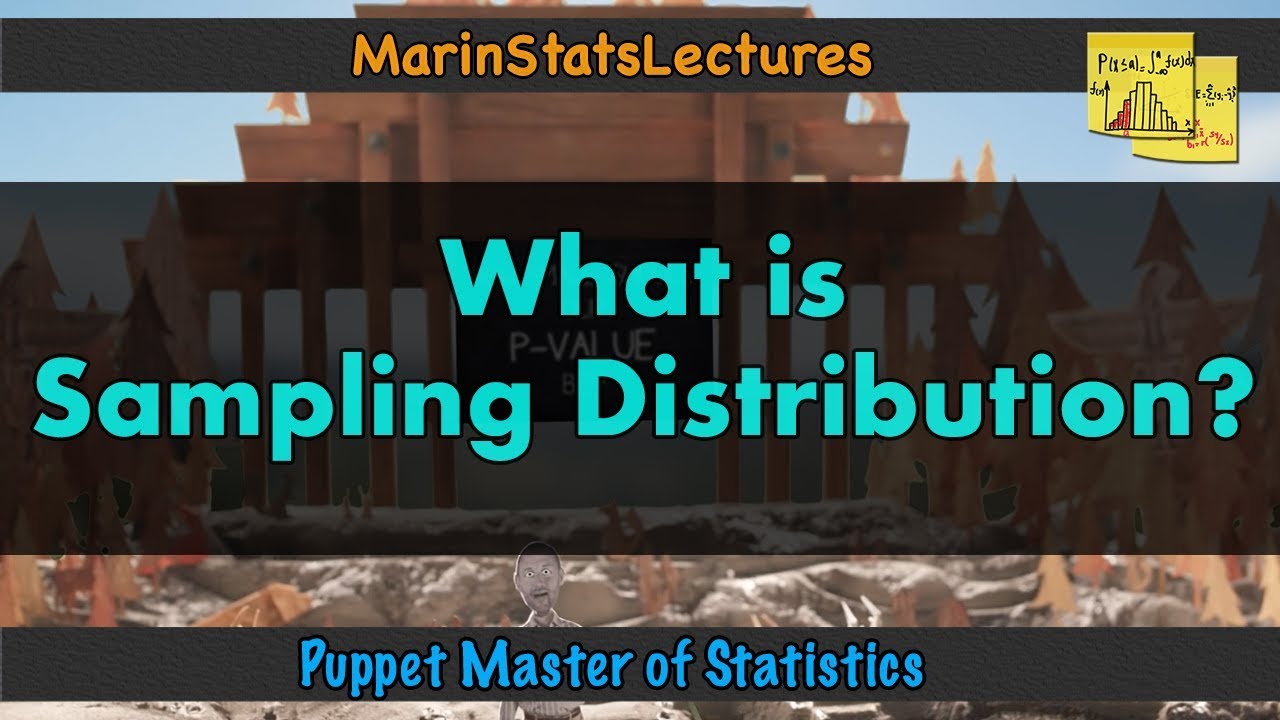
What is a Sampling Distribution? | Puppet Master of Statistics
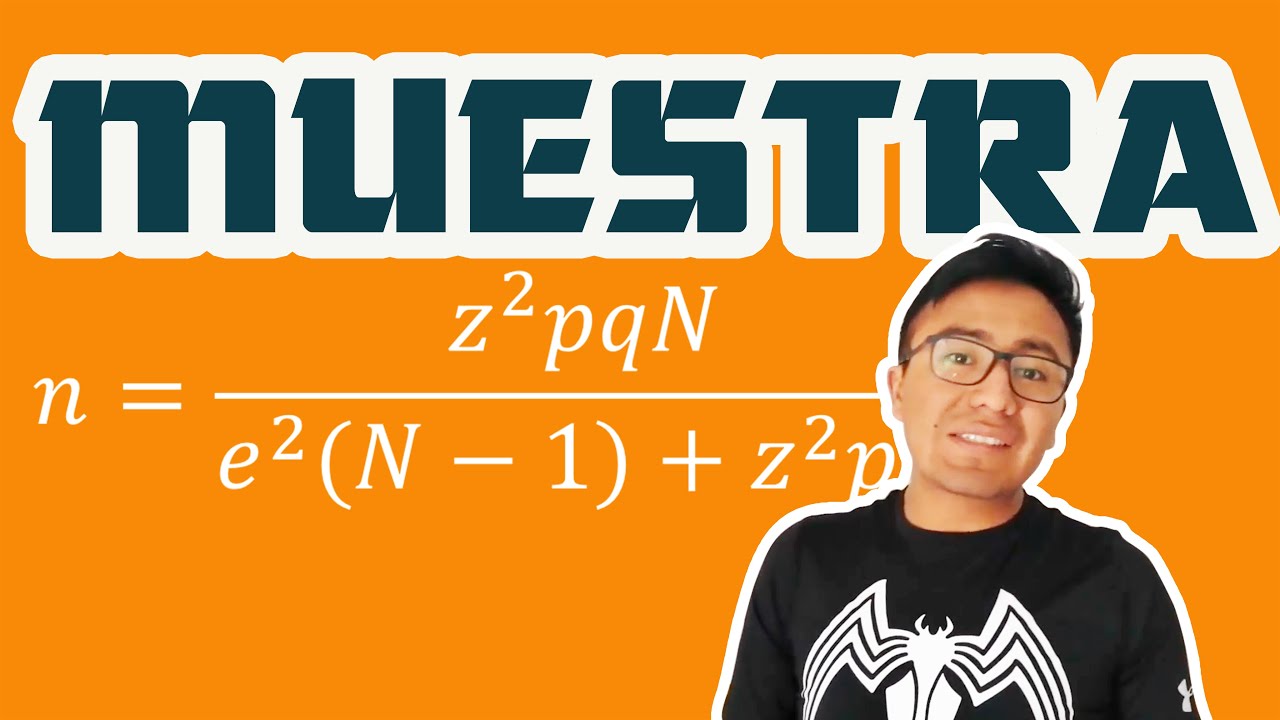
CÓMO OBTENER UNA MUESTRA CON POBLACIÓN FINITA E INFINITA

Two-sample t test for difference of means | AP Statistics | Khan Academy
5.0 / 5 (0 votes)