Adopting AI: Ensuring Business Readiness
Summary
TLDRThe webinar 'Adopting AI: Ensuring Business Readiness' discusses the importance of artificial intelligence in organizational futures. It explores挑选合适的问题用AI解决, launching AI initiatives, and the need for oversight and understanding of AI risks. Industry experts from diverse fields, including healthcare, share insights on AI use cases, challenges, and strategies for successful AI integration, emphasizing the need for robust data infrastructure, a skilled workforce, and compliance with evolving regulations.
Takeaways
- 🤖 AI algorithms are abstract and probabilistic, making them complex and imprecise by nature.
- 🔍 'Explainable AI' techniques can shed light on AI algorithms' predictions but cannot determine fairness or justice.
- 🧠 AI systems are adaptive and learn from data, but lack creativity and may revert to known patterns in unforeseen circumstances.
- 📈 AI systems are typically deployed at scale, which can magnify small errors and require robust feedback loops.
- 👀 AI algorithms are impressionable and know only what they've been exposed to during training and production.
- 🚫 AI algorithms can inadvertently pick up and reinforce biases present in the training data.
- 🛠️ It's crucial to define operating conditions for AI systems and engineer safety controls into their processes.
- 💡 AI solutions must be adopted and scaled effectively, with a focus on value creation and strategic alignment.
- 🌐 AI and machine learning in healthcare hold great promise for predictive analytics, personalized care, and efficiency improvements.
- 🧬 Healthcare data is vast and complex, requiring advanced NLP technologies to unlock valuable insights.
- 🔄 The success of AI in healthcare relies on a collaborative effort between data scientists, clinicians, and end-users.
Q & A
What are the six characteristics of AI algorithms that require additional business due diligence?
-The six characteristics are: 1) AI algorithms are abstract with complex inner workings; 2) They are probabilistic systems with imprecise outputs; 3) They are adaptive and respond to changes in data input; 4) They are not creative and revert to known patterns in unforeseen circumstances; 5) They are typically deployed at scale, which can magnify small errors; 6) They are impressionable and learn from the data they are exposed to during training and production.
How can organizations ensure their AI solutions deliver the intended outcomes?
-Organizations can ensure intended outcomes by establishing robust feedback loops, clearly defining operating conditions, engineering safety controls, monitoring actual usage alongside intended use, and educating users on how the application is meant to be used.
What are some challenges in adopting AI solutions in healthcare?
-Challenges include technical issues such as interpretability, bias, and drift, as well as non-technical issues like clinical validation, workflow integration, privacy concerns, data governance, and deciding whether to build or buy AI solutions.
What is the importance of explainable AI in business applications?
-Explainable AI is crucial as it provides insights into which factors most influence an algorithm's predictions. This helps in making informed decisions about whether those factors are fair, just, and aligned with the business's strategic goals.
How can businesses mitigate the inherent risks of AI?
-Businesses can mitigate risks by implementing robust safety controls, investing in explainable AI technologies, ensuring proper oversight, and maintaining a feedback loop to correct errors and improve the system over time.
What are some best practices for adopting AI solutions at scale?
-Best practices include starting with a clear understanding of business strategy, defining and finding value in AI applications, ensuring the basics are done brilliantly, focusing on people capability and culture, and proceeding responsibly while building trust.
What is the role of AI in enhancing healthcare delivery systems?
-AI can enhance healthcare delivery by providing predictive analytics for disease progression and readmission risks, personalized preventative care, natural language processing for unstructured data, and image recognition for various medical imaging needs.
How can companies balance the need for speed in AI adoption with the slower process of cultural change?
-Companies can balance speed with cultural change by prioritizing education and awareness about AI across the organization, leveraging existing talent, and fostering partnerships between industry and academia to develop a skilled workforce.
What are some strategies for finding the right AI talent?
-Strategies include looking within the organization for potential talent, partnering with academic institutions, investing in internal training programs, and considering a mix of STEM and SHAPE (sociology, humanities, ethics, sustainability) skills.
How can organizations prepare for and comply with international, regional, and national regulations regarding AI?
-Organizations should have a dedicated team or individual to understand and monitor regulatory changes, engage with regulators, anticipate future compliance needs, and integrate legal and ethical considerations into AI development and deployment.
What are some indicators of data-science maturity within an organization?
-Indicators of data-science maturity include the ability to build and implement AI solutions that improve quality, service, cost, and provider sustainability, as well as the capacity to communicate effectively with end-users and stakeholders.
Outlines
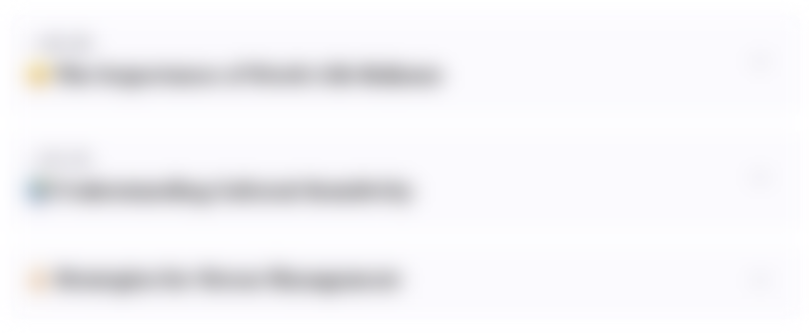
This section is available to paid users only. Please upgrade to access this part.
Upgrade NowMindmap
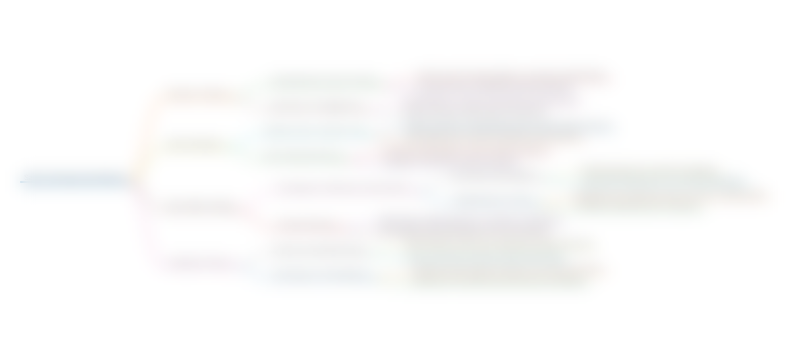
This section is available to paid users only. Please upgrade to access this part.
Upgrade NowKeywords
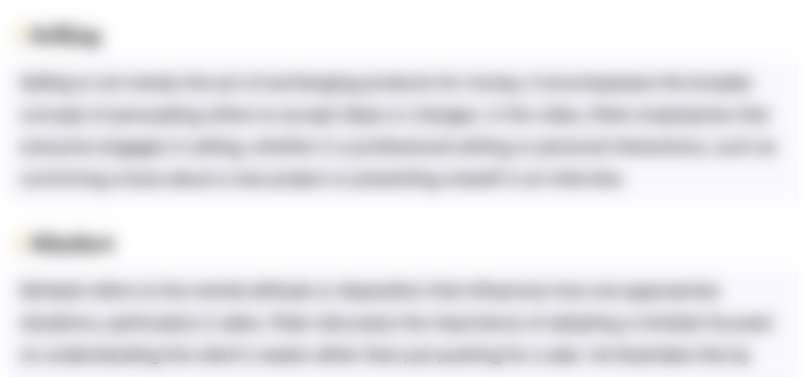
This section is available to paid users only. Please upgrade to access this part.
Upgrade NowHighlights
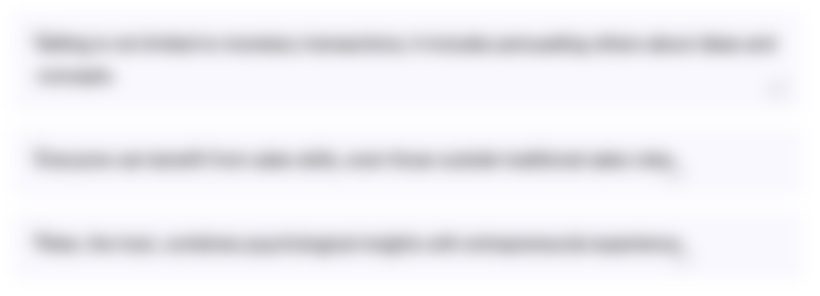
This section is available to paid users only. Please upgrade to access this part.
Upgrade NowTranscripts
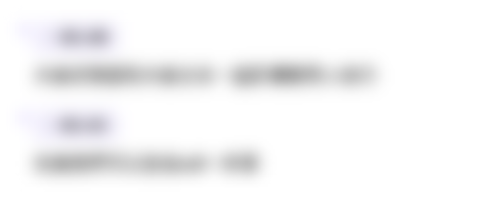
This section is available to paid users only. Please upgrade to access this part.
Upgrade NowBrowse More Related Video
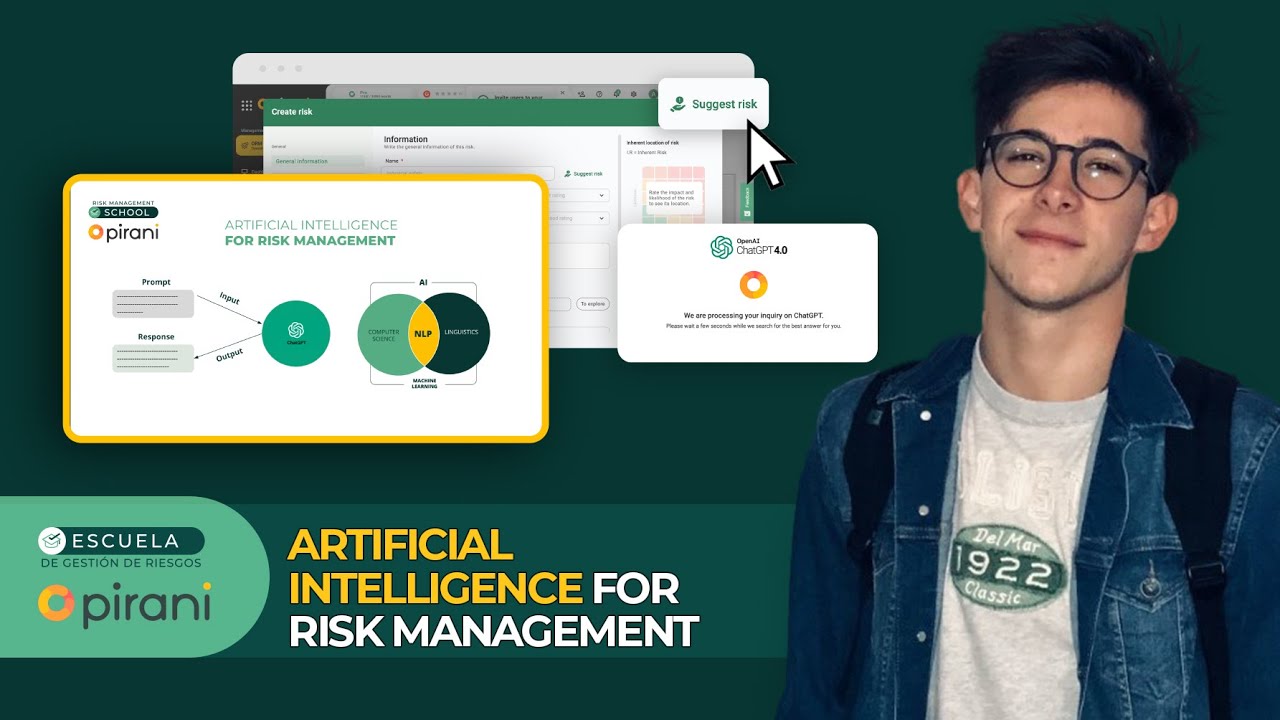
How works Artificial Intelligence for risk management

IA's Generativas - Aula 7
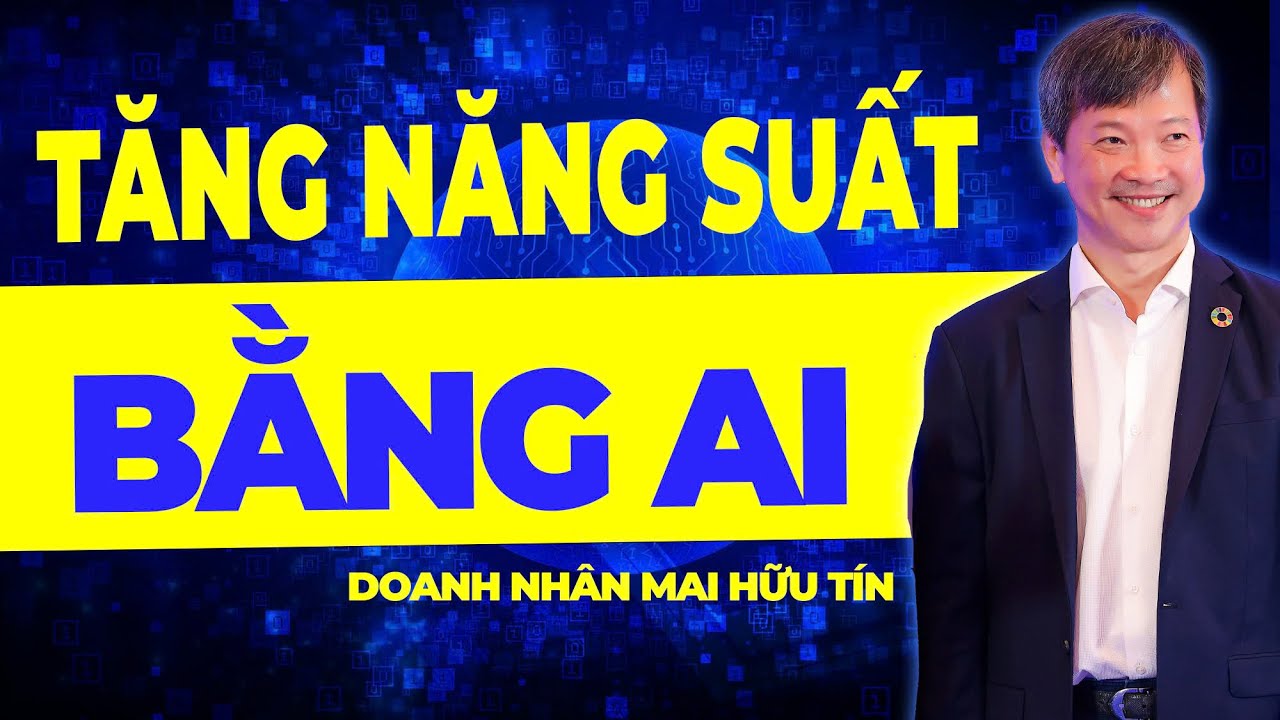
Doanh nhân Mai Hữu Tín: Tăng năng suất bằng AI| Bình Giấy
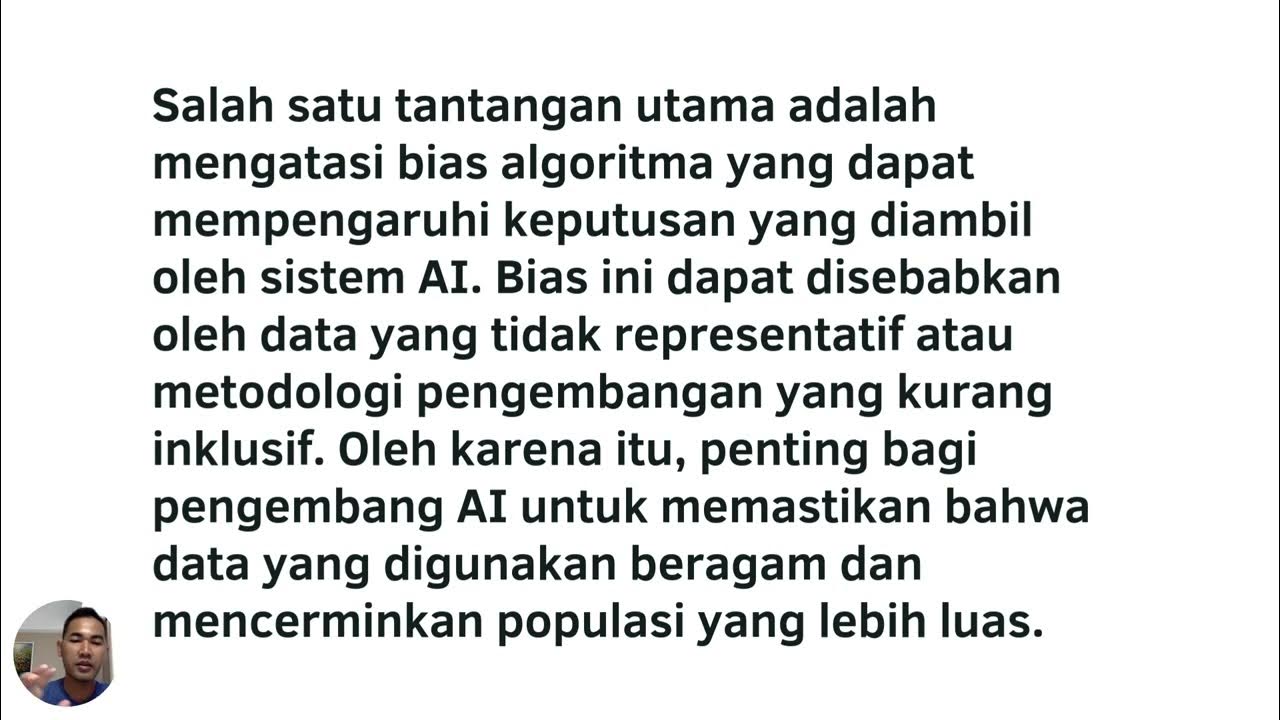
Etika dan Regulasi Penggunaan Kecerdasan Buatan AI Presentation
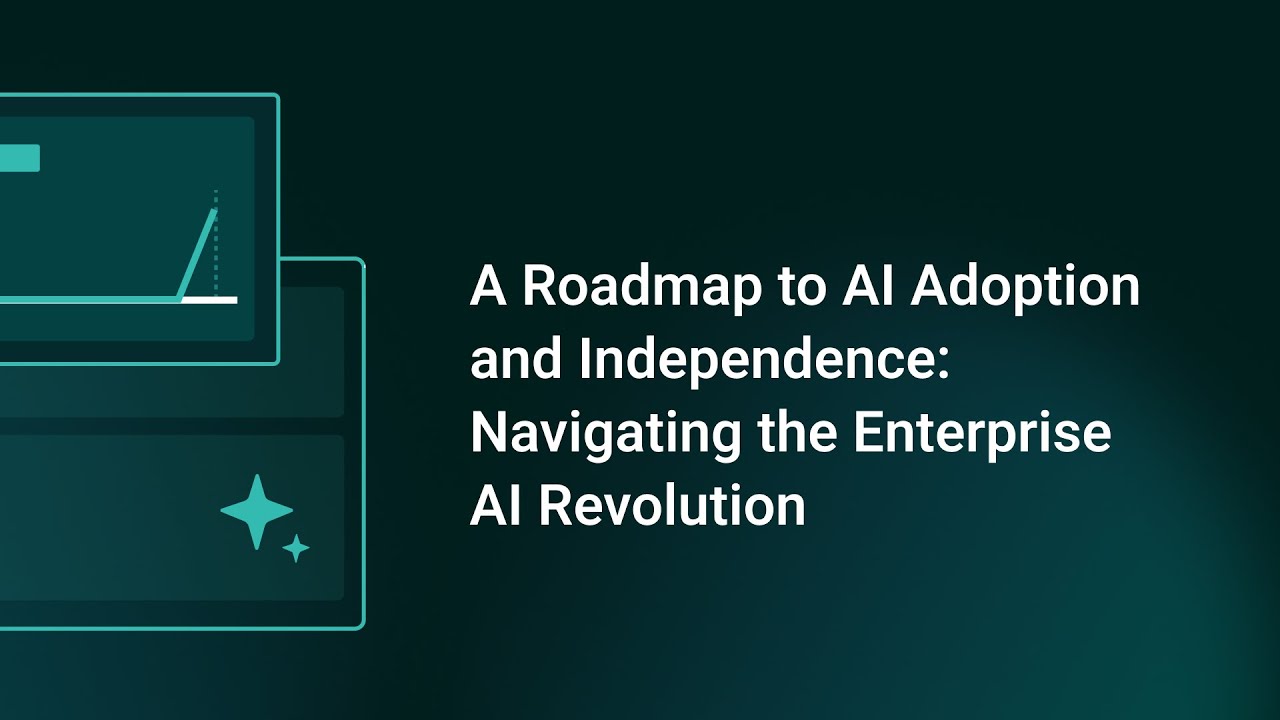
A Roadmap to AI Adoption and Independence: Navigating the Enterprise AI Revolution
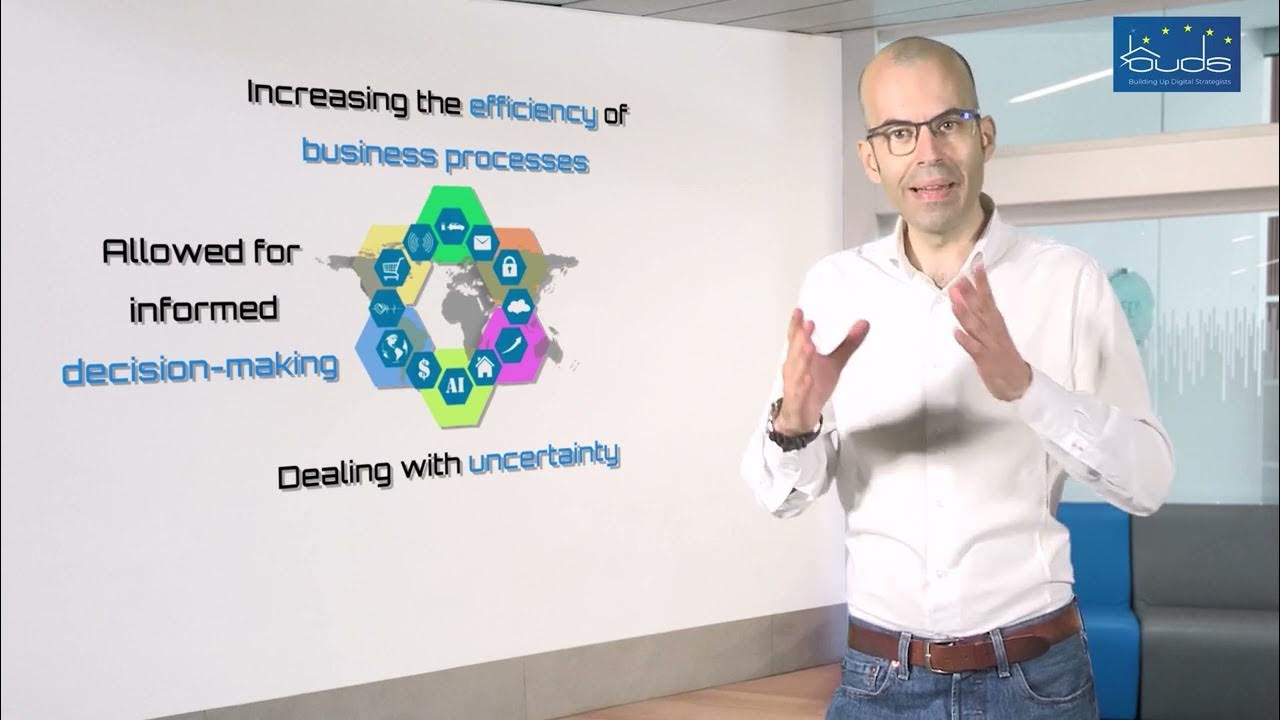
1.3.4. Artificial Intelligence for Digital Transformation: Advantages and Challenges
5.0 / 5 (0 votes)