What Is AlphaFold? | NEJM
Summary
TLDRAlphaFold 2, an AI system developed by Google DeepMind, revolutionizes protein structure prediction by accurately determining 3D structures from a protein's amino acid sequence. Unlike experimental methods like x-ray crystallography, it leverages multiple sequence alignment (MSA) and neural networks, including the Evoformer and Structure module, to refine predictions. This method enables faster, cost-effective predictions that can aid in drug discovery, genetic variant analysis, protein engineering, and more. Though not as precise as experimental methods, AlphaFold's predictions hold great potential in medicine, biotechnology, and agriculture.
Takeaways
- 😀 AlphaFold AI, developed by Google DeepMind, can predict 3D protein structures from amino acid sequences with accuracy comparable to experimental methods.
- 😀 Traditional methods of determining protein structures, such as x-ray crystallography and cryoelectron microscopy, are time-consuming and expensive.
- 😀 AlphaFold 2, the 2021 iteration of the system, accelerates the process of predicting protein structures by using AI algorithms.
- 😀 The first step in AlphaFold's process is creating a multiple sequence alignment (MSA) by comparing the input protein sequence with known sequences in various databases.
- 😀 AlphaFold searches for templates of similar sequences with experimentally determined structures to form an initial pair representation of the input sequence.
- 😀 The Evoformer neural network, unique to AlphaFold, is responsible for refining the relationships between residue pairs in the protein sequence.
- 😀 Evoformer works in two towers: one focuses on row-wise relationships and the other evaluates pairwise interactions between residues.
- 😀 The process involves 48 iterations within the Evoformer to refine models of the MSA and pair representations before proceeding.
- 😀 After refining, the structure module performs rotations and translations on the amino acids, revealing an initial 3D structure.
- 😀 The final result, after multiple iterations, is a predicted 3D atomic model of the protein structure, but experimental structures remain more accurate and should be preferred when available.
- 😀 Potential applications of AlphaFold's predictions include drug discovery, genetic variant analysis, modeling protein-protein interactions, and protein engineering for biotechnology and medicine.
Q & A
What is the central dogma of molecular biology and how does it relate to protein structure?
-The central dogma of molecular biology explains how genetic information is transferred from DNA to RNA and then to an amino acid sequence, which is crucial for folding into a three-dimensional protein structure.
What are the traditional methods used to determine protein structure experimentally?
-The traditional methods for determining protein structure experimentally include X-ray crystallography, nuclear magnetic resonance (NMR) spectroscopy, and cryoelectron microscopy.
What challenges do researchers face with traditional methods of protein structure determination?
-Traditional methods are time-consuming and expensive, which makes them less efficient compared to computational alternatives like AlphaFold.
How does AlphaFold 2 differ from experimental methods in determining protein structures?
-AlphaFold 2, developed by Google DeepMind, is the first non-experimental method that can rapidly predict 3D protein structures with accuracy comparable to experimental methods.
What is the first step in AlphaFold 2's process of predicting protein structure?
-The first step involves comparing the input sequence of amino acids to various protein sequence databases to generate a multiple sequence alignment (MSA) and an initial MSA representation.
What role do templates of experimentally determined protein structures play in AlphaFold 2?
-AlphaFold 2 searches for similar sequence proteins with known experimentally determined structures, which help create an initial pair representation of the input sequence and its residue relationships.
What is the Evoformer in AlphaFold 2 and what does it do?
-The Evoformer is a neural network unique to AlphaFold 2. It uses two towers to communicate and refine residue relationships by evaluating row-wise and column-wise data, which helps triangulate relationships between residue pairs.
How does AlphaFold 2 ensure the accuracy of residue relationships during processing?
-AlphaFold 2 uses the triangle inequality theorem during the triangulation of residue pairs to ensure that the sum of two edges in a triangle is greater than or equal to the third edge, maintaining accuracy in structure prediction.
What happens after the Evoformer refines the MSA and pair representations?
-The refined MSA and pair representations are then passed to the structure module, which performs rotations and translations on the amino acids to generate an initial 3D protein structure while applying physical and chemical constraints.
How many cycles does AlphaFold 2 go through to finalize a predicted protein structure?
-AlphaFold 2 undergoes four cycles of refinement, using the Evoformer and structure module processes iteratively to arrive at the final predicted 3D atomic coordinates of the protein's structure.
What are some potential applications of predicted protein structures from AlphaFold 2?
-Predicted 3D protein structures from AlphaFold 2 can be used in drug discovery, estimating the impact of genetic variants on protein function, modeling protein-protein interactions, and engineering proteins for various applications in medicine, biotechnology, agriculture, and environmental sciences.
Why are experimentally determined protein structures preferred over predicted ones?
-Experimentally determined 3D structures are typically more accurate than predicted structures and should be used preferentially when available, as they provide more reliable data for understanding protein function.
Outlines
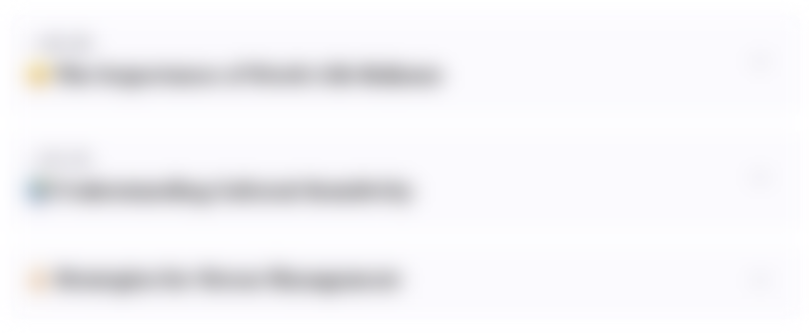
此内容仅限付费用户访问。 请升级后访问。
立即升级Mindmap
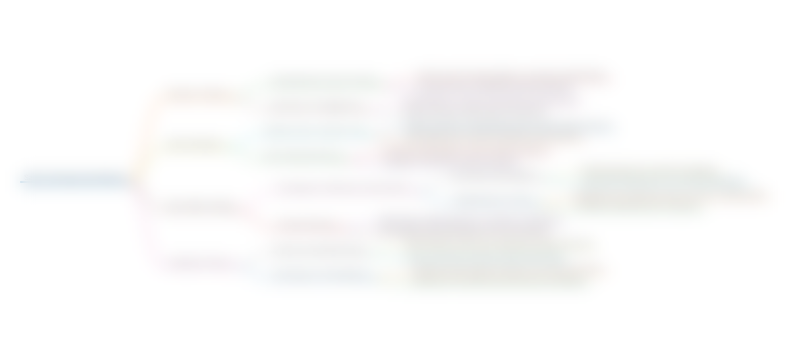
此内容仅限付费用户访问。 请升级后访问。
立即升级Keywords
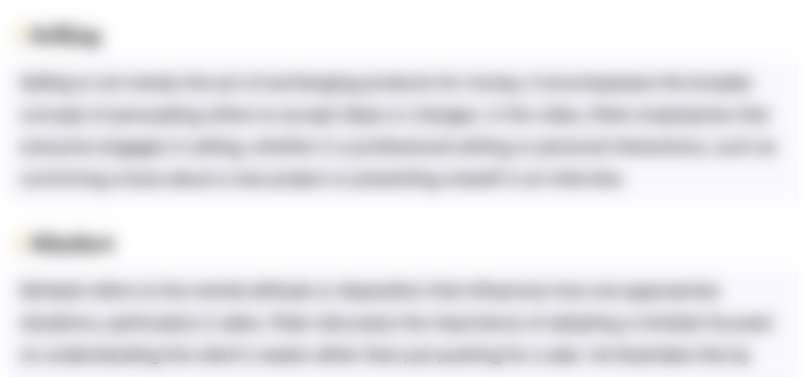
此内容仅限付费用户访问。 请升级后访问。
立即升级Highlights
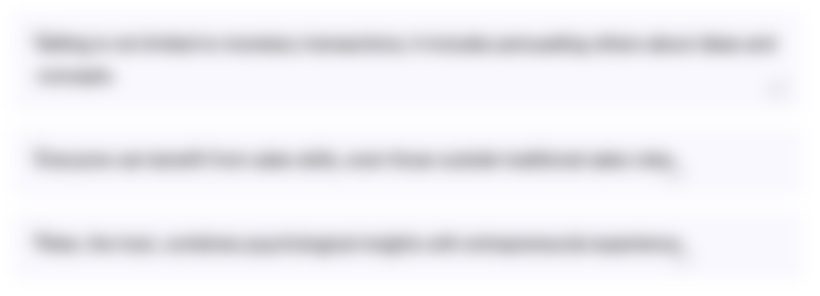
此内容仅限付费用户访问。 请升级后访问。
立即升级Transcripts
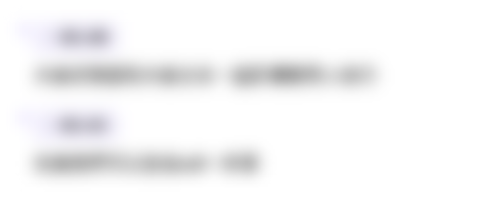
此内容仅限付费用户访问。 请升级后访问。
立即升级浏览更多相关视频

Protein Synthesis (Part 2 of 2) - Translation
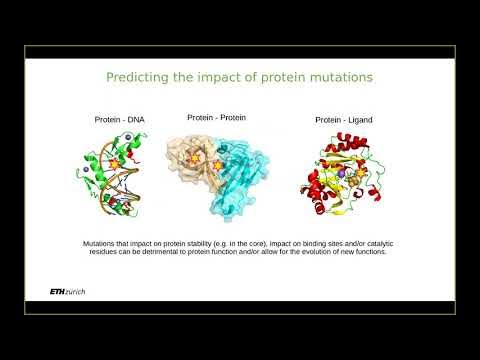
PTP - Structural Bioinformatics 3
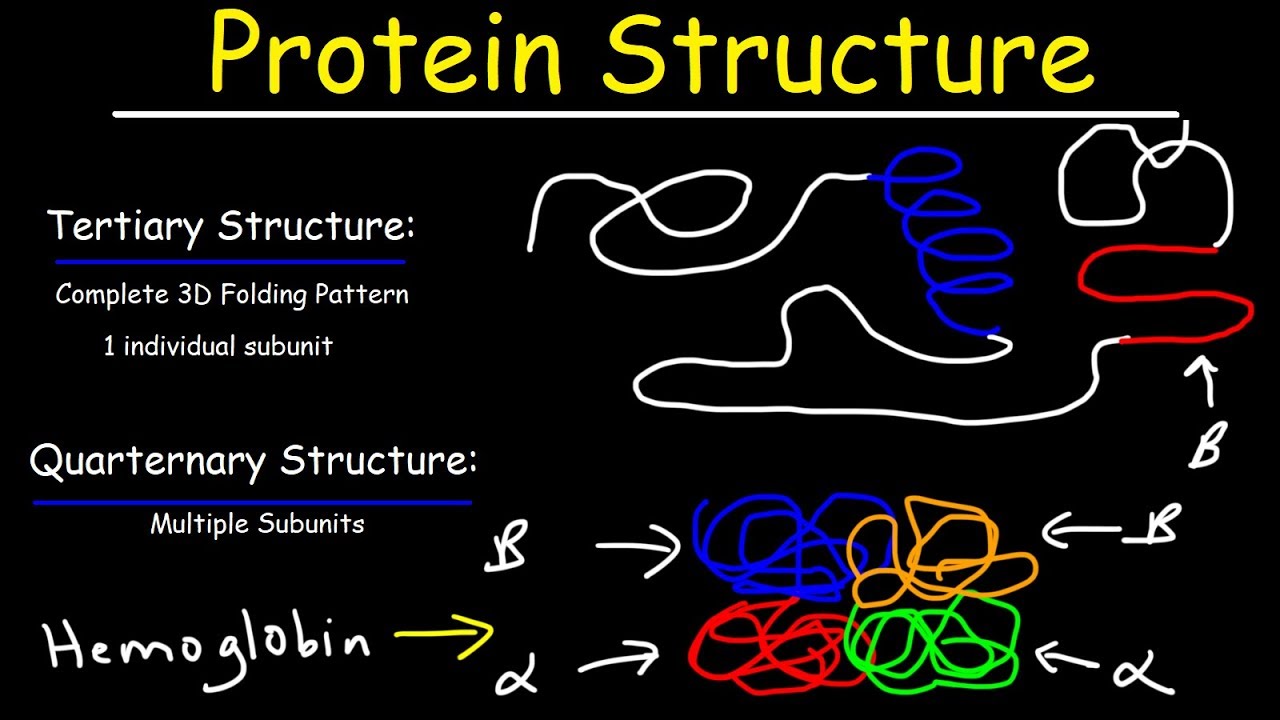
Protein Structure - Primary, Secondary, Tertiary, & Quarternary - Biology
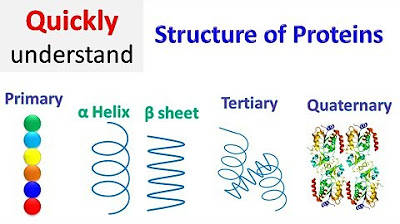
Protein structure | Primary | Secondary | Tertiary | Quaternary
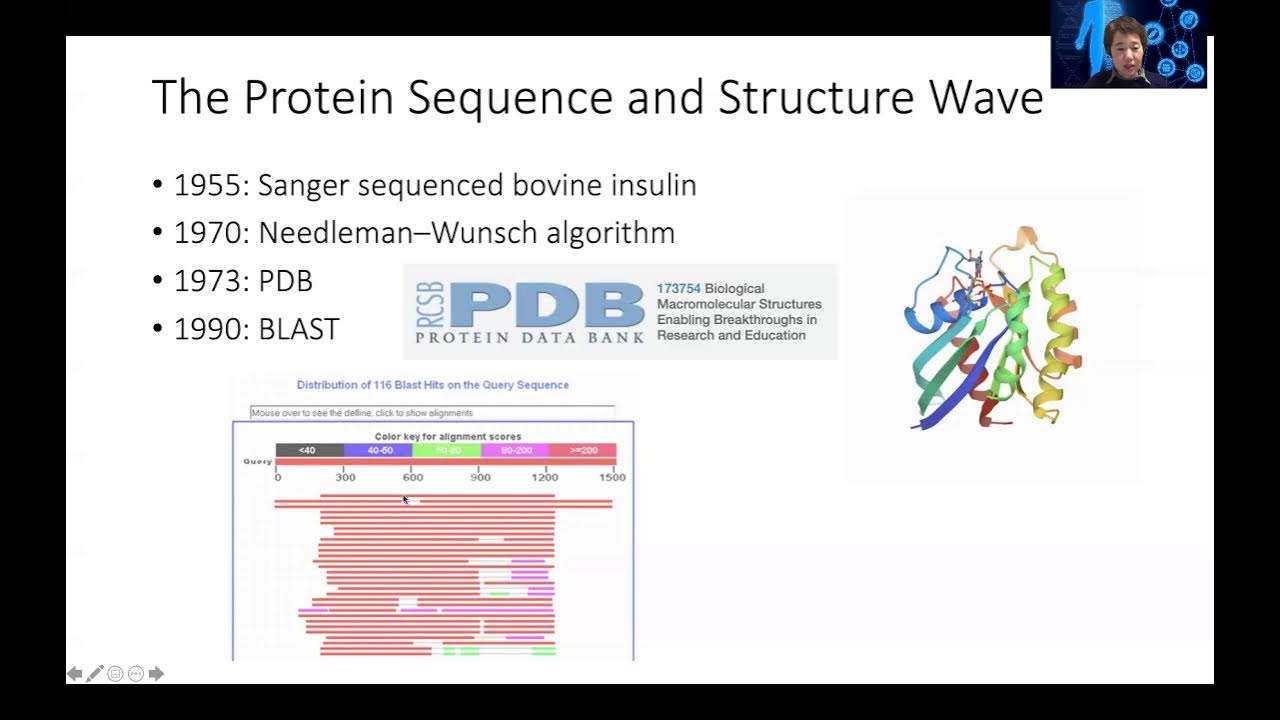
STAT115 Chapter 2.1 Protein Wave
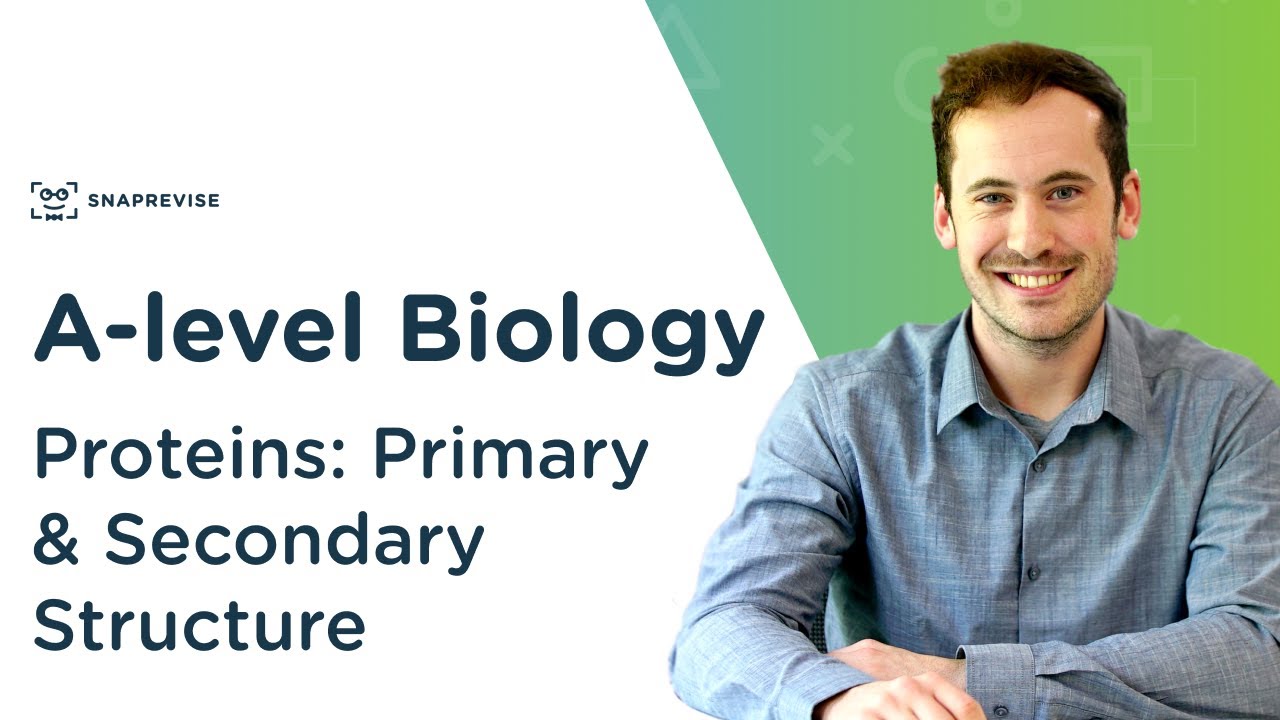
Proteins: Primary and Secondary Structure | A-level Biology | OCR, AQA, Edexcel
5.0 / 5 (0 votes)