AI isn't gonna keep improving
Summary
TLDRThe video script discusses the concept of hitting an 'AI Plateau,' suggesting that despite advancements in AI models like ChatGPT and Claude, we may be nearing a limit in performance improvements due to physical constraints, similar to the end of Moore's Law. It explores the idea that future progress in AI might not come from incremental improvements but from entirely new architectures and approaches. The script also touches on the environmental impact of AI and the need for a shift in focus towards more general AI methods that can scale effectively.
Takeaways
- π The AI industry might be reaching a plateau in terms of performance improvements, despite the rapid advancements in AI models like GPT-2 to GPT-4 and the emergence of open-source models.
- π The script references Moore's Law, which predicted the doubling of transistors on a microchip every two years, but acknowledges that this trend has slowed due to physical limitations in manufacturing processes.
- π The manufacturing complexity for advanced chips is so high that only a few companies like TSMC can produce them, leading to a concentration in the industry and affecting the pace of innovation.
- π A study suggests that the performance growth of processors has flattened, with the rate of improvement decreasing significantly from the past decades.
- π‘ The script discusses the potential of different architectures, like Apple's approach with specialized cores for efficiency and performance, as a way to circumvent the limitations of traditional CPUs.
- π― There's a call for a shift in focus from just increasing compute power to developing more general AI methods that can scale effectively, such as search and learning algorithms.
- π The environmental impact and cost of AI research, which often requires massive amounts of compute resources, are becoming concerns as the field matures.
- π Despite the plateau in performance, there's potential for AI to grow in new areas, like video generation, that are not yet fully explored and could provide the next wave of advancements.
- π The script highlights the need for new benchmarks and methods to measure AI progress, as current models struggle with tasks that require general intelligence and adaptability.
- π§ The future of AI may lie in hybrid models that combine the strengths of both handcrafted algorithms and AI, moving beyond the limitations of large language models (LLMs).
Q & A
What is the AI Plateau mentioned in the script?
-The AI Plateau refers to the perceived slowing down of significant advancements in AI capabilities, despite the continuous release of new models. It suggests that we might be reaching a limit in how much AI can improve within the current paradigm.
What is Moore's Law and how does it relate to the script's discussion on AI?
-Moore's Law is the observation that the number of transistors on a microchip doubles approximately every two years, leading to increased performance at a lower cost. The script discusses how this law has seemingly plateaued in recent years, which parallels the perceived plateau in AI improvements.
What is the significance of the flatlining in the performance graph presented in the script?
-The flatlining in the performance graph indicates that the rate of improvement in AI performance has slowed down, suggesting that we may be reaching a physical or theoretical limit in how much further AI can advance using current technologies and methods.
What does the script suggest about the future of AI if we continue to rely on increasing compute power?
-The script suggests that relying solely on increasing compute power may not yield the same exponential improvements in AI as it has in the past. Instead, it implies that innovative architectural changes and new methods will be necessary to continue AI's advancement.
What is the script's view on the comparison between CPUs and AI models in terms of hitting a plateau?
-The script draws a parallel between CPUs, which have seen a slowdown in performance improvements due to physical limitations, and AI models, which seem to be reaching a similar plateau in terms of the improvements seen with each new generation.
What is the role of TSMC in the context of chip manufacturing as discussed in the script?
-TSMC (Taiwan Semiconductor Manufacturing Company) is highlighted as one of the few companies capable of manufacturing chips at the smallest, most efficient scales. The script notes that reliance on TSMC by major tech companies signifies a bottleneck in innovation due to the complexity of these manufacturing processes.
What does the script imply about the relationship between the number of transistors and AI performance?
-The script implies that historically, an increase in the number of transistors has been a measure of progress in processors and by extension, AI performance. However, it suggests that this measure may no longer be as indicative of progress due to the plateau in AI improvements.
What alternative to Moore's Law is proposed in the script, and how does it compare?
-The script mentions an alternative law proposed in a study that attempts to account for theζΎηΌ in performance gains. This alternative law is shown as a flatter line compared to Moore's Law, indicating a slower rate of improvement in recent years.
How does the script discuss the potential of different architectures for AI advancement?
-The script suggests that different architectures, such as those being researched by companies like IBM for analog AI chips, could potentially offer new paths for AI advancement that bypass the limitations of current models and hardware.
What does the script suggest about the future of AI research and development?
-The script suggests that the future of AI research and development may need to focus on creating and iterating on entirely new architectures and methods, rather than simply increasing the compute power or refining existing models.
What is the significance of the ARC AI prize mentioned in the script?
-The ARC AI prize is significant as it represents a public competition aimed at encouraging new ideas and solutions in AI that can efficiently acquire new skills and solve open-ended problems, which the script argues is a more accurate representation of general intelligence.
Outlines
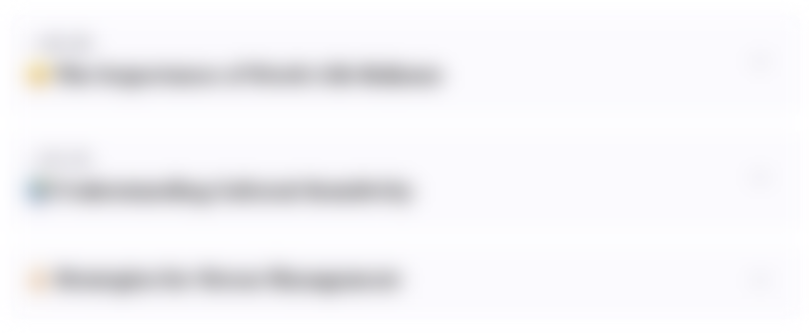
This section is available to paid users only. Please upgrade to access this part.
Upgrade NowMindmap
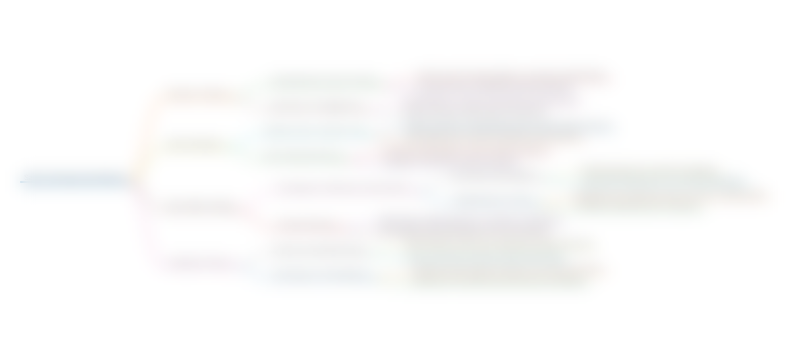
This section is available to paid users only. Please upgrade to access this part.
Upgrade NowKeywords
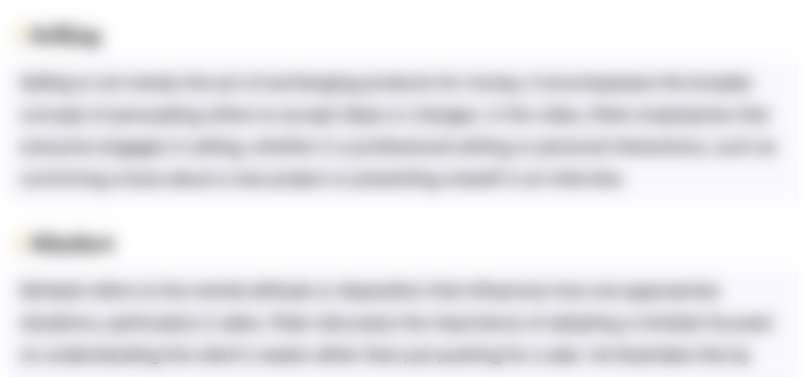
This section is available to paid users only. Please upgrade to access this part.
Upgrade NowHighlights
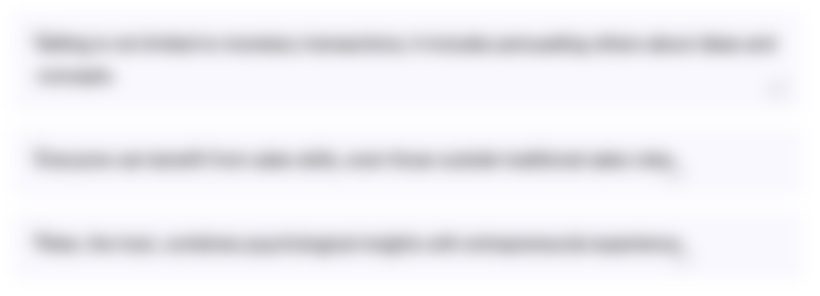
This section is available to paid users only. Please upgrade to access this part.
Upgrade NowTranscripts
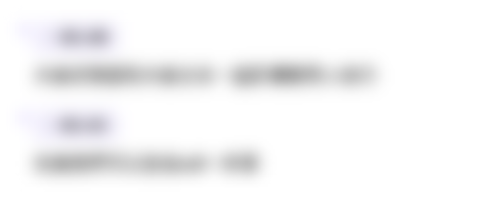
This section is available to paid users only. Please upgrade to access this part.
Upgrade NowBrowse More Related Video
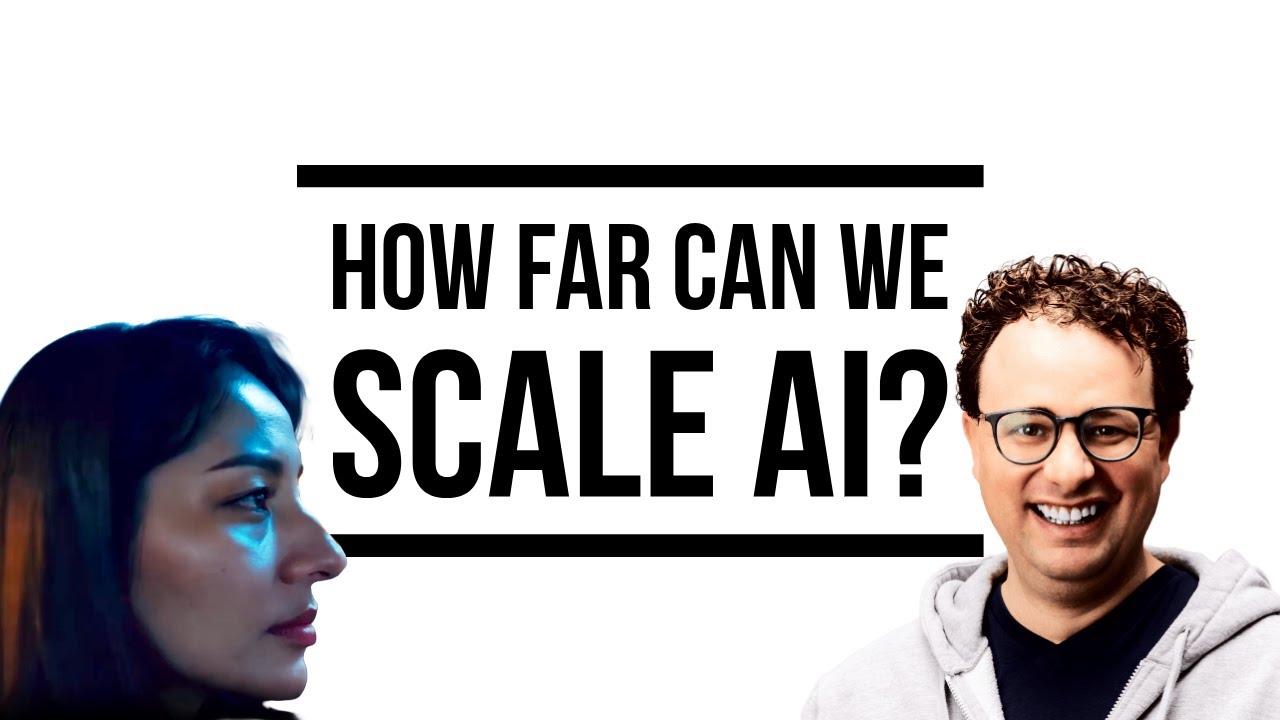
How Far Can We Scale AI? Gen 3, Claude 3.5 Sonnet and AI Hype
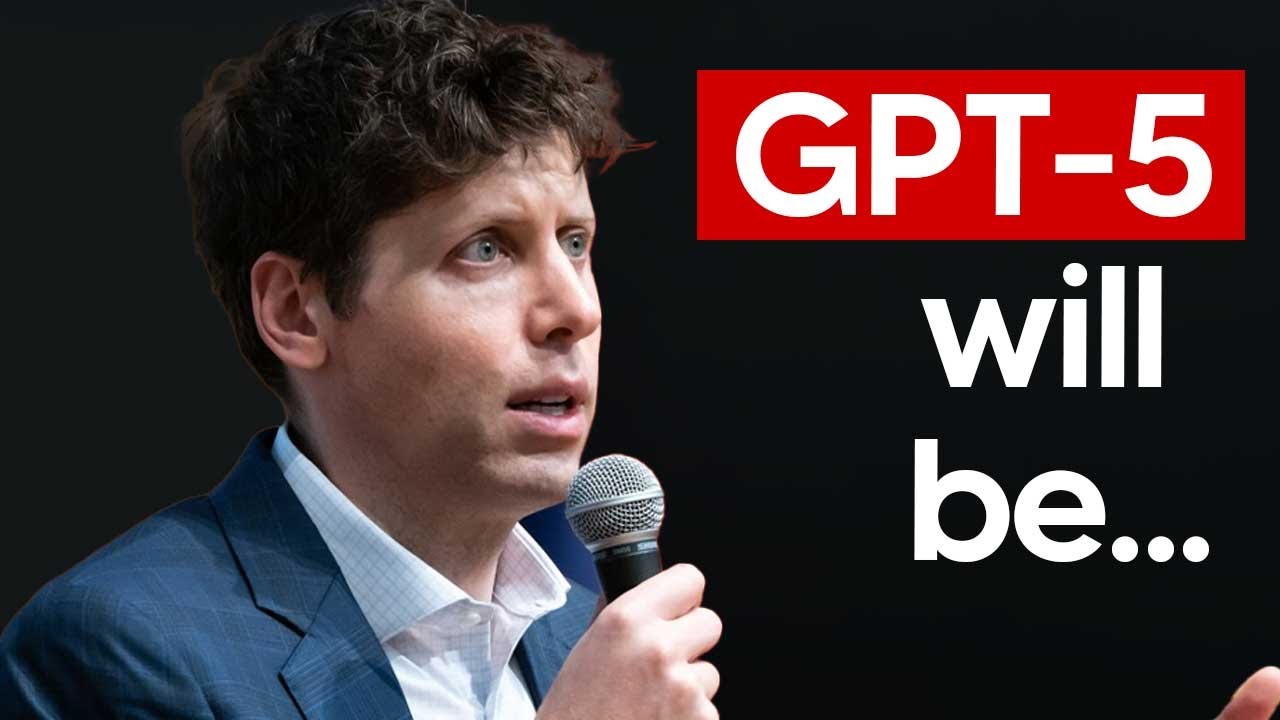
The AI Hype is OVER! Have LLMs Peaked?
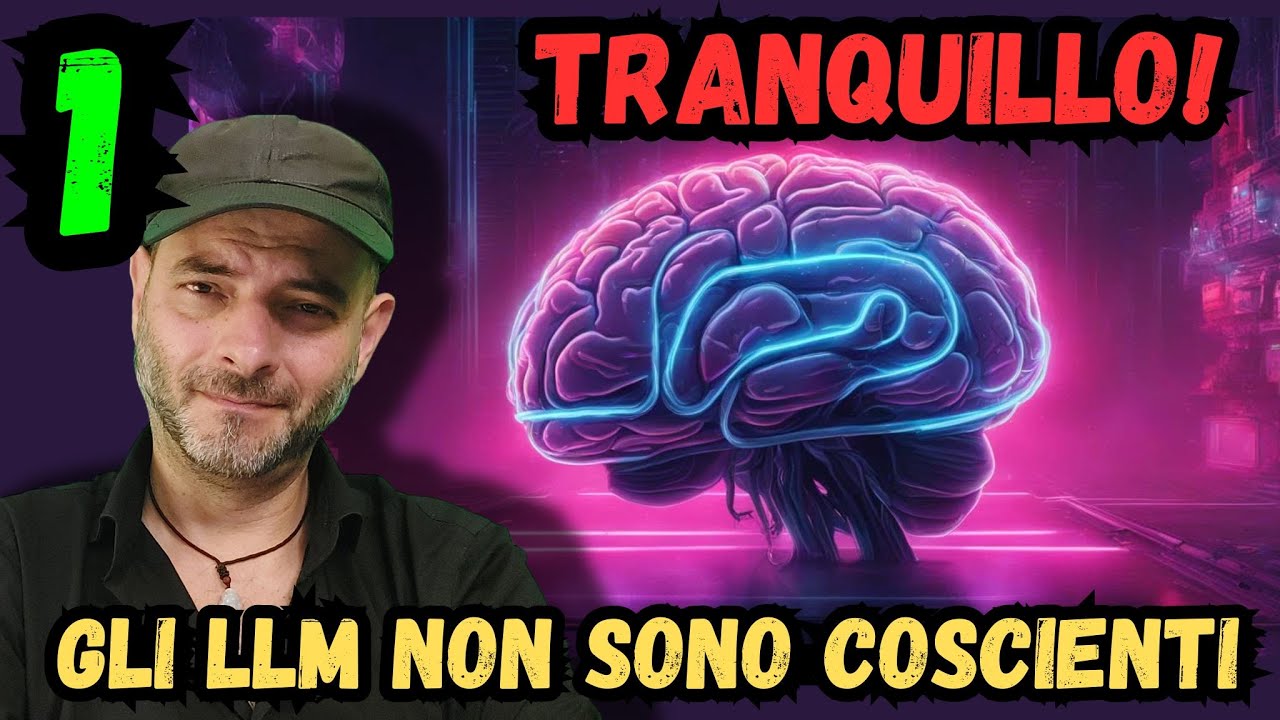
NO: GPT, Claude e gli altri NON SONO COSCIENTI. Propongo una soluzione.

So Google's Research Just Exposed OpenAI's Secrets (OpenAI o1-Exposed)
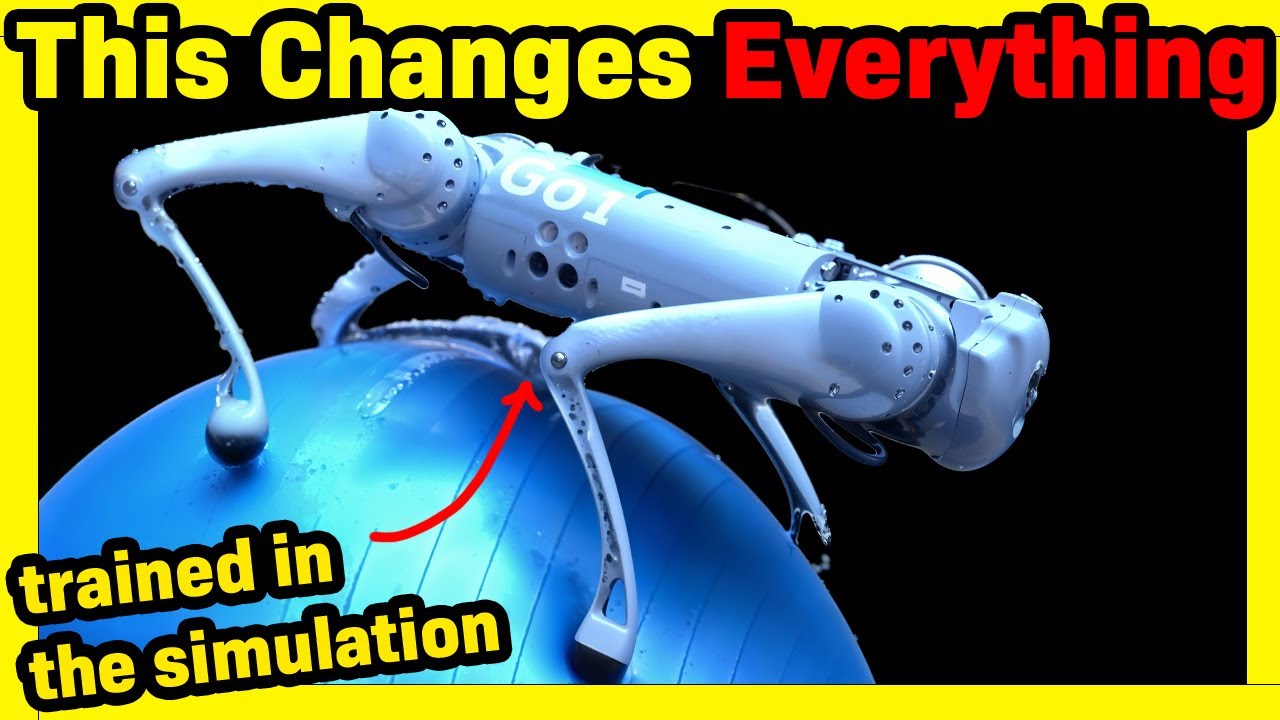
SHOCKING Robots EVOLVE in the SIMULATION plus OpenAI Leadership Just... LEAVES?

The Biggest AI Company in the World...
5.0 / 5 (0 votes)