The AI Hype is OVER! Have LLMs Peaked?
Summary
TLDRThe video script discusses the current perception of AI, particularly generative AI and large language models (LLMs), and counters the notion that AI hype is fading. It references the Gartner Hype Cycle to illustrate the stages of technological maturity and adoption, arguing that while some believe we are at a plateau, significant advancements are still to come. The speaker highlights key developments such as OpenAI's Sora, advancements in voice recognition, and the potential for AI to exceed human performance in complex tasks. They also emphasize the importance of energy and compute capacity as potential bottlenecks for future AI growth. The script suggests that internal developments at companies like OpenAI are far from slowing down, withๆ็คบ (hints) at groundbreaking models like GPT 5 that could revolutionize the field. The summary concludes by emphasizing that despite external appearances, the AI industry is on the cusp of significant breakthroughs that will propel it into a new era of innovation and capability.
Takeaways
- ๐ **AI Hype and Progression**: Despite perceptions that AI hype is waning, significant advancements are still being made in the field of generative AI, with large language models (LLMs) continuing to evolve.
- ๐ **Gartner Hype Cycle**: The Gartner Hype Cycle is a useful tool for understanding the trajectory of technological development, but some argue that AI, particularly LLMs, is not following the traditional pattern of the cycle.
- ๐ก **Innovation in AI**: Companies like OpenAI are making consistent breakthroughs in AI, some of which are not publicly disclosed, indicating that internal progress is much faster than what is visible externally.
- ๐ฅ **Energy and Compute Bottlenecks**: Energy consumption and compute capacity are significant bottlenecks for the future of AI, with the need for vast amounts of power and advanced infrastructure to support increasingly complex models.
- ๐ผ **Business Dynamics**: The shift from open research to closed, proprietary development means that companies are less likely to share their innovations, leading to a perception that progress is slower than it actually is.
- ๐ **Global AI Race**: There is a global race in AI development, with companies investing heavily to stay competitive, which is driving rapid advancements and the potential for new breakthroughs.
- ๐ฌ **Advanced Reasoning Engines**: The incorporation of advanced reasoning engines on top of existing models like GPT-4 is pushing the boundaries of what AI can achieve, particularly in complex problem-solving tasks.
- ๐ **Benchmarking and Competition**: Companies are incentivized to surpass the current benchmarks like GPT-4, leading to a focus on marginal improvements rather than acknowledging the potential for more significant leaps in capability.
- ๐ **Internal Developments at OpenAI**: There is an expectation that OpenAI is significantly ahead of its current public releases, suggesting that future releases could bring substantial advancements in AI capabilities.
- ๐ฅ **Sora and Other Innovations**: OpenAI's release of Sora, a video generation model, and other undisclosed projects indicate that the company is exploring various AI applications beyond just text-based models.
- โณ **Future Predictions**: Industry leaders like Sam Altman suggest that the current state-of-the-art models will be considered primitive in the near future, hinting at the potential for massive jumps in AI performance.
Q & A
What is the current debate about AI on social media platforms like Twitter?
-The current debate is centered around whether the hype surrounding AI, particularly generative AI, is wearing off and if we have reached an exhaustion point in terms of its capabilities and future developments.
What is Sam Altman's stance on the investment in building AGI, despite the high costs?
-Sam Altman is quoted as saying that he doesn't care if they burn $50 billion a year because they are building AGI, and he believes it will be worth it.
What is the Gartner Hype Cycle and how is it relevant to AI technologies?
-The Gartner Hype Cycle is a graphical representation used to illustrate the maturity, adoption, and social application of specific technologies. It provides a conceptual framework to understand how technologies evolve from introduction to mainstream adoption, which is particularly useful for evaluating emerging technologies like generative AI and large language models.
What are some of the current criticisms of large language models (LLMs)?
-Criticisms include biases in the models, the need for vast data training, high operational and inference costs, environmental impacts, and issues like hallucination where the model generates incorrect or misleading information.
What is the 'slope of Enlightenment' in the Gartner Hype Cycle?
-The 'slope of Enlightenment' is a phase where previous issues with a technology are ironed out. For LLMs, this would involve improvements in model efficiency, reduced biases, and increased reliability.
What are some of the advancements in AI that suggest it is not stagnating?
-null
What does Sam Altman imply about the future of AI models beyond GPT-4?
-Sam Altman implies that future models, such as GPT-5, will represent a significant leap from the current state-of-the-art, suggesting that GPT-4 will be considered 'dumb' in comparison to what is to come.
What are the potential bottlenecks that could slow down the progress of generative AI?
-The potential bottlenecks include energy constraints, due to the high power requirements for training and running large models, and compute capacity, as the demand for processing power may exceed current supply.
null
-null
Why might companies be hesitant to publicly release all their AI research and advancements?
-Companies like OpenAI may keep their research and advancements secret to maintain a competitive edge. Revealing too much could allow competitors to replicate their successes and potentially surpass them.
How does the release of GPT-4 as a benchmark affect the AI industry?
-The release of GPT-4 as a benchmark creates an incentive for other companies to train models that just surpass GPT-4, rather than aiming for a significant leap in capabilities. This can create an illusion of a plateau in AI progress.
What does the future hold for AI capabilities according to the advancements and statements made by industry leaders?
-The future of AI is expected to be transformative, with significant advancements in reasoning capabilities, efficiency, and the potential for AI agents to perform complex tasks at a level surpassing current human capabilities.
Outlines
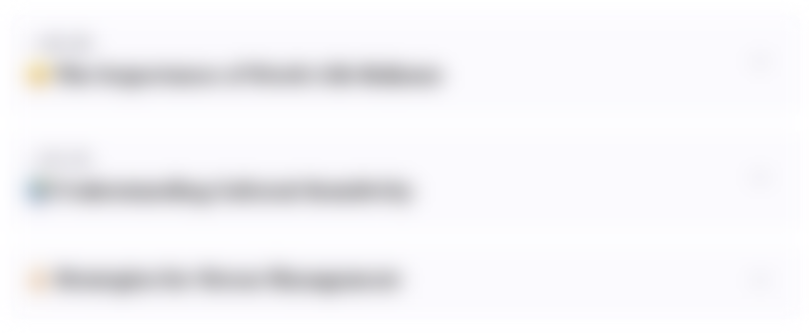
This section is available to paid users only. Please upgrade to access this part.
Upgrade NowMindmap
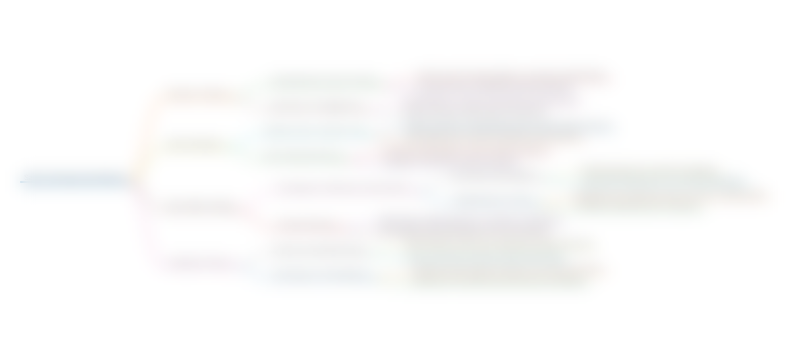
This section is available to paid users only. Please upgrade to access this part.
Upgrade NowKeywords
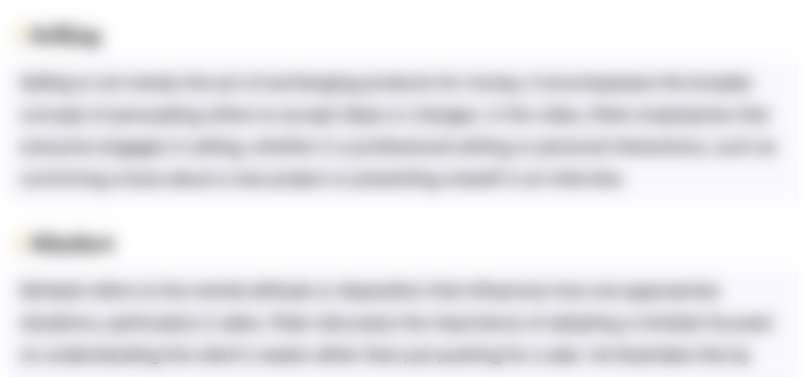
This section is available to paid users only. Please upgrade to access this part.
Upgrade NowHighlights
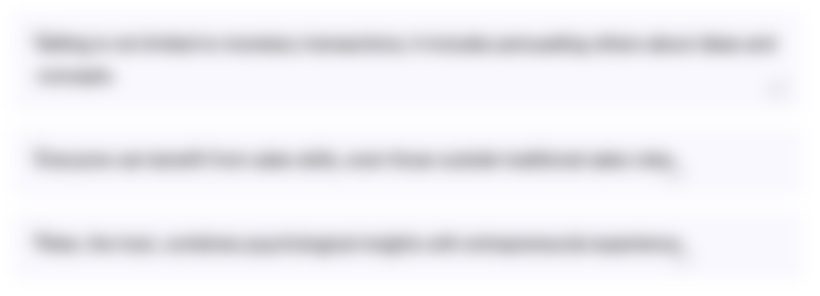
This section is available to paid users only. Please upgrade to access this part.
Upgrade NowTranscripts
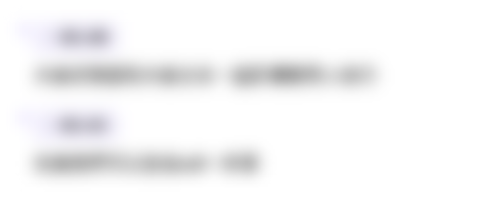
This section is available to paid users only. Please upgrade to access this part.
Upgrade NowBrowse More Related Video
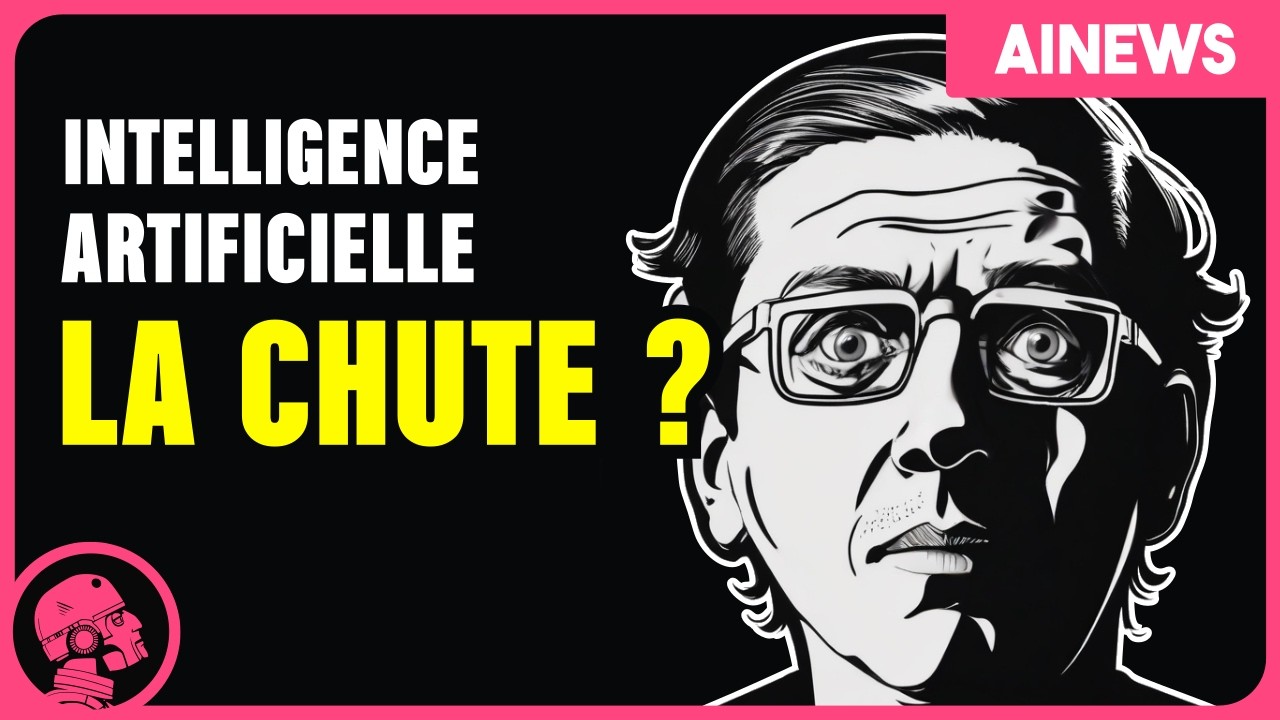
Intelligence Artificielle: la fin du bullshit ? (AI News)
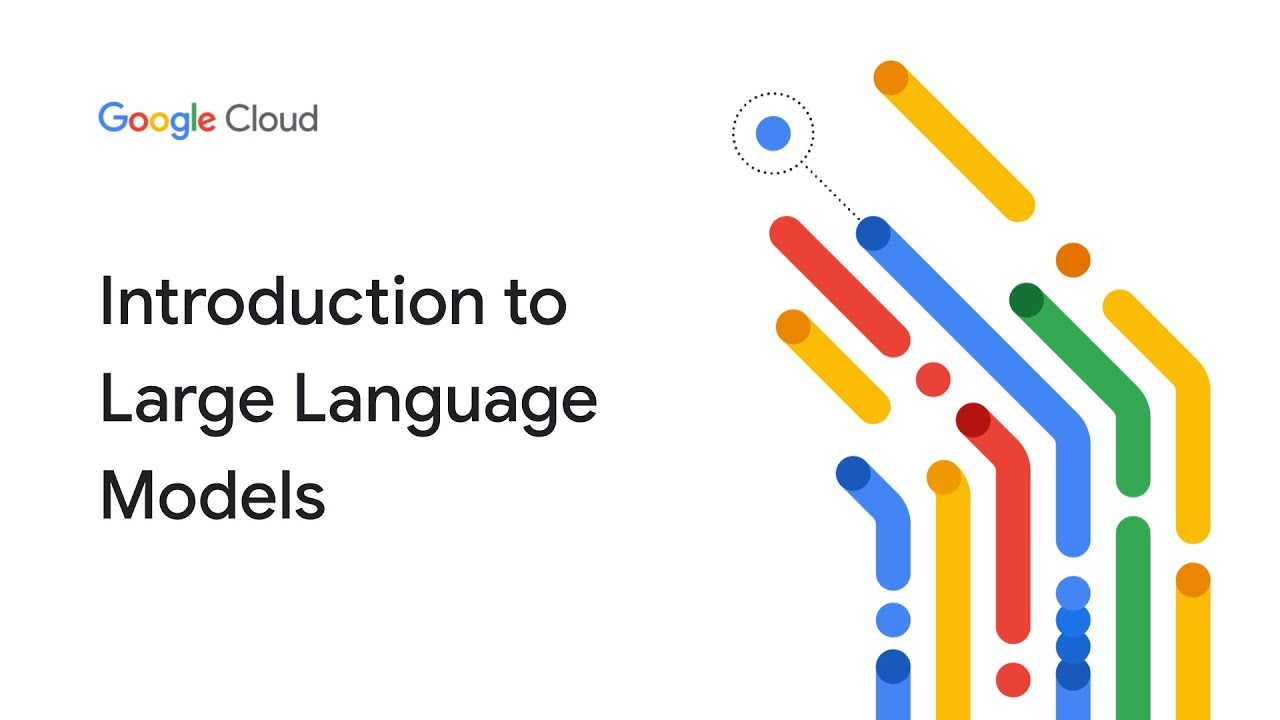
Introduction to large language models
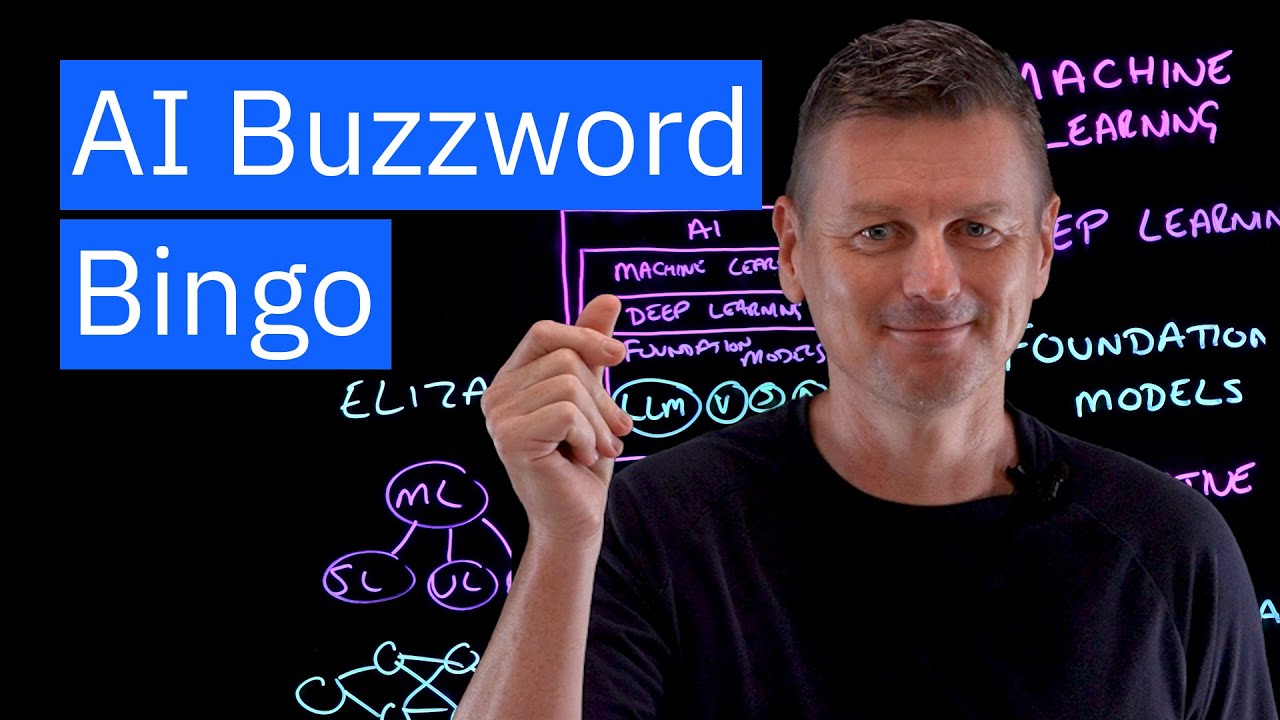
Machine Learning vs. Deep Learning vs. Foundation Models
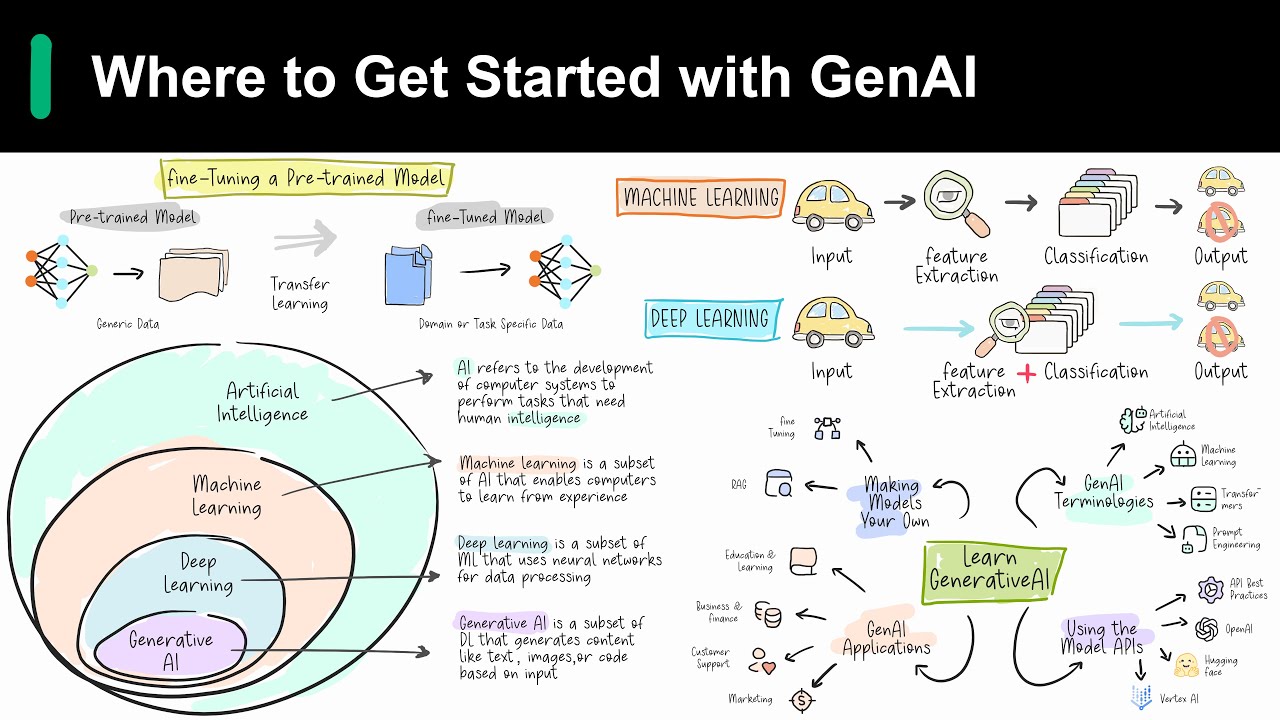
Introduction to Generative AI
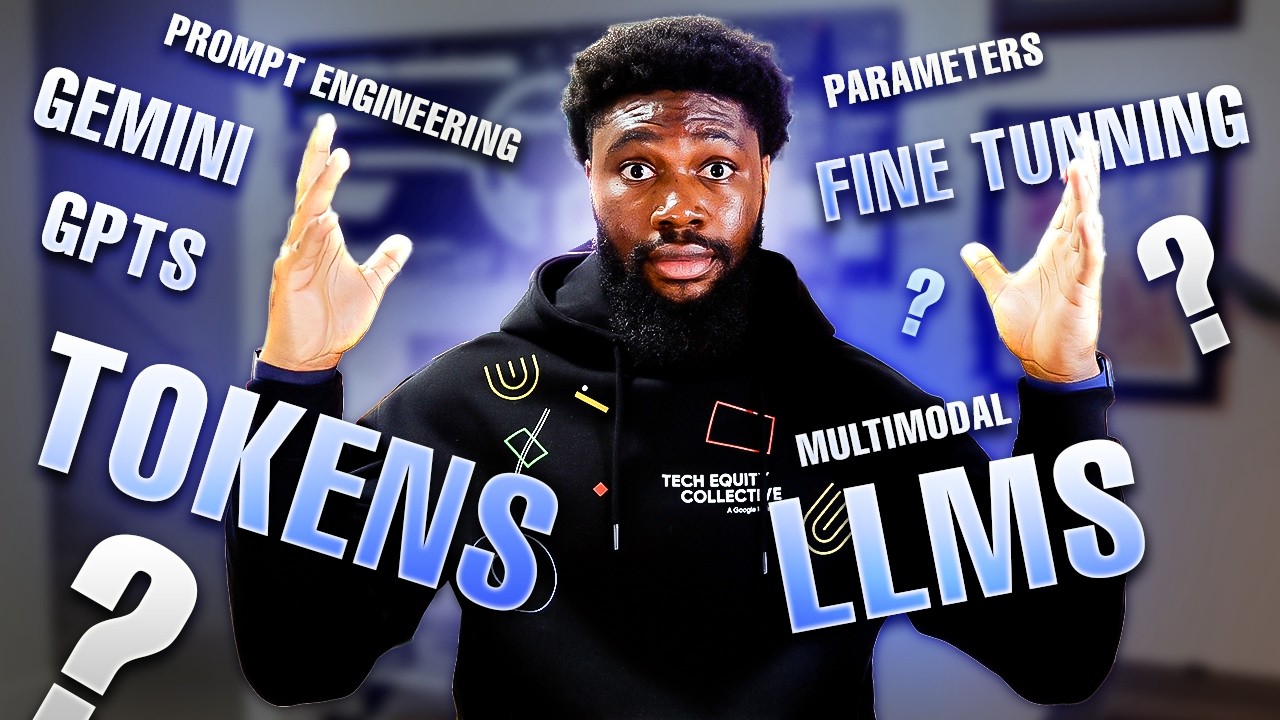
Simplifying Generative AI : Explaining Tokens, Parameters, Context Windows and more.
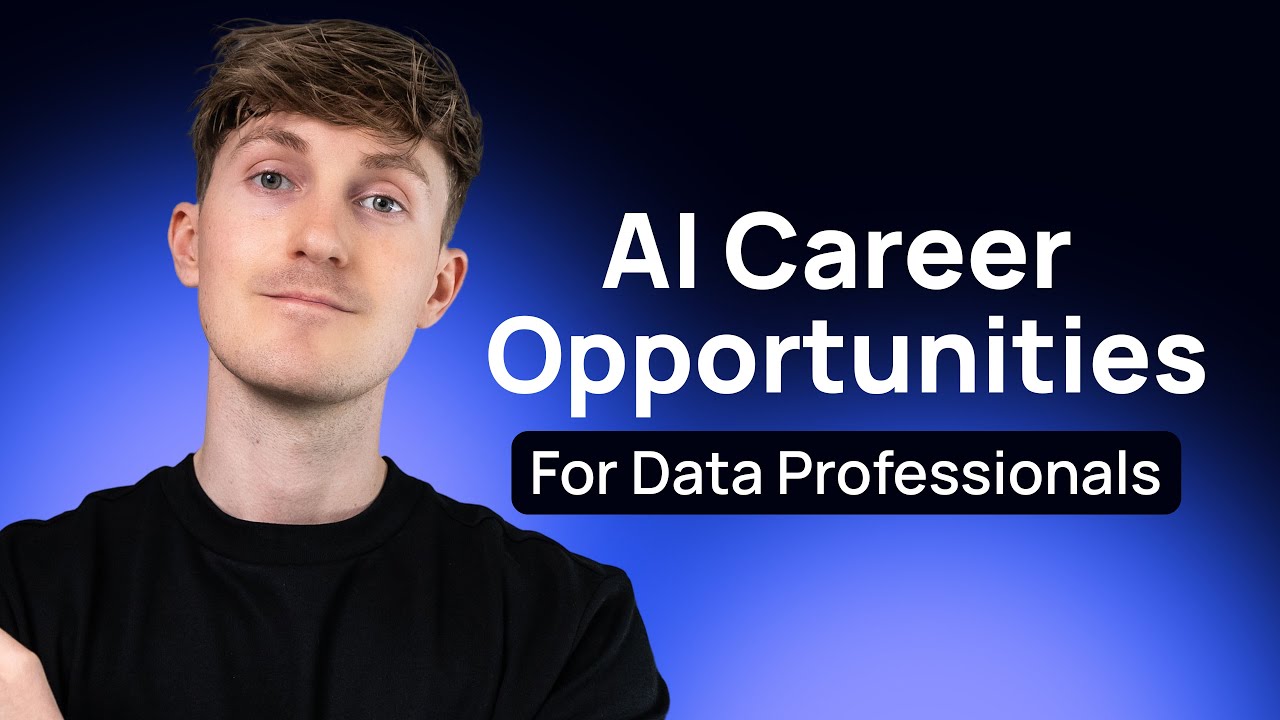
AI Career Opportunities for Data Professionals - Time to Pivot?
5.0 / 5 (0 votes)