TUTORIAL SMARTPLS 4: CARA MEMBUAT LAPORAN SEM PLS REFERENSI HAIR ET AL, 2022 (TERBARU)
Summary
TLDRThis video tutorial covers the process of generating a research report using the latest guidelines from Hair et al. (2022) for Structural Equation Modeling (SEM) in Partial Least Squares (PLS). It delves into the essentials of model evaluation, including measurement models, structural models, reflective and formative indicators, and prediction methods. Key topics include understanding R-squared, the significance of multicollinearity, validity tests, and advanced analysis techniques for mediation and moderation. The presenter also discusses the importance of predicting accuracy and the new procedures recommended in the 2022 edition, offering valuable insights for researchers and academics in the field.
Takeaways
- 😀 The video discusses how to create the latest SEM-PLS report based on Hair et al. (2022), emphasizing key updates in model evaluation and measurement techniques.
- 😀 The speaker stresses the importance of subscribing to their channel for further learning on statistical methods and aims to release 200 videos this year.
- 😀 In SEM-PLS, there are two main types of model evaluation: measurement model evaluation and structural model evaluation.
- 😀 SEM-PLS is defined as a 'prediction-oriented' method, which focuses on maximizing the R-squared value to explain the variation in endogenous variables.
- 😀 Reflective measurement models involve evaluating reliability (internal consistency) and validity (convergent, discriminant), while formative models focus on collinearity and significance of weights.
- 😀 The speaker explains that in reflective measurement models, indicators with loading between 0.40 and 0.70 should not be discarded immediately, as they may still be valuable in improving reliability or AVE.
- 😀 For formative models, redundancy analysis (convergent validity) is necessary but often neglected in Indonesia, requiring at least one reflective indicator to be included in the model.
- 😀 Structural models require multicollinearity checks, hypothesis testing, and evaluating the R-squared value, with the latest guidelines from Hair et al. (2022) deemphasizing fixed thresholds like 0.25, 0.50, and 0.75.
- 😀 Three key metrics for evaluating prediction accuracy in SEM-PLS are Q-squared (predictive relevance), PLS-predict, and CV-AT (cross-validation analysis).
- 😀 Hair et al. (2022) recommends using the percentile bootstrap method for significance testing when data distribution is normal, and BCA (bias-corrected accelerated) for non-normal distributions.
- 😀 In moderation analysis, Hair suggests using the two-stage approach over the orthogonization approach, and recommends interpreting effect sizes (EV) based on the latest guidelines from Kenny for better model estimation.
Q & A
What is the main focus of the video content?
-The video focuses on providing insights into the latest methods of reporting and analysis in Structural Equation Modeling (SEM) based on the Hair et al. (2022) guidelines, particularly in the context of Partial Least Squares (PLS) methodology.
What does the speaker emphasize about SEM-PLS?
-The speaker emphasizes that SEM-PLS is a prediction-oriented approach, with the primary goal being to maximize the R Square, thereby explaining as much of the variation in the endogenous variables as possible through the exogenous variables.
What are the two main types of model evaluation in PLS according to Hair et al. (2022)?
-The two main types of model evaluation in PLS are measurement model evaluation and structural model evaluation.
What is the significance of the R Square value in SEM-PLS according to Hair's 2022 book?
-R Square is viewed as a function of the number of predictor variables. Unlike previous guidelines, the new version does not classify R Square values as low, moderate, or high, emphasizing that it depends on the number of predictors and the specific context of the model.
What changes did Hair (2022) introduce regarding the use of HTMT?
-Hair (2022) has shifted the focus from using Fornell-Larcker criteria and cross-loadings to using the HTMT (Heterotrait-Monotrait ratio) for discriminant validity, with thresholds set at 0.85 for similar constructs and 0.90 for dissimilar ones.
How does Hair recommend handling indicators with low loadings (between 0.40 and 0.70)?
-Indicators with loadings between 0.40 and 0.70 should not be immediately discarded. They should be considered if they help increase the Average Variance Extracted (AVE) and Composite Reliability, otherwise, they should be removed if they do not contribute meaningfully.
What is the rule for evaluating outer weights in formative measurement models?
-In formative measurement models, if the outer weight is below 0.5 and is not significant, it should be discarded. If it is significant but below 0.5, the researcher should carefully consider whether to retain the indicator based on its relevance.
What are the three key measures of predictive power in SEM-PLS?
-The three key measures of predictive power in SEM-PLS are Q² predict, PLS predict, and CV-RT (cross-validation statistics), which evaluate how well the model performs in predicting the variance of endogenous variables.
What does Hair (2022) say about the use of the Sobel test for mediation analysis?
-Hair (2022) states that the Sobel test is no longer recommended for mediation analysis in SEM-PLS. Instead, researchers should use bootstrapping techniques, which offer more robust results for testing mediation effects.
What does the speaker suggest regarding the use of the Two-Stage approach in moderation analysis?
-The speaker recommends using the Two-Stage approach for moderation analysis in PLS version 4, as it has been shown to provide more accurate parameter estimates compared to the older orthogonal or product-indicator approaches.
Outlines
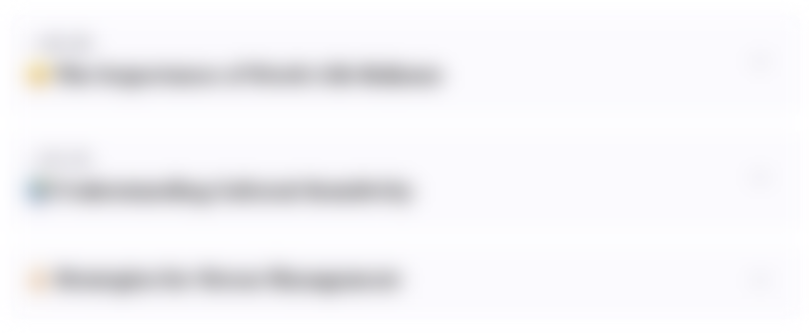
Dieser Bereich ist nur für Premium-Benutzer verfügbar. Bitte führen Sie ein Upgrade durch, um auf diesen Abschnitt zuzugreifen.
Upgrade durchführenMindmap
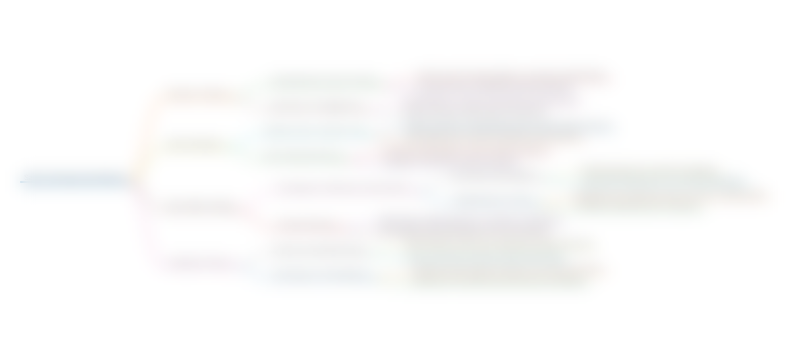
Dieser Bereich ist nur für Premium-Benutzer verfügbar. Bitte führen Sie ein Upgrade durch, um auf diesen Abschnitt zuzugreifen.
Upgrade durchführenKeywords
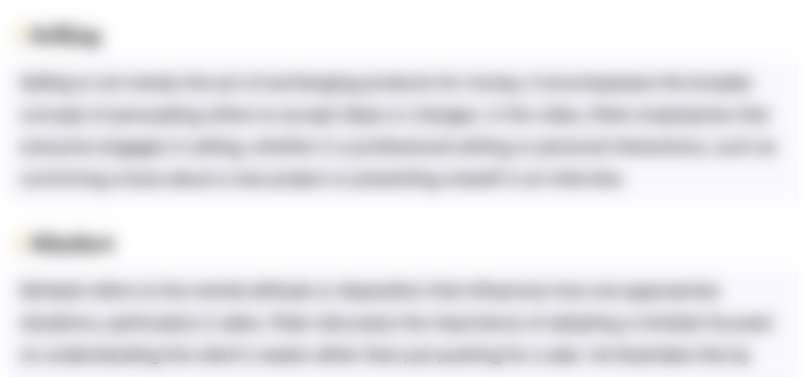
Dieser Bereich ist nur für Premium-Benutzer verfügbar. Bitte führen Sie ein Upgrade durch, um auf diesen Abschnitt zuzugreifen.
Upgrade durchführenHighlights
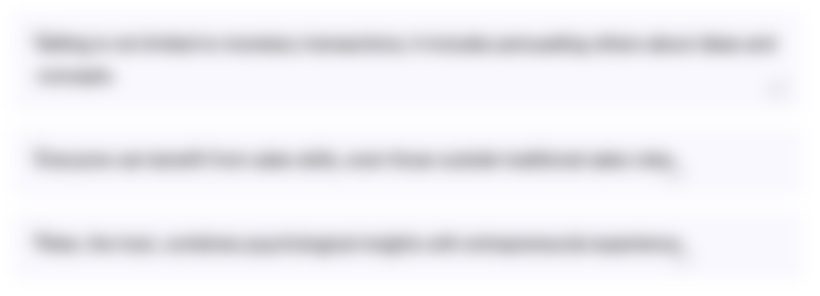
Dieser Bereich ist nur für Premium-Benutzer verfügbar. Bitte führen Sie ein Upgrade durch, um auf diesen Abschnitt zuzugreifen.
Upgrade durchführenTranscripts
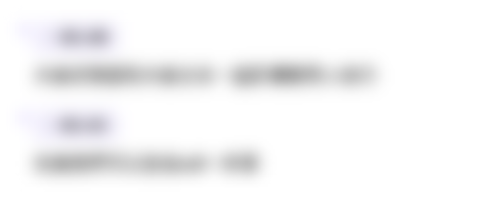
Dieser Bereich ist nur für Premium-Benutzer verfügbar. Bitte führen Sie ein Upgrade durch, um auf diesen Abschnitt zuzugreifen.
Upgrade durchführenWeitere ähnliche Videos ansehen
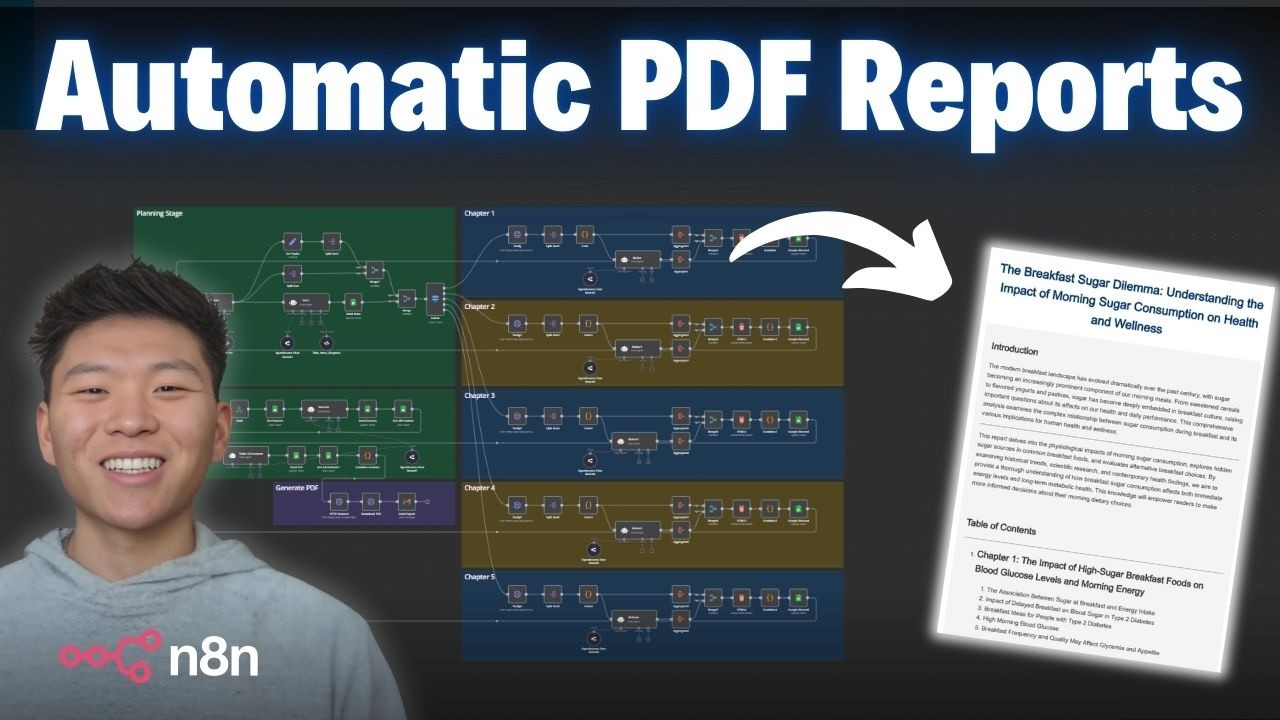
I Built Deep Research That Beats Perplexity & OpenAI (free template) n8n tutorial
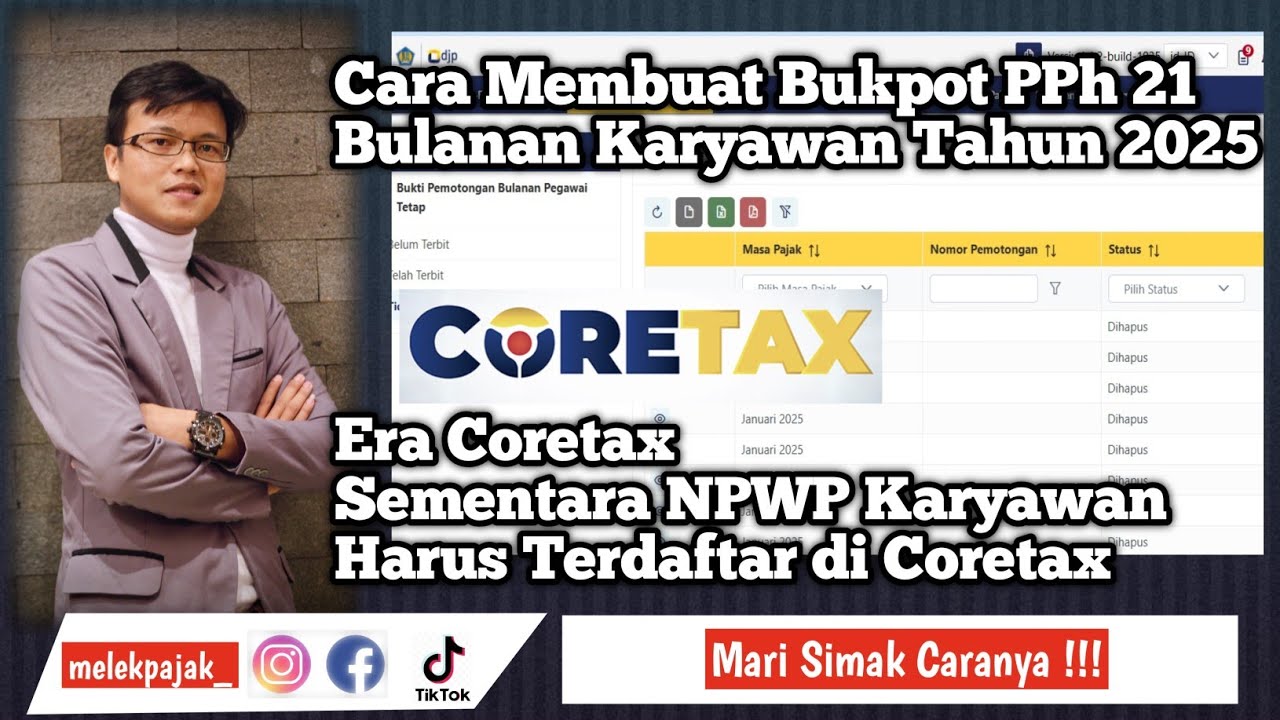
Cara Membuat Bukti Potong PPh 21 Karyawan Tahun 2025 Coretax
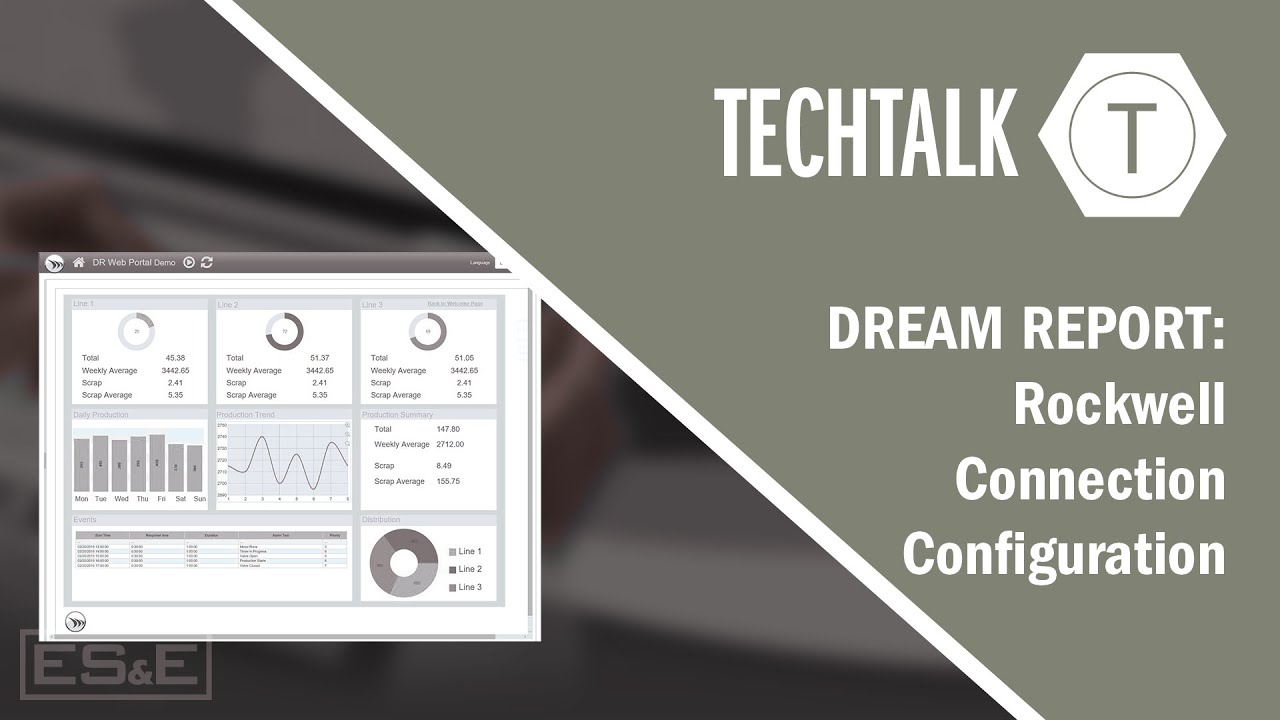
Dream Report: Rockwell Connection Configuration
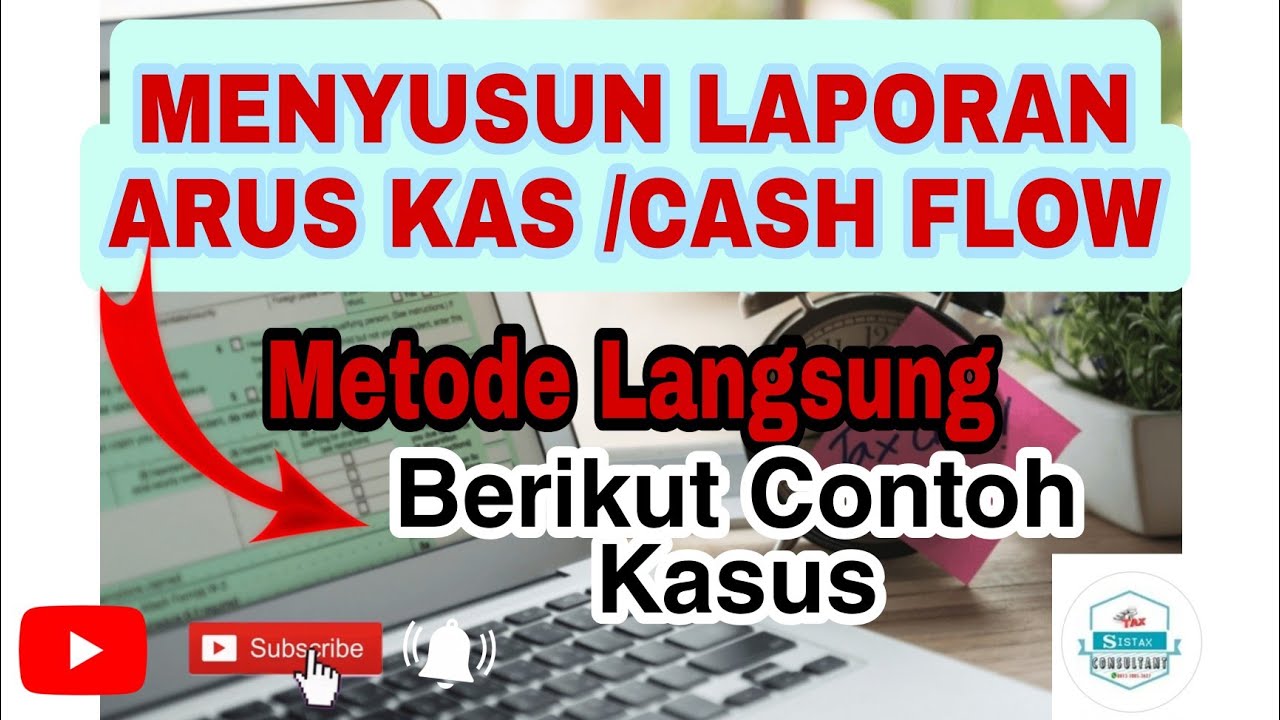
Cara Membuat Arus Kas Metode Langsung || Cash Flow Statement Direct Method #TutorialAkuntansi

A PESQUISA CIENTÍFICA: TIPOS E FASES
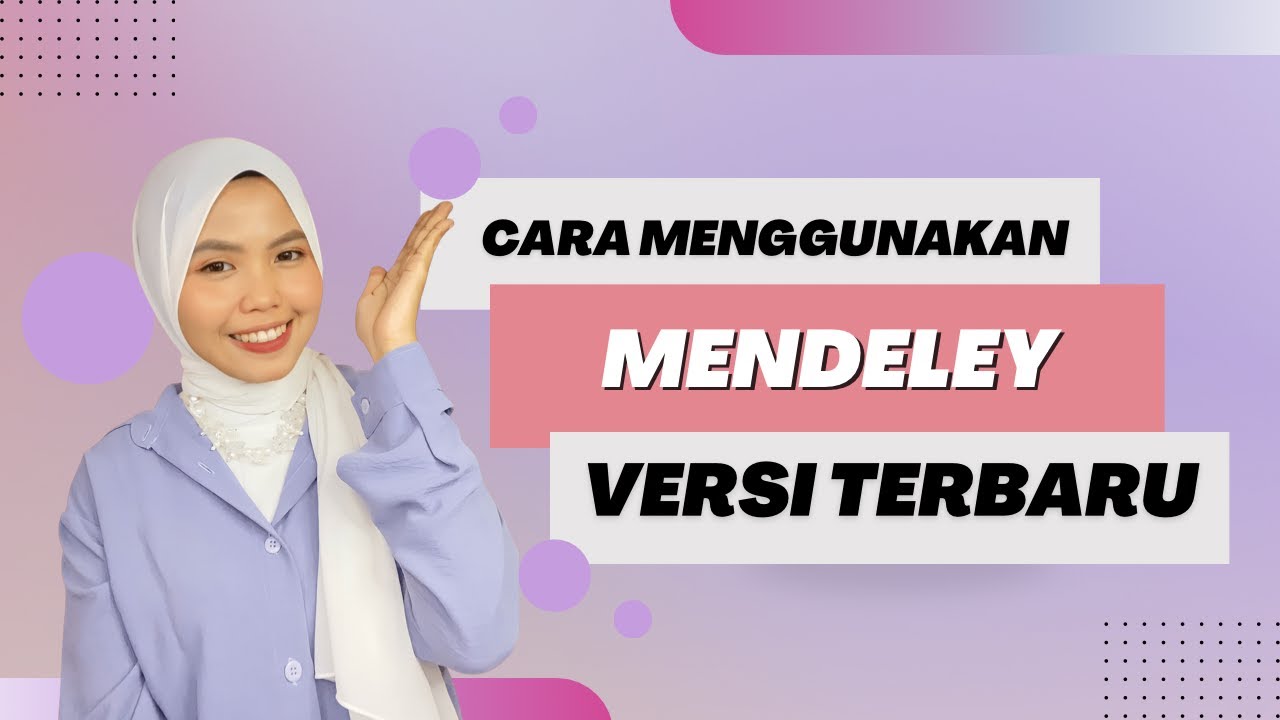
Tutorial Menggunakan Mendeley Versi Terbaru (Tutorial Lengkap Bodynote-Daftar pustaka di Mendeley)
5.0 / 5 (0 votes)