Nvidia's Announces Game Changer AI Platform That Changes Everything For AMD & Intel, Worth Trillions
Summary
TLDRDieses Skript fasst die Entwicklung von Nvidia und die Bedeutung von Deep Learning in der Generativen KI zusammen. Es erzählt von der Geburt von Nvidia, der Erfindung des programmierbaren Shading GPUs, bis hin zu den Fortschritten in der Computergrafik und der Einführung von AI in der Industrie. Es präsentiert auch die Herausforderungen und Lösungen im Bereich der Energieeffizienz und zeigt die Vision einer zukünftigen KI, die nicht nur die Produktivität steigert, sondern auch neue Wissenschaft entdeckt und die Energieeffizienz verbessert.
Takeaways
- 🚀 Die Beschleunigung des Deep Learnings um eine Million Mal hat die Schaffung großer Sprachmodelle und die Entstehung von generativer KI ermöglicht.
- 📉 Der Kosten- und Energieverbrauch für die Entwicklung von generativer KI hat sich drastisch reduziert.
- 🎨 NVIDIA hat sich durch die Erfindung des programmierbaren Shading und der GPUs für Computer-Grafiken einen Namen gemacht.
- 🧠 Die erste Kontaktaufnahme mit KI erfolgte 2012 mit AlexNet, was ein Durchbruch in der Computer Vision darstellte.
- 🔧 NVIDIA hat seine Forschung und Entwicklung auf Deep Learning umgestellt, um neue Software-Schreibweisen zu erforschen und zu nutzen.
- 🤖 Die Einführung des DJX1 von NVIDIA im Jahr 2016 markierte einen Wendepunkt in der Entwicklung von KI für autonome Fahrzeuge und Robotik.
- 🔄 Der Einsatz von DLSS (Deep Learning Super Sampling) ermöglichte eine enorme Geschwindigkeitssteigerung bei der Ray-Tracing-Grafik.
- 🌐 Generative AI wird in naher Zukunft viele Branchen revolutionieren, einschließlich wissenschaftlicher Computing und autonomer Fahrzeuge.
- 🛡️ Neuere Fortschritte in der KI, wie z.B. die Verstärkung durch menschliches Feedback und die 'Guard railing', haben dazu beigetragen, die Kontrollbarkeit und Genauigkeit von generativer KI zu erhöhen.
- 🔍 Die Einführung von 'Retrieval-augmented generation' hat es möglich gemacht, die KI mit semantischen Datenbanken zu beliefern, um eine fundierterere und zielgerichtetere Antwortfähigkeit zu erreichen.
- 🌍 Generative AI ist energieintensiv, doch es gibt Hoffnung, dass durch neue Technologien wie Blackwell die Energieeffizienz signifikant gesteigert werden kann.
Q & A
Wie wurde das Deep-Learning vor einigen Jahren beschleunigt?
-Das Deep-Learning wurde durch eine Millionfache Beschleunigung vor einigen Jahren vorangetrieben, was es möglich machte, große Sprachmodelle zu erstellen.
Was war der Grund für die Entstehung von generativer KI?
-Die Entstehung von generativer KI wurde durch die Millionfache Beschleunigung und Kostenreduktion ermöglicht, was die Entwicklung von allgemeiner generativer KI erlaubte.
Was ist der Unterschied zwischen traditioneller Computergrafik und der durch AI unterstützten Computergrafik?
-Traditionelle Computergrafik basiert auf programmierbaren Schattierungen und Rendering-Techniken, während AI-unterstützte Computergrafik in der Lage ist, komplexe Aufgaben wie Ray Tracing in Echtzeit durchzuführen, was zu einer höheren Qualität bei interaktiven Visualisierungen führt.
Welche Rolle spielten die Tensor Core GPUs und das MVLink Switch Fabric in der Entwicklung von AI?
-Tensor Core GPUs und das MVLink Switch Fabric sind grundlegende Bausteine für die Beschleunigung von AI-Anwendungen und haben es ermöglicht, Deep-Learning-Modelle effizienter und schneller zu trainieren und zu nutzen.
Wie hat sich die Einführung von DLSS (Deep Learning Super Sampling) auf die Computergrafik ausgewirkt?
-DLSS hat es ermöglicht, hochauflösende, vollständig raytrace-basierte Simulationen mit einer hohen Framerate zu rendern, was durch die Verwendung von AI und generativer KI erreicht wurde, die die Qualität bei einer reduzierten Anzahl von berechneten Pixeln erhält.
Was ist der Unterschied zwischen Chat GPT und früheren AI-Modellen?
-Chat GPT nutzt reinforcement learning with human feedback, um die AI an unseren Kernwerten auszurichten und die gewünschten Fähigkeiten zu erlernen, was zu einer größeren Kontrollbarkeit und Genauigkeit führt.
Wie wird die zukünftige Entwicklung von generativer AI durch die Energieeffizienz beeinflusst?
-Generative AI hat das Potenzial, die Energieeffizienz in vielen Bereichen zu verbessern, indem sie die Notwendigkeit reduziert, Daten aus Datenzentren abzurufen und stattdessen lokal generiert werden kann.
Was sind die Vorteile von Domain-Specific Libraries (DSLs) in Bezug auf generative AI?
-DSLs wie CDNN für generative AI und CDF für SQL-Verarbeitung ermöglichen spezialisierte Beschleunigung für bestimmte Anwendungen, was die Leistung und Effizienz in diesen Bereichen erhöht.
Wie plant NVIDIA, die Herausforderungen der Energieintensität von generativer AI zu bewältigen?
-NVIDIA plant, durch die Entwicklung von Technologien wie Blackwell, die die Anwendungsleistung bei konstanter Energie und Kosten erhöht, sowie durch die Verlagerung von Datenzentren in Bereiche mit überschüssiger Energie, die Herausforderungen zu bewältigen.
Was ist das Konzept hinter Omniverse und wie wird es in der generativen AI eingesetzt?
-Omniverse ist eine Plattform zur Zusammensetzung von multimodalen Daten, die es ermöglicht, Inhalte aus verschiedenen Quellen zu verbinden und zu steuern. In Kombination mit generativer AI kann dies zu einer präziseren und kontrollierteren Erstellung von Inhalten führen.
Wie wird die zukünftige Entwicklung von Hardware im Bereich der generativen AI aussehen?
-Die Entwicklung von Hardware wird sich darauf konzentrieren, die Leistung von generativer AI weiter zu verbessern, indem man neue Prozessoren und Systeme entwirft, die speziell für die Anforderungen von AI-Anwendungen optimiert sind.
Outlines
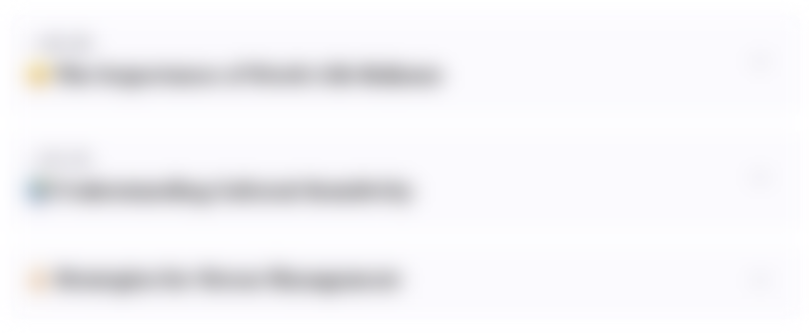
Этот раздел доступен только подписчикам платных тарифов. Пожалуйста, перейдите на платный тариф для доступа.
Перейти на платный тарифMindmap
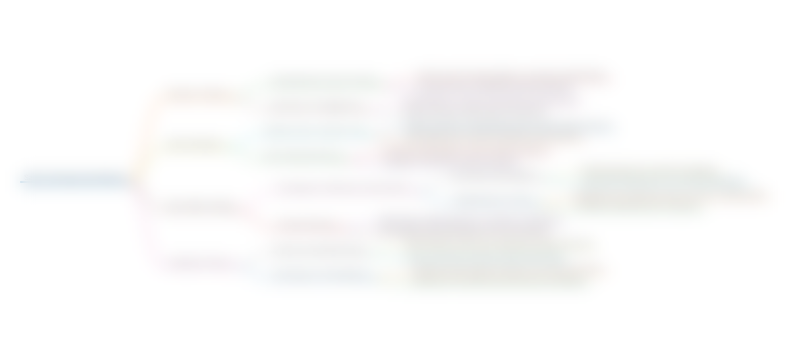
Этот раздел доступен только подписчикам платных тарифов. Пожалуйста, перейдите на платный тариф для доступа.
Перейти на платный тарифKeywords
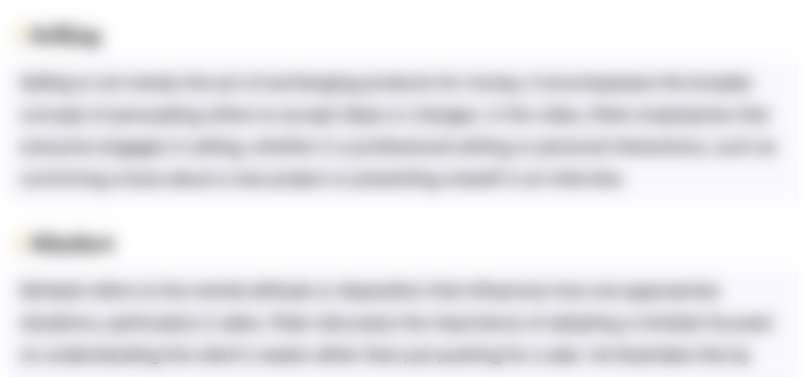
Этот раздел доступен только подписчикам платных тарифов. Пожалуйста, перейдите на платный тариф для доступа.
Перейти на платный тарифHighlights
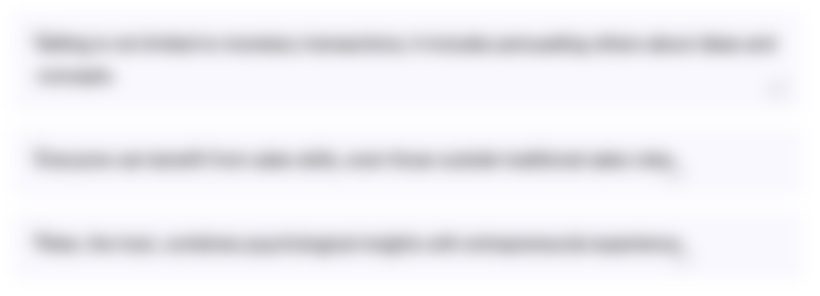
Этот раздел доступен только подписчикам платных тарифов. Пожалуйста, перейдите на платный тариф для доступа.
Перейти на платный тарифTranscripts
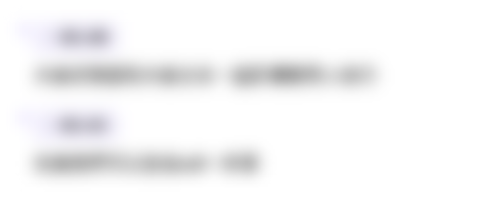
Этот раздел доступен только подписчикам платных тарифов. Пожалуйста, перейдите на платный тариф для доступа.
Перейти на платный тарифПосмотреть больше похожих видео
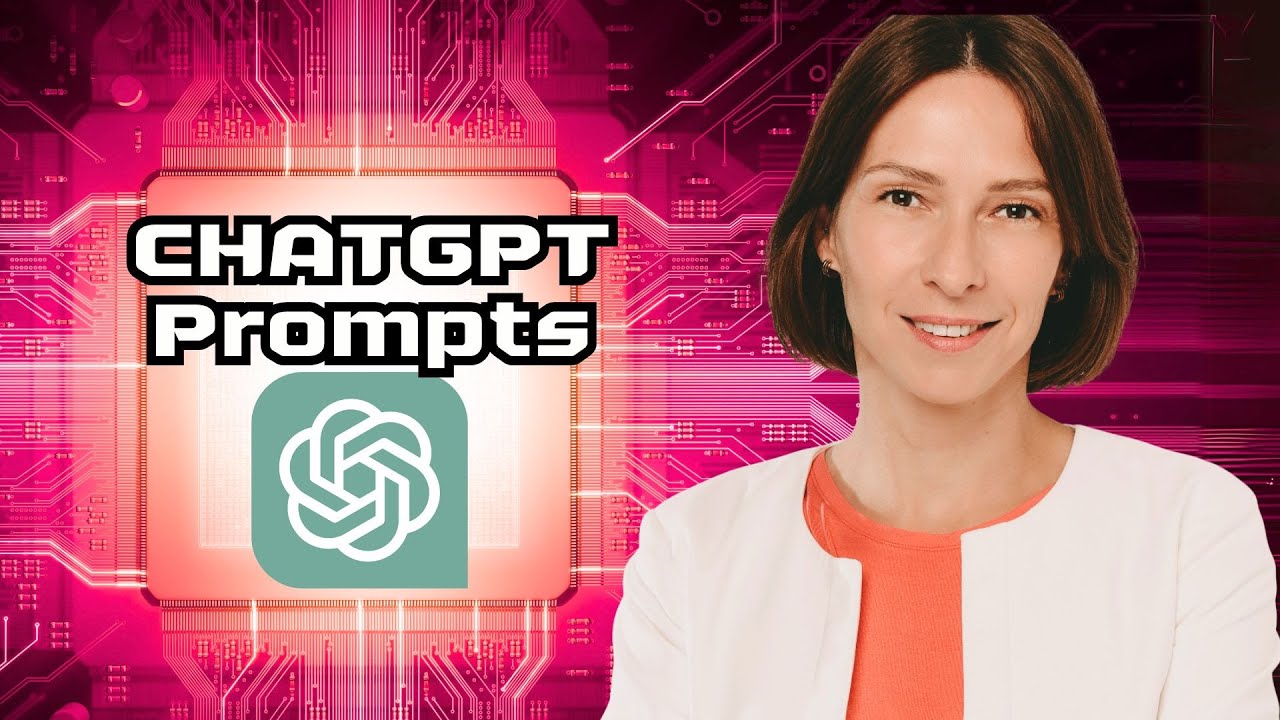
Wie erstelle ich gute Prompts für ChatGPT & Co?
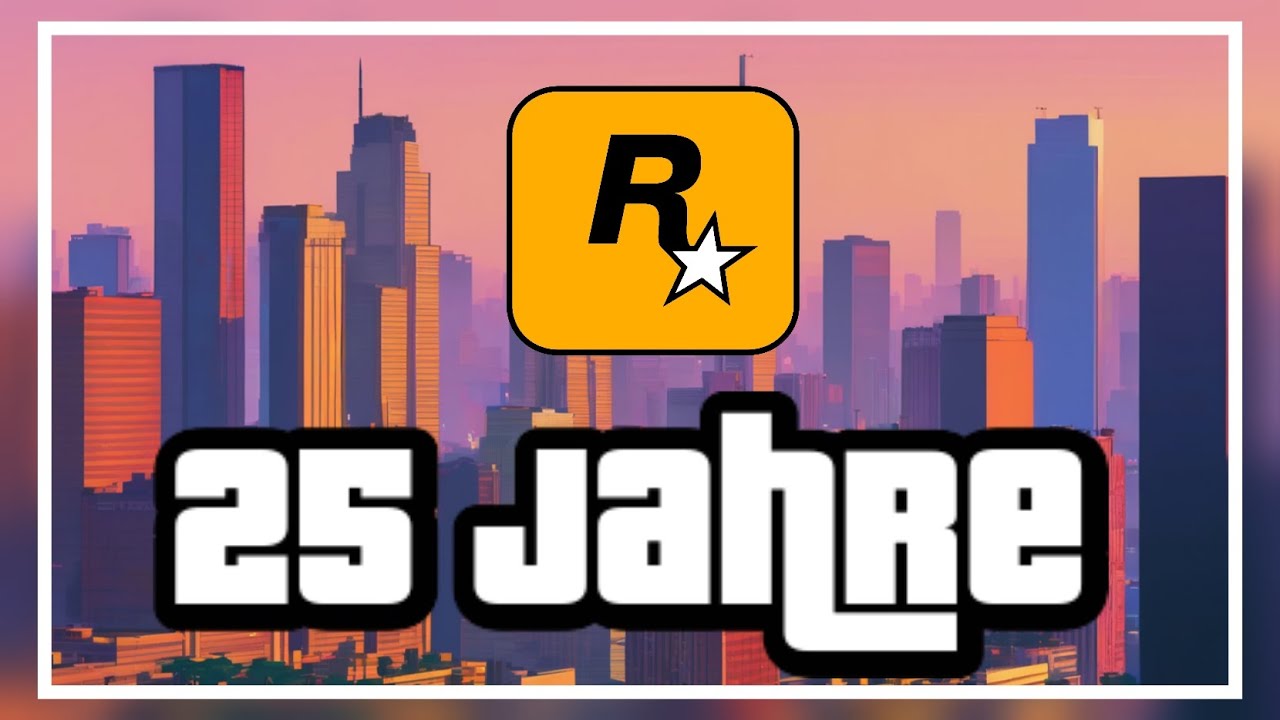
Mehr als nur GTA - Ein Rückblick auf 25 Jahre Rockstar Games
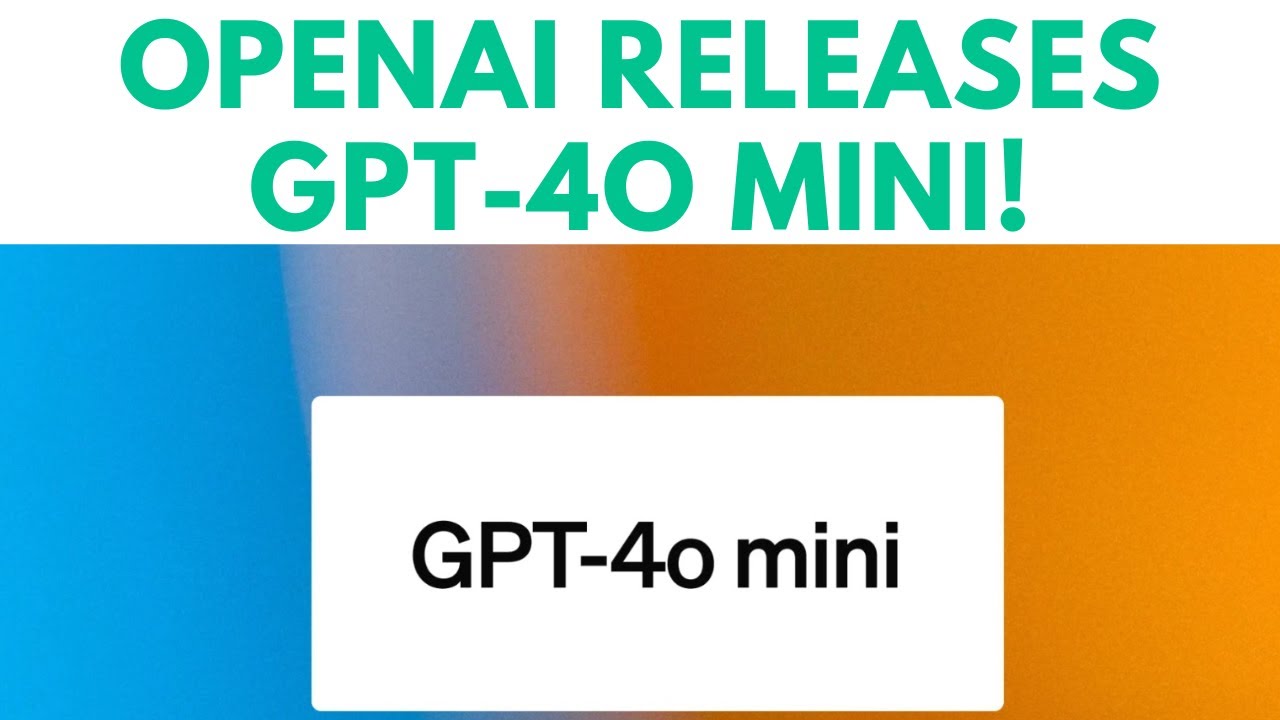
OpenAI Releases GPT-4o Mini! How Does It Compare To Other Models?
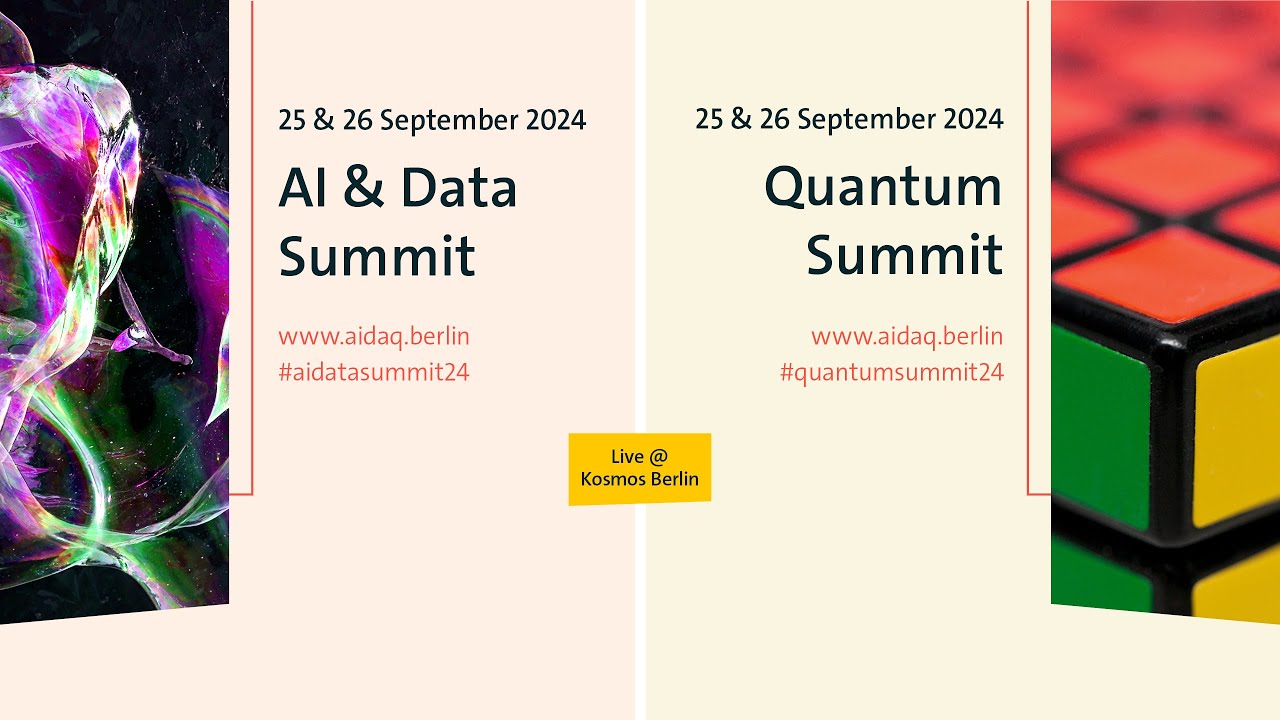
Aerospace & AI - Airbus’ responsible and human-centric approach to AI | #aidatasummit24

Recycling: Wie aus Scherben Flaschen werden | Wie geht das? | NDR Doku
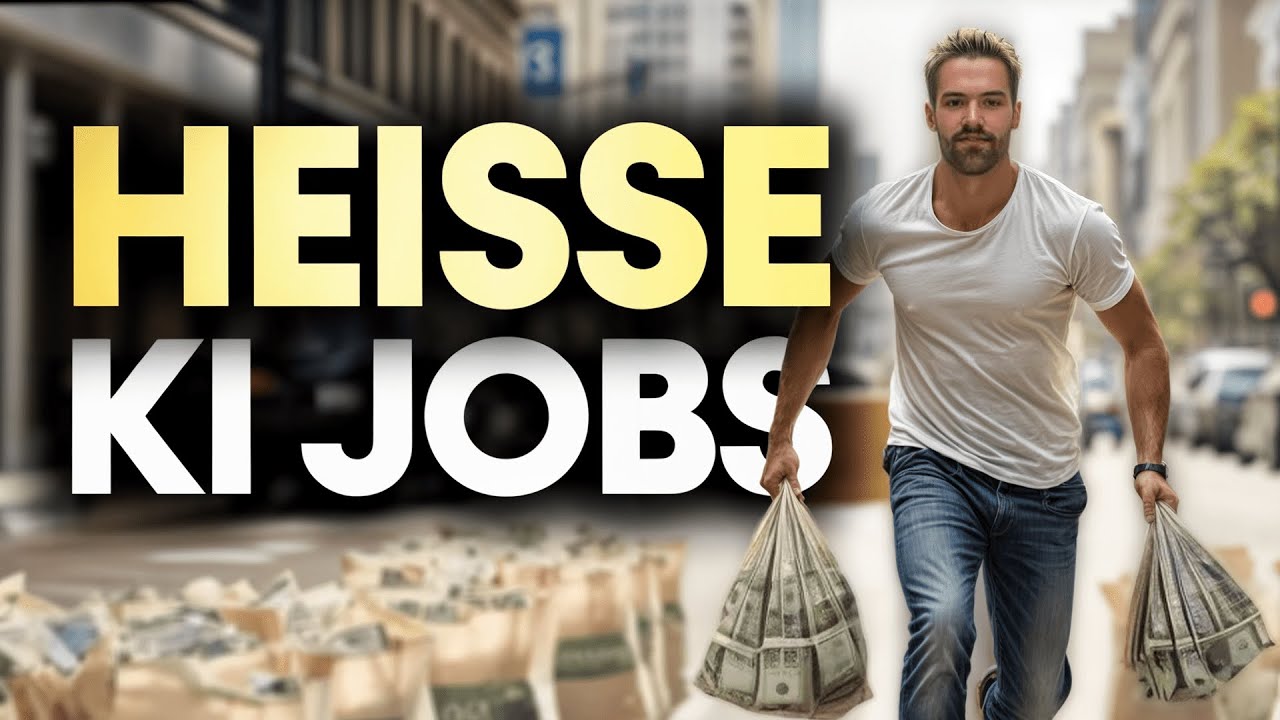
KI-Jobs der ZUKUNFT - 6 Top-Bezahlte Jobs in der KI-REVOLUTION
5.0 / 5 (0 votes)