Overcoming the Complexities of Generative AI
Summary
TLDRこのビデオスクリプトでは、NvidiaとWeights & Biasesが共同で開発した新しいツール「Nims」を紹介しています。Nimsは、企業がAIモデルを迅速に展開し、最適化するためのプラットフォームで、言語モデルのデプロイメントを簡素化し、セキュリティとパフォーマンスの両面からサポートします。スクリプトでは、2022年のChat GPTの登場以来のAIの発展とそれに伴う企業のニーズの変化について触れ、2023年に始まった多様なコミュニティモデルの登場とそれに伴う評価、最適化、展開の課題についても説明しています。さらに、NvidiaのAI EnterpriseとWeights & Biasesが提供するソリューションを通じて、これらの課題をどのように克服し、効果的なモデル展開を実現するかについて詳細に紹介しています。
Takeaways
- 🚀 NvidiaはWeights & Biasesツールを利用し、AIモデルの最適化と展開に注力している。
- 📈 2022年にChat GPTが登場し、ビジネス分野における生成型AIの可能性が認められるようになった。
- 🌐 2023年にはコミュニティモデルが登場し、様々なユースケースへの応用が試みられた。
- 🛠️ 2024年にはそれまでの試みから得られた知識をもとに、実際のビジネスアプリケーションへと展開が進んでいる。
- 🔧 NvidiaはAIモデルの展開と推論に関する長い歴史を持ち、ハイパースケーラー企業と協力してモデルの最適化を行っている。
- 💡 企業はAIモデルの展開において、時間やリソースが限られているため、Nvidiaはその問題を解決するソリューションを提供している。
- 🔄 RAG(Retrieval-Augmented Generation)が導入され、ビジネスデータの埋め込みとファクトチェックが可能になった。
- 🔒 コミュニティモデルの登場により、セキュリティやバグフィックス、コードの脆弱性などの新たな問題が生じた。
- 📦 Nvidia Nim(Neural Inference Microservice)は、企業環境におけるAIアプリケーションの展開を簡素化し、制御を維持するプラットフォームとして開発された。
- 🔄 Lauraアダプターを使用することで、異なるユースケースに対して同じベースモデルを使い、より効率的なモデル展開が可能になる。
- 🔧 評価とカスタマイズのためのNemoフレームワークが提供されており、エンドツーエンドのデータ管理、モデル構築、評価、展開が可能となっている。
Q & A
NvidiaはWeights & Biasesツールを使ってどのような成果を上げていますか?
-NvidiaはAIの展開と推論に長い間携わっており、Weights & Biasesツールを使ってAIモデルの最適化を行っています。これにより、多くの人が関与するハイパースケーラー企業と比較しても、企業は短時間でモデルを展開し、推論の遅延を最小限に抑えることが可能です。
2022年に起こった重要なAI技術の出来事とは何ですか?
-2022年に重要な出来事は、Chat GPTの発売でした。これはビジネスが生成的AIを応用する可能性を認識する瞬間であり、AIが画像やビデオだけでなく言語理解にも関わると気づく契機となりました。
生成的AIの実験とコミュニティモデルの登場はどのようにビジネスに影響を与えましたか?
-生成的AIの実験とコミュニティモデルの登場により、ビジネスは自社の適用事例に合わせてモデルを調整できると認識し、モデルの選定、最適化、展開の方法を模索する動きが広まりました。
Nvidia Nim(Nims)とはどのようなプラットフォームですか?
-Nvidia Nimは、汎用性を保ちながらも生成的AIアプリケーションを制御し、データに近い場所で展開できる柔軟性を提供するプラットフォームです。企業環境でのスムーズな運用を可能にし、迅速な統合と展開を促進します。
Nimsはどのようにして企業のAIアプリケーションの展開プロセスを簡素化するのですか?
-Nimsはモデルの最適化、ドメイン固有のコードの提供、カスタム機能のサポート、業界標準APIの利用など、多岐にわたる機能を組み合わせて、企業のAIアプリケーションの展開プロセスを簡素化しています。
LoRAアダプターとは何で、どのような利点がありますか?
-LoRAアダプターは、モデルの重みの完全なサブセットを最適化する代わりに、追加のパラメータの低い秩分解を表す小さなサブセットを最適化するものです。これにより、基本モデルにアダプターを切り替えることで、モデルの用途を素早く変更することができ、多くの用途に対応するより小さな展開が可能になります。
NvidiaはどのようにしてAIモデルの展開においてセキュリティとバグ修正を確保するのですか?
-NvidiaはNimsを介してAIモデルの展開を管理し、ITおよびビジネス部門からの要求に応じてセキュリティ確保、バグ修正、コードの脆弱性への対処などを行っています。これにより、企業はコアビジネスに集中し、非コアの分野に時間を費やすことを避けることができます。
Weights & BiasesはどのようにしてNvidia AI Enterpriseと統合されていますか?
-Weights & Biasesは、Nvidia AI Enterpriseと統合されており、モデルのトレーニング、評価、展開のプロセスを一元管理できるプラットフォームを提供しています。これにより、開発者はカスタマイズされたNimsを簡単に展開し、管理することができます。
Nvidia AI Enterpriseのモデル月曜日とは何ですか?
-モデル月曜日は、Nvidiaが毎週月曜日に新しいモデルをリリースするプログラムです。これにより、開発者は最新のモデルを試して評価し、自分たちのビジネスに適したモデルを選択することができます。
Weights & Biasesのプラットフォームにおける実験の追跡とチームコラボレーションの利点とは何ですか?
-Weights & Biasesのプラットフォームは、実験の追跡とチーム間の協力を容易に行えるように設計されており、実験がどこで実行されているかにかかわらず、一貫性のある追跡エクスペリエンスを提供します。これにより、チームは効果的に協力してプロジェクトを進めることができます。
Outlines
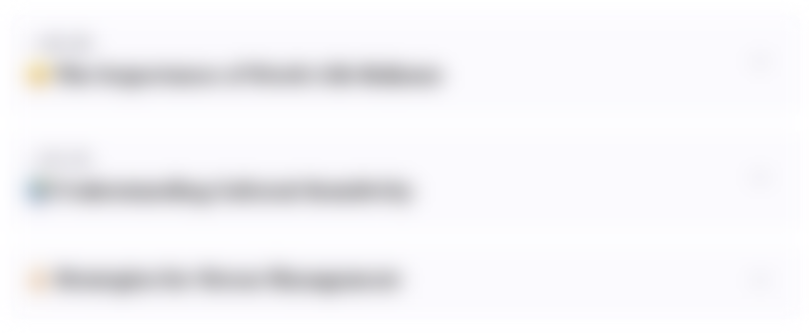
This section is available to paid users only. Please upgrade to access this part.
Upgrade NowMindmap
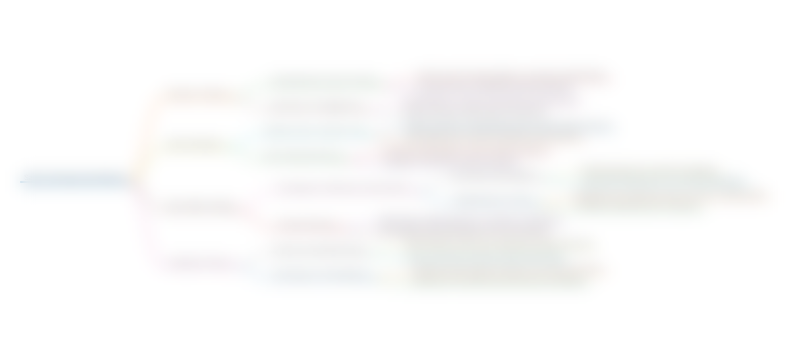
This section is available to paid users only. Please upgrade to access this part.
Upgrade NowKeywords
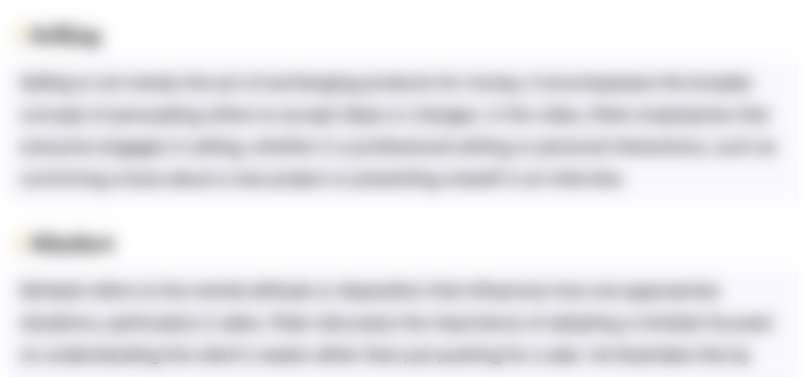
This section is available to paid users only. Please upgrade to access this part.
Upgrade NowHighlights
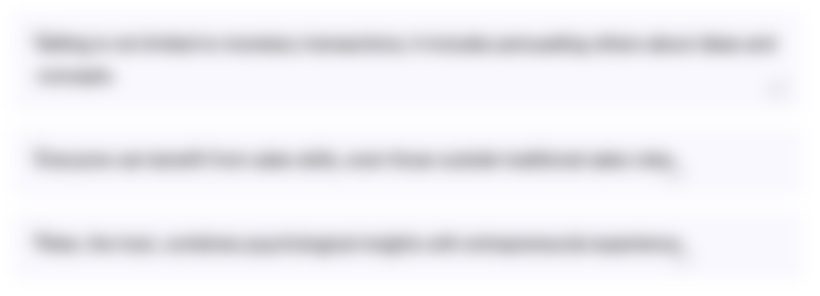
This section is available to paid users only. Please upgrade to access this part.
Upgrade NowTranscripts
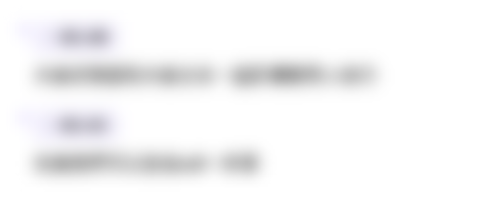
This section is available to paid users only. Please upgrade to access this part.
Upgrade NowBrowse More Related Video
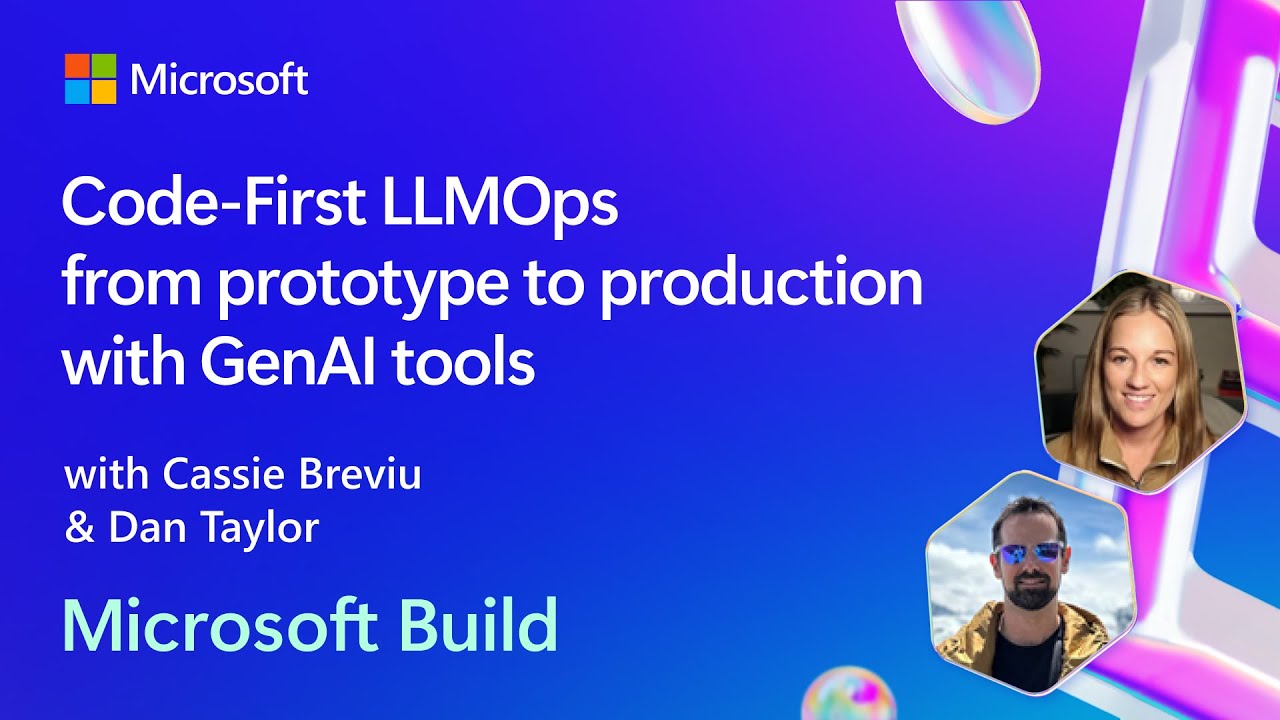
Code-First LLMOps from prototype to production with GenAI tools | BRK110
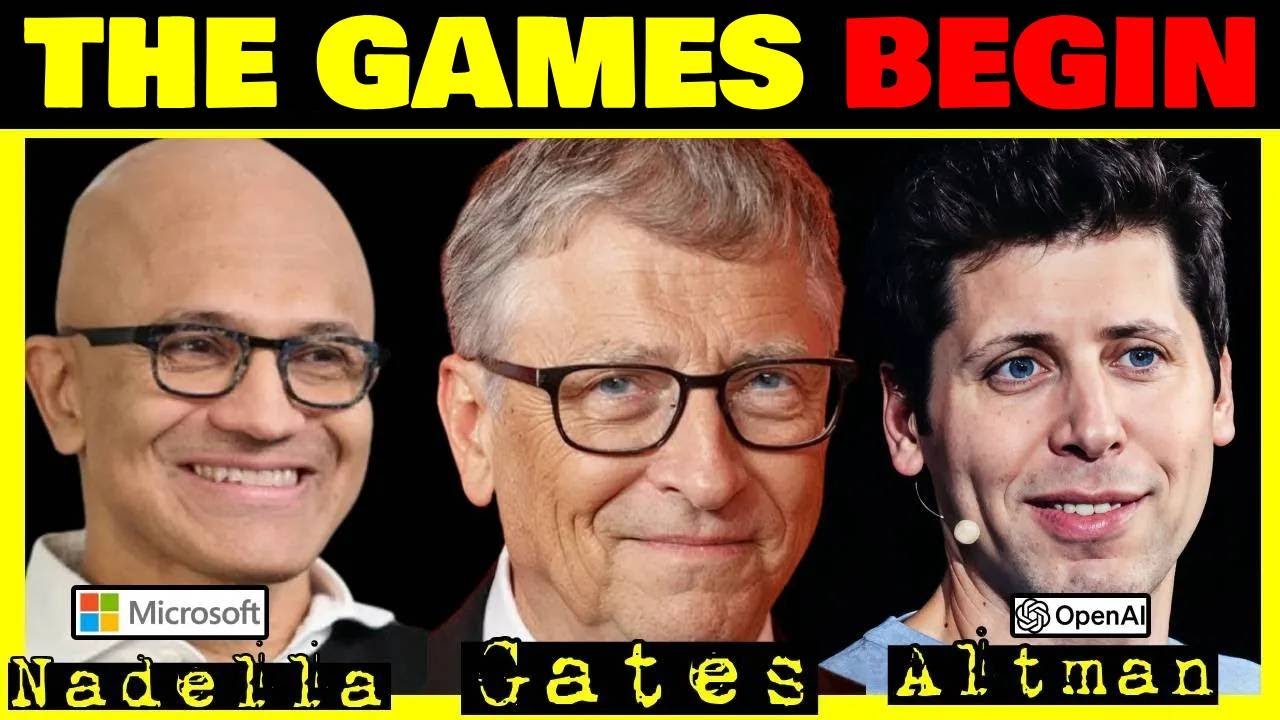
Microsoft's new "Embodied AI" SHOCKS the Entire Industry! | Microsoft's Robots, Gaussian Splat & EMO
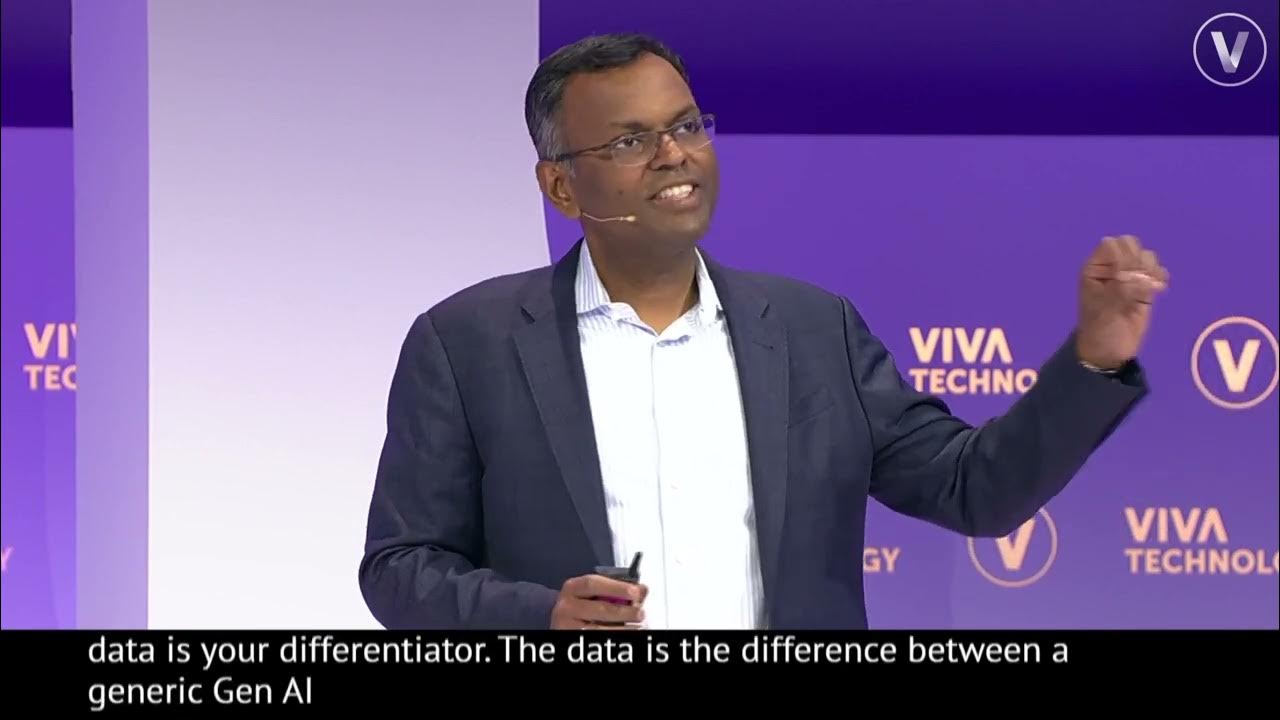
🚀 VivaTech 2024 : Keynote - More than a Model: The Gen AI Essentials for Business Innovation

ChatGPTの大型アップデートを徹底解説セミナー ~新機能:GPTー4o、デスクトップアプリ、音声会話~
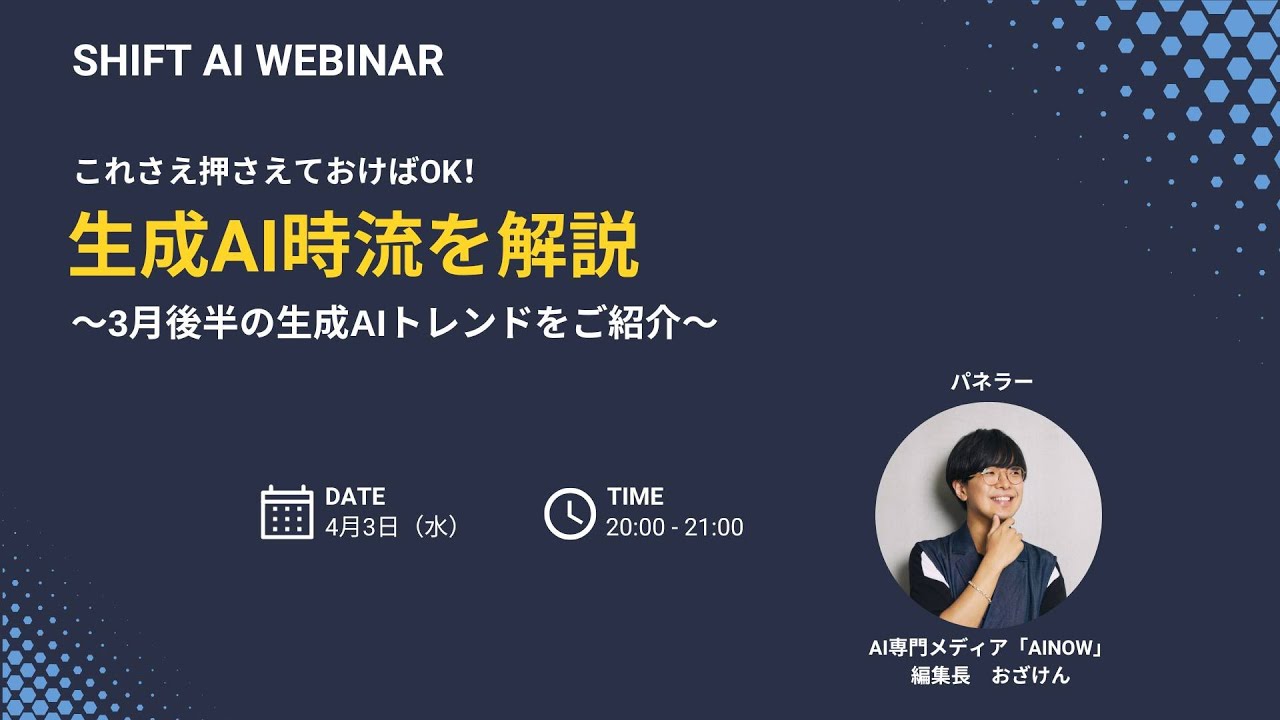
これさえ押さえておけばOK! 生成AI時流を解説 〜3月後半の生成AIトレンドをご紹介〜(2024/04/03)

Exploring the Inner Workings of Azures Advanced AI Infrastructure Presented by Microsoft
5.0 / 5 (0 votes)