PTP - Structural Bioinformatics 3
Summary
TLDRThis video class covers two main topics in structural bioinformatics: predicting protein structures from sequences and assessing the impact of mutations. It begins by explaining how protein sequences encode the 3D structure through secondary structures like alpha helices and beta sheets, highlighting key prediction methods such as homology and evolution-based co-evolution. The role of neural networks, particularly in AlphaFold 2, in enhancing structure prediction is discussed. The second part focuses on mutation impact, using computational methods to predict how changes in protein structure affect stability, with a practical example of using FoldX to analyze mutation consequences.
Takeaways
- π Protein sequences encode the information needed to predict their three-dimensional structures, including secondary structures like alpha-helices and beta-sheets.
- π Homology-based methods are effective for predicting protein structures when sequences share high similarity (over 30%).
- π The folding problem is complicated by the vast number of possible conformations, making random search infeasible. Instead, local interactions guide the folding process.
- π Evolutionary co-evolution of residues in homologous sequences provides valuable insights for predicting protein structure and stability.
- π Machine learning, particularly neural networks like AlphaFold, has dramatically improved the accuracy of protein structure predictions.
- π AlphaFold can predict protein structures with near-experimental accuracy by learning from multiple sequence alignments and predicting residue distance constraints.
- π The CAST competition evaluates protein structure prediction methods by providing experimentally unsolved protein structures for prediction.
- π AlphaFold has revolutionized protein structure prediction, achieving results that are comparable to experimental methods for well-studied proteins.
- π Once a protein structure is predicted, mutation impact predictions can assess whether a mutation destabilizes or stabilizes the protein based on changes in energy (ΞΞG).
- π The stability of a protein can be impacted by mutations in conserved regions, with core mutations typically being destabilizing and surface mutations less so.
- π Tools like FoldX help assess the impact of mutations by calculating energy terms related to steric clashes, hydrophobic interactions, and other stability factors.
Q & A
What is the main focus of this class in structural bioinformatics?
-The class focuses on two main topics: predicting protein structures from sequences and predicting the impact of mutations on protein stability.
What is the folding problem in protein structure prediction?
-The folding problem refers to the challenge of predicting the three-dimensional structure of a protein based solely on its amino acid sequence. The complexity arises from the vast number of possible conformations that a protein could adopt.
Why is it difficult to predict protein structures directly from sequences?
-It is difficult because there are an enormous number of possible conformations that a protein could fold into, making random search infeasible. The process needs to be guided by local interactions and evolutionary information.
What is the Levinthal paradox and how does it relate to protein folding?
-Levinthal's paradox states that the number of possible conformations a protein could adopt is so large that finding the correct folded state through random search would take longer than the age of the universe. This paradox highlights the need for a guided search process in protein folding.
How does homology help in predicting protein structures?
-Homology helps by using the idea that proteins with similar sequences tend to adopt similar structures. If a protein sequence has a high sequence identity with a known structure, its structure can often be predicted accurately.
What role does co-evolution play in predicting protein structures?
-Co-evolution provides insights into protein structures by identifying positions in a protein that are likely to be in close contact in three-dimensional space. These positions tend to evolve together, and this information can be extracted from multiple sequence alignments to predict the protein's structure.
What is the role of neural networks in recent advancements in protein structure prediction?
-Neural networks have been integrated into protein structure prediction, particularly by predicting three-dimensional distance constraints between pairs of residues. This approach has led to significant improvements in prediction accuracy, especially in models like AlphaFold 2.
What is AlphaFold, and how does it compare to experimental methods in protein structure prediction?
-AlphaFold is a deep learning-based tool developed to predict protein structures from sequences. In 2020, AlphaFold's predictions were shown to be as accurate as experimental methods for many proteins, although it works best for proteins that are well-organized and not membrane-bound.
What are some of the key confidence estimates in AlphaFold predictions?
-AlphaFold provides two key confidence estimates: the local confidence of each residue (PLDDT) and the predicted local error (Pae), which measures the confidence in the relative position between two residues.
How can mutations be studied using protein structures?
-Mutations can be studied by modeling them in silico on a protein's structure and predicting how they impact the stability of the protein. For example, a mutation can be evaluated based on whether it causes destabilization or stabilization of the folded structure.
What is Delta Delta G, and how is it used to predict the impact of mutations?
-Delta Delta G (ΞΞG) is the change in the Gibbs free energy of a protein structure caused by a mutation. A positive ΞΞG indicates that the mutation is destabilizing, while a negative value suggests that the mutation is stabilizing.
What is FoldEx, and how is it used in mutation impact prediction?
-FoldEx is a computational tool used to predict the impact of mutations on protein stability by evaluating energy terms such as steric clashes and hydrophobic interactions. It can assess how mutations affect protein structure and stability based on these energy calculations.
Outlines
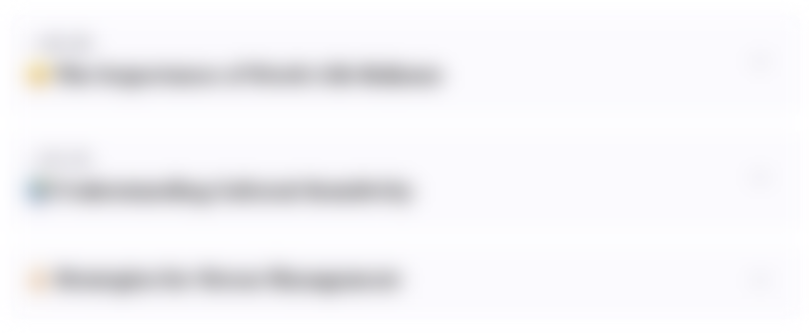
This section is available to paid users only. Please upgrade to access this part.
Upgrade NowMindmap
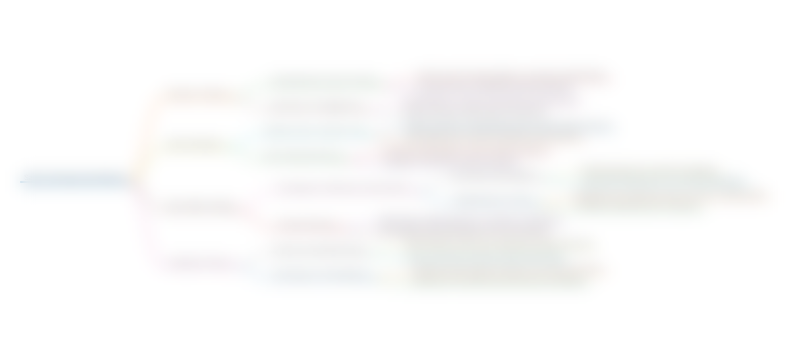
This section is available to paid users only. Please upgrade to access this part.
Upgrade NowKeywords
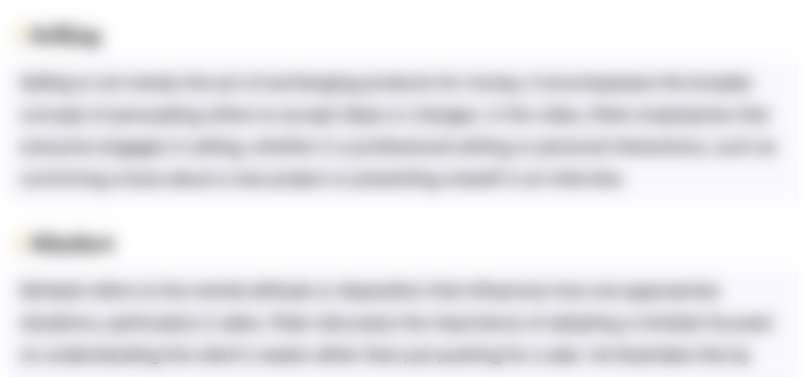
This section is available to paid users only. Please upgrade to access this part.
Upgrade NowHighlights
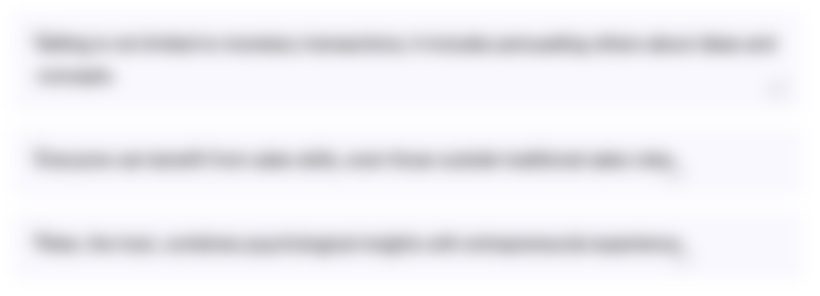
This section is available to paid users only. Please upgrade to access this part.
Upgrade NowTranscripts
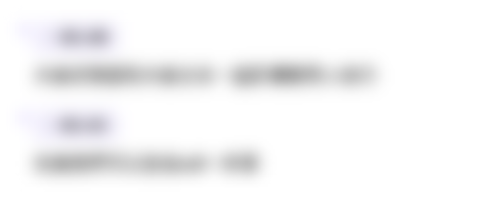
This section is available to paid users only. Please upgrade to access this part.
Upgrade NowBrowse More Related Video
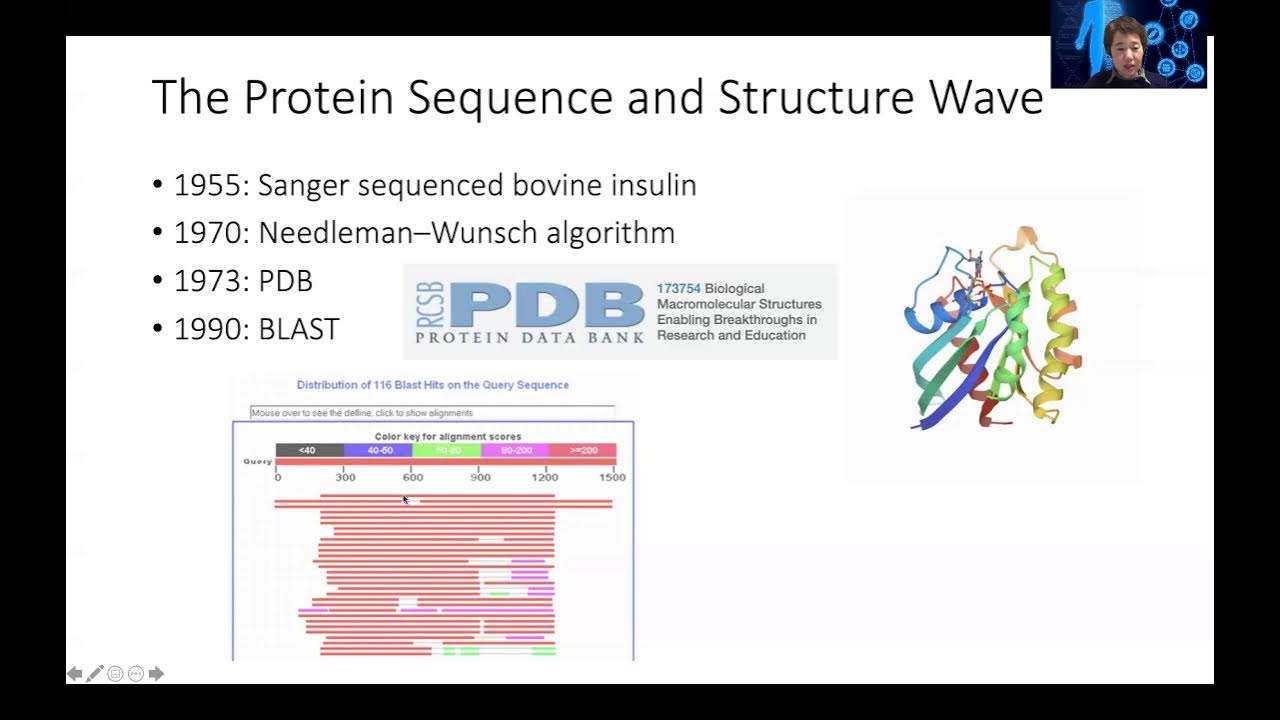
STAT115 Chapter 2.1 Protein Wave

Chem 1307 Ch 19.3 Proteins - Secondary Structure
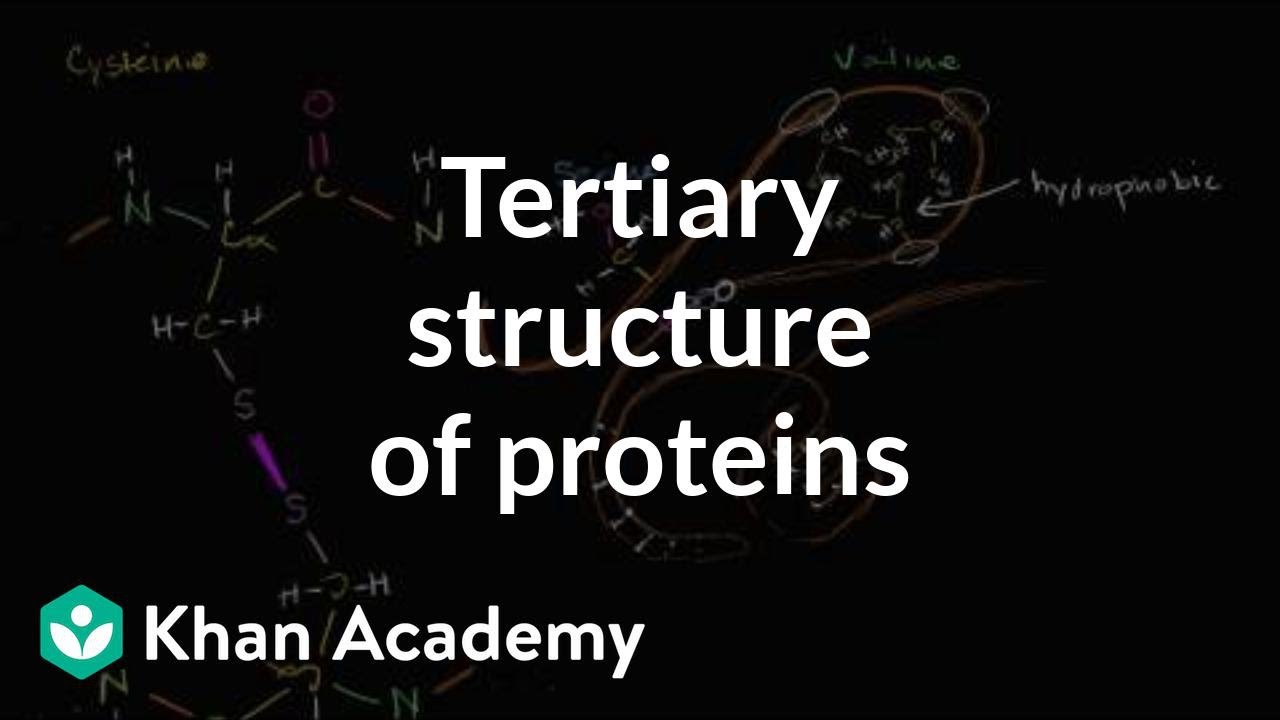
Tertiary structure of proteins | Macromolecules | Biology | Khan Academy
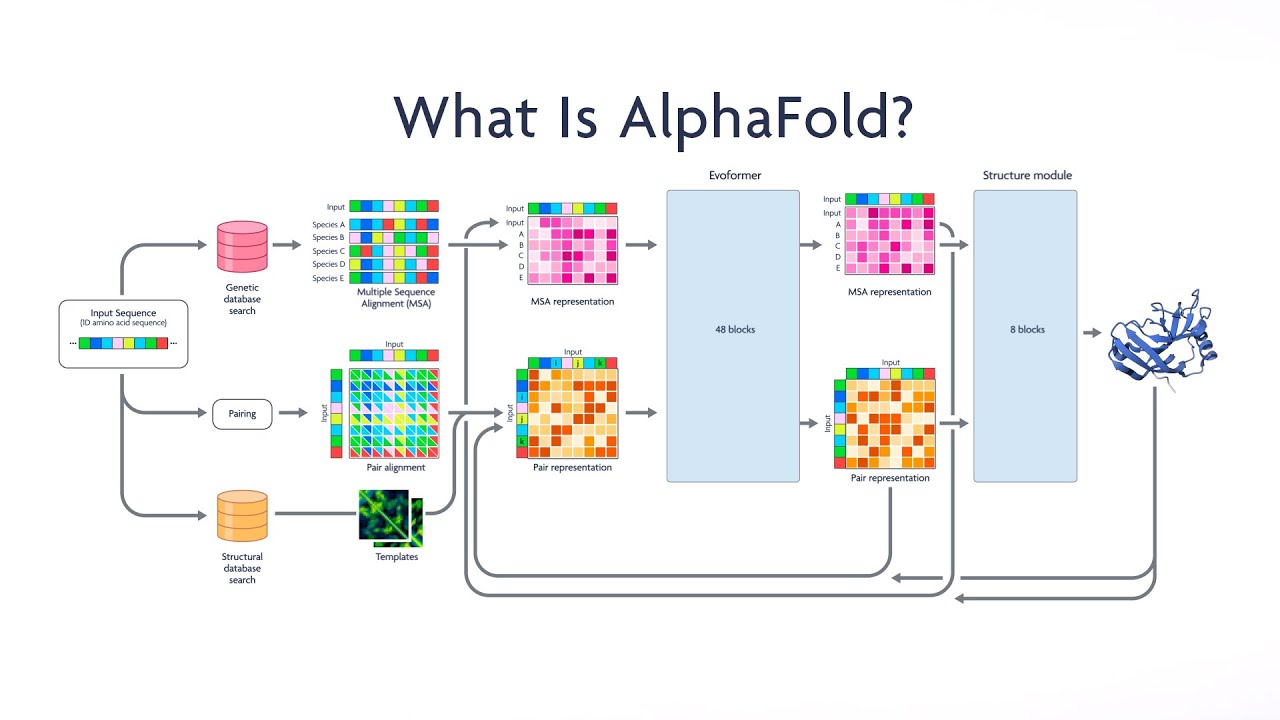
What Is AlphaFold? | NEJM
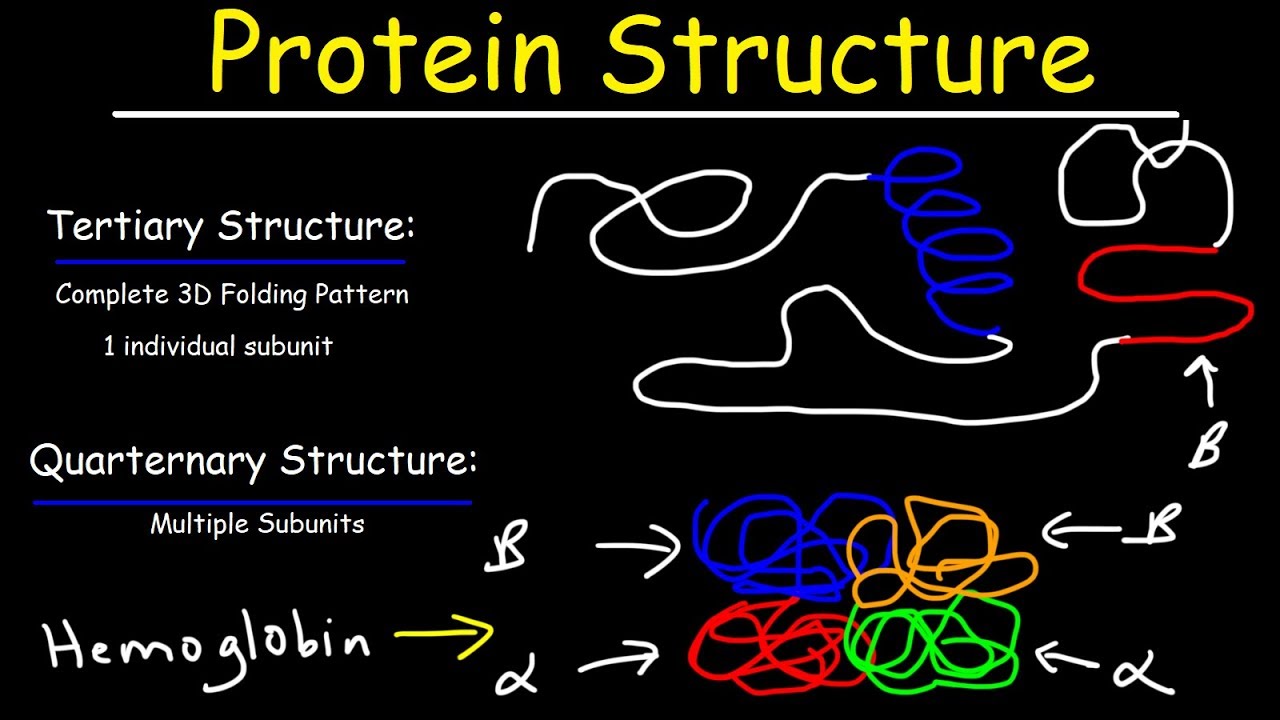
Protein Structure - Primary, Secondary, Tertiary, & Quarternary - Biology
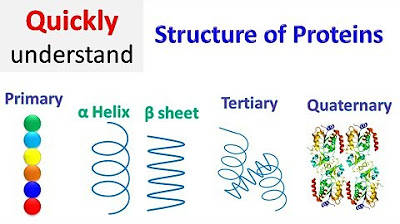
Protein structure | Primary | Secondary | Tertiary | Quaternary
5.0 / 5 (0 votes)