How P-Values Help Us Test Hypotheses: Crash Course Statistics #21
Summary
TLDRIn this episode of Crash Course Statistics, Adriene Hill delves into statistical inference, exploring how to make inferences about unknown data based on existing data. She introduces the concept of uncertainty and hypothesis testing, using examples like the impact of listening to Mozart on calculus grades and the average number of spots on baby giraffes. Hill explains the Null Hypothesis Significance Testing (NHST) and the role of p-values in determining statistical significance, challenging the traditional 0.05 cutoff and encouraging a deeper understanding of the evidence provided by p-values.
Takeaways
- π Statistical inference is about making inferences about data we don't have, based on data we already have.
- π Uncertainty is central to inferential statistics, which involves making decisions about ideas or hypotheses.
- π΅ An example given is whether listening to Mozart improves calculus grades, illustrating the need for evidence to support hypotheses.
- π Data is essential for testing hypotheses; it provides the basis for comparison and analysis.
- π§ Sample parameters, such as the mean, are estimates and may not fully represent the true population mean.
- π To account for random variation, we need methods to test hypotheses beyond just comparing sample means.
- π¦ A giraffe spots example is used to demonstrate how data can be used to evaluate the likelihood of different hypotheses.
- βοΈ Null Hypothesis Significance Testing (NHST) is a common method for testing hypotheses, which assumes no effect and tests for deviations.
- π’ P-values are used to determine how 'rare' observed data is under the null hypothesis, indicating the probability of such data occurring by chance.
- π A p-value threshold, often set at 0.05, is used to decide whether the observed data is sufficiently 'extreme' to reject the null hypothesis.
- β οΈ The concept of 'statistical significance' does not always equate to practical significance or meaningfulness.
Q & A
What is the main topic of this Crash Course Statistics video?
-The main topic of this video is statistical inference, which is the process of making inferences about data we don't have based on the data we do have.
What is the core concept of Inferential Statistics?
-The core concept of Inferential Statistics is uncertainty, which is about making decisions about ideas or hypotheses based on the analysis of data.
Why can't we claim a hypothesis is correct just by having an idea without any evidence?
-We can't claim a hypothesis is correct without evidence because it lacks empirical support; hypotheses must be tested and validated through data collection and analysis.
What is the significance of sampling in hypothesis testing?
-Sampling is significant in hypothesis testing because it allows us to estimate population parameters and test our hypotheses without examining the entire population, which is often impractical.
Why is it a problem if we find a higher average score in the Mozart group compared to the silent group?
-It's a problem because a higher sample mean doesn't necessarily indicate a true difference in the population; it could be due to random variation, and we need to account for this when testing our hypothesis.
What is the concept of a 'null hypothesis' in Null Hypothesis Significance Testing (NHST)?
-The null hypothesis in NHST is a statement of no effect or no difference. It serves as a baseline against which we compare our observed data to determine if the results are statistically significant.
What is a p-value and why is it important in statistical testing?
-A p-value is the probability of obtaining data as extreme as the observed data, assuming the null hypothesis is true. It's important because it helps determine the likelihood of observing the data by chance alone, thus informing whether to reject the null hypothesis.
What does it mean to 'reject' the null hypothesis?
-To 'reject' the null hypothesis means that the data provides sufficient evidence against the null hypothesis, suggesting that the observed effect or difference is statistically significant and not likely due to random chance.
Why might a one-sided p-value be different from a two-sided p-value?
-A one-sided p-value considers only the probability of the sample mean being higher than a certain value, while a two-sided p-value considers how far the sample mean is from the population mean in either direction. This difference is important when the direction of the effect is unknown or when the effect could go in either direction.
What is the significance of the 0.05 cutoff in p-value interpretation?
-The 0.05 cutoff is a commonly used threshold in statistical testing. If the p-value is less than 0.05, it is considered statistically significant, indicating that there is strong evidence against the null hypothesis and in favor of the alternative hypothesis.
What is reductio ad absurdum, and how is it related to Null Hypothesis Significance Testing?
-Reductio ad absurdum is a form of argument that disproves a proposition by assuming it to be true and then showing that this leads to a contradiction. In NHST, it is used by assuming the null hypothesis to be true and then demonstrating that the observed data is unlikely under this assumption, thus discrediting the null hypothesis.
Outlines
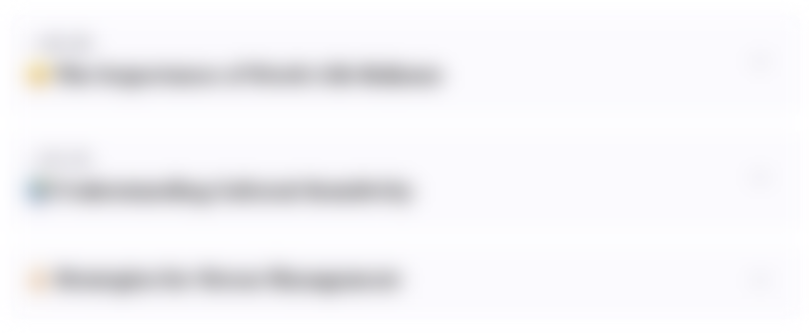
This section is available to paid users only. Please upgrade to access this part.
Upgrade NowMindmap
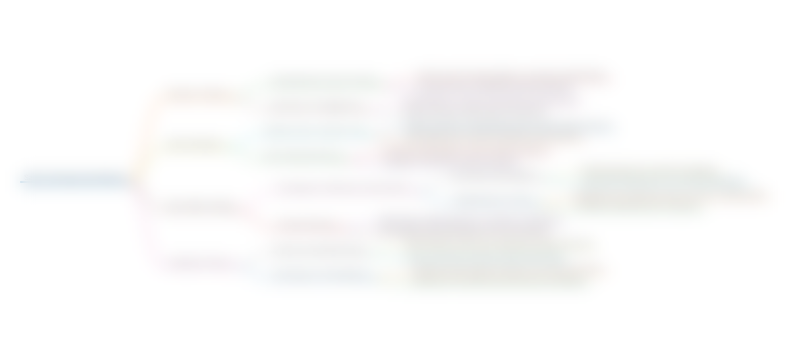
This section is available to paid users only. Please upgrade to access this part.
Upgrade NowKeywords
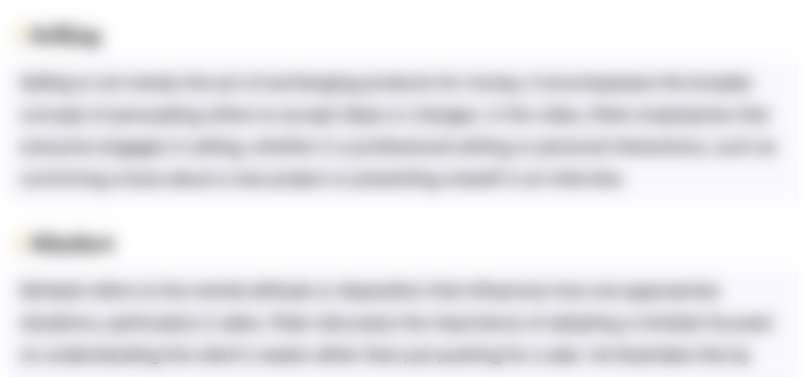
This section is available to paid users only. Please upgrade to access this part.
Upgrade NowHighlights
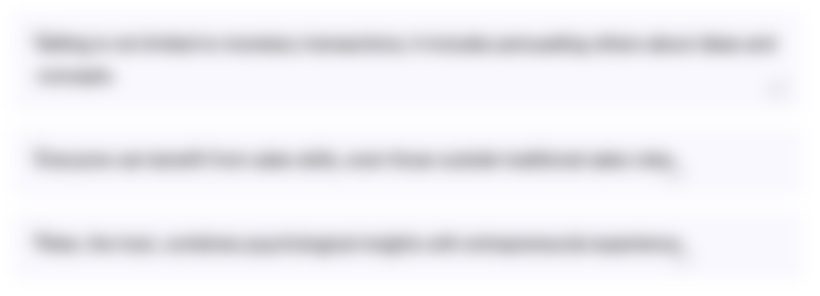
This section is available to paid users only. Please upgrade to access this part.
Upgrade NowTranscripts
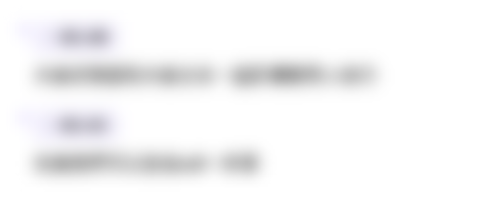
This section is available to paid users only. Please upgrade to access this part.
Upgrade NowBrowse More Related Video

Test Statistics: Crash Course Statistics #26
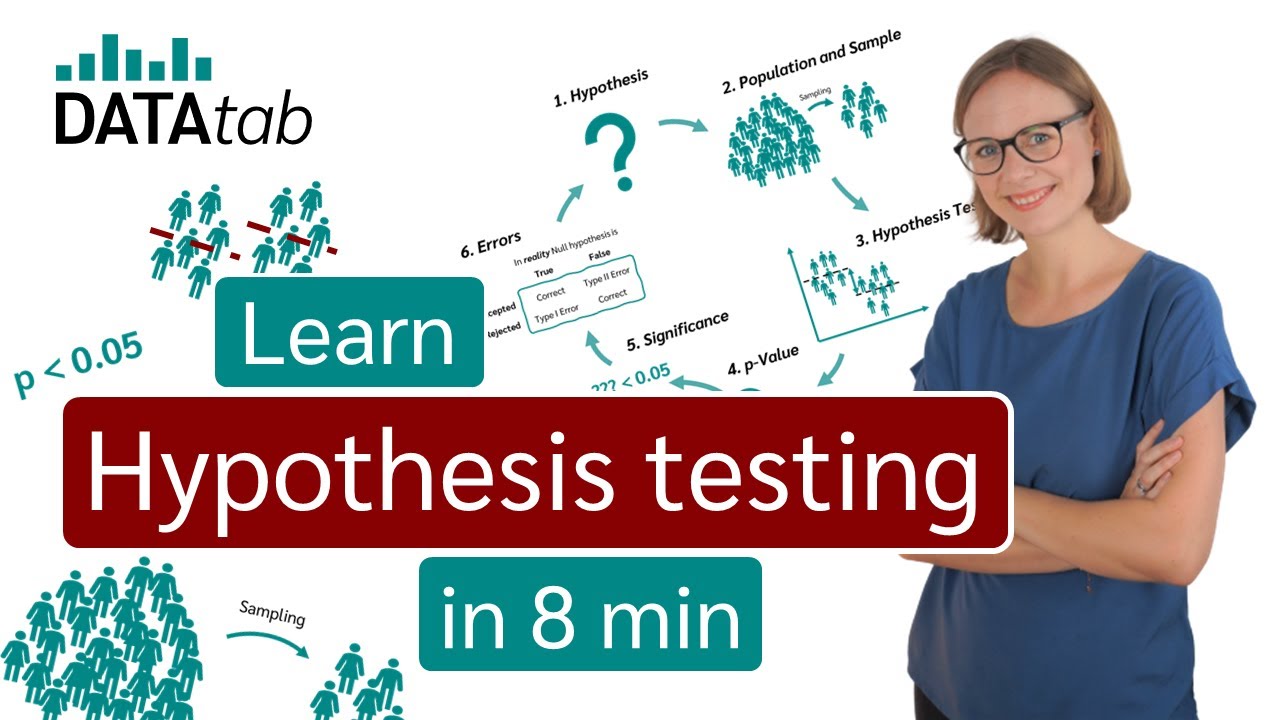
What is a hypothesis test? A beginner's guide to hypothesis testing!
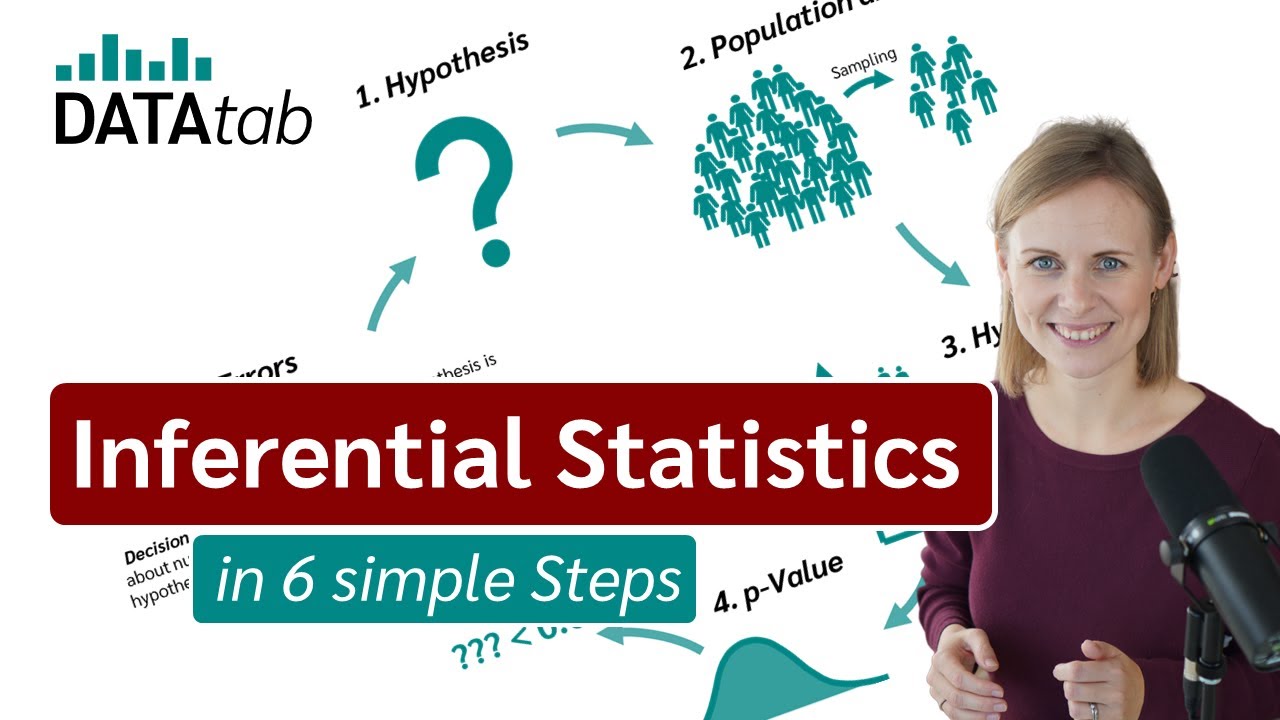
What is inferential statistics? Explained in 6 simple Steps.
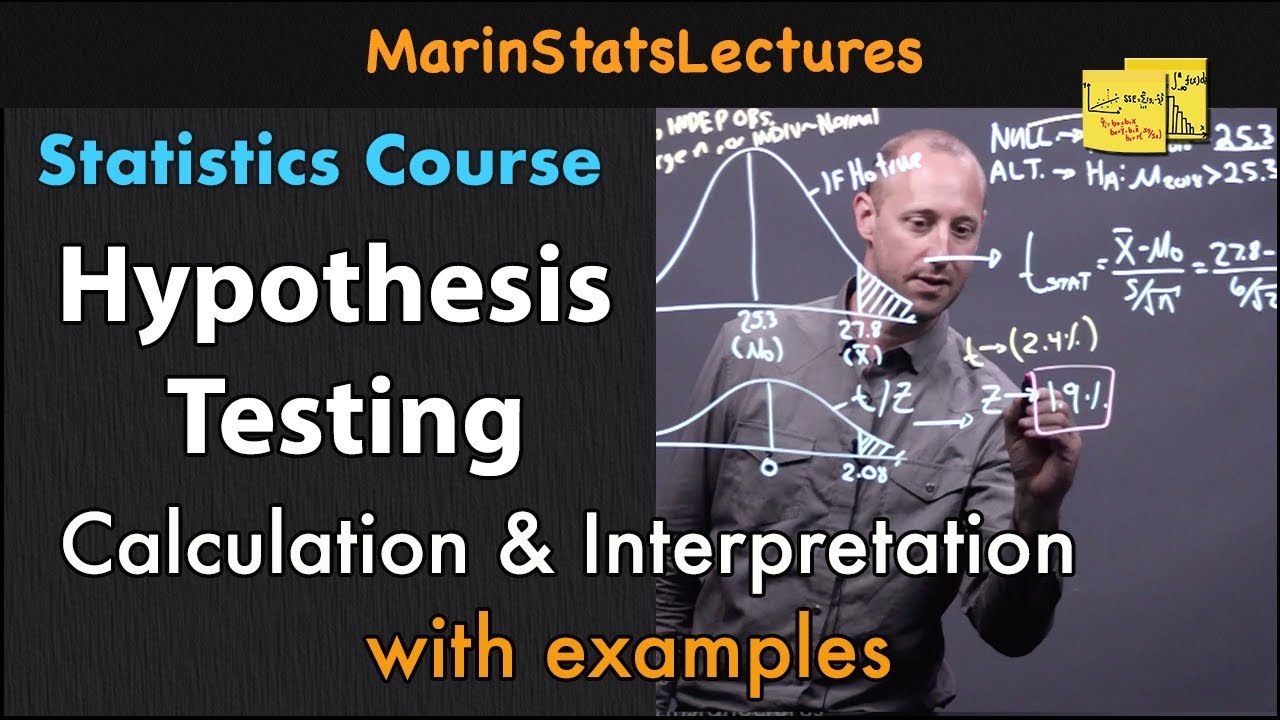
Hypothesis Testing: Calculations and Interpretations| Statistics Tutorial #13 | MarinStatsLectures
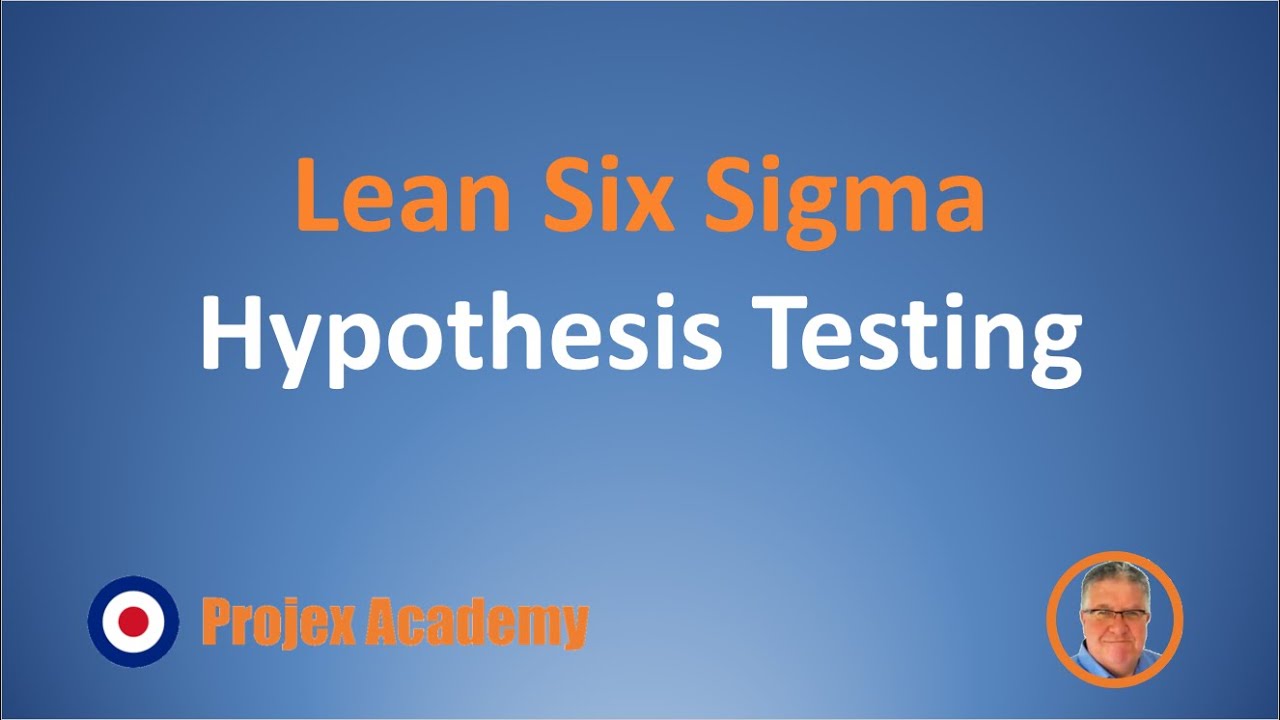
Lean 6 Sigma -- Module 2 Hypothesis Testing

Statistical Inference: Introduction and Terminology (in Hindi)
5.0 / 5 (0 votes)