5. From Panic to Suffering
Summary
TLDR在这段视频脚本中,马文·明斯基教授深入探讨了意识和人工智能(AI)的复杂关系。明斯基教授首先提到,一些学者如史蒂夫·平克认为意识问题是AI领域中最重要的问题。他提出,如果不能解决意识的谜题,我们构建的AI系统可能会缺少某些重要的属性。接着,明斯基教授对罗杰·彭罗斯的观点进行了讨论,彭罗斯认为量子力学和哥德尔不完全性定理是AI无法实现的原因。明斯基教授还讨论了关于意识的不同观点,特别是关于“感受质”(qualia)的问题,即不同感官体验之间的区别。他通过举例说明,意识可能不是一个单一的大问题,而是许多小问题的集合。此外,明斯基教授还提出了关于计算机重启时间的问题,以及为什么计算机不能记住上次的状态,这涉及到了计算机科学中的一些有趣问题。最后,他以对意识的进一步思考结束,提出了关于人类意识和机器意识之间可能存在的相似性和差异的思考。整个讨论涵盖了哲学、认知科学和计算机科学等多个领域,展现了明斯基教授对这些复杂问题的深刻见解。
Takeaways
- 🤖 人工智能领域的一些专家,如Steve Pinker,认为意识问题是人工智能中最重要的问题。
- 🧐 Marvin Minsky提到,有些AI怀疑者,如物理学家Penrose,认为由于量子力学和哥德尔不完备定理等因素,人工智能是不可能的。
- 😕 Pinker对于机器如何拥有感受(qualia)表示怀疑,特别是机器能否体验到看红色和看绿色不同的感受。
- 🔍 Minsky认为意识不是一个单一的大问题,而是许多不同的小问题,每个问题都可以通过编程的方式来探索解决方案。
- 🚦 在讨论意识时,Minsky提出了一个关于Joan过马路时决策过程的例子,说明意识可能涉及多种不同的心智活动。
- 🧠 他提出大脑中可能存在不同的“自我模型”,它们在任何时候都在执行不同的任务,如讲述故事、制作视觉表示等。
- 💡 Minsky讨论了颜色感知的问题,指出颜色的感知并不仅仅取决于一个区域的颜色,而是该区域与附近区域颜色的差异。
- 📈 他批评了统计学习方法,认为它只能解决简单的问题,而在面对复杂问题时则显得无能为力。
- 📚 他提到了Solomonoff归纳理论,这是一种考虑所有可能程序来预测数据集的理论,尽管它难以计算,但可以启发人们如何更好地进行预测。
- 👶 Minsky还谈到了儿童心理学和语言学习,强调了儿童发展的重要性,并对当前儿童心理学研究的缺乏表示遗憾。
- 📖 他提到了文学作品和科幻小说的价值,认为科幻小说通过探索不同情感和思维方式的生物,提供了更多的想象空间。
Q & A
MIT OpenCourseWare是如何提供高质量教育资源的?
-MIT OpenCourseWare通过社会人士的捐赠来支持其继续提供免费的高质量教育资源。捐赠者可以通过访问ocw.mit.edu来提供支持或查看来自数百门MIT课程的额外材料。
Marvin Minsky对于意识问题有何看法?
-Marvin Minsky认为意识问题并不是一个单一的大问题,而是可以分解为多个较难的问题。他反对将意识视为一个特殊的、不可解的谜题,并认为通过编程和人工智能的方法可以逐步解决这些问题。
为什么计算机重启需要的时间似乎没有随着硬件速度的提升而减少?
-尽管现代计算机比过去快了1000倍,但重启时间并没有显著减少。观众提出可能是因为计算机需要加载更多的内容。Minsky教授提出疑问,为什么不能加载上次运行的内容,而是每次都重新加载所有内容。
Marvin Minsky对于统计学习的看法是什么?
-Minsky教授认为统计学习在处理具有大量小影响因素的简单问题上很有用,但它并不适用于需要智能假设的复杂问题。他鼓励研究者们应该寻找更智能的方法来提出关于正在发生事情的假设。
什么是Solomonoff归纳,它与统计学习方法有何关联?
-Solomonoff归纳是一种理论,它假设所有数据都是由程序生成的,并且使用程序的描述长度作为可能程序空间的先验。这与统计学习方法相关,因为它们都涉及到根据数据集做出预测,但Solomonoff归纳更侧重于找到最简洁的程序来生成数据集。
为什么Marvin Minsky认为语言不太可能是基于语法的?
-Minsky教授认为语言不太可能完全基于语法,因为语法无法充分解释语言的递归性和复杂性。他怀疑语言是基于某些类型的框架操作,这些操作可以更好地适应推理系统。
为什么Marvin Minsky认为情感对于人类智能不是特别重要?
-Minsky教授认为情感主要是为了保持生物的生存,比如通过监测血糖水平来确保进食,但情感并不帮助人类进行复杂的思考或撰写论文。情感在人类智能中的作用被科幻作家过度夸大了。
为什么Marvin Minsky认为孩子们的学习方式对于人工智能的发展很重要?
-Minsky教授认为孩子们如何学习是一个重要的研究领域,因为它可能揭示了人类智能的发展机制。这对于设计能够模拟人类学习过程的人工智能系统至关重要。
Marvin Minsky对于人工智能未来的展望是什么?
-Minsky教授认为人工智能的未来不仅仅是模拟人类智能,而是要超越人类,解决人类不擅长的问题。他强调了创造能够独立思考和推理的智能机器的重要性。
为什么Marvin Minsky认为我们需要超越我们的生物体?
-Minsky教授认为,由于生物体的脆弱性和有限性,例如会生病、死亡和衰老,我们需要将自己转变为智能机器人以超越这些限制。这是为了应对未来挑战,如太阳变成红巨星时的地球不再适宜居住的问题。
Marvin Minsky对于早期教育的看法是什么?
-Minsky教授认为早期教育非常重要,他对于尼克松总统未能投入大量资金进行儿童学习研究表示遗憾。他强调了对儿童如何学习进行研究的价值,并认为这是教育改进的关键。
Outlines
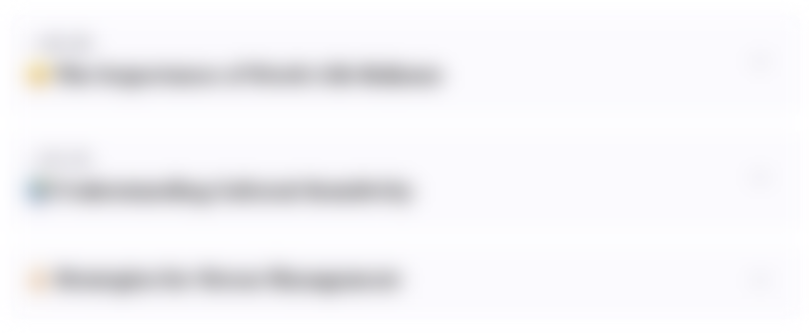
This section is available to paid users only. Please upgrade to access this part.
Upgrade NowMindmap
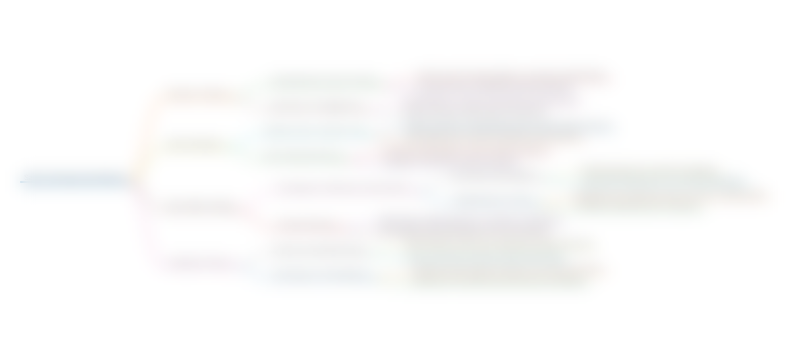
This section is available to paid users only. Please upgrade to access this part.
Upgrade NowKeywords
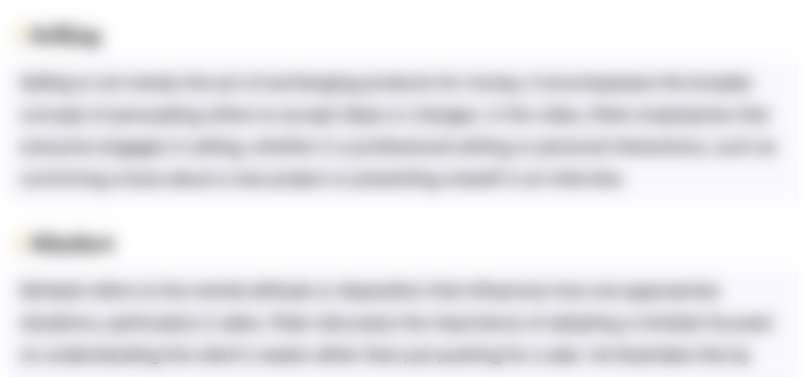
This section is available to paid users only. Please upgrade to access this part.
Upgrade NowHighlights
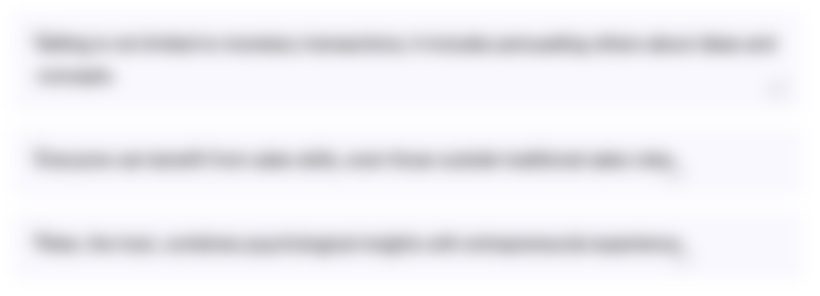
This section is available to paid users only. Please upgrade to access this part.
Upgrade NowTranscripts
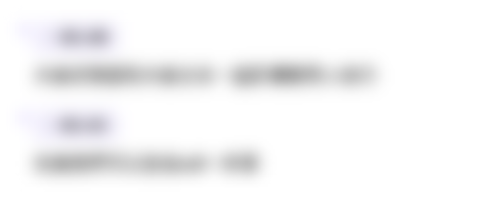
This section is available to paid users only. Please upgrade to access this part.
Upgrade Now5.0 / 5 (0 votes)