2024 Physics Nobel Prize Explained!
Takeaways
- ๐ The 2024 Nobel Prize in Physics was awarded to John Hopfield and Geoffrey Hinton for their groundbreaking work in artificial neural networks and machine learning, advancing the field of computer science using physics principles.
- ๐ Machine learning works differently from traditional software; rather than following pre-defined steps, it learns from examples and adjusts based on neural network knowledge.
- ๐ In 1940, research on the brain showed that neurons have connections, and in 1943, Donald Hebb proposed that these connections could be weak or strong depending on their relationship, laying the foundation for neural network concepts.
- ๐ John Hopfield's work in 1982 on associative memory led to the development of the Hopfield Network, which relies on associative memory for pattern recognition, such as recalling a name by its similarity to another word.
- ๐ Hopfield's physics background played a key role in developing the Hopfield Network, using atomic spin and energy formulas to store and retrieve images by minimizing energy states.
- ๐ The Hopfield Network, inspired by atomic spin in physics, helps in storing multiple images by altering energy states to achieve the lowest possible energy, much like a ball moving to the bottom of a valley.
- ๐ Geoffrey Hinton contributed to the field by solving the issue of image interpretation in Hopfield Networks, leveraging statistical mechanics and the Boltzmann Equation to predict energy states and probabilities.
- ๐ Hinton and colleagues developed the Boltzmann Machine, a neural network model that introduced a hidden layer, allowing the system to learn patterns and interpret images through training and probabilistic reasoning.
- ๐ In 2006, Geoffrey Hinton introduced the pre-training method for neural networks, enhancing efficiency by stacking multiple Boltzmann Machines and simplifying computation with restricted Boltzmann Machines.
- ๐ Although neural networks are inspired by the brain, artificial neural networks are simplified models, using symmetric connections between nodes instead of complex, asymmetric biological networks.
- ๐ Machine learning advancements have revolutionized fields like particle physics, protein structure analysis, and gravitational wave detection, demonstrating the practical applications of artificial neural networks in both physics and engineering.
Q & A
Why did the 2024 Nobel Prize in Physics go to John Hopfield and Geoffrey Hinton despite their contributions to artificial intelligence and machine learning?
-The Nobel Prize was awarded to John Hopfield and Geoffrey Hinton for their groundbreaking work that used physics foundations to advance the fields of artificial neural networks and machine learning. While these fields are traditionally associated with computer science, the methods they developed were based on fundamental principles of physics, particularly the second law of thermodynamics and the study of atomic spins.
What is the fundamental difference between software and machine learning?
-Software follows a set of predefined steps (like a recipe) to achieve a specific outcome, such as baking a cake. In contrast, machine learning involves providing examples to a model, which then learns from these examples and can make predictions or decisions without following specific instructions for every scenario.
How did John Hopfield contribute to the field of artificial neural networks?
-John Hopfield made significant contributions by developing the Hopfield network in 1982. His work focused on associative memory, where a system can recall information based on partial or incomplete input. This concept played a key role in advancing neural networks.
What was the role of Hopfield's knowledge of physics in his work on neural networks?
-Hopfield applied his knowledge of physics, particularly from molecular biology and thermodynamics, to the study of neural networks. By drawing parallels between atomic spins and neural connections, he introduced concepts like energy states and the lowest energy configurations to model how neural networks could process information.
What is associative memory in the context of Hopfield's work?
-Associative memory refers to the ability to recall information based on partial or related inputs. For example, if you forget someone's name but can recall a similar-sounding word, the system uses that connection to help you eventually remember the correct name, which is the principle behind Hopfield networks.
How does Hopfield's network work with incomplete images?
-Hopfield's network uses energy minimization to complete an image. When given an incomplete image, the network adjusts the connections between neurons to find the lowest energy state, gradually filling in missing parts of the image until it matches a stored pattern.
What is the Boltzmann machine, and how does it relate to Hopfield's network?
-The Boltzmann machine, developed by Geoffrey Hinton and his colleagues, is an extension of Hopfieldโs network. It incorporates probabilistic reasoning to determine which energy states are more likely. This machine includes a hidden layer, which helps in training the system to recognize and interpret patterns in a more efficient manner.
What improvements did Geoffrey Hinton bring to the field of neural networks?
-Geoffrey Hinton improved upon earlier models by developing methods like the restricted Boltzmann machine in 2006, which allowed for more efficient training by reducing the computational load. He also introduced the concept of pre-training neural networks, which further enhanced machine learning capabilities.
What is the significance of the second law of thermodynamics in neural networks?
-The second law of thermodynamics, which states that systems tend to move towards a state of lower energy, is crucial to the functioning of neural networks. This principle was applied in Hopfield's network to help find the optimal solutions by minimizing energy states, thus enabling the network to learn and recognize patterns.
How did the development of machine learning influence fields beyond computer science?
-Machine learning, through its foundational physics principles, has significantly impacted areas such as particle physics, protein structure analysis, gravitational wave detection, and more. Its ability to process large datasets and recognize patterns has revolutionized multiple scientific fields, including physics.
Outlines
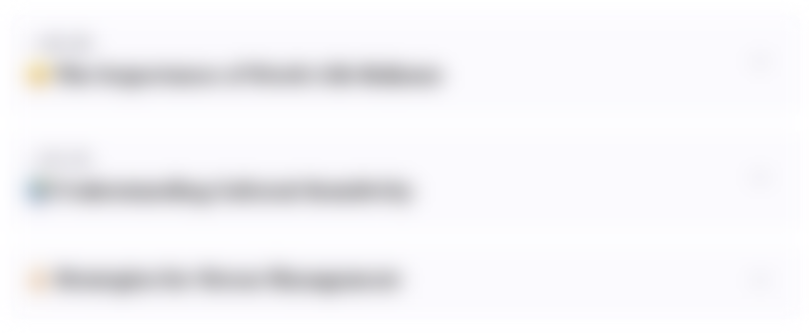
This section is available to paid users only. Please upgrade to access this part.
Upgrade NowMindmap
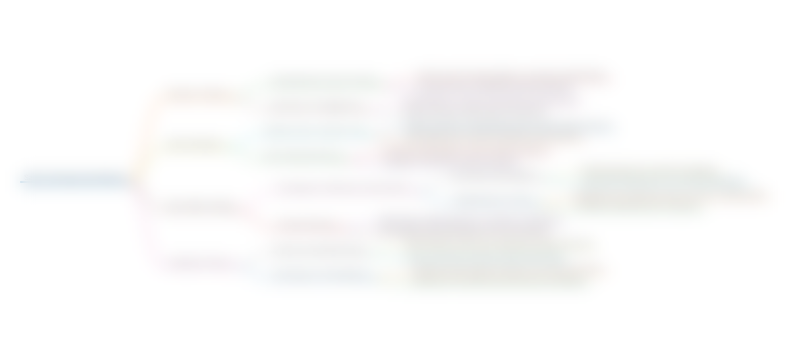
This section is available to paid users only. Please upgrade to access this part.
Upgrade NowKeywords
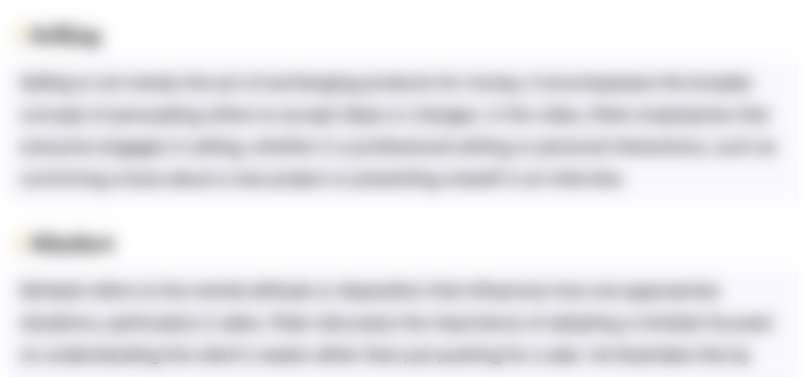
This section is available to paid users only. Please upgrade to access this part.
Upgrade NowHighlights
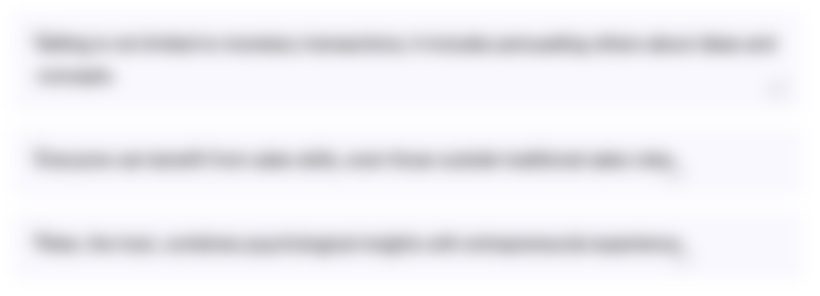
This section is available to paid users only. Please upgrade to access this part.
Upgrade NowTranscripts
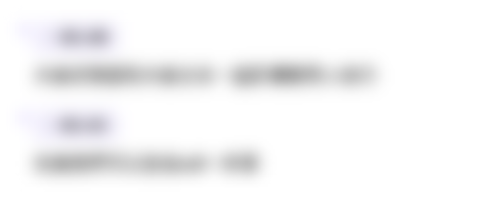
This section is available to paid users only. Please upgrade to access this part.
Upgrade NowBrowse More Related Video
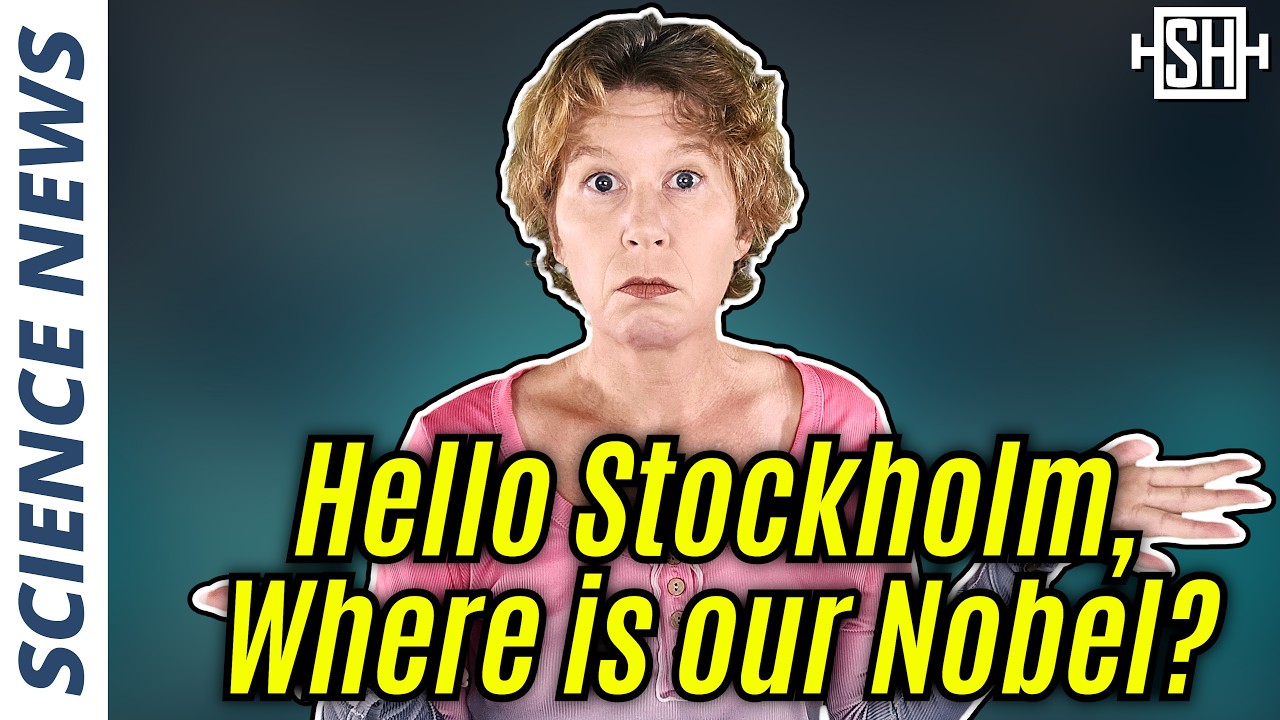
The 2024 Nobel Prize in Physics Did Not Go To Physics -- This Physicist is very surprised
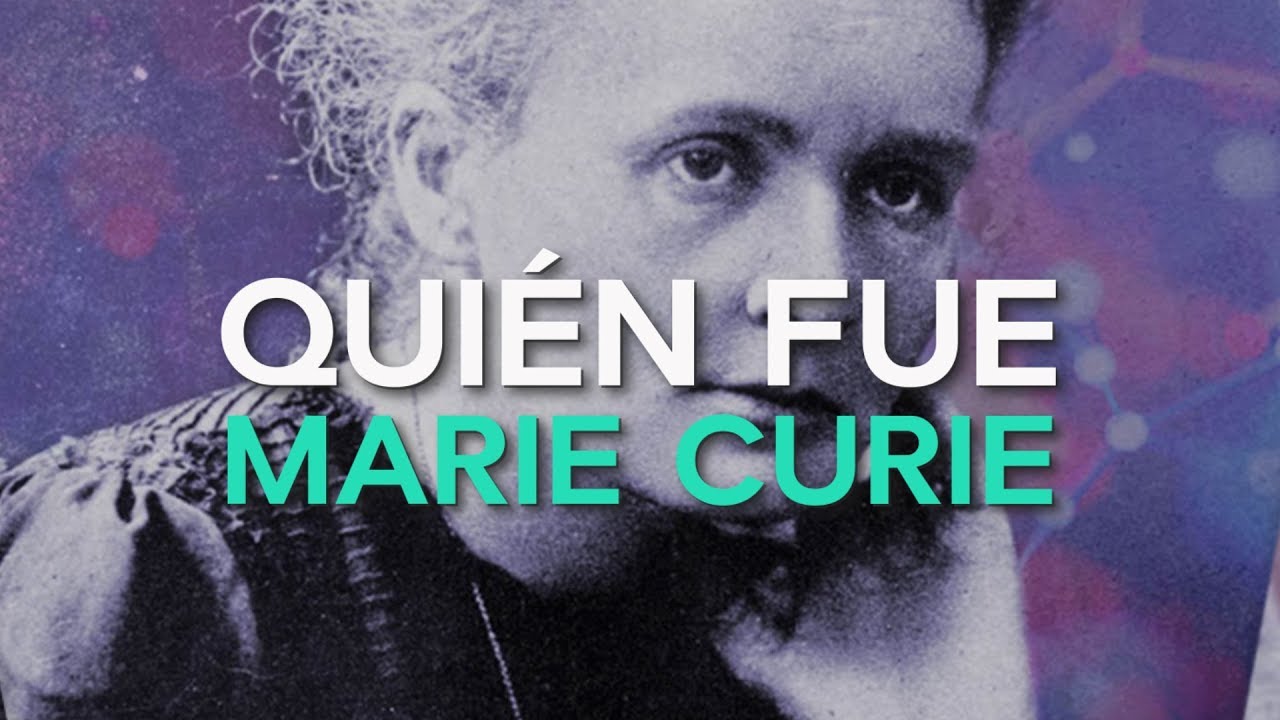
Quiรฉn fue Marie Curie ๐ | Cientรญfica y primera mujer en ganar un Nobel

First Reactions | Geoffrey Hinton, Nobel Prize in Physics 2024 | Telephone interview
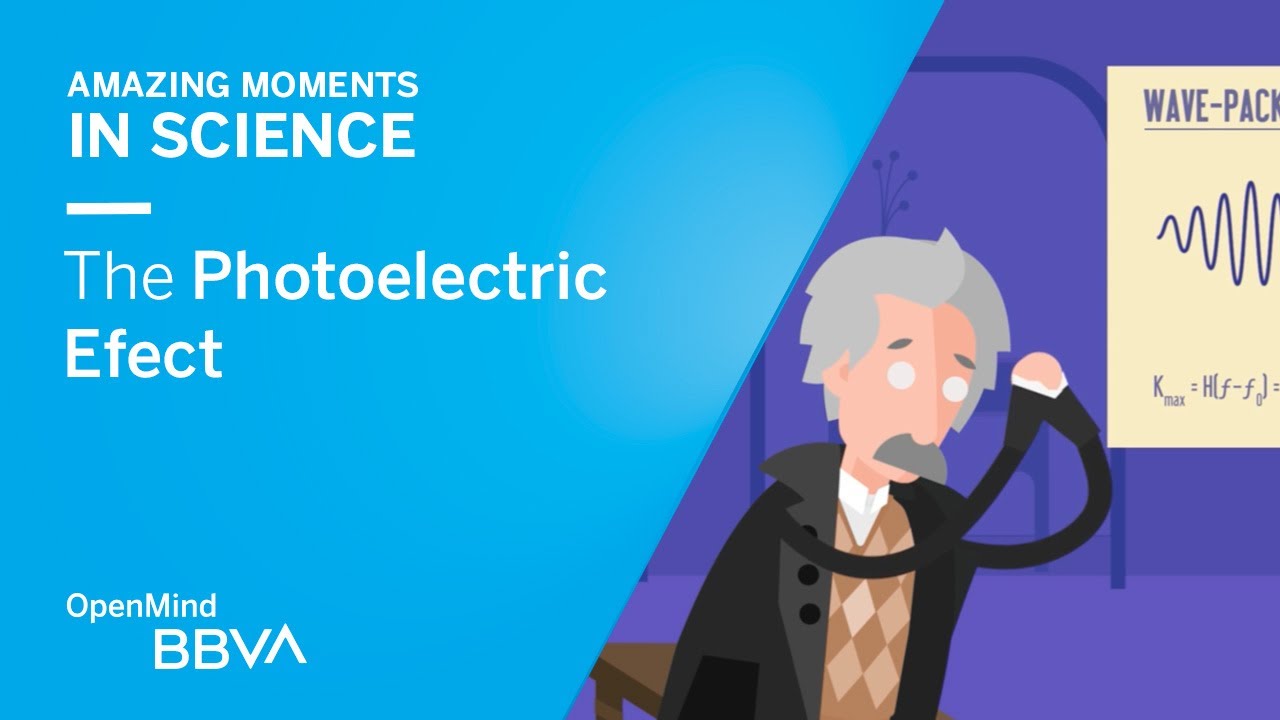
Albert Einstein and The Photoelectric Effect | AMS OpenMind
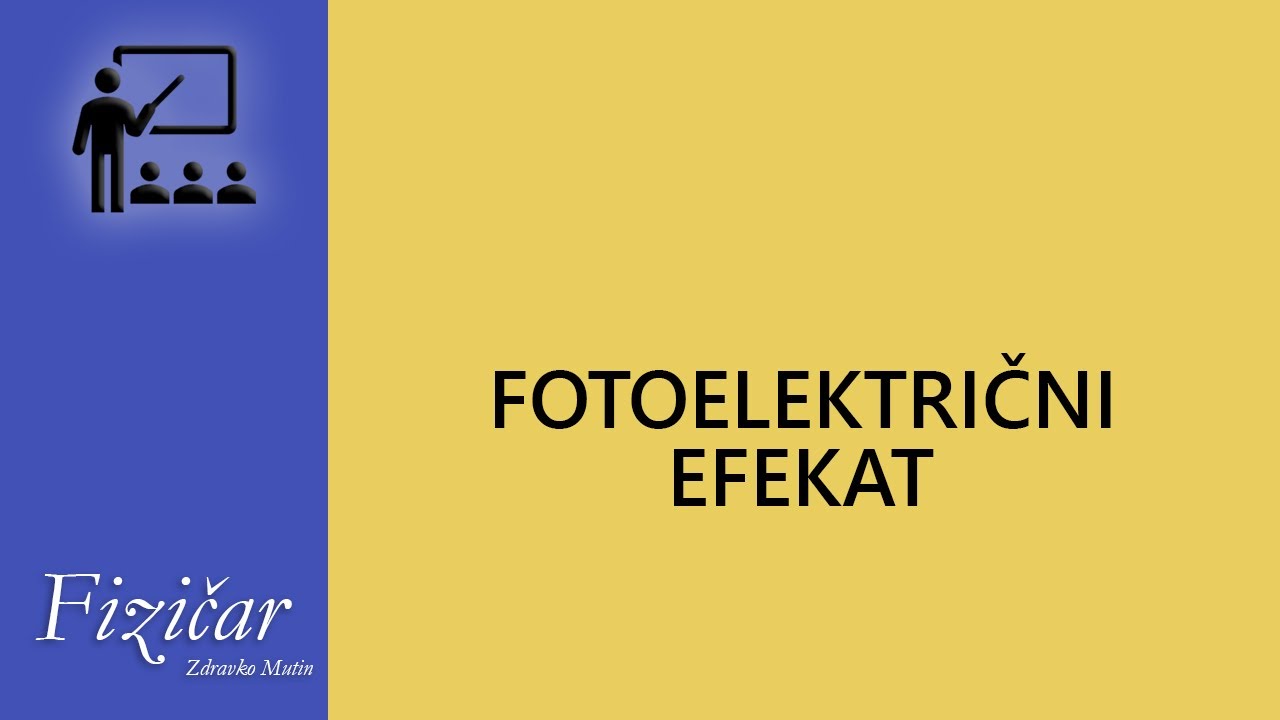
Physics - Photoelectric effect
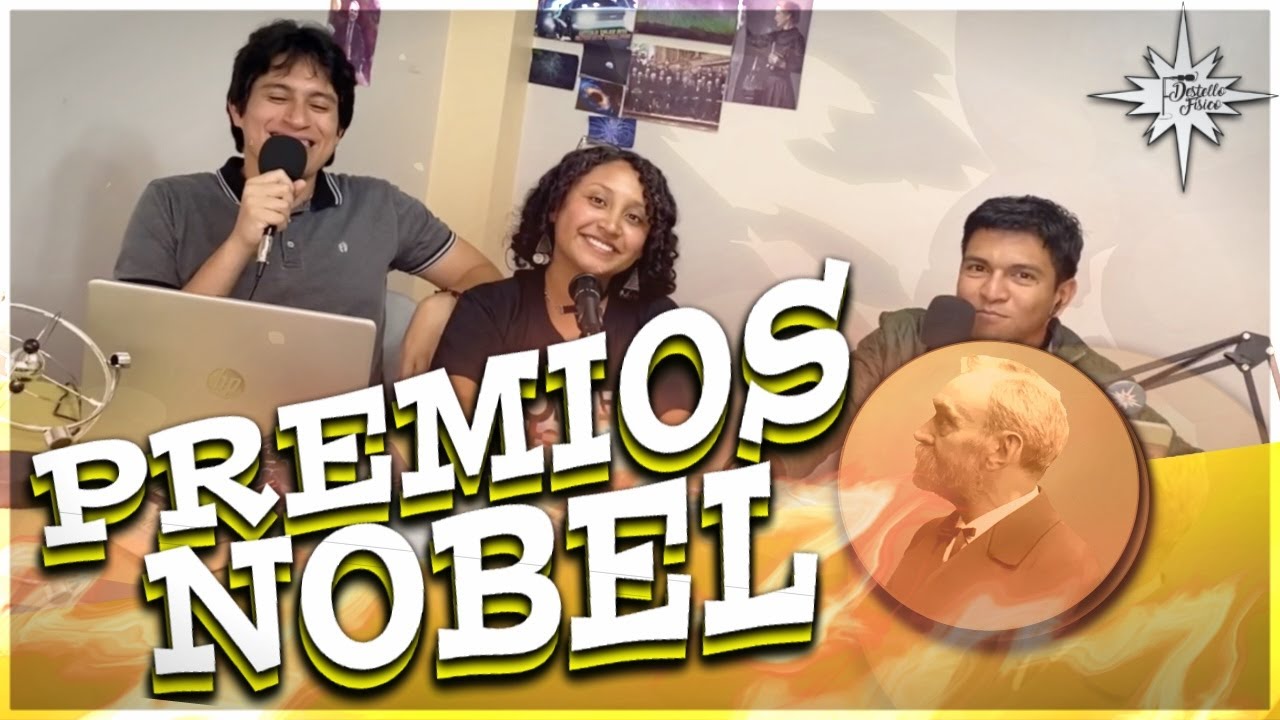
LOS PREMIOS NOBEL!!| Capรญtulo 5| Destello Fรญsico

Roentgen anniversary: 125 years of new insights - Part 1
5.0 / 5 (0 votes)