What does the second derivative actually do in math and physics?
Summary
TLDR视频脚本深入探讨了物理学家费曼关于“球面上的平均值”的概念,通过直观地理解二阶导数在数学和物理学中的作用,揭示了它在薛定谔方程、电磁学等领域的重要性。视频首先回顾了一阶导数的直观意义,即函数随变量微小变化的速率。随后,通过费曼的“平均在球上”的概念,引入了二阶导数,并解释了它与函数曲率的关系。通过一维和三维的类比,视频推导出了二阶导数的几何意义,并将其应用于电势能函数的推导,直观地导出了麦克斯韦方程之一。此外,视频还联系了量子力学中的动能算符,展示了如何通过二阶导数理解量子现象,尤其是海森堡不确定性原理。最后,视频鼓励观众利用这一理解去构建对其他物理现象的新直觉。
Takeaways
- 📚 费曼在1964年康奈尔大学的讲座中提出了一个概念,即通过了解一个小球体表面的物理量,而不需要考虑外部情况,来理解该点的势能。
- 🎓 视频旨在深入探讨费曼的“球面上的平均值”概念,并发展对数学和物理中二阶导数的直观理解。
- 🔗 哈佛量子计划的量子短片大赛为不同背景的人提供了一个展示量子物理知识的机会,参赛者可以通过视频解释量子物理的某个方面。
- 📈 一阶导数直观地告诉我们,当x发生微小变化时,函数f(x)的变化量。
- 📉 二阶导数通常被理解为一阶导数随输入变化的变化率,但视频中提供了一种更直观的理解方式,即函数在一点的曲率。
- 🧮 通过泰勒级数展开,可以量化函数在x0点附近值的平均值与x0点值的差,这与二阶导数有关。
- 🟢 函数的曲率可以通过“球面上的平均值”来量化,这有助于我们理解二阶导数。
- 🟠 在三维空间中,类似的概念涉及在一个小球面上计算函数的平均值,并与三维空间的二阶导数(拉普拉斯算子)相关。
- ⚡ 利用对二阶导数的直观理解,可以推导出电荷分布产生的电势能函数的微分方程,这与麦克斯韦方程组之一相符。
- 🌌 在量子力学中,动能算符可以表示为位置波函数的二阶导数,即使没有量子力学背景,也能理解其代表的物理意义。
- ⚪️ 高斯波函数的峰值处,周围点的平均值远小于峰值,根据海森堡不确定性原理,这意味着粒子的动量不确定性增加,从而导致动能增加。
- 🤔 视频鼓励观众思考如何利用对二阶导数的理解来构建新的直觉,例如热方程或量子物理中波函数的波长与能量的关系。
Q & A
费曼在1964年康奈尔大学的讲座中提到了什么概念,让当时的本科生感到困惑?
-费曼在讲座中提到了“平均在一个球上”的概念,这个概念让当时的本科生感到困惑,因为它涉及到对函数在某点附近值的平均情况的考量,以及如何通过这种方式来理解势能和量子力学中的某些现象。
在数学和物理学中,二阶导数通常用来描述什么?
-在数学和物理学中,二阶导数通常用来描述函数在某点的曲率,或者用来描述一阶导数随输入变量微小变化的变化率。
费曼是如何使用‘球上的平均’概念来直观理解二阶导数的?
-费曼通过考虑一维空间中某点x0周围的点(构成一个‘球’),计算这些点的函数值的平均数,然后从这个平均数中减去x0点的函数值,以此来直观理解二阶导数。这种方法可以量化函数在一点的曲率。
在量子力学中,薛定谔方程中出现的二阶导数有什么含义?
-在量子力学中,薛定谔方程中的二阶导数与系统的动能有关。通过费曼的‘球上的平均’概念,我们可以将其理解为粒子的波函数在空间中某点周围的平均值与该点波函数值的差,这与粒子的动能相关联。
在多变量微积分中,三维空间中的二阶导数是如何表示的?
-在多变量微积分中,三维空间中的二阶导数使用拉普拉斯算子(Laplacian)来表示,它是二阶偏导数的一种特殊形式,用于描述函数在三维空间中的曲率。
如何使用费曼的‘球上的平均’概念来推导电荷分布产生的电势能函数的方程?
-通过考虑负电荷区域,我们可以推断出,当我们从负电荷区域拉走一个正电荷时,由于吸引力,电荷会获得电势能。类似地,如果我们从正电荷区域拉走一个粒子,由于排斥力,电荷会失去电势能。使用这种直觉,我们可以猜测电势能函数的二阶导数与该点的电荷密度成比例,这实际上就是麦克斯韦方程之一。
量子力学中的位置波函数为高斯函数时,其动能算符的物理意义是什么?
-如果位置波函数是一个高斯函数,这意味着粒子在空间中的位置被很好地局部化。根据费曼的‘球上的平均’概念,我们可以推断出在波函数的峰值处,周围的点的平均值要小得多,因此二阶导数会是一个大的负数。这意味着粒子具有相对较大的动能,这与海森堡不确定性原理相符,即粒子的位置不确定性越低,其动量的不确定性就越高。
为什么在量子力学中,动能算符不仅能测量粒子的速度,还能告诉我们不确定性原理如何影响其运动?
-动能算符在量子力学中的形式是波函数的二阶导数,它不仅反映了粒子的速度,还隐含了位置和动量的不确定性关系。当波函数越局部化,即粒子的位置不确定性越低,其动量的不确定性就越高,从而粒子的动能和运动就会受到更大的影响。
在量子力学中,波函数的局部化程度和动能之间的关系是什么?
-在量子力学中,波函数的局部化程度越小,即粒子的位置不确定性越低,根据海森堡不确定性原理,其动量的不确定性就越高。这种动量的不确定性可以导致粒子具有较大的动能,从而影响粒子的运动。
如何理解热方程中的二阶导数?
-热方程中的二阶导数可以被理解为描述热如何在介质中扩散的量度。类似于费曼的‘球上的平均’概念,它可能表示在一点周围区域的温度平均值与该点温度的差,这与热流和能量传递有关。
在量子物理学中,为什么高能波函数倾向于有更小的波长?
-在量子物理学中,波函数的波长与其能量成反比。根据德布罗意假说,一个粒子的波长与其动量成反比,而动量与动能成正比。因此,高能波函数意味着粒子具有较大的动量,从而导致更小的波长。
费曼是如何通过简单的物理直觉来解释复杂的数学和物理概念的?
-费曼通过使用直观的物理模型和类比来解释复杂的数学和物理概念。例如,他使用‘球上的平均’概念来解释二阶导数,并通过这个概念来推导电荷分布产生的电势能函数的方程,以及量子力学中的动能算符。这种方法使得复杂的理论更加易于理解和可视化。
Outlines
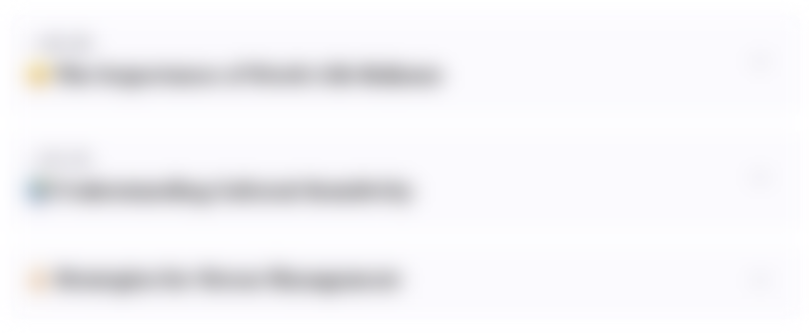
This section is available to paid users only. Please upgrade to access this part.
Upgrade NowMindmap
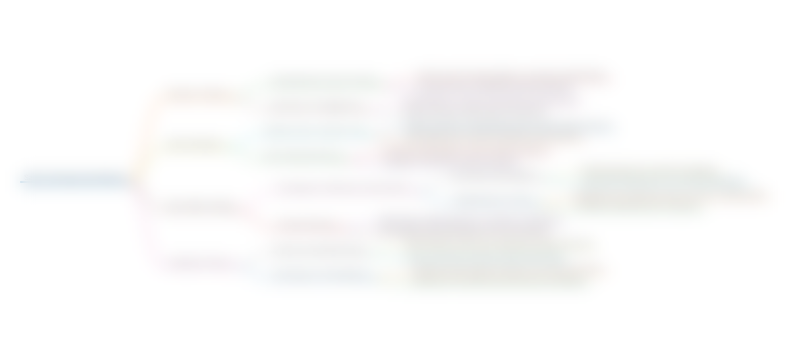
This section is available to paid users only. Please upgrade to access this part.
Upgrade NowKeywords
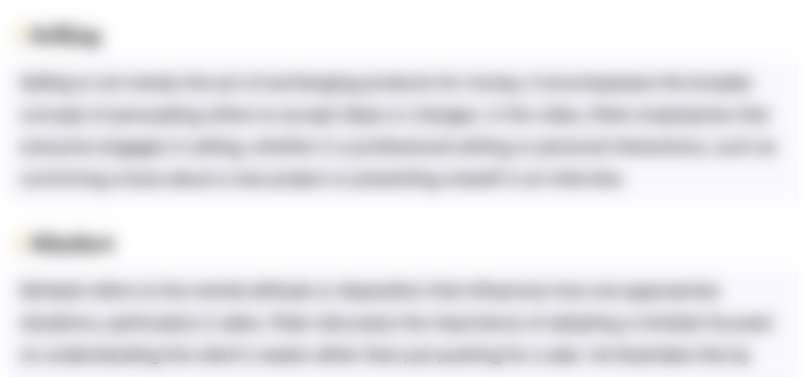
This section is available to paid users only. Please upgrade to access this part.
Upgrade NowHighlights
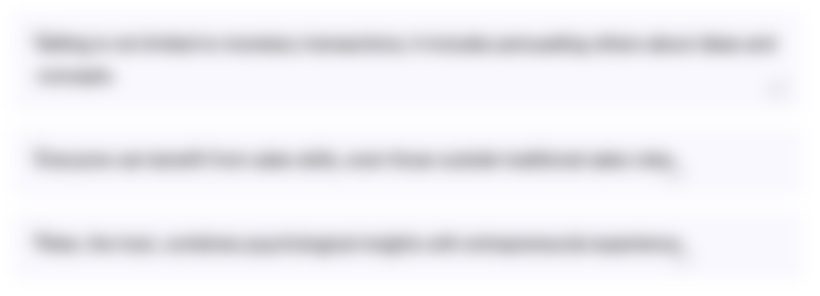
This section is available to paid users only. Please upgrade to access this part.
Upgrade NowTranscripts
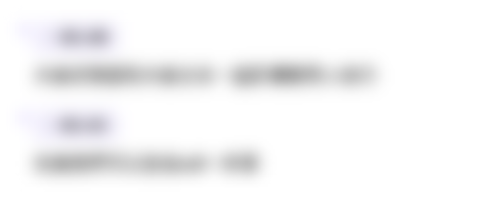
This section is available to paid users only. Please upgrade to access this part.
Upgrade Now5.0 / 5 (0 votes)