PlotDigitizer - How to Automatically Extract Data from Graph Image (#3)
Summary
TLDRThis video tutorial introduces the process of automatically extracting data from a graph image using PlotDigitizer. It emphasizes the efficiency of automation over manual extraction and outlines the three-step autotracing method involving color selection, mask application, and algorithm selection. The importance of calibration, the use of different mask types, and the need for minor adjustments due to the algorithms being in beta phase are also highlighted. The tutorial guides users through extracting red colored scattered points and curves, showcasing the software's capabilities and directing them to the official documentation for further information.
Takeaways
- 📈 Start by uploading the graph image to PlotDigitizer.com for data extraction.
- 🎨 Calibrate the graph by setting X1, X2, Y1, and Y2 markers to known coordinates.
- 🖌️ Choose the color of the data points or curves you wish to extract using the color picker tool.
- 🎭 Apply a mask to the area of interest; options include pen mask and box mask for precise selection.
- ✏️ Use the eraser mask to remove unwanted portions, such as overlapping or interfering elements.
- 🔍 Select the appropriate algorithm for the type of data extraction; point, curve, or histogram algorithms are available.
- 🔄 Algorithms are in beta, so minor adjustments may be necessary for accurate data extraction.
- 🔄 For overlapping points, manual corrections can be made to ensure data accuracy.
- 📊 View extracted data in the dataset table and save it for further analysis or processing.
- 🔄 Repeat the process for different colors or types of data within the same graph.
- 📈 Adjust point density for curves using the provided slider for a clearer representation.
Q & A
What is the main topic of the video tutorial?
-The main topic of the video tutorial is how to automatically extract data from a graph image using PlotDigitizer.com.
Why is watching the earlier videos in the series important before starting this tutorial?
-Watching the earlier videos is important because they provide foundational knowledge and context necessary for understanding the more advanced concepts taught in this video.
What is the first step in the automatic data extraction process?
-The first step in the automatic data extraction process is to calibrate the graph by moving the calibration markers X1 and X2, and Y1 and Y2 to known X and Y coordinates.
How does one calibrate a graph in PlotDigitizer?
-To calibrate a graph in PlotDigitizer, you use the zoom panel to drag and drop the calibration markers to the respective known X and Y coordinates on the graph.
What are the different types of masks available for autotracing in PlotDigitizer?
-The different types of masks available for autotracing in PlotDigitizer are the pen mask and the box mask.
What is the purpose of the pen mask in autotracing?
-The pen mask is used for extracting curves and provides more flexibility as you can control its thickness, making it ideal for precise tracing.
How can the eraser mask be utilized in the autotracing process?
-The eraser mask is used to remove or eliminate unwanted portions from the masked region, such as noises or interfering objects with the same color as the object of interest.
What is the recommended algorithm for extracting points in PlotDigitizer?
-For extracting points, the point algorithm is recommended in PlotDigitizer.
What should one do if the algorithms detect overlapping points as a single point?
-If the algorithms detect overlapping points as a single point, manual corrections can be made to fix such minor issues.
How can one view the extracted data?
-The extracted data can be viewed in the dataset table on the PlotDigitizer interface.
What is the process for extracting data from both curves and scattered points in the graph?
-The process involves calibrating the graph, selecting the desired color for extraction, applying the appropriate mask, choosing the correct algorithm, and making manual adjustments if necessary. This is repeated for each type of data to be extracted.
Outlines
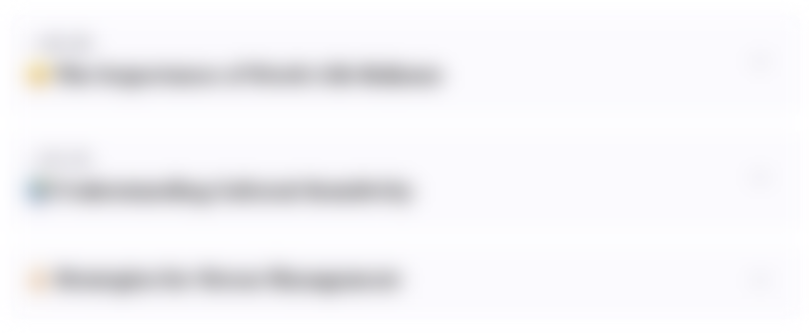
This section is available to paid users only. Please upgrade to access this part.
Upgrade NowMindmap
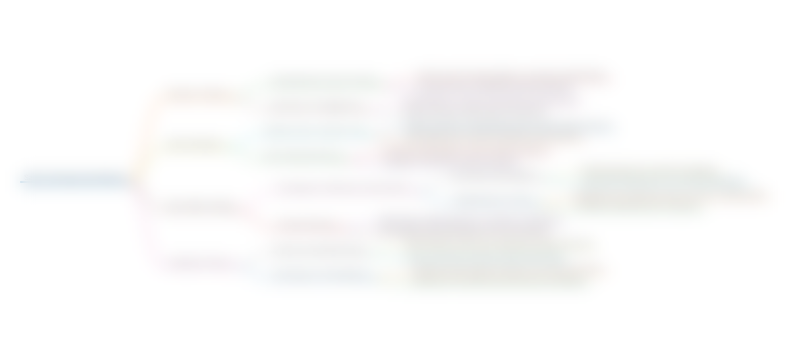
This section is available to paid users only. Please upgrade to access this part.
Upgrade NowKeywords
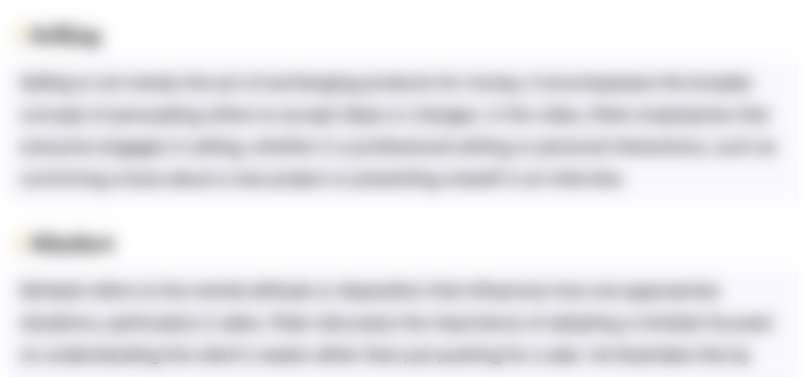
This section is available to paid users only. Please upgrade to access this part.
Upgrade NowHighlights
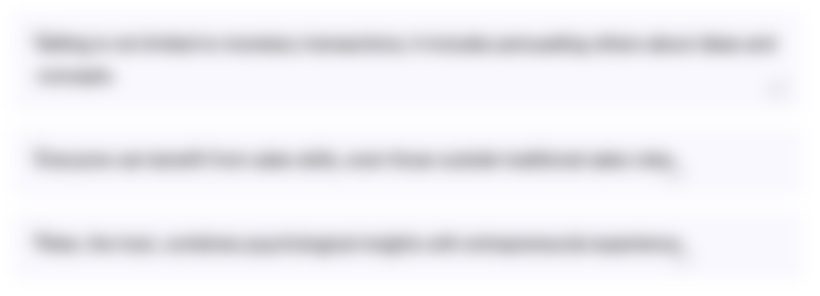
This section is available to paid users only. Please upgrade to access this part.
Upgrade NowTranscripts
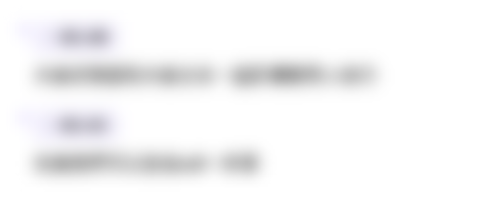
This section is available to paid users only. Please upgrade to access this part.
Upgrade NowBrowse More Related Video
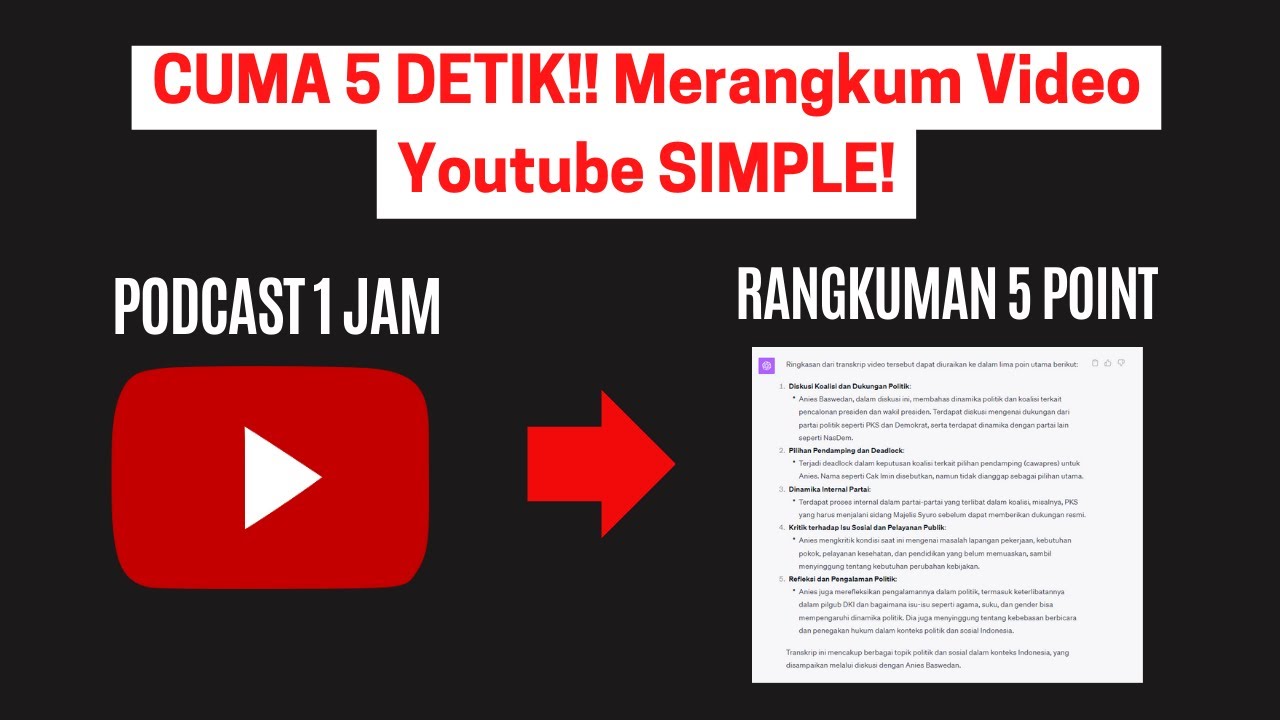
Ubah Video Youtube Menjadi Teks! Begini Cara Merangkum Video Youtube dengan ChatGPT
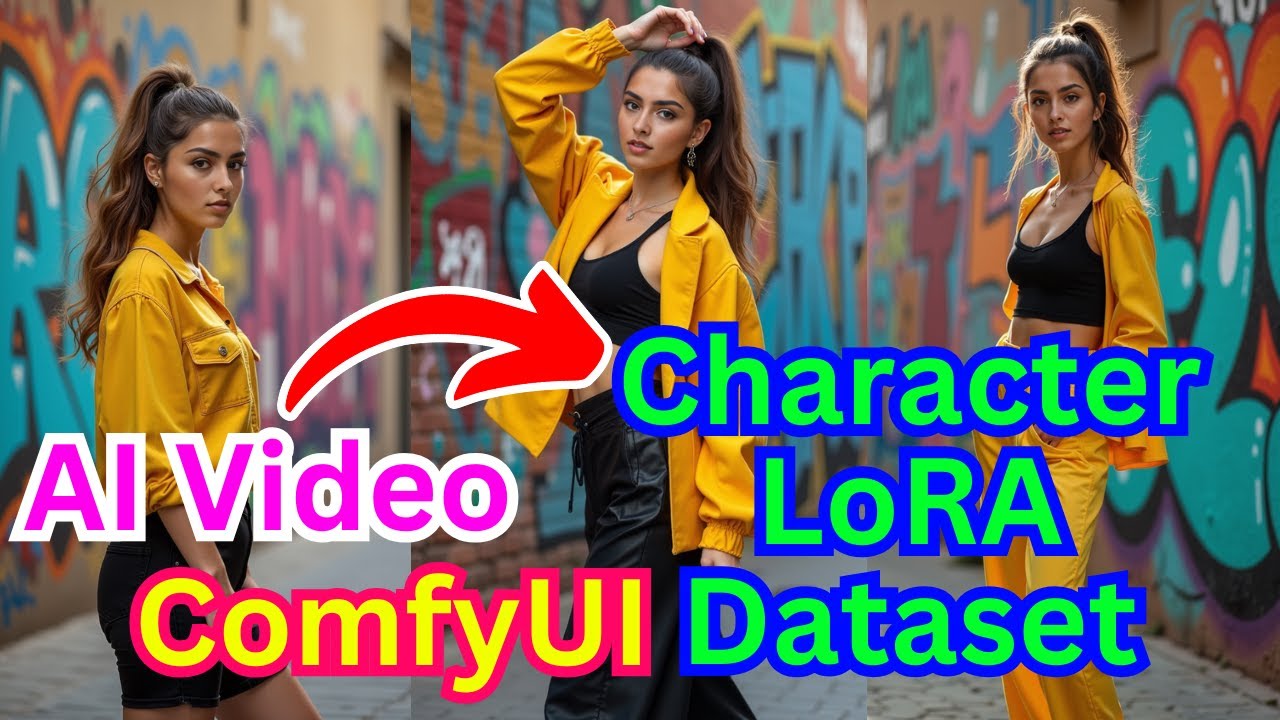
Wan 2.1 In ComfyUI - Create Character LoRA Dataset Using AI Video
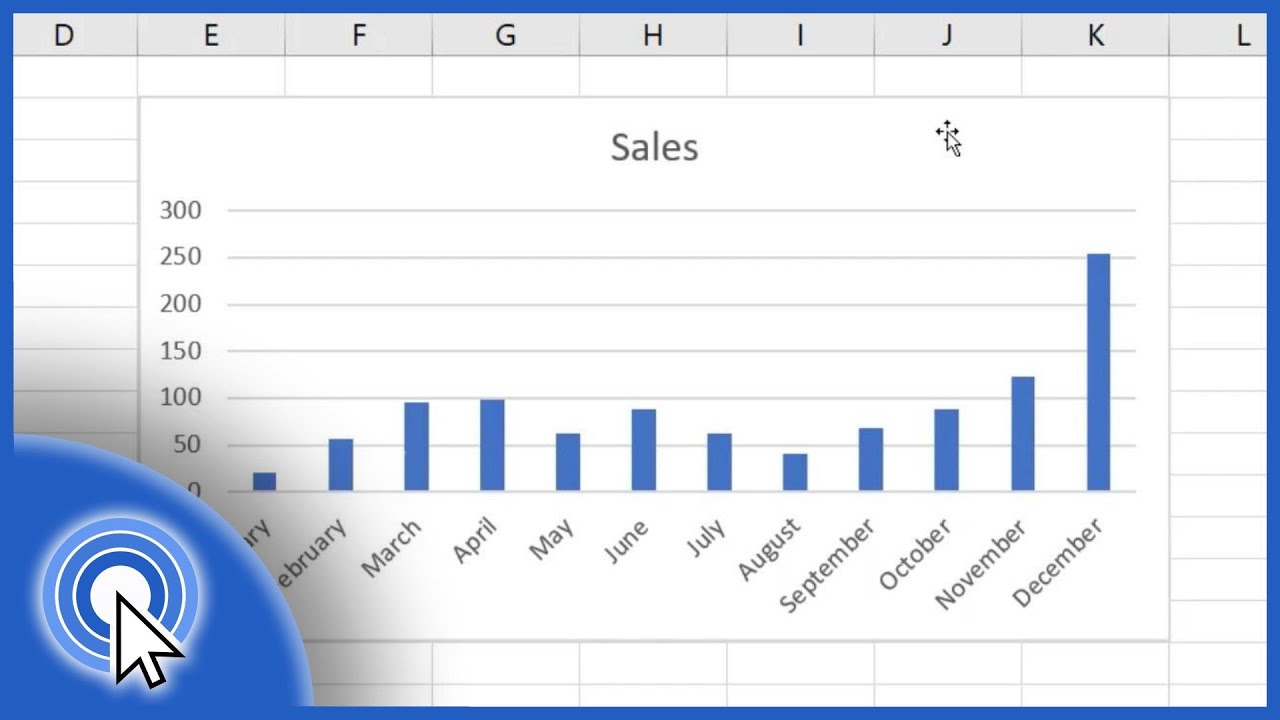
How to Make a Bar Graph in Excel
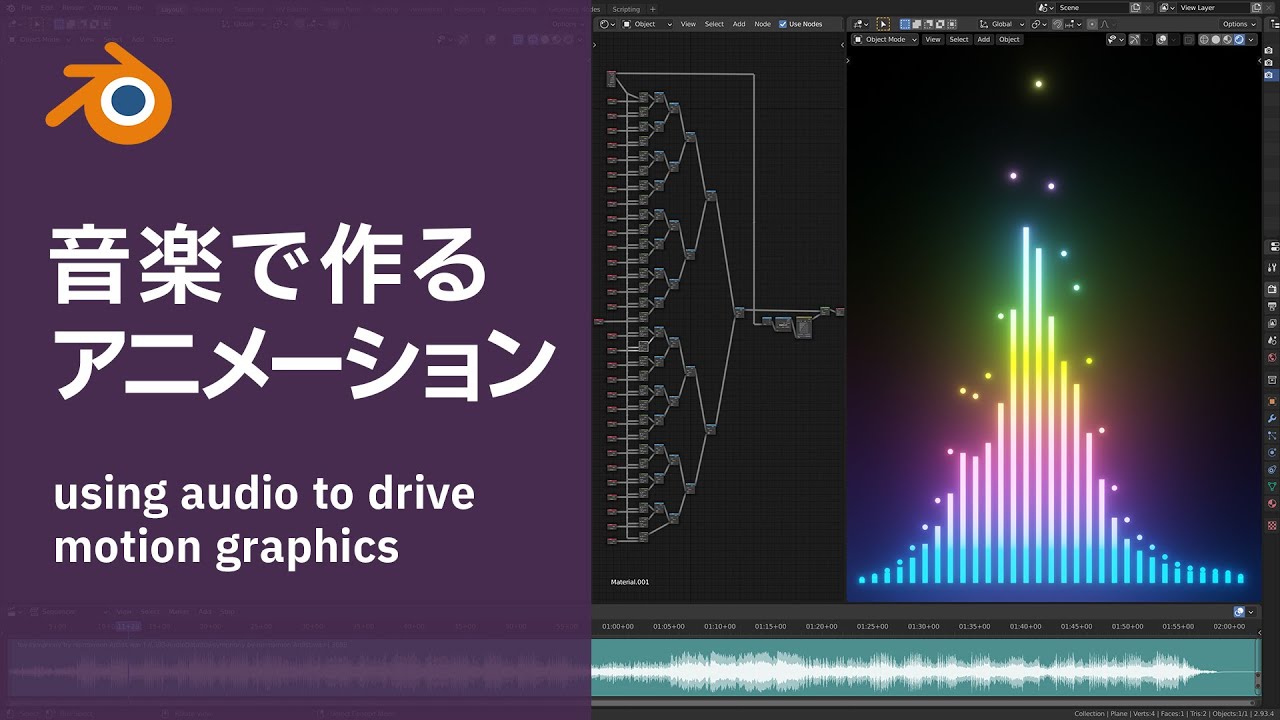
【Blender】 Animation made with audio data.
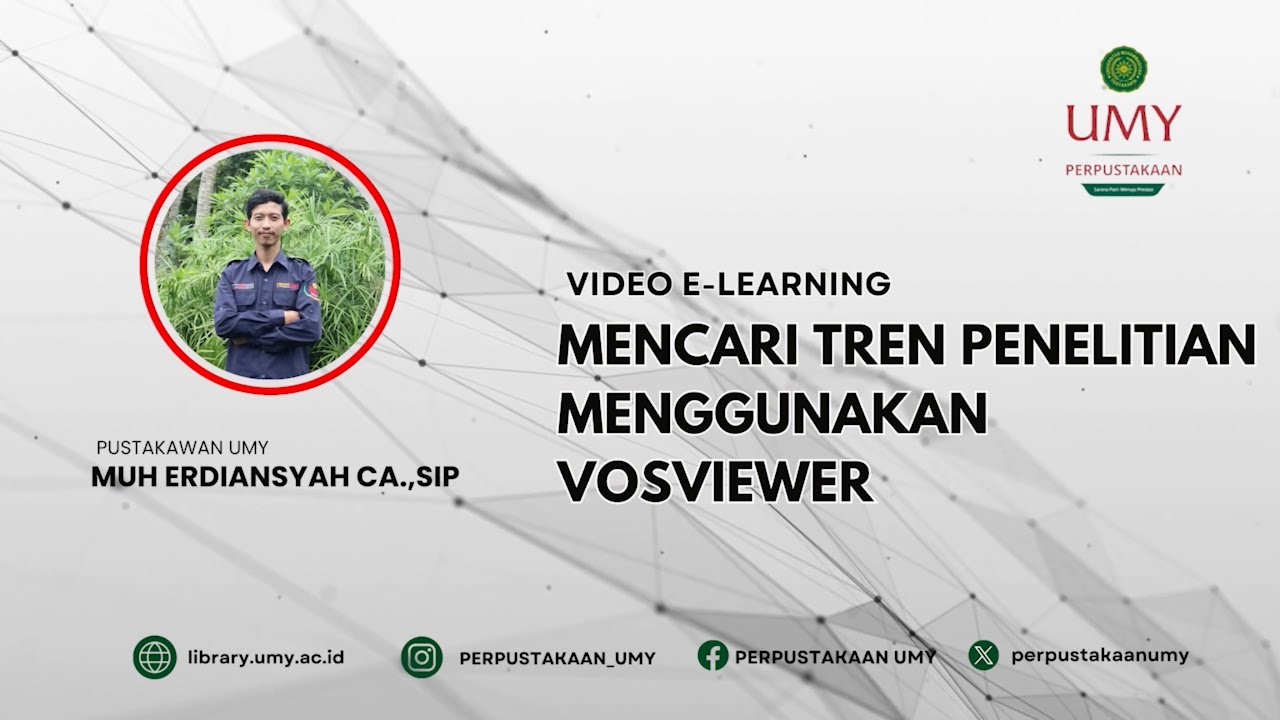
Mencari Tren Penelitian menggunakan Vosviewer
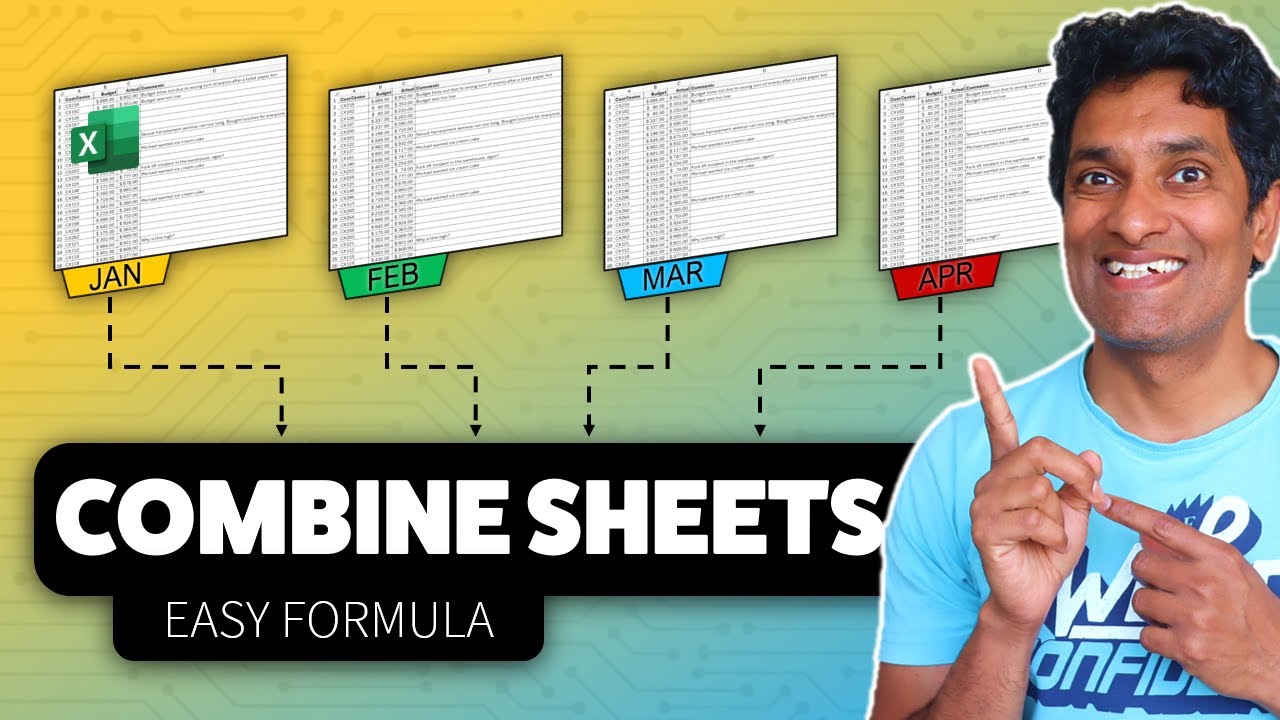
Combine Excel Sheets with *this* simple formula
5.0 / 5 (0 votes)