AI-Driven Supply Chains: 3 Cases | MIT SCALE Webinar | English
Summary
TLDR在这段视频脚本中,来自MIT全球供应链与物流卓越网络(MIT Global SCALE Network)的专家们深入讨论了人工智能(AI)在供应链管理中的应用。Maria Jesus Saenz,MIT数字供应链转型实验室的主任,介绍了AI在供应链转型中的作用,强调了AI不仅是技术更新,而是一个涉及领导力、战略和性能预期的全面方法。通过Dell公司的案例,她展示了如何利用AI实现端到端的供应链优化,提高订单履行的准确性和效率。Dr. Yasel Costa介绍了如何从自然界中汲取灵感,运用生物启发式算法优化运输路线,特别是在Samsonite公司的配送路径优化中的应用。Cagil Kocyigit讨论了AI在资源分配决策中的效率与可解释性之间的平衡,通过洛杉矶无家可归者住房资源分配的实际项目,展示了如何实现两者的兼顾。整个讨论强调了AI在供应链管理中的实用性和战略重要性,以及如何通过AI提高决策的质量、效率和透明度。
Takeaways
- 🌟 人工智能(AI)在供应链管理中的应用是现实且多样的,涵盖了从供应链网络设计到绿色车辆路径规划等多个领域。
- 📈 AI 驱动的供应链转型不仅仅是技术或算法的更新,而是通过数据实现价值驱动的端到端供应链的转变。
- 🤝 MIT Global SCALE Network 是一个全球性的教育和研究中心网络,专注于物流和供应链的实用研究,并与150多个企业合作。
- 📊 Dell 利用 AI 技术优化其供应链,通过预测能力、实时执行和根源分析来提高性能,并使用“完美订单指数”作为衡量标准的 KPI。
- 🚀 Yasel Costa 博士讨论了生物启发式 AI 在优化送货路线中的应用,特别是在动态和随机性高的情境下,如何通过 AI 算法提高效率。
- 💡 Cagil Kocyigit 探讨了 AI 在数据驱动决策中的效率与可解释性之间的平衡,强调了在实践中同时实现高效率和高可解释性的可能性。
- 🔍 AI 可以帮助提高端到端的可见性,不仅限于内部 ERP 系统的数据,还包括外部信号,如供应商的 ESG 评分等实时信息。
- 📉 在智利圣地亚哥的一个案例中,通过使用蚁群优化(ACO)算法,成功将车队规模减少了50%,并显著降低了运输成本。
- 🤔 AI 在供应链中的应用需要考虑数据的成熟度和质量,以及如何结合公司的具体需求和上下文来定制解决方案。
- 📚 即使是非供应链直接相关的项目,如洛杉矶无家可归者的住房分配问题,其数据驱动的解决方案方法也可以启发供应链和物流中资源分配问题的新思路。
- ⚖️ 在使用 AI 进行决策时,需要考虑潜在的伦理问题,如歧视等,确保 AI 系统的公平性和透明度。
Q & A
如何理解MIT Global SCALE Network在供应链管理中应用AI的愿景?
-MIT Global SCALE Network的愿景是通过应用研究分享供应链的未来,与150多个企业合作伙伴合作,每年教育200多名学生,并拥有遍布全球的校友网络。他们强调AI在当今供应链和运营中的实际应用,展示了AI作为现实的一部分。
在供应链中应用AI时,如何避免‘弗兰肯斯坦效应’?
-‘弗兰肯斯坦效应’指的是AI的不同组件如最后一公里交付和预测等不相互交流,像孤立的部分需要不断磨合以形成对AI的全面视角。解决这个问题需要一个长期的旅程,需要公司有能力扩展AI应用,从原型制作到更广泛的区域、流程和产品。
Dell如何使用AI来优化其端到端供应链?
-Dell通过将AI集成到其供应链中,专注于其愿景和战略,以及性能预期。他们开发了五个体验,特别关注‘做出正确的承诺’。Dell使用AI进行预测、执行实时的预定动作,并在订单后进行根因分析,以提高供应链的效率和响应能力。
Yasel Costa博士如何将自然启发式算法应用于物流优化?
-Yasel Costa博士通过使用自然启发式算法,如进化算法和蚁群优化,来解决物流中的配送路线优化问题。这些算法模仿自然界的行为,如物种进化和蚂蚁寻找食物的最短路径,以提高物流网络设计的效率。
Cagil Kocyigit博士如何平衡AI决策的效率和可解释性?
-Cagil Kocyigit博士通过讨论她的项目,展示了在实践中同时实现AI决策的效率和可解释性的可能性。她强调了人类理解决策过程的重要性,以便信任并实施由模型做出的决策,特别是在资源分配问题上。
如何使用AI提高供应链的端到端可见性?
-除了使用ERP等内部系统外,还可以利用AI集成外部信号,如供应商的实时数据、市场变化等,以丰富供应链的可见性。AI可以从结构化和非结构化数据中学习,提供更全面的决策支持。
在AI驱动的供应链转型中,如何量化价值创造?
-可以通过开发关键绩效指标(KPI)来量化价值创造,如Dell的完美订单指数(POI),它衡量订单从准备到交付的每个环节的准确率。此外,通过关键学习指标(KLIs)监测AI学习进度,并将其转化为经济效益。
在实施新的AI算法驱动的补货软件时,如何处理数据成熟度不足的问题?
-即使数据成熟度不足,也可以采用AI模型,这些模型可以从有限的数据中生成信息,或者使用模拟数据和模糊推理系统来处理数据稀缺性问题。关键是找到适合当前数据条件的AI应用方法。
使用AI进行需求预测的改进百分比范围是多少?
-没有固定的百分比范围,因为AI在需求预测中的改进取决于多种因素,包括数据的质量、模型的选择和业务的具体需求。需要根据具体情况定制和优化AI模型,以实现最佳的预测效果。
在AI决策中,如何避免潜在的歧视或伦理问题?
-仅仅从数据中移除某些信息(如种族)并不足以防止AI使用这些信息进行决策,因为其他高度相关的数据可能暗示了这些信息。需要采取更全面的策略,包括使用公平的算法、透明度和持续的监控,以确保AI决策的公正性。
AI和数据科学在供应链管理中有什么不同?
-AI和数据科学在供应链管理中是相互重叠的领域。数据科学更侧重于从数据中发现知识,而AI则强调机器的学习和认知功能。在实际应用中,两者往往结合使用,以提高供应链的效率和效果。
Outlines
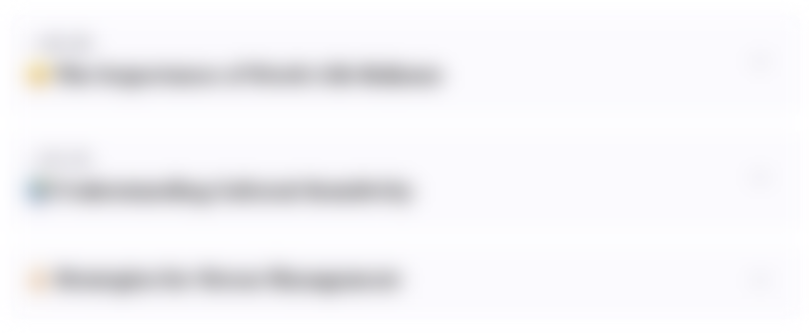
This section is available to paid users only. Please upgrade to access this part.
Upgrade NowMindmap
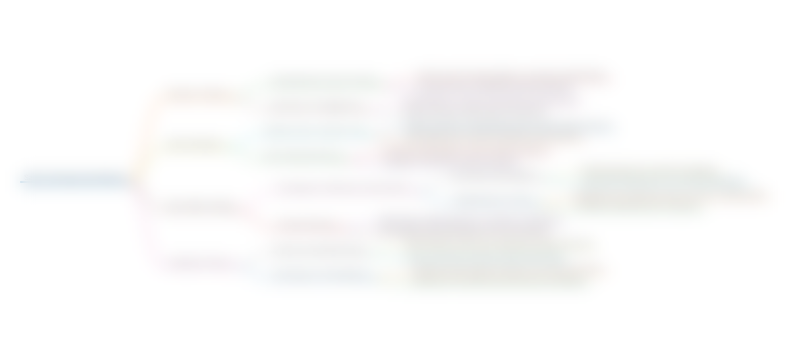
This section is available to paid users only. Please upgrade to access this part.
Upgrade NowKeywords
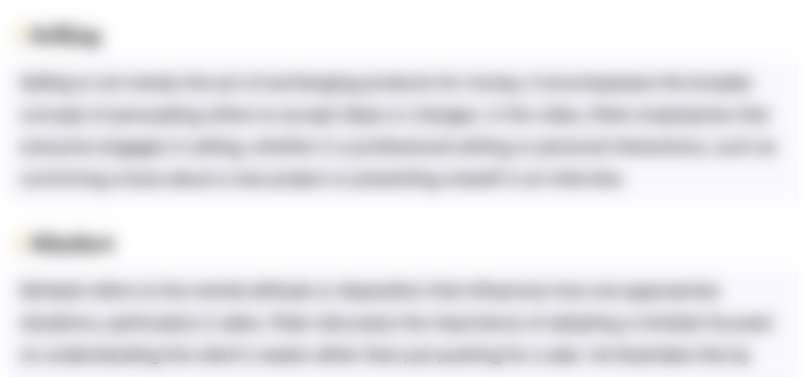
This section is available to paid users only. Please upgrade to access this part.
Upgrade NowHighlights
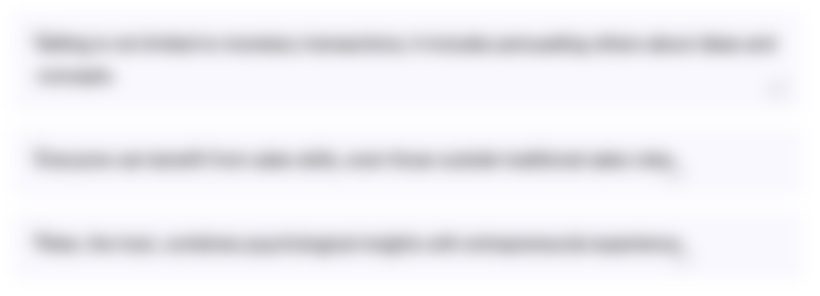
This section is available to paid users only. Please upgrade to access this part.
Upgrade NowTranscripts
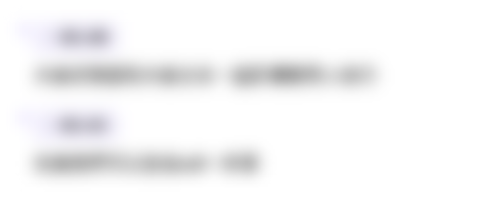
This section is available to paid users only. Please upgrade to access this part.
Upgrade NowBrowse More Related Video
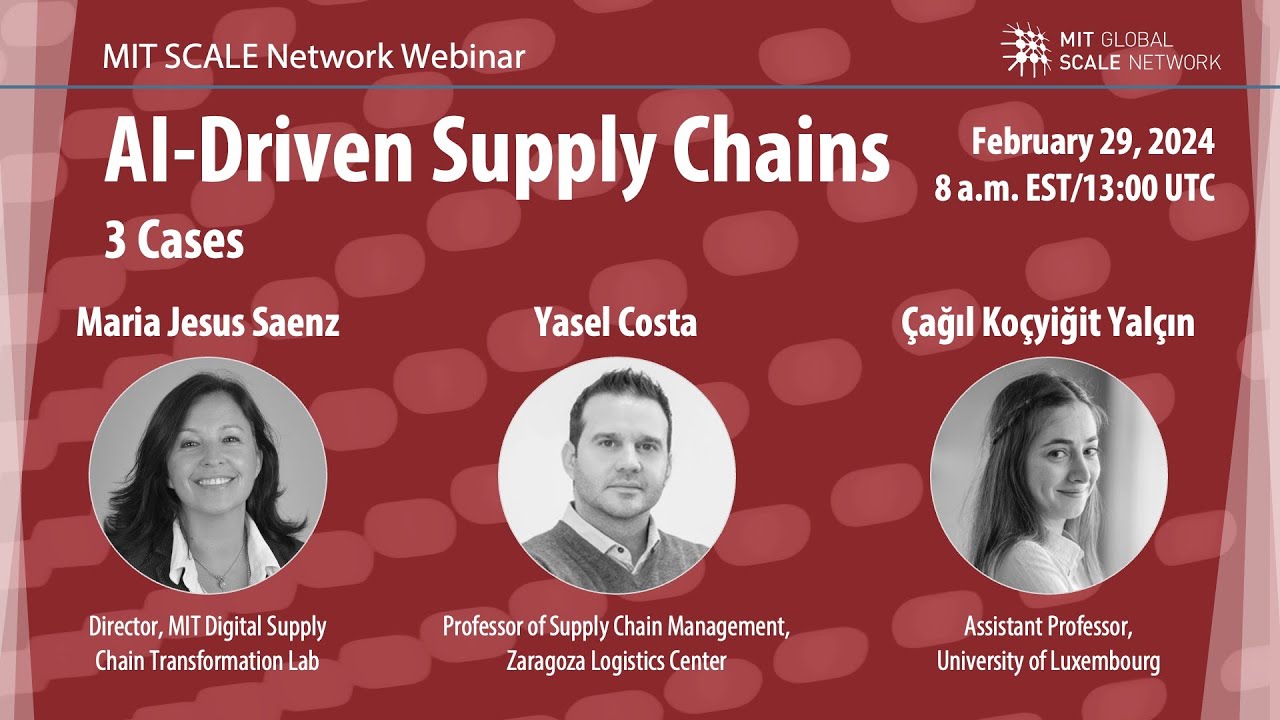
AI-Driven Supply Chains: 3 Cases | MIT SCALE Webinar | Spanish
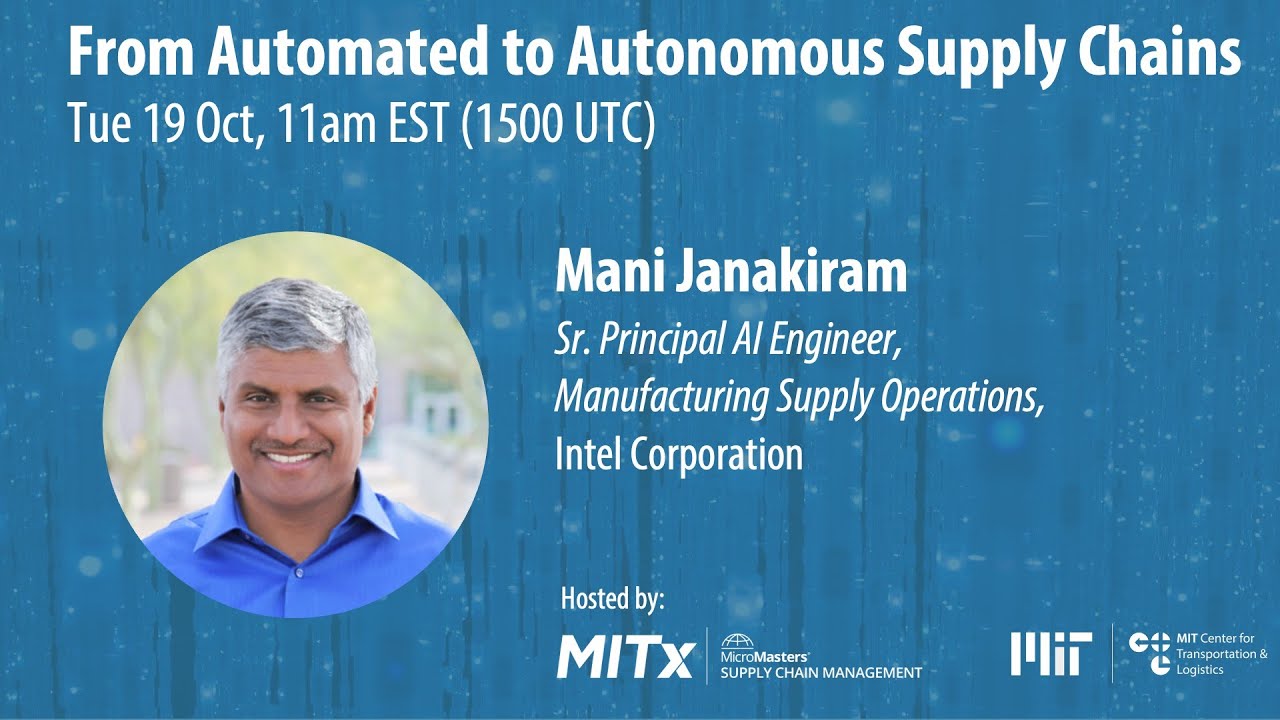
From Automated to Autonomous Supply Chains
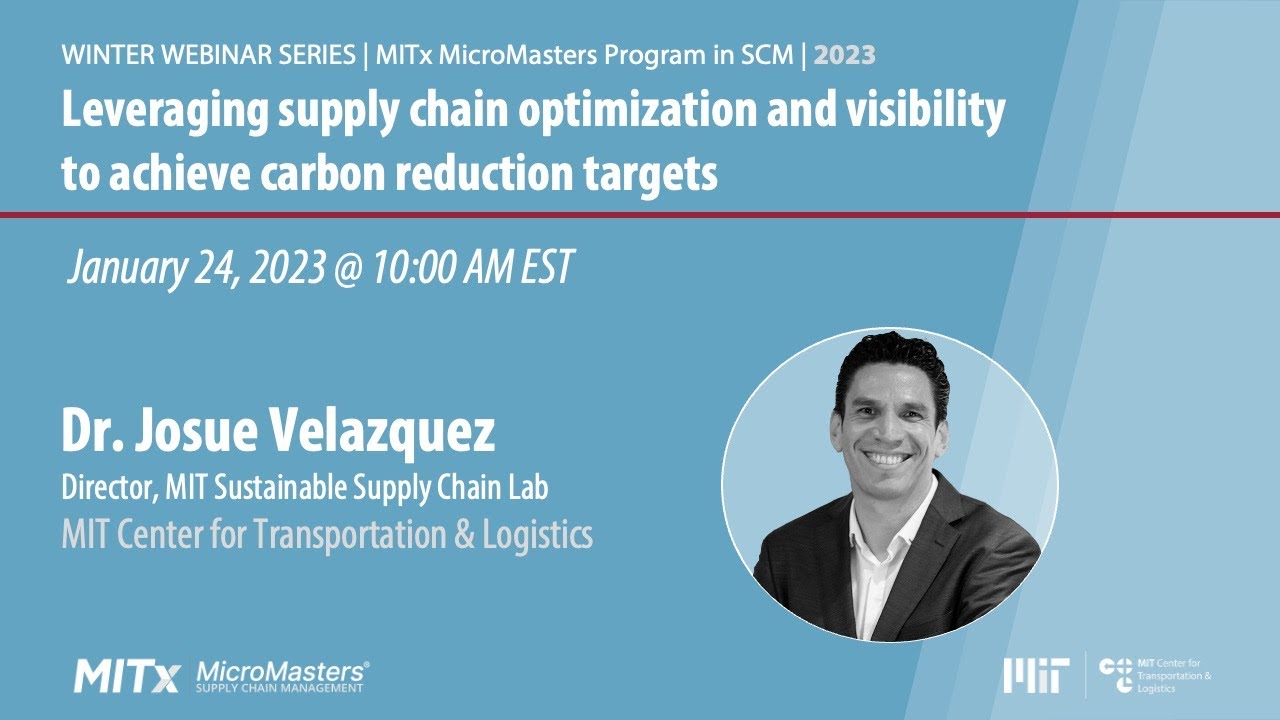
Leveraging supply chain optimization and visibility to achieve carbon reduction targets
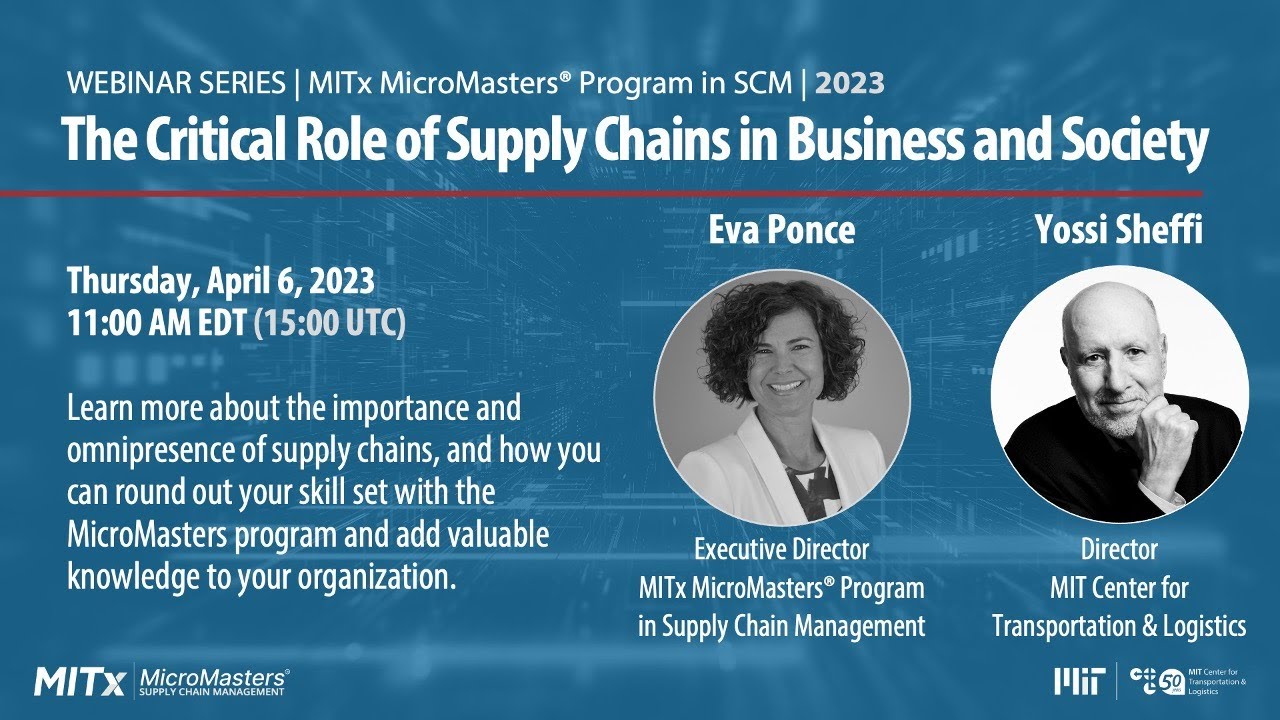
The Critical Role of Supply Chains in Business and Society
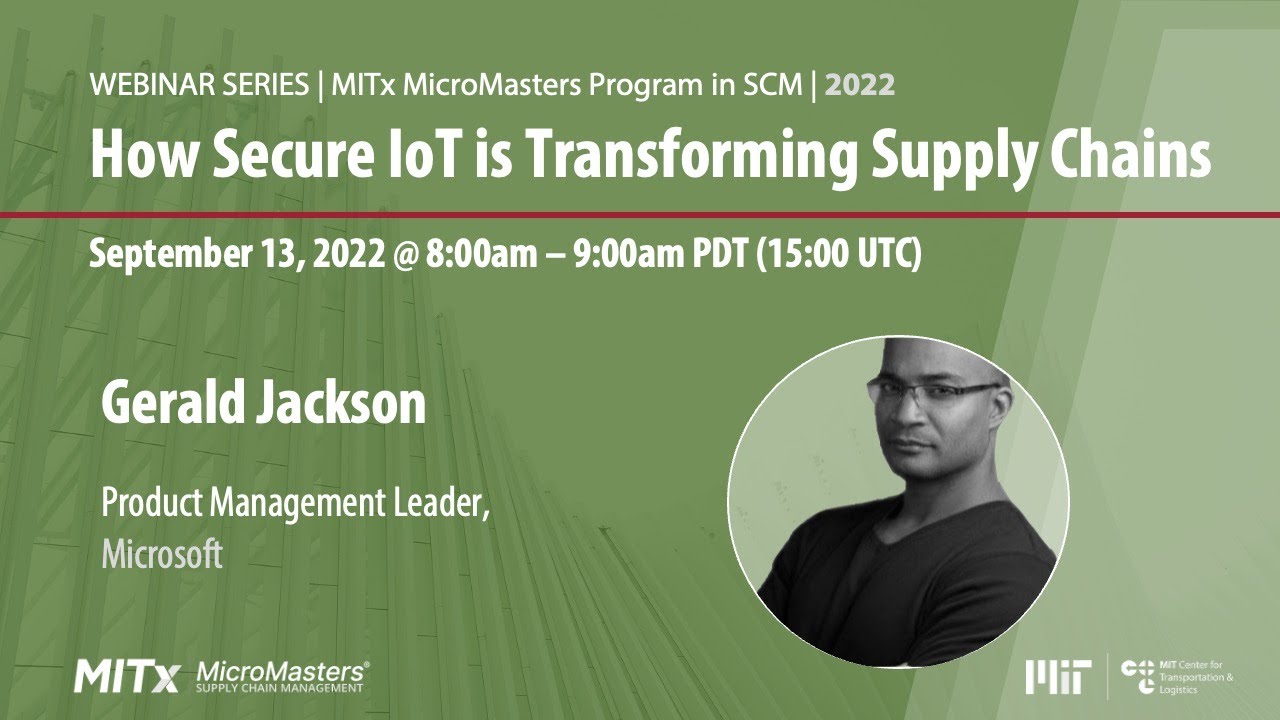
How Secure IoT is Transforming Supply Chains
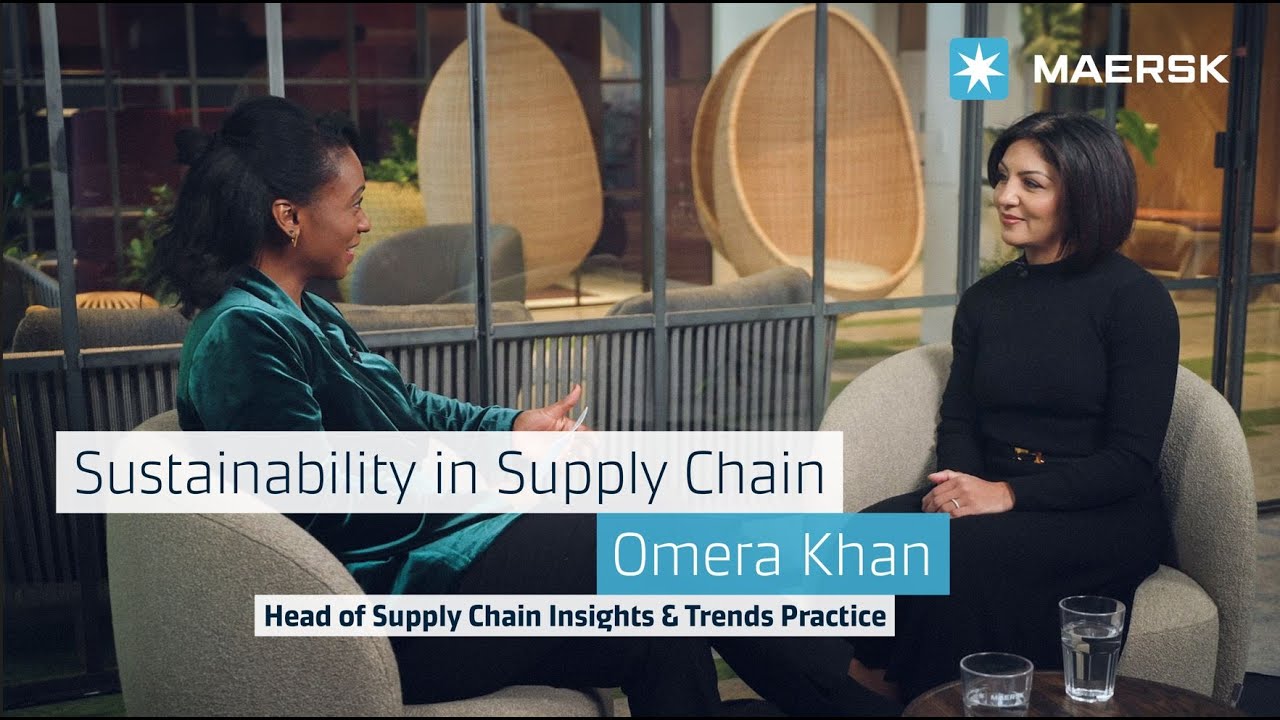
Omera Khan: Building robust & resilient supply chains in today's dynamic business environment
5.0 / 5 (0 votes)