What is a hypothesis test? A beginner's guide to hypothesis testing!
Summary
TLDRThis video explains hypothesis testing, a statistical method for determining if there is sufficient evidence in a sample to make inferences about a population. It distinguishes between populations and samples, outlines the steps involved in hypothesis testing, and clarifies the concepts of null and alternative hypotheses. Key components include calculating the P-value to assess statistical significance and recognizing Type I and Type II errors. The video also highlights tools for selecting and interpreting appropriate hypothesis tests, enhancing the viewer's understanding of data analysis.
Takeaways
- 😀 Hypothesis testing helps determine if there is enough evidence in a sample to infer a condition about a population.
- 👥 The population is the entire group of interest, while the sample is a smaller subset used for analysis.
- 📝 A hypothesis is a statement we test about a population parameter, such as the effect of a drug on blood pressure.
- 🔄 The null hypothesis (H0) assumes no effect, while the alternative hypothesis (H1) suggests there is an effect.
- 📊 To conduct a hypothesis test, researchers collect sample data and calculate how it deviates from the null hypothesis.
- 📉 The P-value indicates the probability of obtaining the sample results under the null hypothesis; a low P-value suggests significant evidence against H0.
- ✅ If the P-value is less than a predetermined threshold (commonly 0.05), the null hypothesis is rejected, indicating statistical significance.
- ⚖️ There are two types of errors in hypothesis testing: Type I error (false positive) occurs when the null hypothesis is wrongly rejected, and Type II error (false negative) occurs when it is not rejected when it should be.
- 🔧 Data analysis tools can help determine the appropriate hypothesis test and interpret the results efficiently.
- 📈 Understanding both parametric and nonparametric tests is crucial for selecting the right method for hypothesis testing.
Q & A
What is the purpose of hypothesis testing?
-Hypothesis testing is used to determine whether there is enough evidence in a sample of data to infer that a certain condition is true for the entire population.
What distinguishes a population from a sample?
-The population is the whole group of interest (e.g., all adults in the U.S.), while a sample is a smaller group selected from that population for analysis (e.g., 150 adults).
What are the two types of hypotheses involved in hypothesis testing?
-The two types of hypotheses are the null hypothesis (H0), which assumes no effect or difference, and the alternative hypothesis (H1), which is what you want to prove.
What is a P-value in hypothesis testing?
-The P-value indicates the probability of observing the sample data, or something more extreme, assuming the null hypothesis is true.
How do you determine whether to reject the null hypothesis?
-You compare the P-value to a predetermined significance level (commonly set at 0.05). If the P-value is less than this threshold, you reject the null hypothesis.
What does a statistically significant result imply?
-A statistically significant result implies that the observed data is unlikely to have occurred by chance, providing evidence against the null hypothesis.
What are Type I and Type II errors in hypothesis testing?
-A Type I error occurs when a true null hypothesis is rejected (false positive), while a Type II error occurs when a false null hypothesis is not rejected (false negative).
How can you find a suitable hypothesis test for your data?
-You can use data analysis tools (like data.net) that suggest appropriate tests based on your data, such as Chi-square or ANOVA, and provide interpretations of the results.
What is the significance of the predetermined threshold in hypothesis testing?
-The predetermined threshold, often set at 0.05, helps researchers decide whether the evidence is strong enough to reject the null hypothesis.
Can a large P-value prove that the null hypothesis is true?
-No, a large P-value does not prove that the null hypothesis is true; it only suggests that the observed data is consistent with the null hypothesis.
Outlines
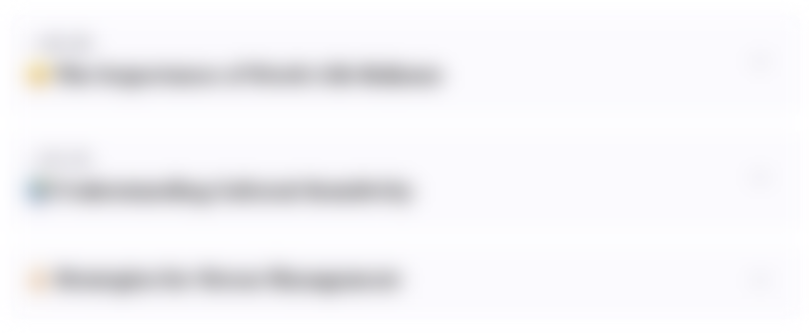
This section is available to paid users only. Please upgrade to access this part.
Upgrade NowMindmap
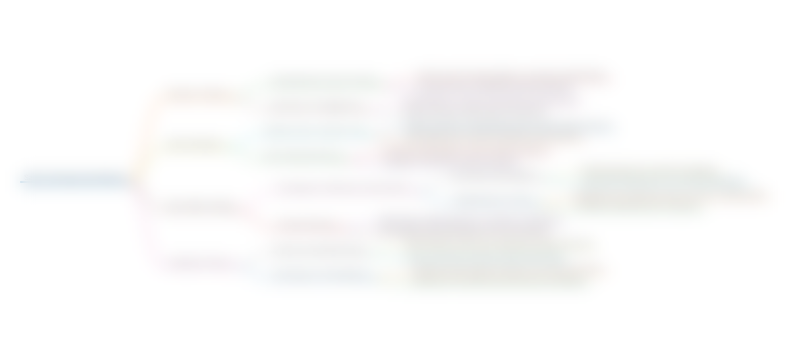
This section is available to paid users only. Please upgrade to access this part.
Upgrade NowKeywords
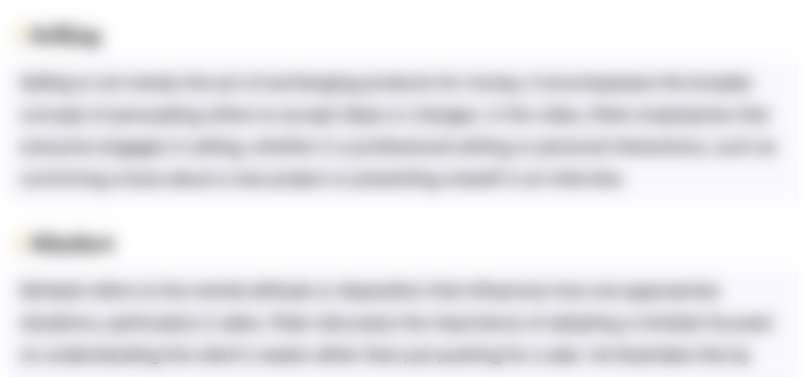
This section is available to paid users only. Please upgrade to access this part.
Upgrade NowHighlights
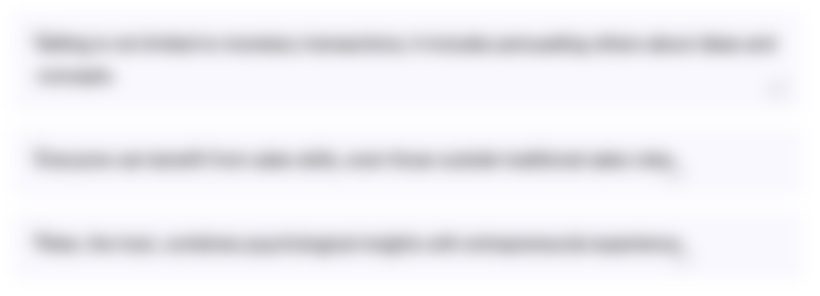
This section is available to paid users only. Please upgrade to access this part.
Upgrade NowTranscripts
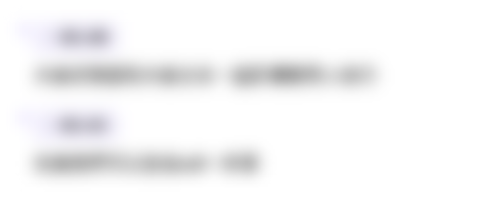
This section is available to paid users only. Please upgrade to access this part.
Upgrade NowBrowse More Related Video
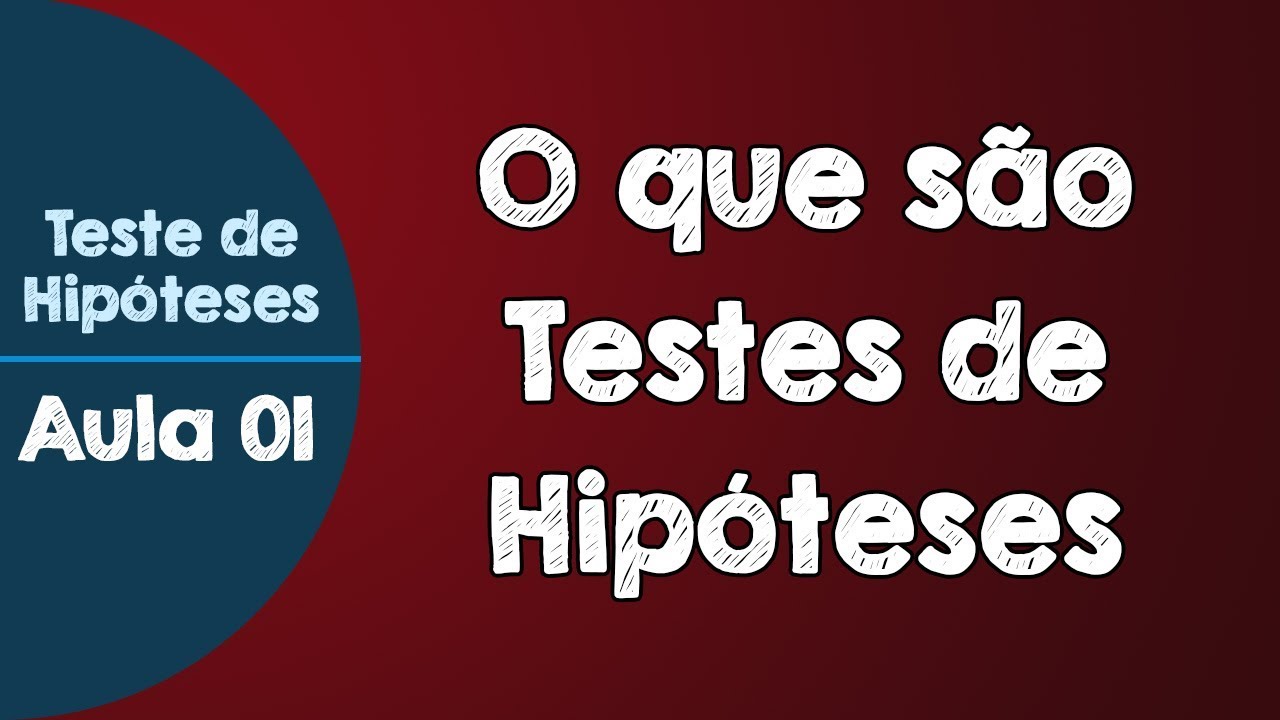
#01 - O que são Testes de Hipóteses | Para que servem os Testes de Hipóteses

Statistical Inference: Introduction and Terminology (in Hindi)
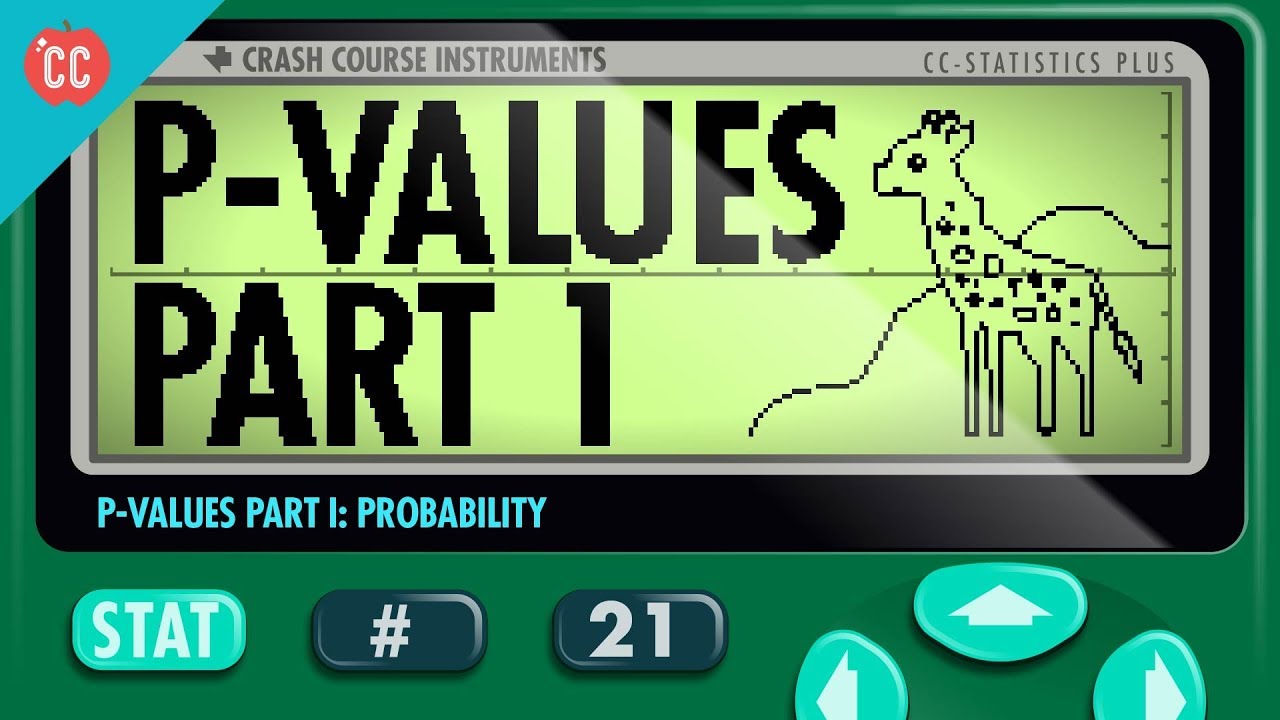
How P-Values Help Us Test Hypotheses: Crash Course Statistics #21
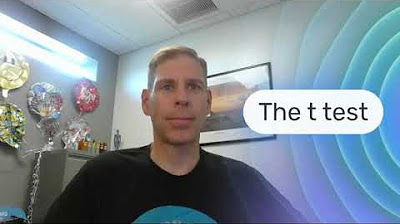
t-tests mini lecture

Understanding Statistical Inference - statistics help
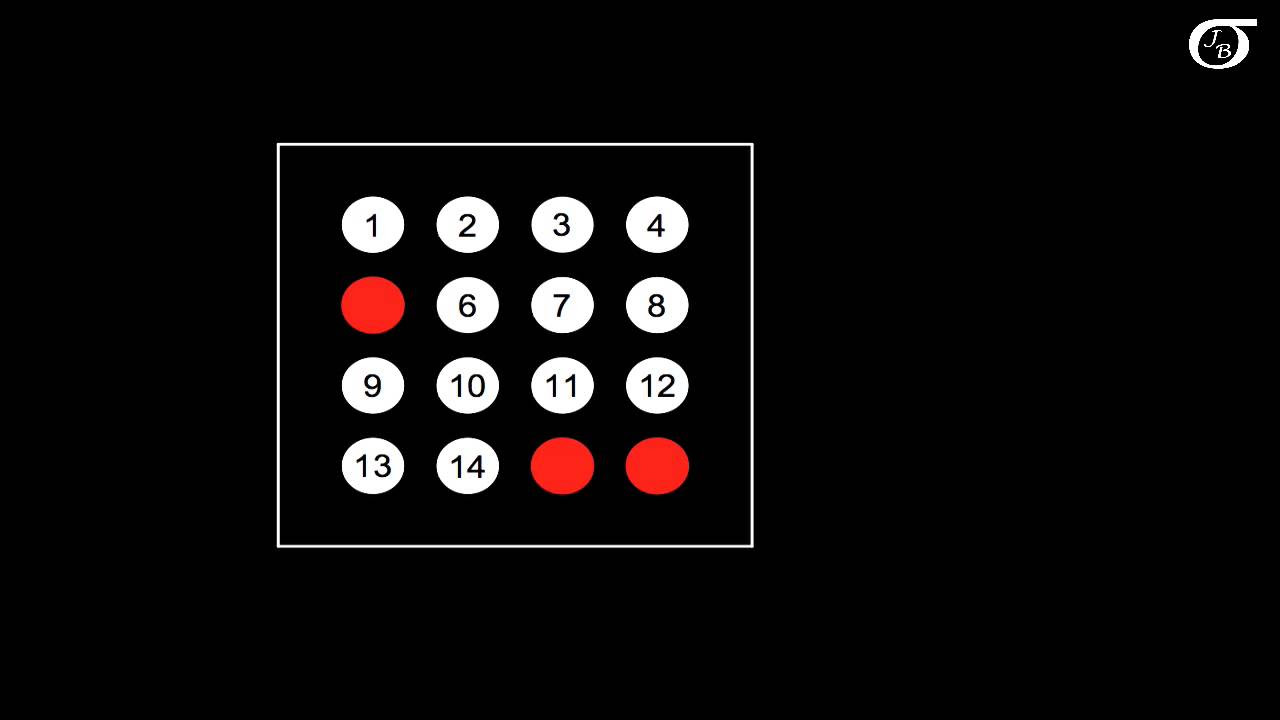
Sampling Distributions: Introduction to the Concept
5.0 / 5 (0 votes)