Calculating Power and the Probability of a Type II Error (A One-Tailed Example)
Summary
TLDRThis script discusses the calculation of power and the probability of a type II error, known as beta, using a one-sample Z test for a mean. It explains how to determine the rejection region for the null hypothesis that the population mean is 50, with a significance level of 0.09 and a known population standard deviation of 21. The script also explores how to calculate the probability of a type II error when the true mean is 43 or 40, illustrating the process with visual aids and standard normal distribution tables. It concludes by emphasizing the importance of correctly identifying the areas for power calculations in different scenarios.
Takeaways
- π The script discusses calculating power and the probability of a type II error (beta) in the context of a one-sample Z test on a mean.
- π― The example involves a sample size of 36 from a normally distributed population with a known standard deviation (sigma = 21) and an unknown mean (mu).
- βοΈ The null hypothesis is that the population mean is 50, and a one-sided alternative hypothesis is considered with a significance level (alpha) of 0.09.
- π The Z test statistic is appropriate due to the normal distribution and known sigma, and the rejection region is determined based on the standard normal distribution.
- π The rejection region is initially defined in terms of Z values and then translated into sample mean values (X bar) for power calculations.
- π The script demonstrates how to find the critical value for rejecting the null hypothesis when the true mean is 43, resulting in a rejection region of X bar β€ 45.31.
- π The probability of a type II error is calculated by determining the area under the sampling distribution of X bar to the right of the rejection region, given the true mean is 43.
- π The script shows how to standardize the sample mean to calculate the probability of a type II error and the power of the test.
- π Power is defined as the probability of correctly rejecting a false null hypothesis, which is calculated as 1-beta.
- π The process is reiterated for a different true mean (40) to illustrate how power changes with different population means under the alternative hypothesis.
- π The script emphasizes the importance of correctly identifying the areas under the standard normal curve for accurate power and type II error calculations.
Q & A
What is the significance level (alpha) used in the one-sample Z test example?
-The significance level (alpha) used in the one-sample Z test example is 0.09.
What is the population standard deviation (sigma) in the example?
-The population standard deviation (sigma) is known to be 21.
What is the null hypothesis being tested in the example?
-The null hypothesis being tested is that the population mean (mu) is 50.
What is the alternative hypothesis for the one-sample Z test?
-The alternative hypothesis is that the population mean (mu) is less than 50.
What is the Z value that corresponds to the alpha level of 0.09 in the standard normal distribution?
-The Z value with an area of 0.09 to the left is approximately -1.34.
What is the rejection region for the null hypothesis in terms of the sample mean (X bar)?
-The rejection region for the null hypothesis in terms of the sample mean (X bar) is less than or equal to 45.31.
What is the probability of committing a type I error in this scenario?
-The probability of committing a type I error, which is the probability of rejecting the null hypothesis when it is true, is 0.09.
If the true population mean is 43, what is the probability of a type II error?
-If the true population mean is 43, the probability of a type II error (beta) is approximately 0.255.
What is the power of the test when the true population mean is 43?
-The power of the test when the true population mean is 43 is 1 - beta, which is approximately 0.745.
If the true population mean is 40, what is the power of the test?
-If the true population mean is 40, the power of the test is the probability that the sample mean (X bar) is less than or equal to 45.31, which is approximately 0.935.
How is the power of a test defined in the context of hypothesis testing?
-The power of a test is defined as the probability of rejecting the null hypothesis when it is false, which is equivalent to 1 - beta.
Outlines
π Calculating Power and Type II Error in a One-Sample Z Test
This paragraph discusses a method for calculating the power of a statistical test and the probability of a type II error, known as beta. The scenario involves a one-sample Z test for a mean from a normally distributed population with a known standard deviation (sigma = 21) but an unknown mean (mu). The null hypothesis is that the population mean is 50, and a one-sided test is conducted at a significance level (alpha) of 0.09. The Z test statistic is used, and it is shown how to determine the critical Z value for rejecting the null hypothesis. The rejection region is then translated into a sample mean (X bar) value, which is less than or equal to 45.31. The concept of type I error is introduced as the probability of rejecting the null hypothesis when it is true. An example is given where the true mean is 43, and the probability of a type II error is calculated by determining the probability that the sample mean is greater than 45.31.
π Visualizing Type II Error and Power Calculations
The second paragraph continues the discussion on type II errors and introduces power calculations. It visually contrasts the sampling distribution of the sample mean (X bar) under the null hypothesis with the distribution when the true mean is 43. The probability of a type II error is shaded in red, representing the error of not rejecting a false null hypothesis. The calculation involves standardizing the sample mean to a Z-score and finding the probability that Z is greater than 0.66, which corresponds to a type II error probability of approximately 0.255 or beta. The power of the test, which is the probability of correctly rejecting a false null hypothesis, is then calculated as 1 - beta, resulting in a power of 0.745. The paragraph also briefly mentions how power calculations would differ if the true mean were different, such as 40, and how the visual representation of the sampling distribution would change accordingly.
π Power Calculation with a Different True Mean
The final paragraph explores another power calculation with a different true mean of the population (mu = 40). It explains how to calculate the power of the test when the null hypothesis is false. The power is defined as the probability of rejecting the null hypothesis when it is indeed false, which in this case is when the true mean is 40. The paragraph describes the visual representation of the sampling distribution of X bar under the null hypothesis and when the true mean is 40. It shows how to calculate the probability of obtaining a sample mean less than or equal to 45.31, which is the rejection region, and standardizes this to a Z-score. The probability that Z is less than or equal to 1.517 is found using the standard normal curve, which corresponds to a power of approximately 0.935. The paragraph concludes by emphasizing the importance of correctly identifying the required areas for power calculations depending on the alternative hypothesis.
Mindmap
Keywords
π‘Power of a test
π‘Type II error
π‘Z test statistic
π‘Significance level (alpha)
π‘Null hypothesis
π‘Alternative hypothesis
π‘Population mean (mu)
π‘Population standard deviation (sigma)
π‘Sample mean (X bar)
π‘Rejection region
Highlights
Calculating power and the probability of a type II error, often called beta, using a one-sample Z test on a mean.
Randomly sampling 36 values from a normally distributed population with a known standard deviation of 21 and an unknown population mean.
Testing the null hypothesis that the population mean is 50 against a one-sided alternative hypothesis.
Setting a significance level of 0.09 for the test.
Using the Z test statistic for a normally distributed population with a known standard deviation.
Determining the rejection region for the null hypothesis based on the Z value corresponding to an alpha level of 0.09.
Rejection of the null hypothesis occurs if the Z value is less than or equal to -1.34.
Reexpressing the rejection region in terms of the sample mean for power calculations.
Rejecting the null hypothesis if the sample mean is less than or equal to 45.31.
Visual representation of the sampling distribution of the sample mean under the null hypothesis.
Calculating the probability of a type II error when the true population mean is 43.
The probability of a type II error is the probability of not rejecting the null hypothesis when it is false.
Visualizing the sampling distribution of the sample mean when the true mean is 43 and identifying the type II error region.
Standardizing the sample mean to calculate the probability of a type II error.
The probability of a type II error when the true mean is 43 is approximately 0.255.
The power of a test is the probability of rejecting the null hypothesis when it is false, which is 1-beta.
Calculating the power of the test when the true population mean is 40.
Visual representation of the sampling distribution of the sample mean when the true mean is 40 and calculating power.
The power of the test when the true mean is 40 is approximately 0.935.
The same logical arguments apply to other alternatives, such as the mean being greater than or not equal to the hypothesized value.
Transcripts
Let's look at an example of calculating power
and the probability of a type II error, which we often called beta.
This example is done in the setting of a one-sample Z test on a mean
but similar logic holds for other types of tests.
Suppose we are about to randomly sample 36 values from a normally distributed population,
where the population standard deviation sigma is known to be 21,
but the population mean mu is unknown.
And we wish to test the null hypothesis that the population mean is 50
against this one-sided alternative hypothesis
and we feel that a significance level of 0.09 is appropriate for our test.
Since we are sampling from a normally distributed population,
and the population standard deviation sigma is known
then the appropriate test statistic is this Z test statistic.
If the null hypothesis is true, this Z test statistic will have the standard normal distribution.
And the first question, is for what values of Z will we reject the null hypothesis?
Here I've plotted out the standard normal curve.
Our alpha level is 0.09 and our alternative hypothesis is that mu is less than 50,
so we're going to put the entire alpha level in the left side of the distribution.
If we go to software or the standard normal table
we can find that the Z value with an area of 0.09 to the left is, to 2 decimal places, -1.34.
And so we are going to reject the null hypothesis
If the Z value that we get in our sample is less than or equal to -1.34.
Now we're going to reexpress the rejection region in terms of the sample mean
because that's going to help with our power calculations later on.
So, for what values of X bar will we reject the null hypothesis?
Here's our information from before,
and we said that we are going to reject the null hypothesis
if the observed value of Z is less than or equal to -1.34.
Well this this is our Z test statistic here, and we can isolate X bar,
just multiply by sigma over the square root of n and add mu_0.
and we get that X bar is equal to mu_0 plus sigma over the square root of n times Z.
Well in this case
that's going to be 50 plus 21 over the square root of 36 times -1.34,
and this works out to 45.31.
So we had this rejection region expressed in terms of Z before,
but now we can express it in terms of X bar.
We're going to reject the null hypothesis if X bar is less than or equal to 45.31.
Visually that looks like this.
I've plotted out the sampling distribution of X bar if the null hypothesis is in fact true.
And we are going to reject the null hypothesis
if the value of our sample mean is less than or equal to 45.31.
And this area here is the given alpha level of 0.09.
And that is the probability that we reject the null hypothesis if it is in fact true.
Or in other words, the probability of committing a type I error when the null hypothesis is actually true.
If the true value of the population mean is 43, what is the probability we commit a type II error?
If you recall, a type II error is not rejecting the null hypothesis when it is false.
Our null hypothesis here is that mu is 50,
and our null hypothesis is in fact false
because we're saying the true mean is 43.
So the probability of a type II error in this setting
is the probability that we do not reject the null hypothesis if mu is actually 43.
If you recall, we reject the null hypothesis if X bar is less than or equal to 45.31,
and so we're not going to reject the null hypothesis when it's greater than that
and so the probability of a type II error is going to be
the probability that X bar takes on a value bigger than 45.31,
given the true value of mu is actually 43.
Let's see what that looks like visually.
In white I have the sampling distribution of X bar if the hypothesized value is correct.
And in blue I have the sampling distribution of X bar if mu is actually 43
which we're assuming it to be for this question.
So this distribution in blue is centered here at 43.
We are rejecting the null hypothesis
if the sample mean is less than or equal to 45.31
and we're going to not reject the null hypothesis if it's greater than 45.31,
so the probability of a type II error in this spot
is going to be the area to the right of this value, given mu is actually 43.
Let's shade that area in.
I'm shading that area in red here because it's an error.
It is the probability of committing a type II error if the true value of mu is actually 43 ,
the probability of not rejecting the null hypothesis when it is wrong.
To find that area, we want to find the probability that X bar takes on a value bigger than 45.31,
given the true value of mu is 43.
X bar in this setting is a normally distributed random variable
and we're going to standardize this in the usual way.
And we standardize this by saying that
Z is equal to X bar minus the true value of mu over sigma over the square root of n.
So to do this probability calculation over here we're simply going to standardize it.
We're going to say that this is equal to
the probability that Z takes on a value that's bigger
than 45.31 minus the true value of mu, which is 43,
divided by sigma, which you may recall was 21, that was given earlier.
And our sample size n was 36.
And so this is going to be the probability
the random variables Z takes on a value that's bigger than 0.66.
And if we go to the standard normal curve,
we can see that the area out to the right of 0.66 is approximately 0.255.
And so our probability of a type II error in this spot is approximately 0.255.
And we call that beta, the probability of a type II error.
The power of a test is the probability of rejecting the null hypothesis when it is false.
And that's simply going to be 1-beta.
And in this setting that's 1-0.255, or 0.745.
Let's take a look at another example of a power calculation.
Suppose this time mu is actually 40,
so the null hypothesis is still wrong but the population mean is now 40.
What is the power of the test?
What is the probability of rejecting the null hypothesis,
given that it is false, as it is in this scenario.
So here our power is the probability of rejecting the null hypothesis given the true value of mu is 40,
and recall that we're rejecting the null hypothesis
when X bar is less than or equal to 45.31
so we could express power in terms of the probability of a type 2 error, but we don't have to.
Its easier typically to just leave it in this fashion.
Power is the probability of rejecting the null hypothesis
and we're rejecting the null hypothesis when X bar is less than or equal to 45.31,
given the true value of mu is actually 40.
Let's see what that looks like visually.
Here again I've plotted out the sampling distribution of X bar if the null hypothesis is true,
and the sampling distribution of X bar when mu is actually 40, which is our current situation.
So the sampling distribution of X bar now is centered over here at 40.
We are rejecting the null hypothesis if we get a sample mean that's less than or equal to 45.31,
so let's shade that area in.
I've shaded this area in green this time because this is the power,
and power is the probability of rejecting the null hypothesis when it's false,
and that's a good thing.
And so here power is going to be
the probability that we get a sample mean that's less than or equal to 45.31,
given the true value of mu is actually 40.
And we're going to standardize this again in the usual way,
and say that Z is equal to X bar minus the true mean
over sigma over the square root of n
and come down here and say this is going to be equal to the probability
that Z takes on a value less than or equal to 45.31 minus the true mean, 40,
over sigma which was 21
and the square root of n and n was 36.
This is going to be equal to the probability
that a random variables Z takes on a value that's less than or equal to 1.517.
And if we go to our standard normal curve
we can find that the area out to the left of 1.517 is approximately 0.935,
and that is the power of the test in this situation.
Here we did all of the power calculations
under the alternative hypothesis that mu is actually less than the hypothesized value.
The same logical arguments hold for other alternatives
like it's greater than the hypothesized value or not equal to, but the required areas will change.
So when faced with one of these power calculations,
it's important to think through it and make sure you're coming up with the correct areas.
Browse More Related Video
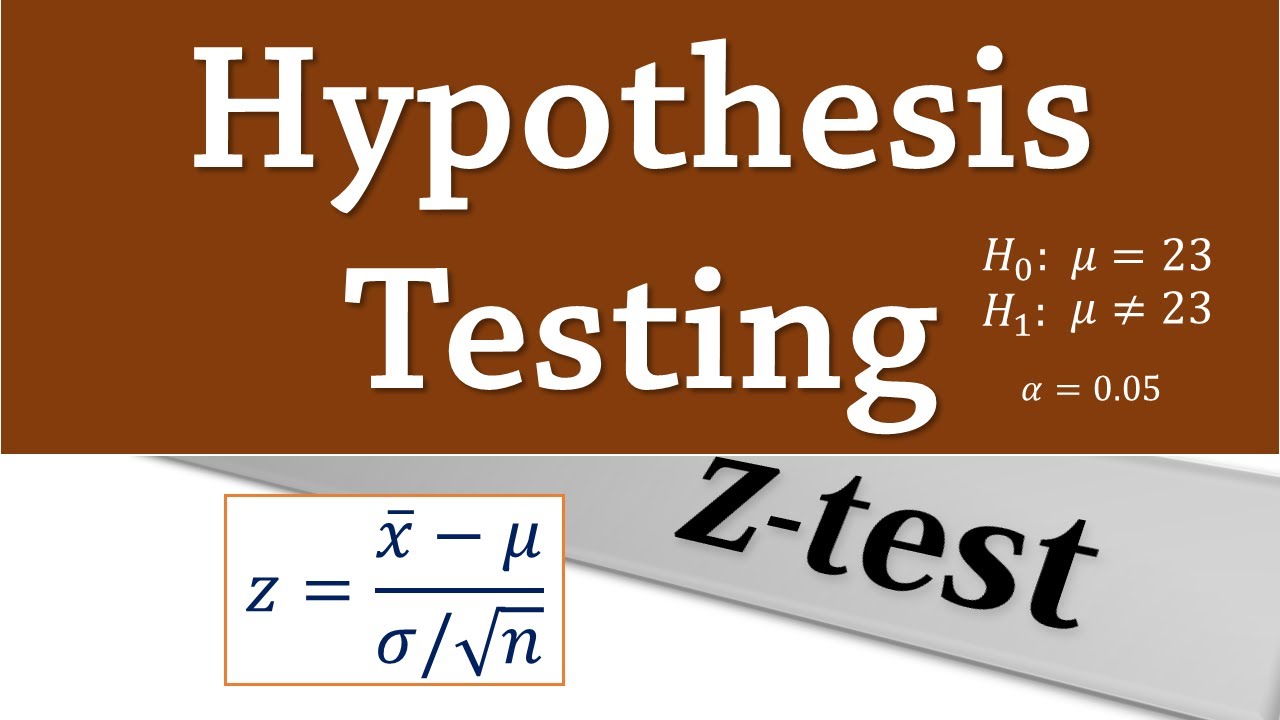
Hypothesis Testing: Two-tailed z test for mean
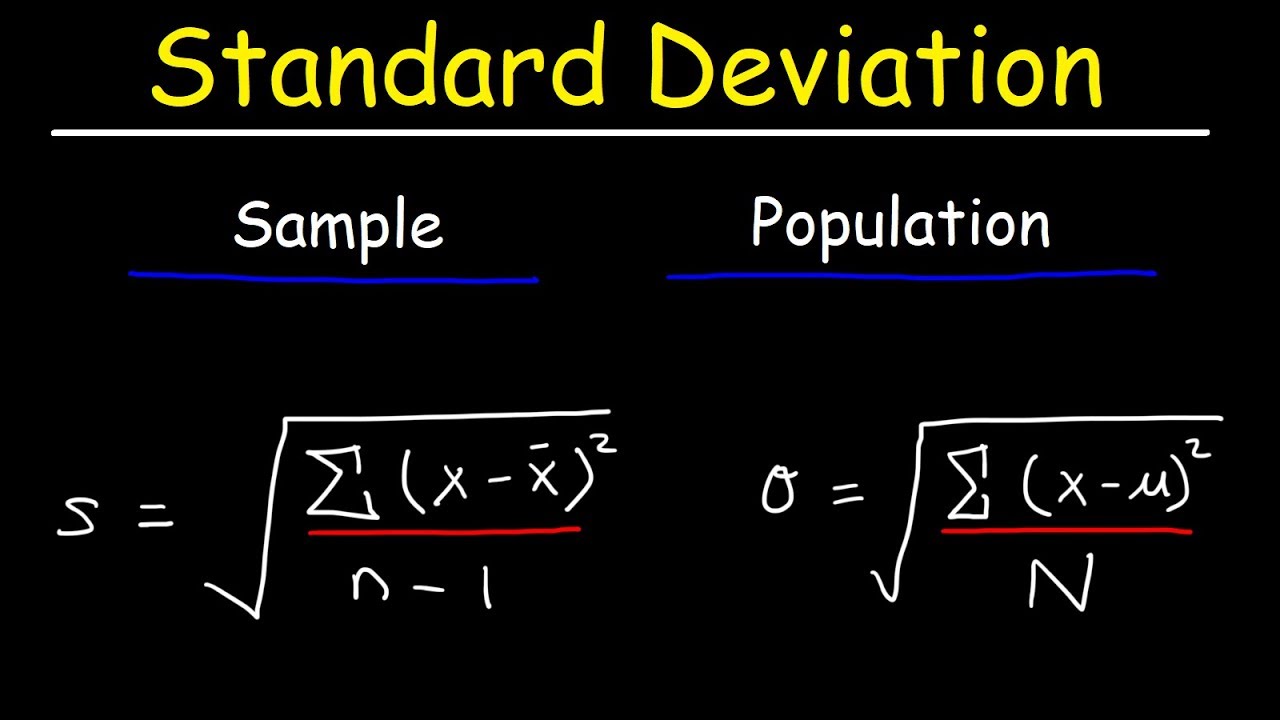
Standard Deviation Formula, Statistics, Variance, Sample and Population Mean
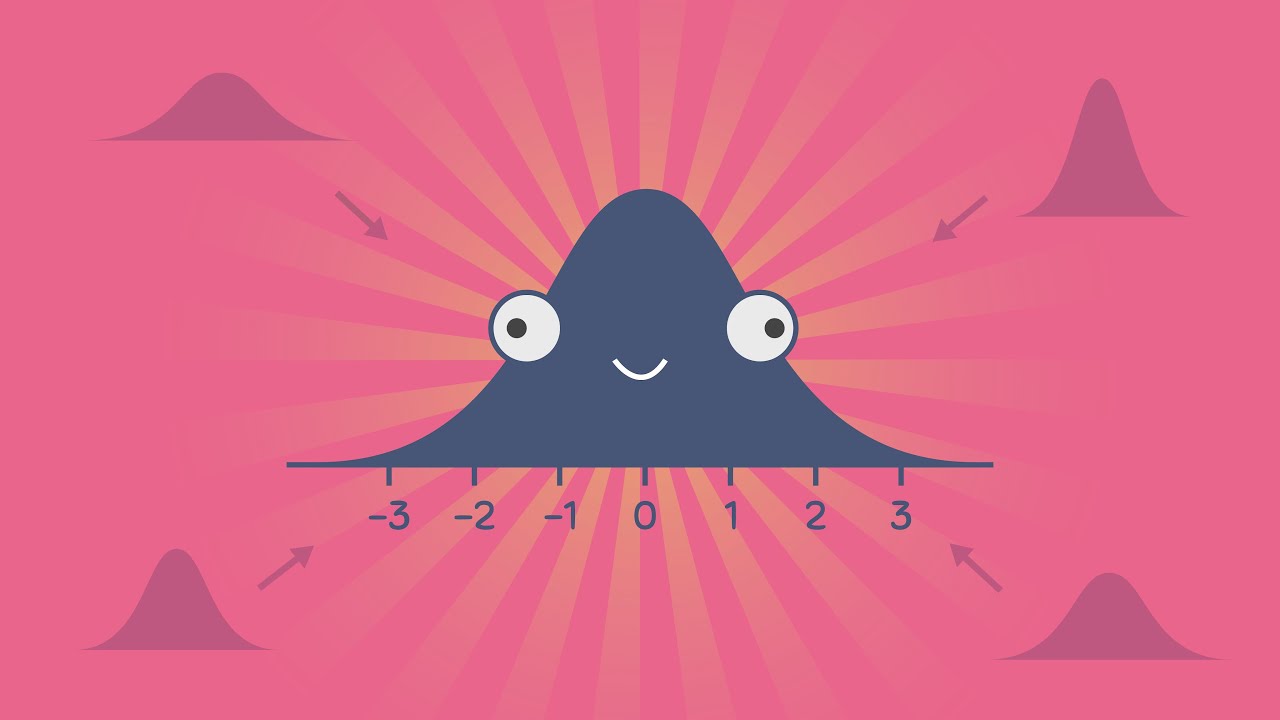
Z-Scores, Standardization, and the Standard Normal Distribution (5.3)
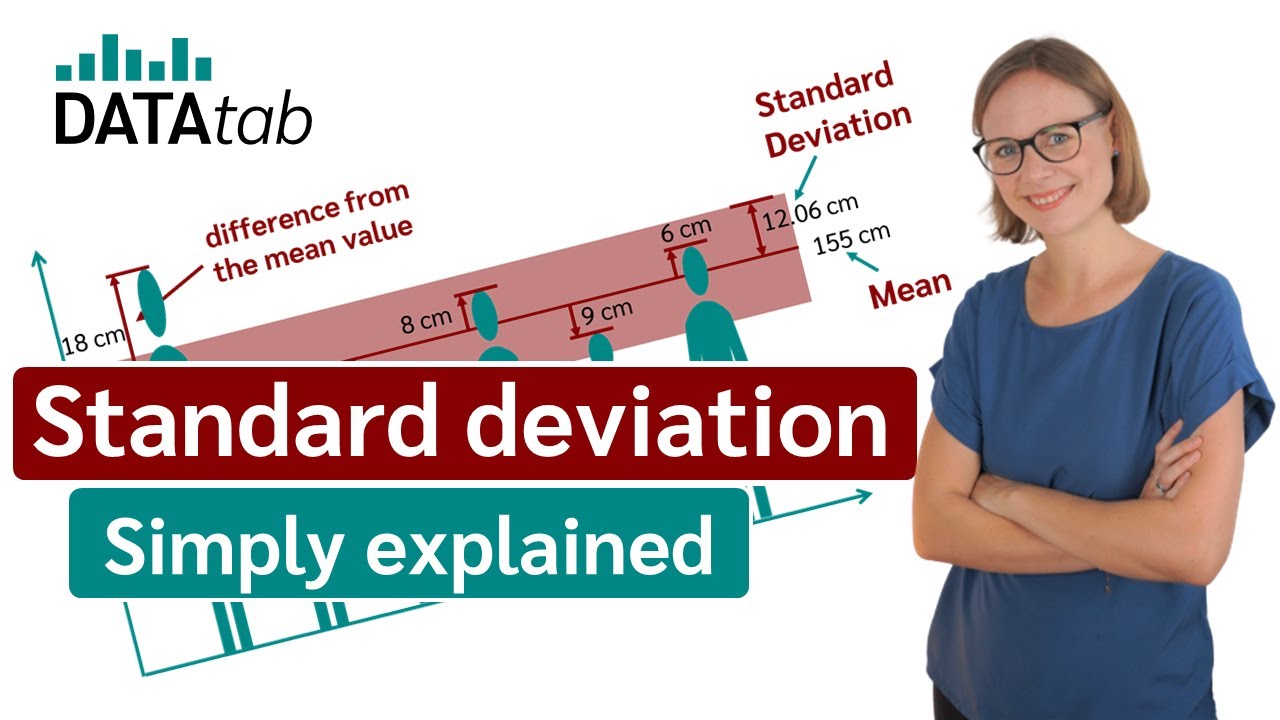
Standard deviation (simply explained)
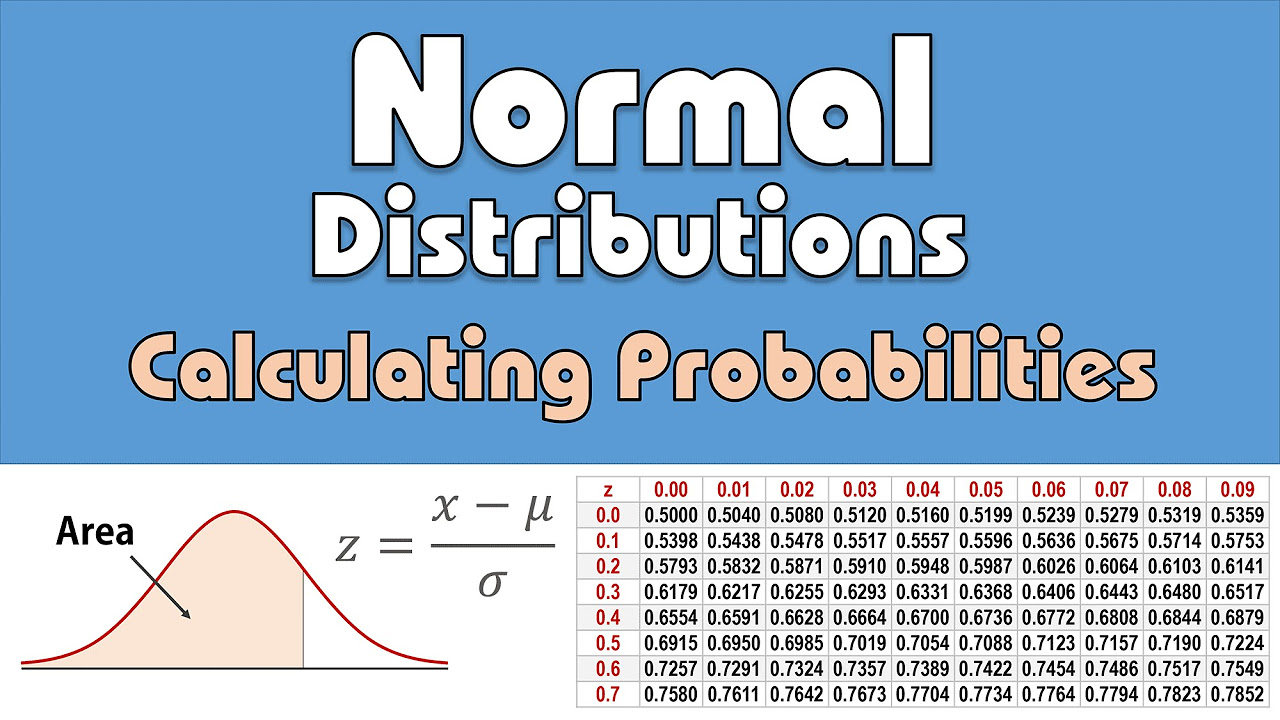
Normal Distribution: Calculating Probabilities/Areas (z-table)
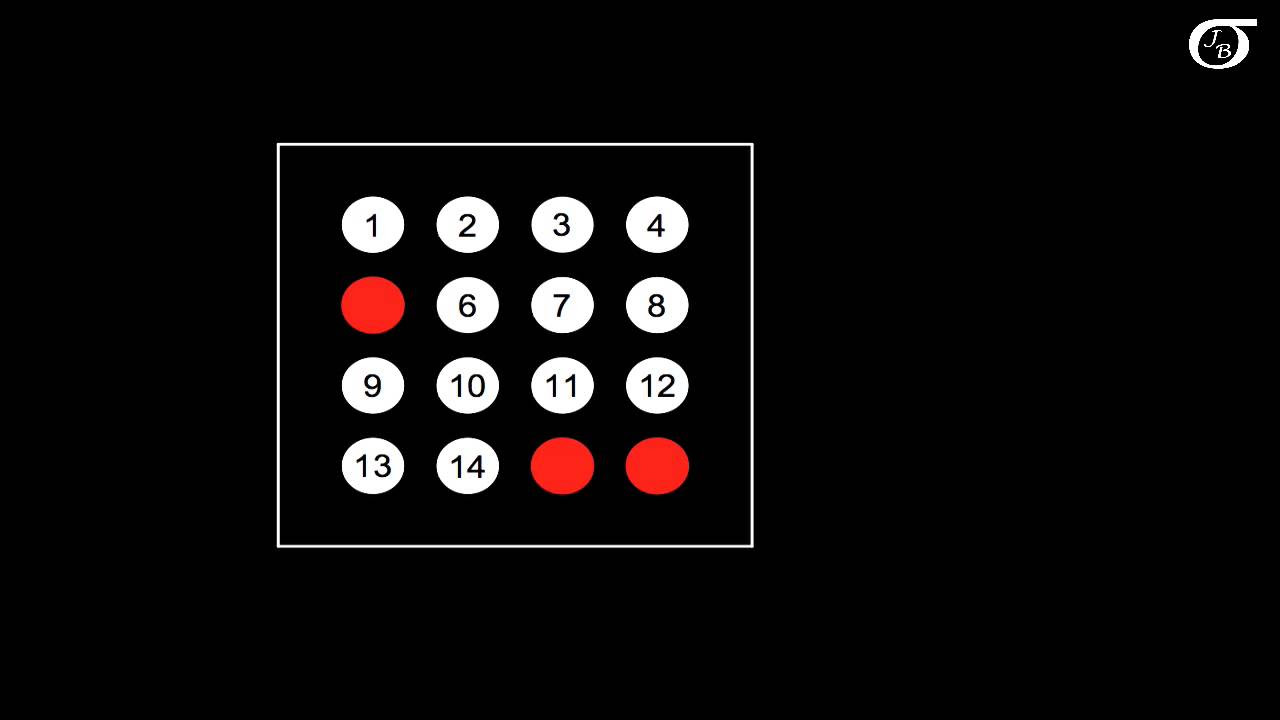
Sampling Distributions: Introduction to the Concept
5.0 / 5 (0 votes)