AI Is Dangerous, but Not for the Reasons You Think | Sasha Luccioni | TED
Summary
TLDRThe speaker, an AI researcher, addresses the growing concerns around the societal impacts of AI models. She highlights the urgent need to measure and mitigate AI's environmental costs, tackle issues like data privacy and consent, and address inherent biases that can perpetuate stereotypes and discrimination. By creating transparent tools and disclosing information, the speaker advocates for a future where AI models are more trustworthy, sustainable, and less likely to cause harm. The overarching message is that instead of obsessing over hypothetical existential risks, we should focus on addressing AI's tangible impacts on people and the planet right now.
Takeaways
- 🌍 AI models contribute to climate change and environmental issues due to their high energy consumption and carbon emissions during training and deployment.
- 📘 AI models use artwork, books, and other creative works for training without consent from the creators, raising copyright concerns.
- ⚖️ AI models can encode biases and stereotypes, leading to discrimination against certain communities when deployed in real-world scenarios.
- 🔍 Transparency and tools are needed to understand and measure the impacts of AI models, such as their environmental footprint, use of copyrighted data, and biases.
- 🛡️ Initiatives like CodeCarbon, Have I Been Trained?, and Stable Bias Explorer aim to provide tools for measuring and mitigating AI's impacts.
- 💻 Companies and developers should prioritize choosing more sustainable, unbiased, and ethically-trained AI models based on measurable impacts.
- 📋 Legislation and governance mechanisms are required to regulate AI's deployment in society and protect against harmful impacts.
- 🙋♀️ Users should have access to information about AI models' impacts to make informed choices about which models to trust and use.
- 🌱 Rather than focusing solely on hypothetical future risks, immediate action is needed to address AI's current, tangible impacts on society and the environment.
- 🤝 Collective effort from researchers, companies, policymakers, and users is required to steer AI's development in an ethical and responsible direction.
Q & A
What was the strangest email the AI researcher received?
-The AI researcher received an email from a random stranger saying that their work in AI is going to end humanity.
What are some of the negative headlines surrounding AI that the researcher mentioned?
-The researcher mentioned headlines about a chatbot advising someone to divorce their wife, and an AI meal planner app proposing a recipe containing chlorine gas.
What are some of the current tangible impacts of AI that the researcher discussed?
-The researcher discussed the environmental impact of training AI models, the use of copyrighted art and books without consent for training data, and the potential for AI models to discriminate against certain communities.
What is the Bloom model, and what did the researcher's study find about its environmental impact?
-Bloom is the first open large language model like ChatGPT, created with an emphasis on ethics, transparency, and consent. The researcher's study found that training Bloom used as much energy as 30 homes in a year and emitted 25 tons of carbon dioxide.
What tool did the researcher help create to measure the environmental impact of AI training?
-The researcher helped create CodeCarbon, a tool that runs in parallel to AI training code and estimates the energy consumption and carbon emissions.
What is the purpose of the "Have I Been Trained?" tool developed by Spawning.ai?
-The "Have I Been Trained?" tool allows users to search massive datasets to see if their images or text have been used for training AI models without their consent.
What did the researcher find when searching for images of herself in the LAION-5B dataset?
-The researcher found some images of herself from events she had spoken at, but also many images of other women named Sasha, including bikini models.
What tool did the researcher create to explore bias in image generation models?
-The researcher created the Stable Bias Explorer, which allows users to explore the bias of image generation models through the lens of professions.
What did the researcher's tool find regarding the representation of gender and race in professions generated by AI models?
-The tool found significant over-representation of whiteness and masculinity across 150 professions, even when compared to real-world labor statistics.
What was the researcher's response to the email claiming that their work would destroy humanity?
-The researcher responded that focusing on AI's future existential risks is a distraction from its current, tangible impacts, and that the work should be focused on reducing these impacts now.
Outlines
🤖 AI Researcher's Bizarre Email: Humanity's Demise?
The speaker, an AI researcher, received an unusual email claiming that their work in AI would lead to the end of humanity. They acknowledge the growing prominence of AI in the news, with both positive (medical breakthroughs) and negative (biased chatbots, dangerous recipe suggestions) headlines. Despite the doomsday scenarios discussed, the researcher admits uncertainty about AI's long-term impacts but highlights pressing current issues like environmental costs, consent violations, and bias. They emphasize the need for transparency, disclosure, and tools to better understand AI's impacts.
🌎 Addressing AI's Environmental Impact and Copyright Infringement
The speaker discusses the environmental toll of training large language models like Bloom and GPT-3, which can consume immense amounts of energy and emit significant carbon dioxide. They highlight the lack of measurement and disclosure from tech companies, and the need for tools like CodeCarbon to estimate emissions. Additionally, they mention the 'Have I Been Trained?' tool, which allows artists to check if their work was used without consent for training AI models. This tool provided evidence for a class-action lawsuit against AI companies for copyright infringement. The speaker also discusses new opt-in/opt-out mechanisms for data sets, emphasizing that artwork shouldn't be an 'all-you-can-eat buffet' for training AI.
⚖️ Tackling AI Bias and Charting a Responsible Path Forward
The speaker addresses the issue of bias in AI systems, citing examples like facial recognition systems failing to detect women of color and wrongful accusations due to biased models. They introduce the 'Stable Bias Explorer' tool, which explores biases in image generation models across professions, revealing significant representation of whiteness and masculinity. The speaker emphasizes the importance of making AI accessible and understandable to people from all walks of life. They argue that by creating tools to measure AI's impact, companies, legislators, and users can make informed choices and develop guardrails to protect society and the planet. The speaker concludes by stating that focusing on AI's future existential risks is a distraction from addressing its current tangible impacts, and that we can collectively decide the direction we want to go.
Mindmap
Keywords
💡AI (Artificial Intelligence)
💡Environmental Impact
💡Copyright and Consent
💡Bias
💡Transparency and Accessibility
💡Ethics and Governance
💡Large Language Models
💡Existential Risk
💡Sustainability
💡Tools and Metrics
Highlights
AI models can contribute to climate change, use art and books without consent, and discriminate against entire communities, but we need to start tracking their impacts.
Training the open-source Bloom language model used as much energy as 30 homes in a year and emitted 25 tons of carbon dioxide, while models like GPT-3 emit 20 times more carbon.
Switching to a larger language model emits 14 times more carbon for the same task.
Introduced CodeCarbon, a tool to estimate the energy consumed and carbon emitted during AI training, to make informed sustainable choices.
Spawning.ai's 'Have I Been Trained?' tool lets artists search datasets to see if their work was used without consent to train AI models.
Artists Karla Ortiz and others used evidence from 'Have I Been Trained?' to file a class action lawsuit against AI companies for copyright infringement.
Spawning.ai partnered with Hugging Face to create opt-in and opt-out mechanisms for artists' work in training datasets.
AI models can encode biases and stereotypes, as demonstrated by facial recognition systems performing worse for women of color.
Porcha Woodruff was wrongfully accused of carjacking due to an AI system's misidentification.
Introduced the Stable Bias Explorer tool to explore biases in image generation models across professions.
Found significant representation of whiteness and masculinity across professions in image generation models, even in contrast with real-world statistics.
Presented the Stable Bias Explorer at a UN event as an example of making AI accessible and understandable to non-technical audiences.
Creating tools to measure AI's impact can inform companies, legislators, and users in making ethical choices and developing regulations.
Focusing on AI's future existential risks is a distraction from addressing its current, tangible impacts.
We are building the road as we walk it, and can collectively decide the direction for AI's development and deployment.
Transcripts
So I've been an AI researcher for over a decade.
And a couple of months ago, I got the weirdest email of my career.
A random stranger wrote to me
saying that my work in AI is going to end humanity.
Now I get it, AI, it's so hot right now.
(Laughter)
It's in the headlines pretty much every day,
sometimes because of really cool things
like discovering new molecules for medicine
or that dope Pope in the white puffer coat.
But other times the headlines have been really dark,
like that chatbot telling that guy that he should divorce his wife
or that AI meal planner app proposing a crowd pleasing recipe
featuring chlorine gas.
And in the background,
we've heard a lot of talk about doomsday scenarios,
existential risk and the singularity,
with letters being written and events being organized
to make sure that doesn't happen.
Now I'm a researcher who studies AI's impacts on society,
and I don't know what's going to happen in 10 or 20 years,
and nobody really does.
But what I do know is that there's some pretty nasty things going on right now,
because AI doesn't exist in a vacuum.
It is part of society, and it has impacts on people and the planet.
AI models can contribute to climate change.
Their training data uses art and books created by artists
and authors without their consent.
And its deployment can discriminate against entire communities.
But we need to start tracking its impacts.
We need to start being transparent and disclosing them and creating tools
so that people understand AI better,
so that hopefully future generations of AI models
are going to be more trustworthy, sustainable,
maybe less likely to kill us, if that's what you're into.
But let's start with sustainability,
because that cloud that AI models live on is actually made out of metal, plastic,
and powered by vast amounts of energy.
And each time you query an AI model, it comes with a cost to the planet.
Last year, I was part of the BigScience initiative,
which brought together a thousand researchers
from all over the world to create Bloom,
the first open large language model, like ChatGPT,
but with an emphasis on ethics, transparency and consent.
And the study I led that looked at Bloom's environmental impacts
found that just training it used as much energy
as 30 homes in a whole year
and emitted 25 tons of carbon dioxide,
which is like driving your car five times around the planet
just so somebody can use this model to tell a knock-knock joke.
And this might not seem like a lot,
but other similar large language models,
like GPT-3,
emit 20 times more carbon.
But the thing is, tech companies aren't measuring this stuff.
They're not disclosing it.
And so this is probably only the tip of the iceberg,
even if it is a melting one.
And in recent years we've seen AI models balloon in size
because the current trend in AI is "bigger is better."
But please don't get me started on why that's the case.
In any case, we've seen large language models in particular
grow 2,000 times in size over the last five years.
And of course, their environmental costs are rising as well.
The most recent work I led, found that switching out a smaller,
more efficient model for a larger language model
emits 14 times more carbon for the same task.
Like telling that knock-knock joke.
And as we're putting in these models into cell phones and search engines
and smart fridges and speakers,
the environmental costs are really piling up quickly.
So instead of focusing on some future existential risks,
let's talk about current tangible impacts
and tools we can create to measure and mitigate these impacts.
I helped create CodeCarbon,
a tool that runs in parallel to AI training code
that estimates the amount of energy it consumes
and the amount of carbon it emits.
And using a tool like this can help us make informed choices,
like choosing one model over the other because it's more sustainable,
or deploying AI models on renewable energy,
which can drastically reduce their emissions.
But let's talk about other things
because there's other impacts of AI apart from sustainability.
For example, it's been really hard for artists and authors
to prove that their life's work has been used for training AI models
without their consent.
And if you want to sue someone, you tend to need proof, right?
So Spawning.ai, an organization that was founded by artists,
created this really cool tool called “Have I Been Trained?”
And it lets you search these massive data sets
to see what they have on you.
Now, I admit it, I was curious.
I searched LAION-5B,
which is this huge data set of images and text,
to see if any images of me were in there.
Now those two first images,
that's me from events I've spoken at.
But the rest of the images, none of those are me.
They're probably of other women named Sasha
who put photographs of themselves up on the internet.
And this can probably explain why,
when I query an image generation model
to generate a photograph of a woman named Sasha,
more often than not I get images of bikini models.
Sometimes they have two arms,
sometimes they have three arms,
but they rarely have any clothes on.
And while it can be interesting for people like you and me
to search these data sets,
for artists like Karla Ortiz,
this provides crucial evidence that her life's work, her artwork,
was used for training AI models without her consent,
and she and two artists used this as evidence
to file a class action lawsuit against AI companies
for copyright infringement.
And most recently --
(Applause)
And most recently Spawning.ai partnered up with Hugging Face,
the company where I work at,
to create opt-in and opt-out mechanisms for creating these data sets.
Because artwork created by humans shouldn’t be an all-you-can-eat buffet
for training AI language models.
(Applause)
The very last thing I want to talk about is bias.
You probably hear about this a lot.
Formally speaking, it's when AI models encode patterns and beliefs
that can represent stereotypes or racism and sexism.
One of my heroes, Dr. Joy Buolamwini, experienced this firsthand
when she realized that AI systems wouldn't even detect her face
unless she was wearing a white-colored mask.
Digging deeper, she found that common facial recognition systems
were vastly worse for women of color compared to white men.
And when biased models like this are deployed in law enforcement settings,
this can result in false accusations, even wrongful imprisonment,
which we've seen happen to multiple people in recent months.
For example, Porcha Woodruff was wrongfully accused of carjacking
at eight months pregnant
because an AI system wrongfully identified her.
But sadly, these systems are black boxes,
and even their creators can't say exactly why they work the way they do.
And for example, for image generation systems,
if they're used in contexts like generating a forensic sketch
based on a description of a perpetrator,
they take all those biases and they spit them back out
for terms like dangerous criminal, terrorists or gang member,
which of course is super dangerous
when these tools are deployed in society.
And so in order to understand these tools better,
I created this tool called the Stable Bias Explorer,
which lets you explore the bias of image generation models
through the lens of professions.
So try to picture a scientist in your mind.
Don't look at me.
What do you see?
A lot of the same thing, right?
Men in glasses and lab coats.
And none of them look like me.
And the thing is,
is that we looked at all these different image generation models
and found a lot of the same thing:
significant representation of whiteness and masculinity
across all 150 professions that we looked at,
even if compared to the real world,
the US Labor Bureau of Statistics.
These models show lawyers as men,
and CEOs as men, almost 100 percent of the time,
even though we all know not all of them are white and male.
And sadly, my tool hasn't been used to write legislation yet.
But I recently presented it at a UN event about gender bias
as an example of how we can make tools for people from all walks of life,
even those who don't know how to code,
to engage with and better understand AI because we use professions,
but you can use any terms that are of interest to you.
And as these models are being deployed,
are being woven into the very fabric of our societies,
our cell phones, our social media feeds,
even our justice systems and our economies have AI in them.
And it's really important that AI stays accessible
so that we know both how it works and when it doesn't work.
And there's no single solution for really complex things like bias
or copyright or climate change.
But by creating tools to measure AI's impact,
we can start getting an idea of how bad they are
and start addressing them as we go.
Start creating guardrails to protect society and the planet.
And once we have this information,
companies can use it in order to say,
OK, we're going to choose this model because it's more sustainable,
this model because it respects copyright.
Legislators who really need information to write laws,
can use these tools to develop new regulation mechanisms
or governance for AI as it gets deployed into society.
And users like you and me can use this information
to choose AI models that we can trust,
not to misrepresent us and not to misuse our data.
But what did I reply to that email
that said that my work is going to destroy humanity?
I said that focusing on AI's future existential risks
is a distraction from its current,
very tangible impacts
and the work we should be doing right now, or even yesterday,
for reducing these impacts.
Because yes, AI is moving quickly, but it's not a done deal.
We're building the road as we walk it,
and we can collectively decide what direction we want to go in together.
Thank you.
(Applause)
Browse More Related Video
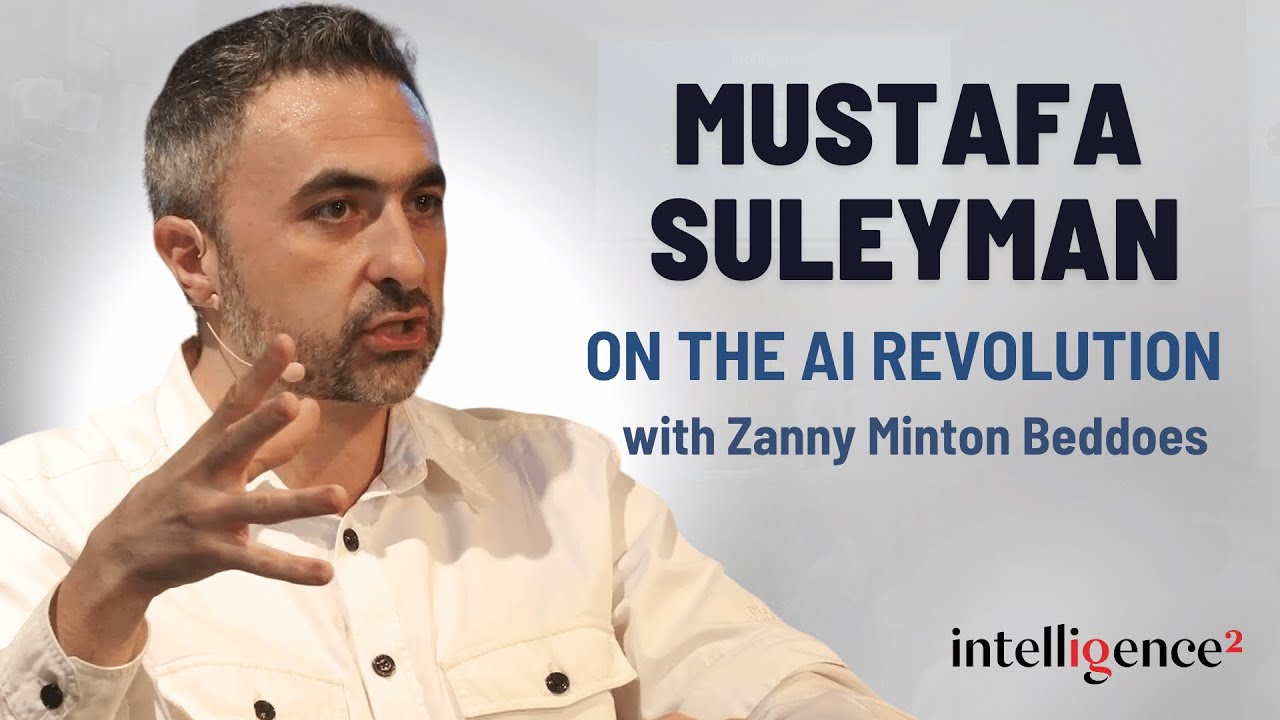
Mustafa Suleyman on The Coming Wave of AI, with Zanny Minton Beddoes
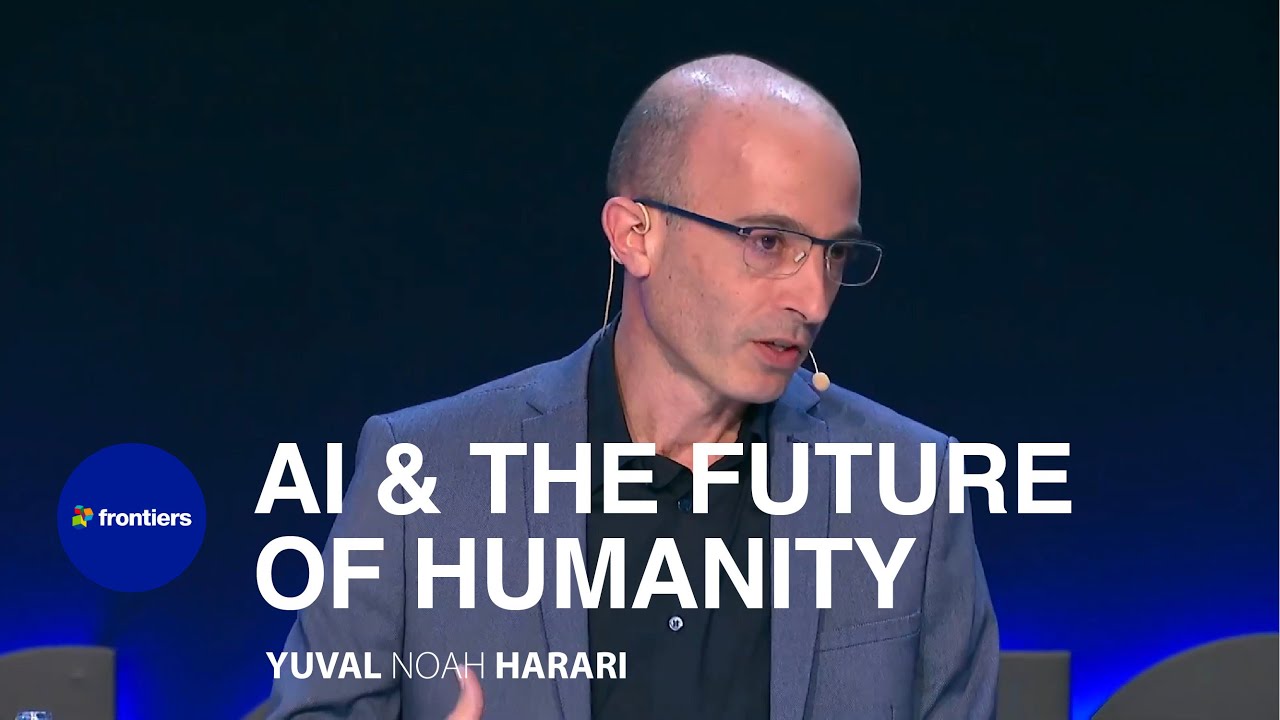
AI and the future of humanity | Yuval Noah Harari at the Frontiers Forum
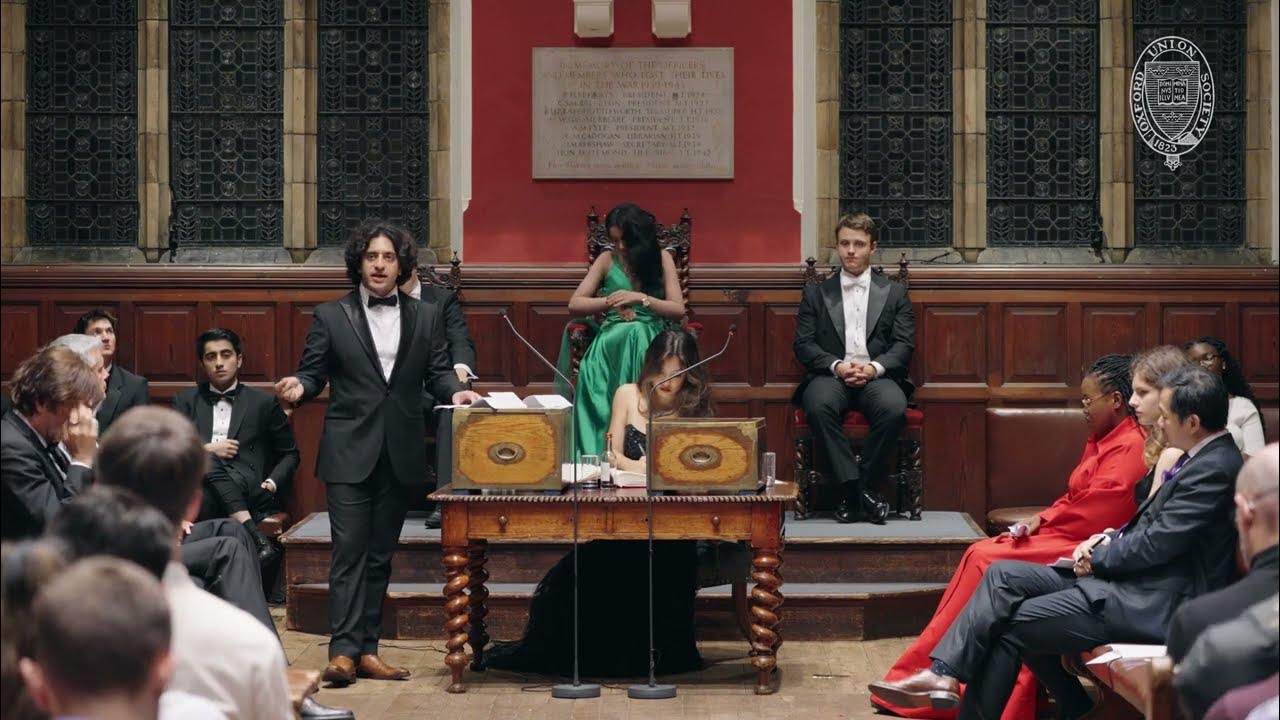
Sultan Khokhar warns of existential risks posed by increasing use of Artifical Intelligence (1/8)
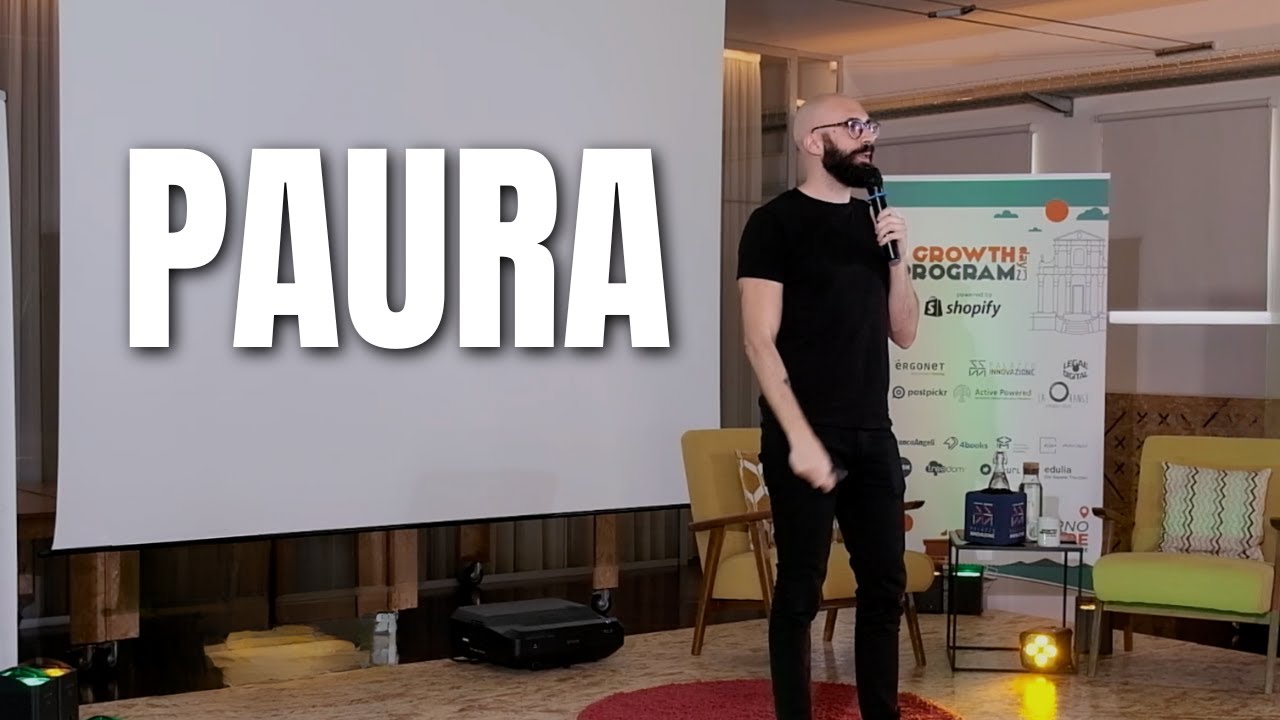
Hai paura dell'intelligenza artificiale? Guarda questo video

We’re already using AI more than we realize

Artificial Intelligence: 10 Risks You Should Know About
5.0 / 5 (0 votes)