e Energy 2024 S4P5 Knowledge Guided Learning of Temporal Dynamics and its Application to Gas Turbine
Summary
TLDRThis talk explores the concept of knowledge-guided machine learning, with a focus on modeling gas turbines for energy systems. By combining domain knowledge with machine learning, the speaker demonstrates how to create efficient, sustainable models that require less data while improving performance. The innovative approach uses a custom loss function to enforce permissible system states, such as ramps in turbine output, and ensures better predictions even with limited data. The method offers a more sustainable, data-efficient alternative to traditional approaches, making it ideal for modeling complex dynamic systems like gas turbines in energy grids.
Takeaways
- 😀 Small, application-specific models are more sustainable and effective in many AI applications, especially in energy systems.
- 😀 Knowledge-guided machine learning (KGML) can significantly improve the performance of machine learning models by leveraging domain-specific knowledge.
- 😀 Gas turbines are critical in energy grids, acting as a backup to renewable energy sources like wind and solar when supply is low.
- 😀 Machine learning models can be used to simulate complex systems like gas turbines, but they may need to be guided by domain knowledge to perform accurately.
- 😀 Traditional modeling approaches, such as the Rowan model, fail when only input-output data is available, highlighting the need for data-driven machine learning approaches.
- 😀 Loss functions in machine learning models can be enhanced by adding knowledge-guided constraints, allowing the model to learn more effectively with less data.
- 😀 The concept of 'permissible system states' in the context of dynamic modeling helps guide the model towards acceptable behaviors, like specific ramp states in gas turbines.
- 😀 The use of 'minimum function as a selector' in the loss function helps the model identify the most suitable permissible state during training, optimizing predictions.
- 😀 Injecting domain knowledge into machine learning models can reduce the amount of training data required, leading to faster convergence and more sustainable models.
- 😀 Sensitivity analysis is crucial when applying domain knowledge in machine learning models to ensure robustness against incorrect or incomplete knowledge.
- 😀 In cases where domain knowledge is incorrect, the model's performance may degrade, emphasizing the importance of validation and tuning to ensure the model adapts appropriately.
Q & A
What is the main focus of the talk?
-The talk focuses on how to apply knowledge-guided machine learning to model complex systems like gas turbines, emphasizing the benefits of smaller, application-specific models for sustainability and efficiency.
How are small, application-specific models more sustainable than larger models?
-Small models are more sustainable because they require less data, converge faster, and are easier to maintain and deploy in real-world applications, making them more resource-efficient.
Why are gas turbines important in energy systems?
-Gas turbines are crucial in energy systems as they serve as backup power sources when renewable energy from wind or solar is insufficient. They are quick to start, flexible in fuel usage, and can operate with low-emission fuels like hydrogen.
What is the challenge of modeling gas turbines traditionally?
-Traditional physical models, such as the Rowan model, are not effective for modeling gas turbines as black-box systems because they require detailed measurements of every component, which isn't feasible with limited input-output data.
How does knowledge-guided machine learning help in modeling gas turbines?
-Knowledge-guided machine learning incorporates domain knowledge (e.g., ramps in turbine outputs) into the loss function, helping the model to learn and predict behaviors more accurately, especially with smaller datasets.
What is the role of loss functions in this approach?
-Loss functions play a critical role by minimizing the error between the predicted and actual outputs while also incorporating additional domain knowledge as constraints, such as permissible system states (e.g., ramps), to guide the model.
What are 'permissible system states' in the context of the talk?
-Permissible system states refer to the expected behaviors or constraints of the system, such as rising, constant, or falling ramps in gas turbine outputs. These states are used to guide the machine learning model and ensure realistic predictions.
Why is the concept of 'minimum as a selector' important in the model?
-The 'minimum as a selector' is crucial because it helps the model select the closest permissible state from multiple alternatives, ensuring that the model behaves in a realistic and constrained way while learning from data.
What were the empirical results of the knowledge-guided approach?
-Empirical results showed that the knowledge-guided machine learning model outperformed a baseline model without any domain knowledge, achieving lower error rates and requiring less data to produce accurate results.
What is the significance of sensitivity analysis when applying domain knowledge?
-Sensitivity analysis is important because it tests how robust the model is when the provided domain knowledge is incorrect or varies slightly. This ensures that the model can still perform well even if the initial knowledge is not perfect.
Outlines
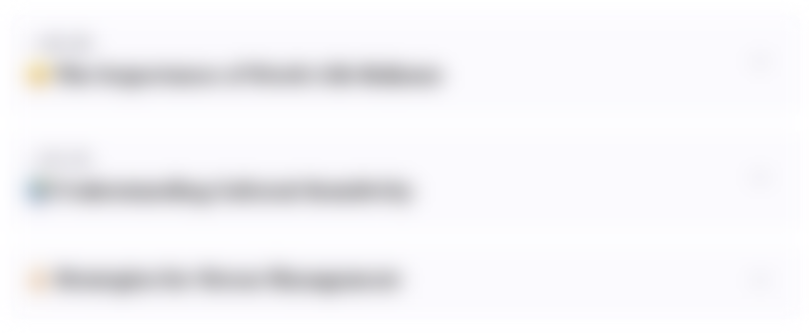
This section is available to paid users only. Please upgrade to access this part.
Upgrade NowMindmap
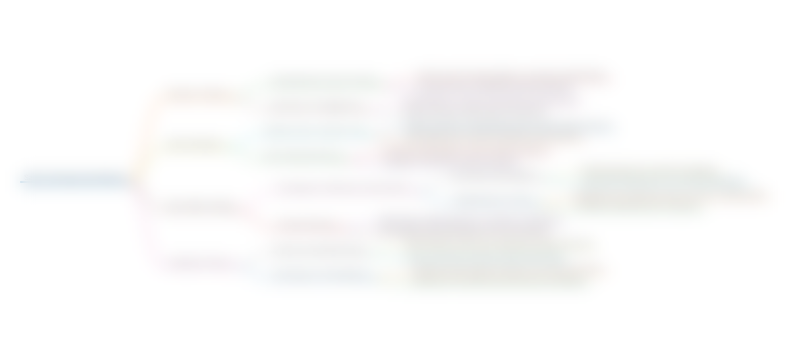
This section is available to paid users only. Please upgrade to access this part.
Upgrade NowKeywords
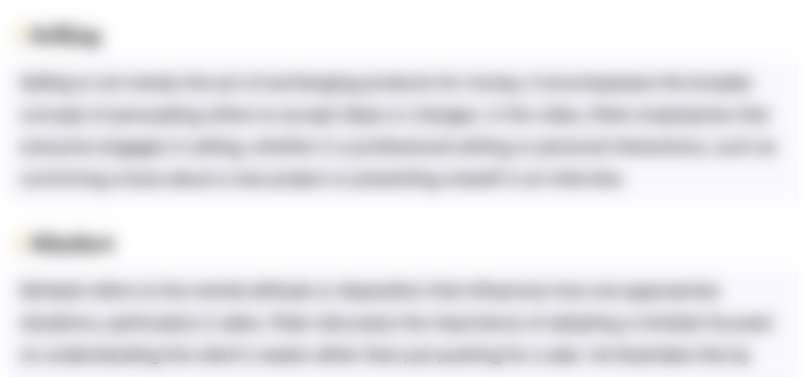
This section is available to paid users only. Please upgrade to access this part.
Upgrade NowHighlights
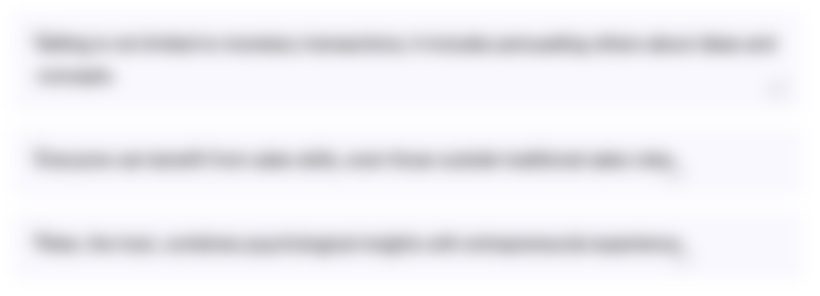
This section is available to paid users only. Please upgrade to access this part.
Upgrade NowTranscripts
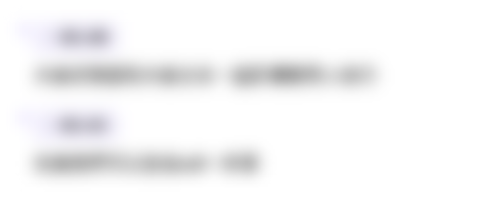
This section is available to paid users only. Please upgrade to access this part.
Upgrade NowBrowse More Related Video
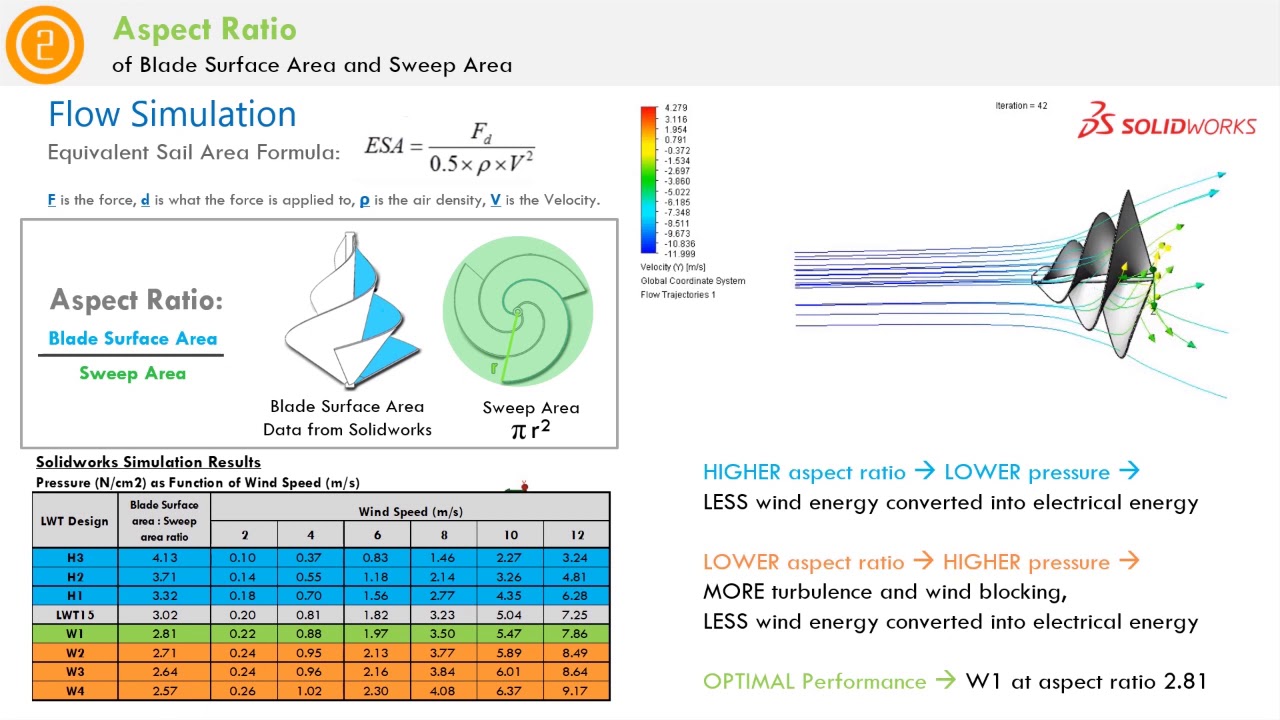
WISPO2020_PE 002_Spira Mirabilis 2 Optimizing the Performance of Logarithmic Spiral Wind Turbine
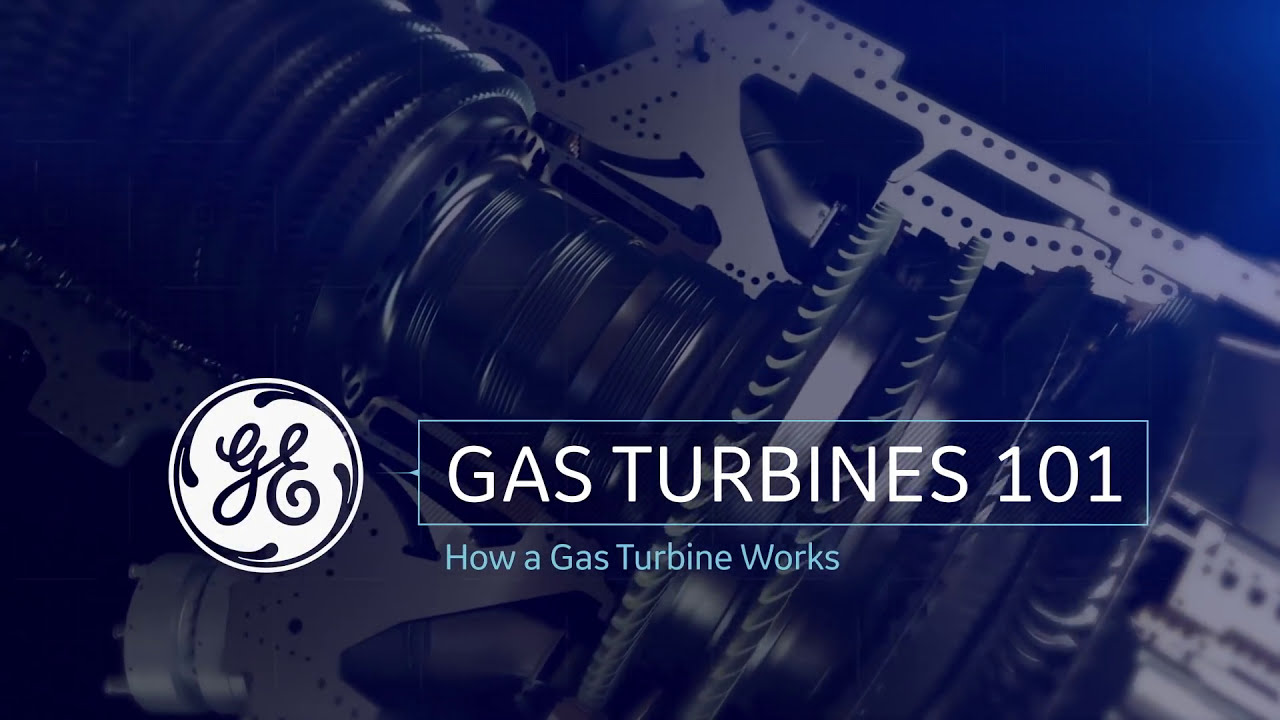
How a Gas Turbine Works | Gas Power Generation | GE Power
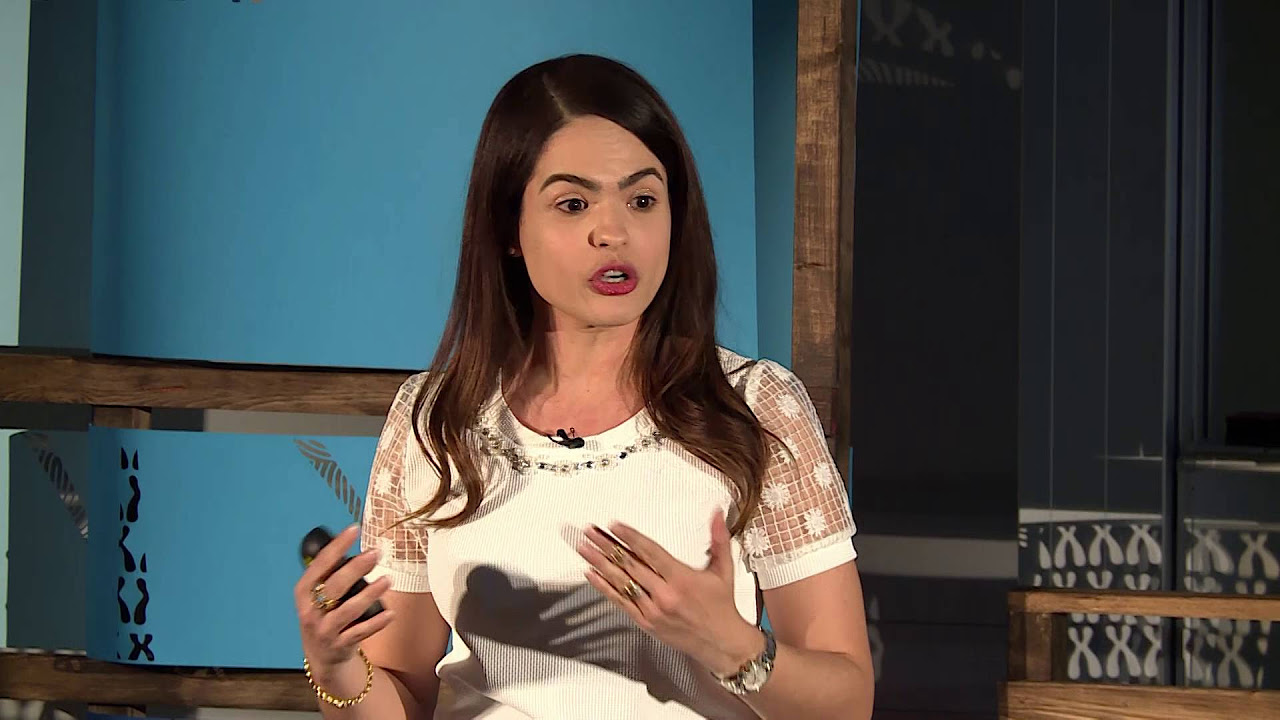
What are Smart Cities? | Larissa Suzuki | TEDxUCLWomen
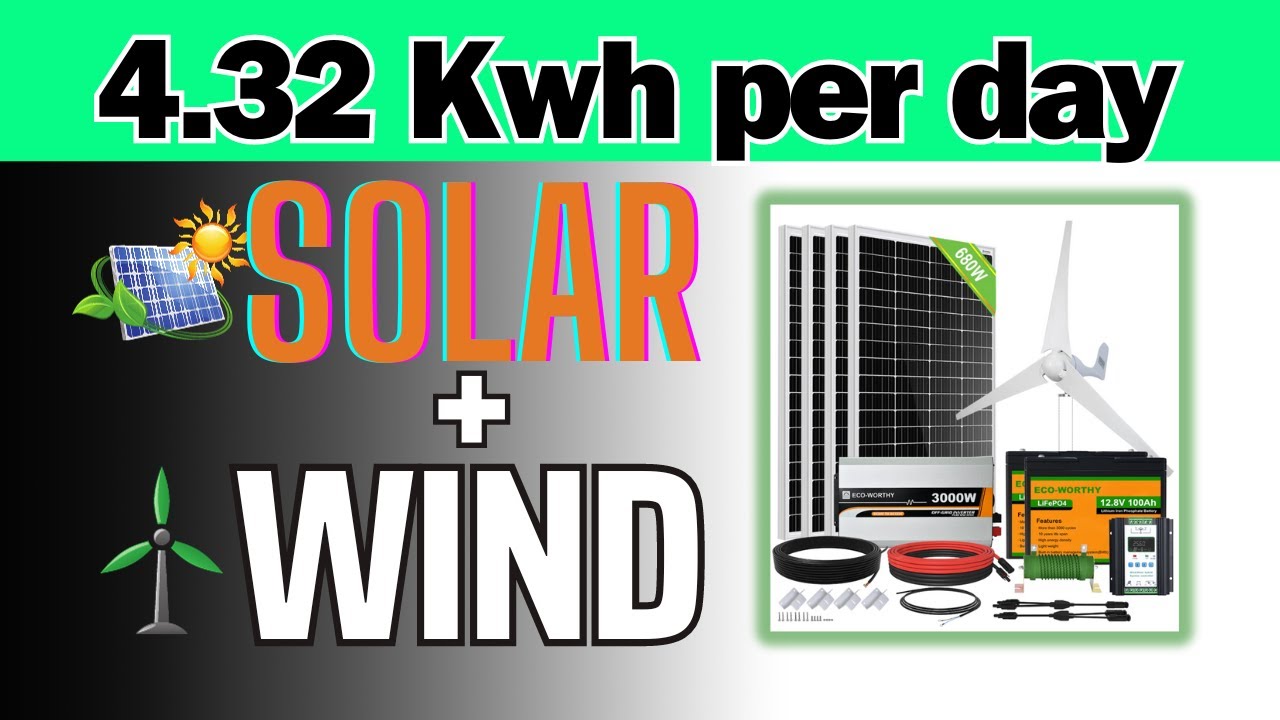
Solar Wind Hybrid System can generate more energy for you | ECO-WORTHY

The Exact Skills and Certifications for an Entry Level Machine Learning Engineer
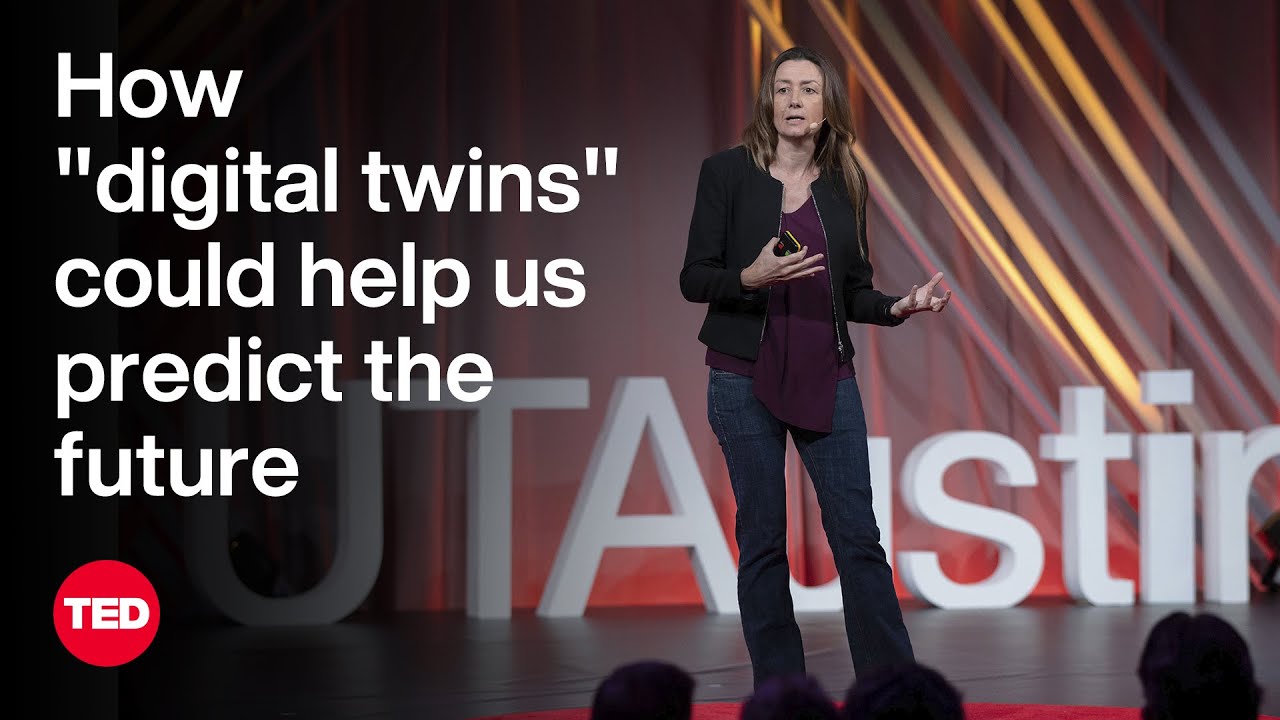
How “Digital Twins” Could Help Us Predict the Future | Karen Willcox | TED
5.0 / 5 (0 votes)