How works Artificial Intelligence for risk management
Summary
TLDRIn this webinar, Nicole Koza and data scientist Hi Faguna explore the integration of artificial intelligence in risk management. They define AI, delve into machine learning and deep learning, and discuss their applications in client segmentation and risk recommendation systems using chat GPT. The session emphasizes the importance of aligning AI techniques with business goals, the significance of data cleaning, and the practical steps for achieving accurate client segmentation. It also highlights the potential of natural language processing in understanding and generating human language for risk assessment.
Takeaways
- π The webinar focuses on the theoretical and practical aspects of artificial intelligence in risk management.
- π The speaker, Faguna, introduces the concept of artificial intelligence (AI) as the ability to reason, solve problems, and learn from experience without human intervention.
- π€ AI is composed of two main areas: machine learning and deep learning, which are responsible for developing and training algorithms and models.
- π The importance of data science is highlighted, emphasizing the need for a clear business goal before applying AI techniques.
- π Two types of machine learning are discussed: supervised learning, which requires a target variable, and unsupervised learning, which does not.
- π¦ An example of supervised learning is identifying fraudulent transactions in a bank's dataset, while unsupervised learning might be used for customer segmentation in a retail company.
- π€ The benefits of machine learning for segmentation include accuracy, automation, scalability, adaptability, and personalization.
- π Two clustering algorithms are mentioned: k-means, which is simple and efficient but sensitive to initialization, and Birch, which is robust to outliers and suitable for large datasets.
- π§Ή The critical step of data cleaning is discussed, including outlier detection, categorical variable encoding, data normalization, and principal component analysis.
- π Two methods for determining the optimal number of segments are presented: the elbow method, which uses inertia, and the silhouette method, which considers cohesion and separation.
- π‘ The platform demonstration shows how to perform client segmentation using the discussed techniques and tools, emphasizing the importance of analyzing results from a business perspective.
Q & A
What is the main focus of the webinar presented by Nicole Koza and Data Scientist Faguna?
-The main focus of the webinar is to explore the theoretical and practical aspects of artificial intervention in risk management, including applications of artificial intelligence, machine learning for segmentation, and natural language processing techniques.
What is the definition of artificial intelligence as discussed in the webinar?
-Artificial intelligence is defined as the ability of a computer program or application to reason, solve problems, understand complex ideas, learn quickly, and learn from experience, without the need for human intervention.
What are the two main sub-areas of artificial intelligence mentioned in the script?
-The two main sub-areas of artificial intelligence mentioned are machine learning and deep learning, which are responsible for developing and training the algorithms and models used in AI applications.
Why is data science important in the context of artificial intelligence applications?
-Data science is important because it helps to identify clear business goals or problems that AI applications aim to solve or opportunities they aim to leverage. It ensures that AI techniques are used as tools to achieve specific business objectives rather than being an end in themselves.
What are the two types of machine learning mentioned in the script?
-The two types of machine learning mentioned are supervised learning, which requires a target variable, and unsupervised learning, which does not require a target variable and is often used for pattern recognition or anomaly detection.
Can you explain the purpose of client segmentation using machine learning techniques?
-The purpose of client segmentation using machine learning is to identify groups of clients with similar characteristics, which can help in understanding the customer base better, discovering unknown patterns, complying with regulations, and improving transaction monitoring.
What are the advantages of using machine learning for client segmentation compared to manual methods?
-The advantages of using machine learning for client segmentation include higher accuracy with large datasets, automation, scalability, adaptability, personalization of segmentation characteristics, and improved time efficiency.
What are the two clustering algorithms mentioned in the script, and what are their main differences?
-The two clustering algorithms mentioned are k-means and Birch. K-means is simpler and more efficient but can be sensitive to initialization and outliers. Birch is more robust to outliers and better suited for large datasets and high-dimensional data.
Why is data cleaning an essential step in the machine learning process for segmentation?
-Data cleaning is essential because it ensures the quality of the data used for training models. Steps like outlier detection, categorical variable encoding, data normalization, and principal component analysis help to prepare the data for accurate and meaningful analysis.
What are the two methods mentioned for determining the optimal number of segments in a dataset?
-The two methods mentioned for determining the optimal number of segments are the elbow method, which uses the inertia metric, and the silhouette method, which considers cohesion and separation parameters.
What is the role of natural language processing (NLP) in risk management applications?
-In risk management, NLP can be used for sentiment analysis to understand customer feedback, text classification to categorize risks, and chatbots to interact with clients and provide risk recommendations or information.
What is the significance of GPT in the context of the presented risk management application?
-GPT, or Generative Pre-trained Transformer, is significant because it can generate new text data based on the input provided. In the risk management application, it uses various client-related variables to suggest potential risks, aiding in the identification and mitigation of such risks.
How does the platform use chat GPT to assist clients in identifying potential risks?
-The platform uses chat GPT by inputting variables such as industry, process, and risk system into the model's prompt. GPT then generates responses and recommendations for potential risks based on the pre-trained data it has from the internet.
What are the precautions taken to ensure client data privacy when using chat GPT in the platform?
-To ensure client data privacy, the platform does not provide specific client information to chat GPT. Instead, it uses general variables like industry and process, relying on the model's pre-trained data to generate risk recommendations.
Outlines
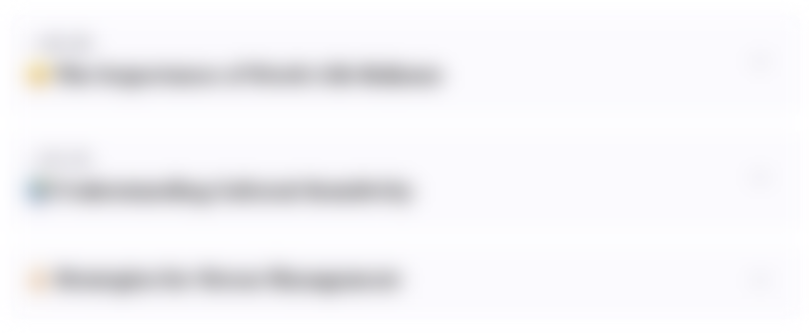
This section is available to paid users only. Please upgrade to access this part.
Upgrade NowMindmap
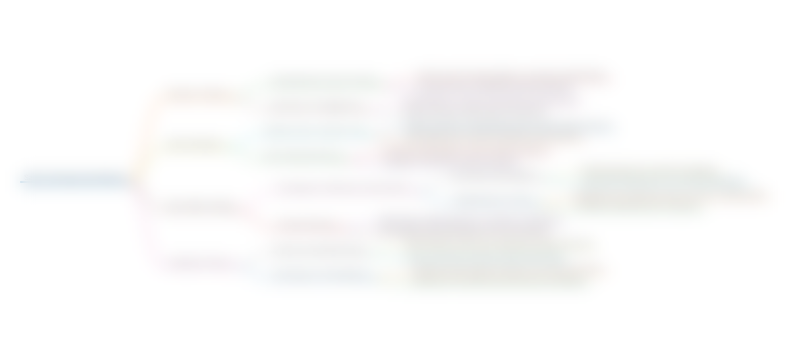
This section is available to paid users only. Please upgrade to access this part.
Upgrade NowKeywords
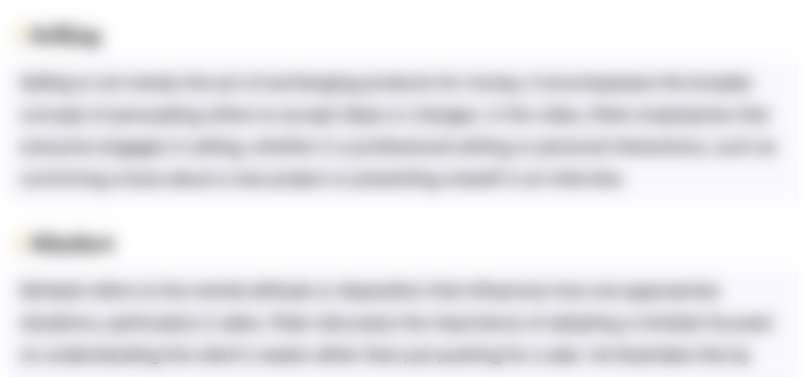
This section is available to paid users only. Please upgrade to access this part.
Upgrade NowHighlights
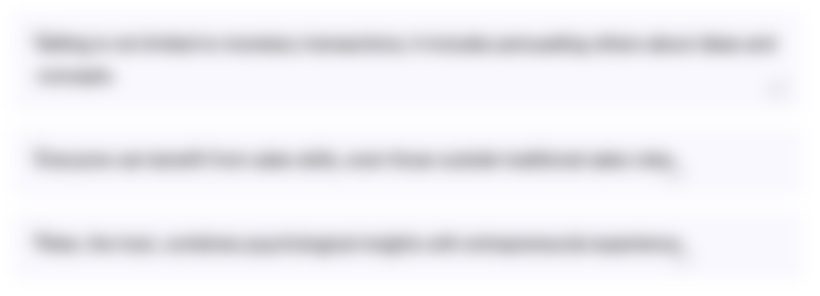
This section is available to paid users only. Please upgrade to access this part.
Upgrade NowTranscripts
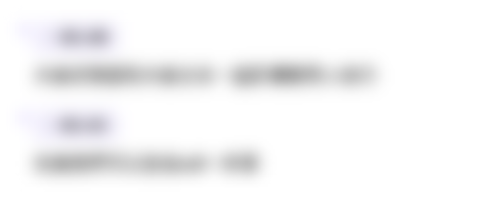
This section is available to paid users only. Please upgrade to access this part.
Upgrade NowBrowse More Related Video
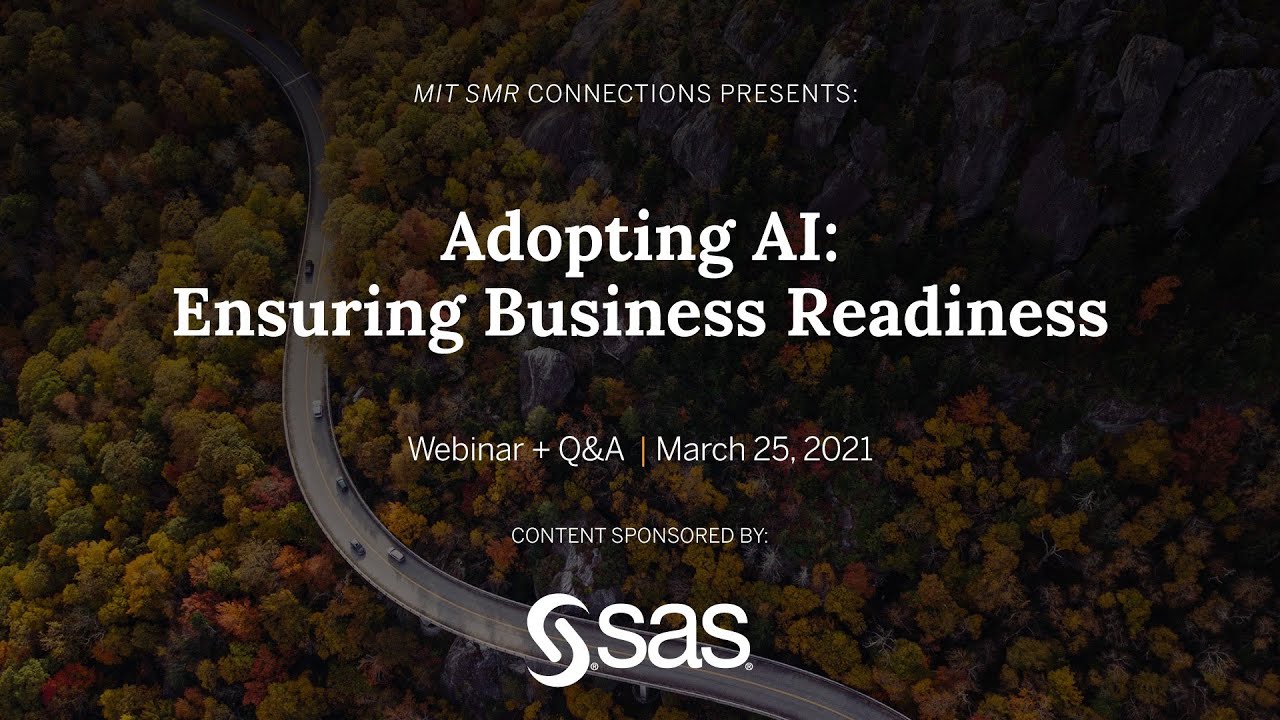
Adopting AI: Ensuring Business Readiness
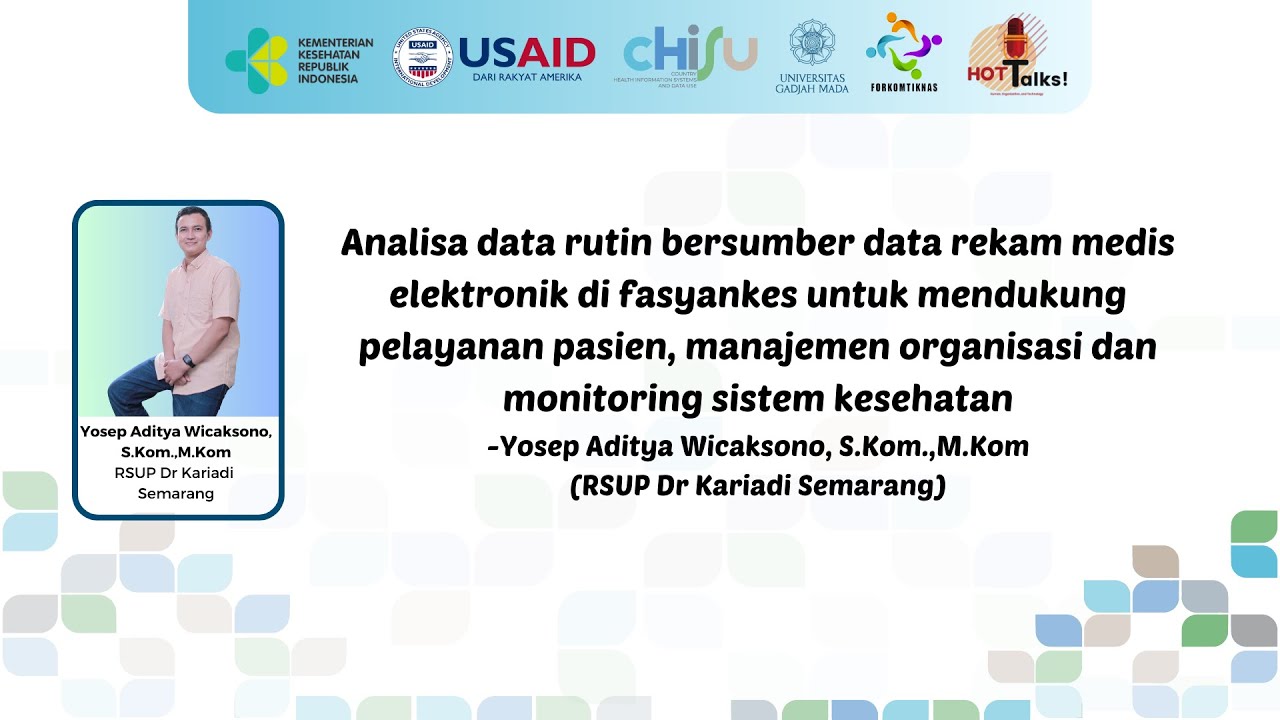
#9 Analisa data rutin bersumber data rekam medis elektronik di fasyankes - Yosep Aditya Wicaksono

We Are Blue Yonder. End-to-end Supply Chain Management Software
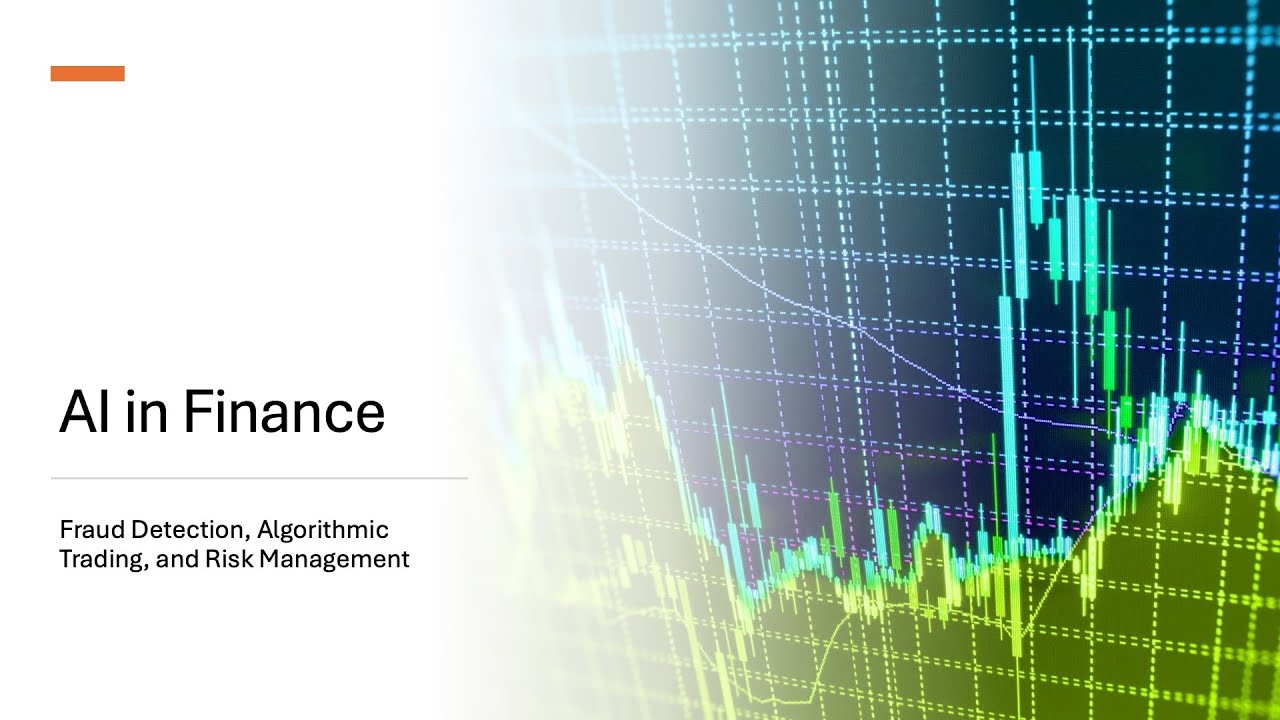
AI in Finance - Fraud Detection, Algorithmic Trading, and Risk Management

Olahraga dan Artificial Intelligence SPORTS AI

How AI is Revolutionizing Finance and Accounting
5.0 / 5 (0 votes)