Principal Component Analysis (PCA) - easy and practical explanation
Summary
TLDRThis video on Principal Component Analysis (PCA) explains how to simplify complex biological data sets by reducing dimensions while preserving essential information. Using PCA, researchers can visualize relationships among multiple variables, such as factors influencing lifespan or gene expression profiles in cancer patients. The first few principal components capture most of the variance, allowing for meaningful interpretation of data trends and clusters. The video emphasizes the importance of loading scores in understanding variable contributions, making PCA a powerful tool for data analysis in biology. Viewers are encouraged to explore PCA further and subscribe for more insights.
Takeaways
- π PCA (Principal Component Analysis) simplifies complex datasets by reducing their dimensions while retaining essential information.
- π It allows researchers to visualize multiple factors without losing critical data, making it easier to interpret biological data.
- π PCA combines original data factors into new variables called principal components, focusing on the most important aspects of the data.
- π Principal components are ranked based on the amount of variance they explain, helping researchers identify key influences on outcomes.
- π A scree plot is used to show how much variance each principal component accounts for, guiding the decision on how many components to retain.
- π₯ PCA helps in clustering similar observations together, revealing patterns in datasets like lifespan or gene expression profiles.
- βοΈ Each original variable contributes differently to principal components, indicated by loading scores that show their relative importance.
- π PCA also illustrates correlations between variables, with positively correlated variables clustering together and negatively correlated ones positioned apart.
- π‘ PCA is especially useful in fields like biology, where datasets can contain hundreds or thousands of variables, such as gene expressions in cancer research.
- π Understanding PCA allows researchers to summarize large datasets effectively, drawing meaningful insights from complex biological information.
Q & A
What is Principal Component Analysis (PCA)?
-PCA is a statistical technique that transforms a dataset with many variables into a smaller number of principal components, which retain most of the original information. It simplifies data interpretation and visualization.
Why is PCA useful in studying biological data?
-PCA helps to reduce the dimensionality of complex biological datasets, allowing researchers to visualize trends, clusters, and outliers without losing significant information.
How does PCA handle datasets with many variables?
-PCA combines multiple variables into new factors called principal components, ranking them from most to least important. This enables researchers to focus on the most influential components for analysis.
What is a scree plot and its significance in PCA?
-A scree plot displays the variance explained by each principal component, helping researchers determine how many components are necessary to retain a significant amount of information from the dataset.
What does it mean if PC1 explains 50% of the variance in a dataset?
-If PC1 explains 50% of the variance, it indicates that this component captures half of the information or variability present in the original data, highlighting its importance in the analysis.
How can PCA reveal relationships between variables?
-PCA shows how variables are correlated through loading scores, which indicate how much each variable contributes to each principal component. Similar variables cluster together, while inversely correlated variables are positioned diagonally in the loading plot.
What can researchers infer from the clustering of points in a PCA plot?
-Clusters of points in a PCA plot suggest that observations (e.g., individuals) share similar profiles based on the variables analyzed. This can indicate patterns related to specific outcomes, such as lifespan or treatment response.
What are loading plots and how are they used in PCA?
-Loading plots visualize the contributions of individual variables to each principal component. They help identify which variables have the most significant impact on the patterns observed in the data.
What is the goal of analyzing PCA results after clustering?
-The goal is to trace back to identify which specific variables or factors differentiate the clusters, helping to draw meaningful biological conclusions from the analysis.
How does PCA facilitate decision-making in biological research?
-By summarizing complex data and revealing trends and clusters, PCA enables researchers to make informed decisions about potential treatments or biological insights, improving the understanding of underlying mechanisms.
Outlines
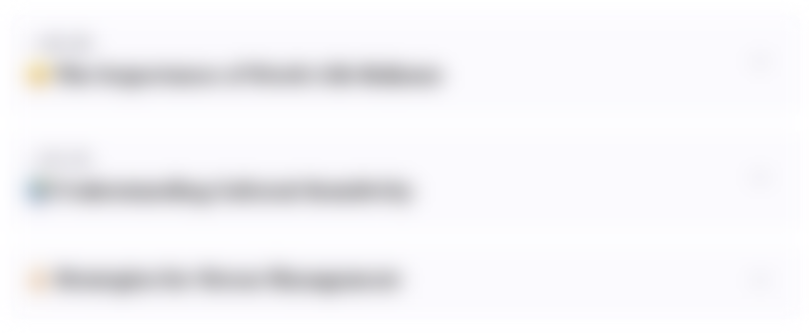
This section is available to paid users only. Please upgrade to access this part.
Upgrade NowMindmap
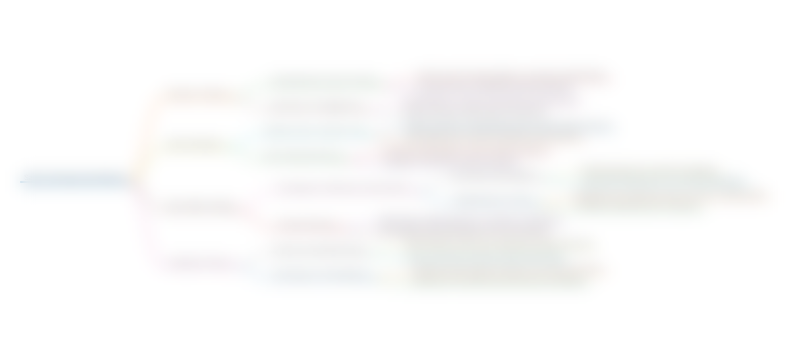
This section is available to paid users only. Please upgrade to access this part.
Upgrade NowKeywords
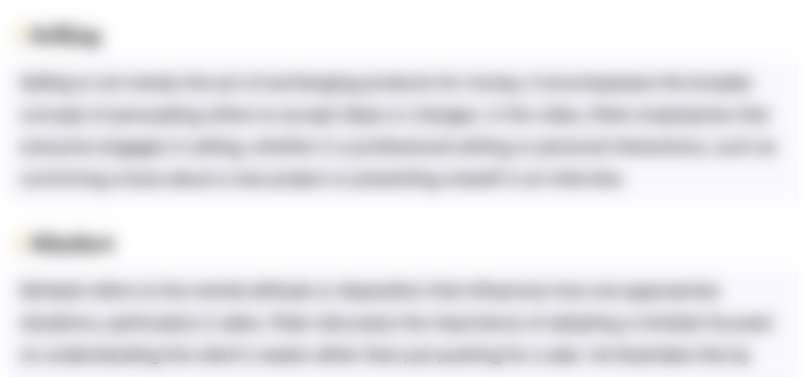
This section is available to paid users only. Please upgrade to access this part.
Upgrade NowHighlights
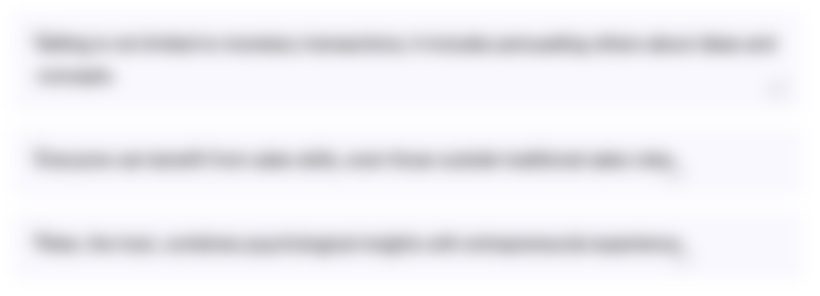
This section is available to paid users only. Please upgrade to access this part.
Upgrade NowTranscripts
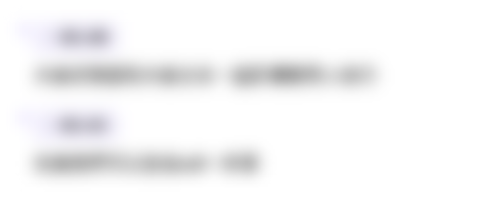
This section is available to paid users only. Please upgrade to access this part.
Upgrade NowBrowse More Related Video
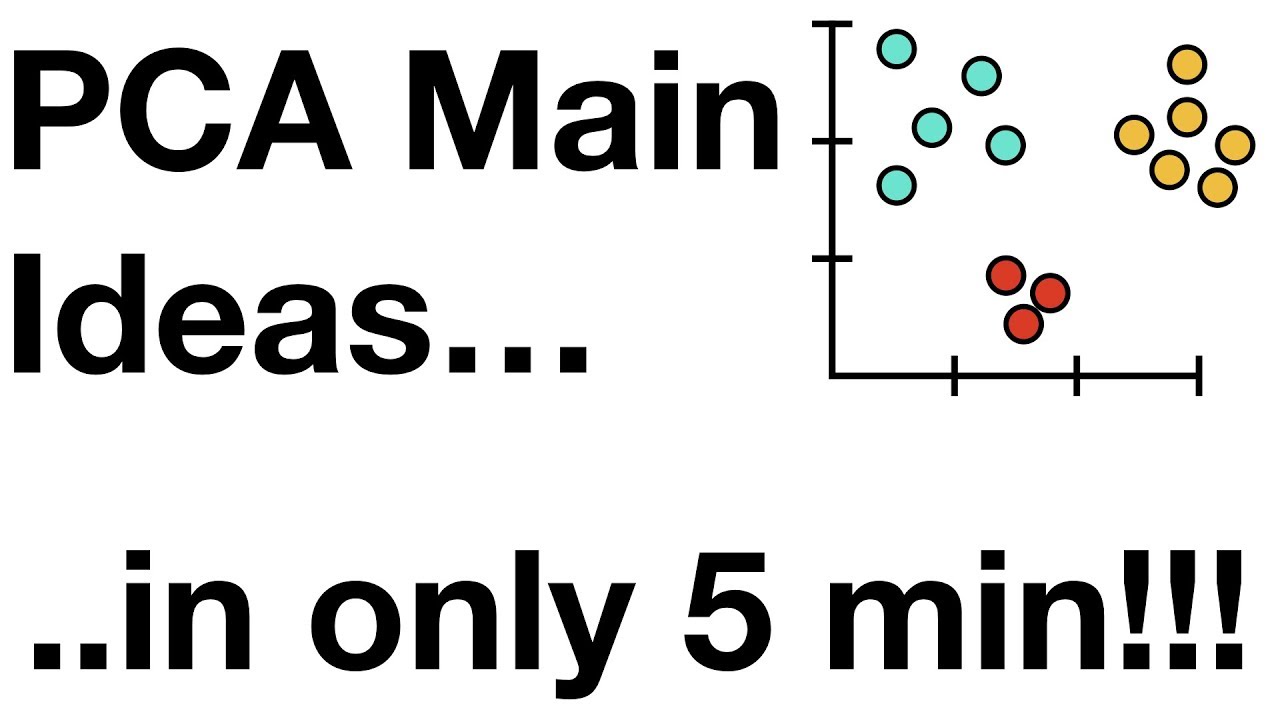
StatQuest: PCA main ideas in only 5 minutes!!!
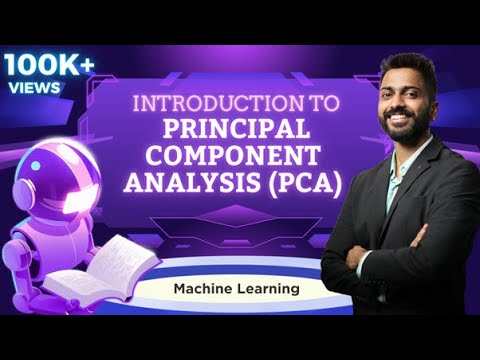
Lec-46: Principal Component Analysis (PCA) Explained | Machine Learning
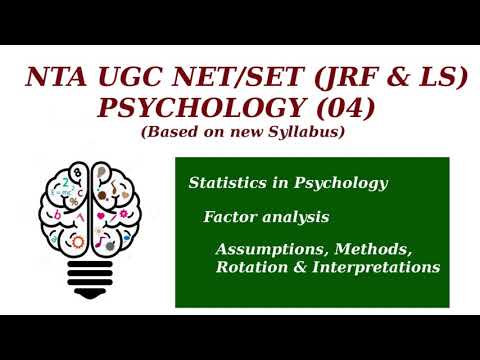
Factor analysis | Statistics in Psychology
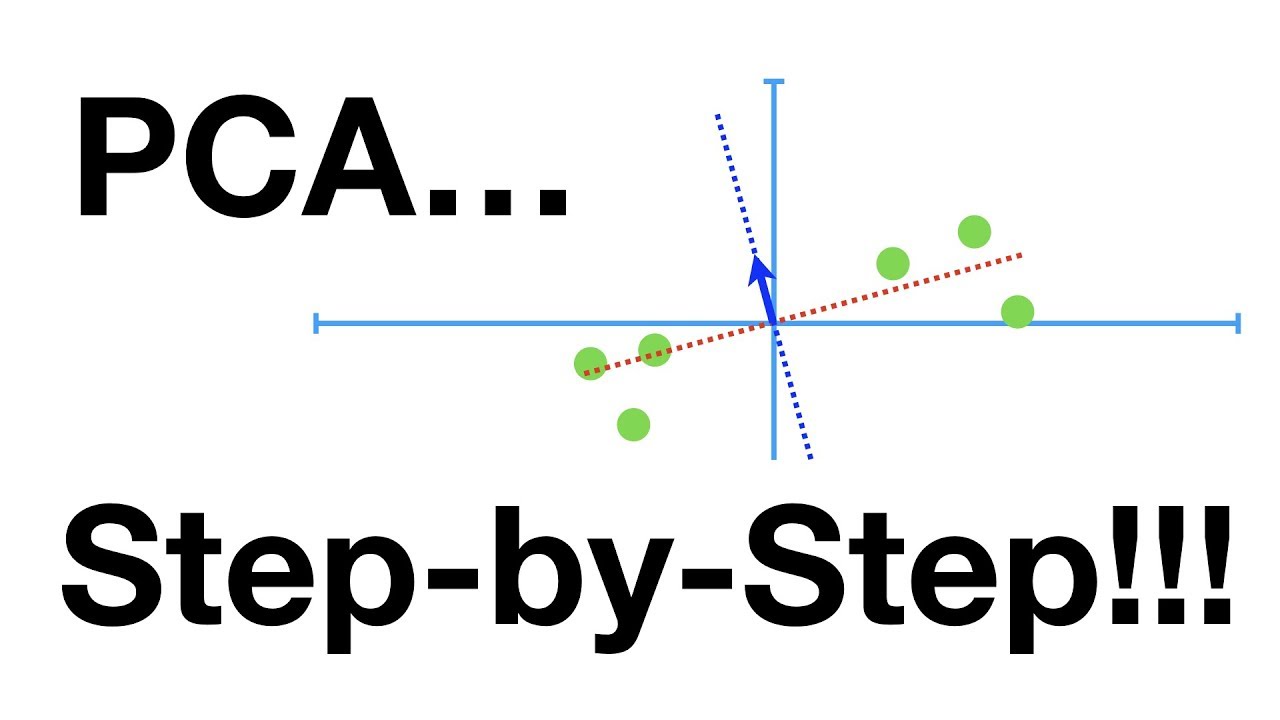
StatQuest: Principal Component Analysis (PCA), Step-by-Step
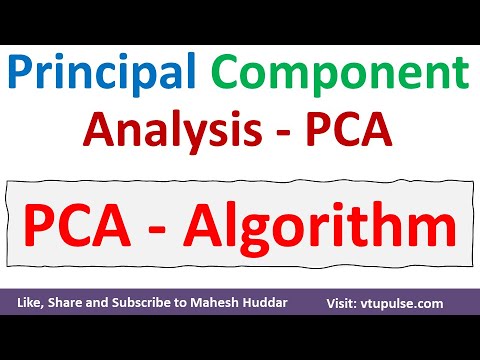
PCA Algorithm | Principal Component Analysis Algorithm | PCA in Machine Learning by Mahesh Huddar
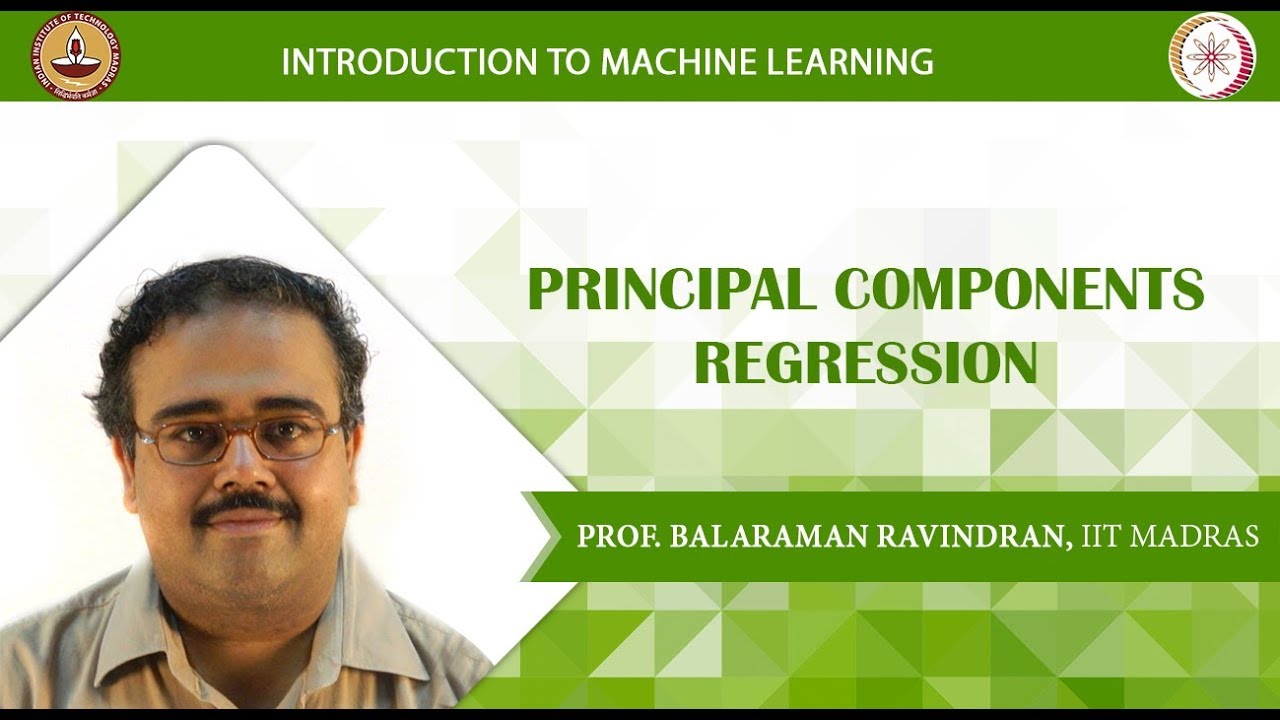
Week 3 Lecture 13 Principal Components Regression
5.0 / 5 (0 votes)