PCA and Biplot Analysis to Identify Determinants of the Human Development Index in Central Java
Summary
TLDRThis presentation focuses on the application of Principal Component Analysis (PCA) and Biplot Analysis to investigate the Human Development Index (HDI) in Central Java, Indonesia. By analyzing data from 35 cities and regencies, the research identifies key factors influencing HDI, such as life expectancy, education, and per capita expenditure. PCA is used to reduce dimensionality and uncover patterns, while Biplot Analysis maps the relationships and variations between these factors. The study highlights significant correlations, such as the positive relationships between education indicators and the negative correlation between life expectancy and per capita spending. The findings offer valuable insights into regional development.
Takeaways
- 😀 The Human Development Index (IPM) is an important indicator to measure human progress in terms of income, welfare, and access to education.
- 😀 IPM in Indonesia reached a high category in 2020, with an average annual increase of 0.72%, reaching 74.39% in 2023.
- 😀 In Central Java, the IPM is also categorized as high, with a value of 73.39% in 2023.
- 😀 The study uses Principal Component Analysis (PCA) and Biplot to analyze IPM data, which includes indicators such as life expectancy, school years, and per capita income.
- 😀 The research uses data from 35 cities and regencies in Central Java, based on statistics from the Indonesian Central Statistics Agency (BPS).
- 😀 A correlation matrix is used to identify relationships between variables like life expectancy, school years, and per capita income.
- 😀 PCA helps to identify the most significant components contributing to human development, with the first two principal components (PC1 and PC2) explaining over 92% of the variance in the data.
- 😀 Eigenvalues and eigenvectors are calculated to form the basis of the PCA, helping to create a linear combination of principal components.
- 😀 The Biplot analysis reveals the similarity between different regions based on their IPM variables, with clusters of cities like Boyolali, Sragen, and Semarang showing similar characteristics.
- 😀 The study concludes that life expectancy and per capita income are negatively correlated, while education-related variables (school years and life expectancy) are positively correlated with each other.
Q & A
What is the main focus of this research presentation?
-The main focus of the research is on using Principal Component Analysis (PCA) and Biplot analysis to identify the determinants of the Human Development Index (IPM) in Central Java.
What does the Human Development Index (IPM) measure?
-The Human Development Index (IPM) measures the level of human development across various aspects such as life expectancy, education (mean years of schooling and expected years of schooling), and per capita income.
What are the key indicators used to represent the Human Development Index in the study?
-The key indicators used to represent IPM in the study are life expectancy (X1), expected years of schooling (X2), mean years of schooling (X3), and per capita expenditure (X4).
What is the purpose of conducting PCA in this research?
-The purpose of conducting PCA in this research is to analyze the variance and correlation between the different variables (indicators) of the Human Development Index across various regions (counties and cities) in Central Java.
How many principal components were determined to be significant in the study?
-The study determined that only two principal components (PC1 and PC2) were significant, explaining over 92% of the variance in the data.
What is the relationship between the variables X2 (expected years of schooling) and X3 (mean years of schooling)?
-There is a strong positive correlation between X2 and X3, as indicated by a correlation coefficient of 0.90, suggesting that an increase in expected years of schooling is closely related to an increase in mean years of schooling.
How does PCA help in interpreting the data for this study?
-PCA helps in reducing the dimensionality of the data, making it easier to interpret by identifying the principal components that explain the most variance and relationships between the variables, which aids in understanding the key determinants of IPM.
What does the Biplot analysis show in this study?
-The Biplot analysis shows the relationships between the variables and the objects (counties and cities), highlighting their proximity or distance and illustrating the correlation between the IPM indicators.
What was the significance of the correlation results found between variables?
-The correlation results reveal that some variables, like X2 and X3, are highly correlated, meaning they influence each other strongly. Additionally, there is a negative correlation between X1 (life expectancy) and X4 (per capita expenditure), indicating that areas with higher life expectancy tend to have lower per capita expenditures.
How were the results of the study used to interpret the characteristics of different regions in Central Java?
-The results were used to identify regions that share similar characteristics based on IPM indicators, such as counties with high life expectancy or those with higher educational attainment. For example, Boyolali, Sragen, and Semarang were identified as having similar characteristics, while Brebes stood out due to differing characteristics.
Outlines
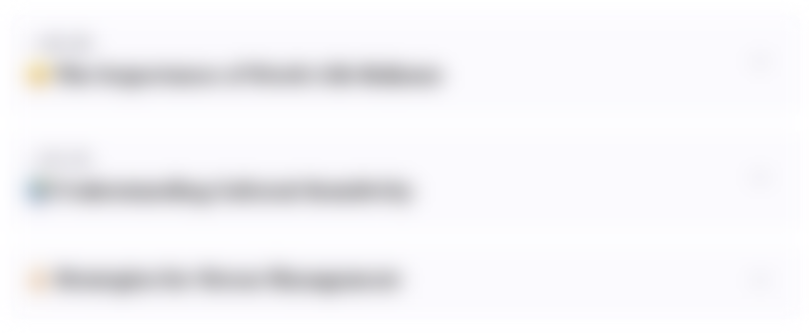
Этот раздел доступен только подписчикам платных тарифов. Пожалуйста, перейдите на платный тариф для доступа.
Перейти на платный тарифMindmap
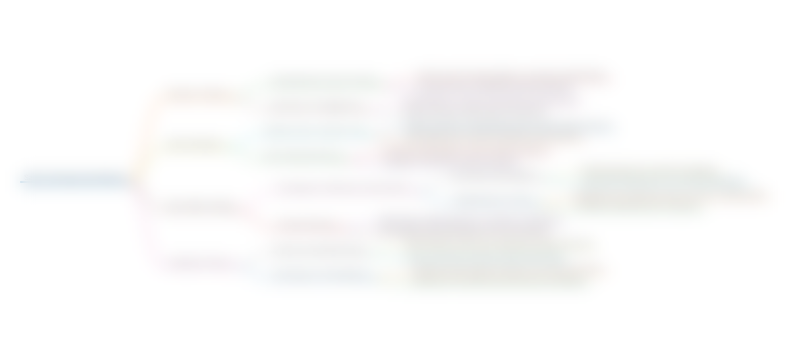
Этот раздел доступен только подписчикам платных тарифов. Пожалуйста, перейдите на платный тариф для доступа.
Перейти на платный тарифKeywords
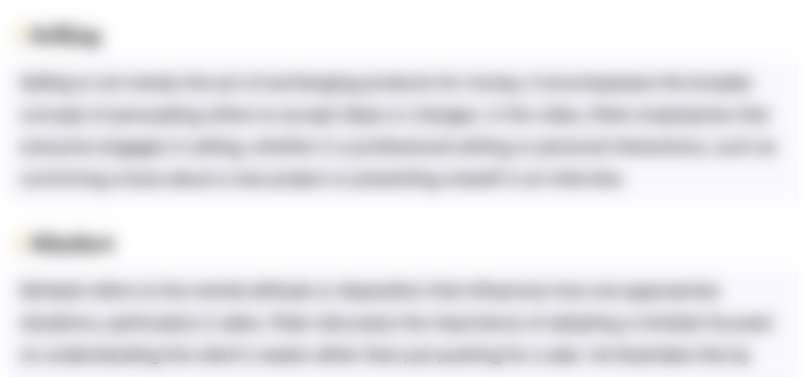
Этот раздел доступен только подписчикам платных тарифов. Пожалуйста, перейдите на платный тариф для доступа.
Перейти на платный тарифHighlights
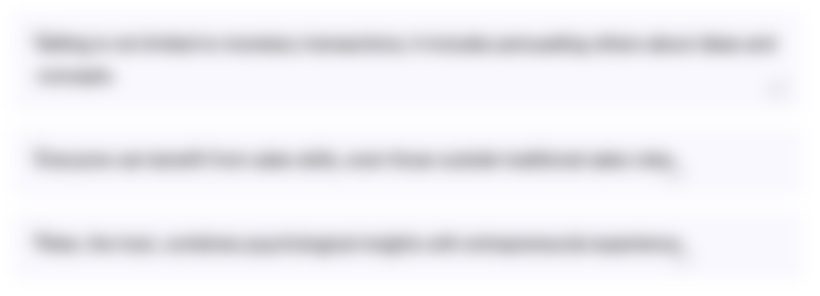
Этот раздел доступен только подписчикам платных тарифов. Пожалуйста, перейдите на платный тариф для доступа.
Перейти на платный тарифTranscripts
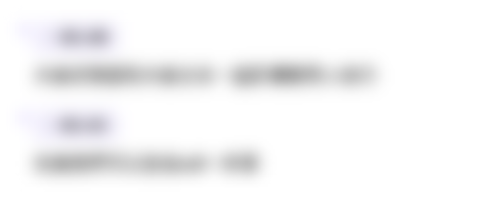
Этот раздел доступен только подписчикам платных тарифов. Пожалуйста, перейдите на платный тариф для доступа.
Перейти на платный тарифПосмотреть больше похожих видео
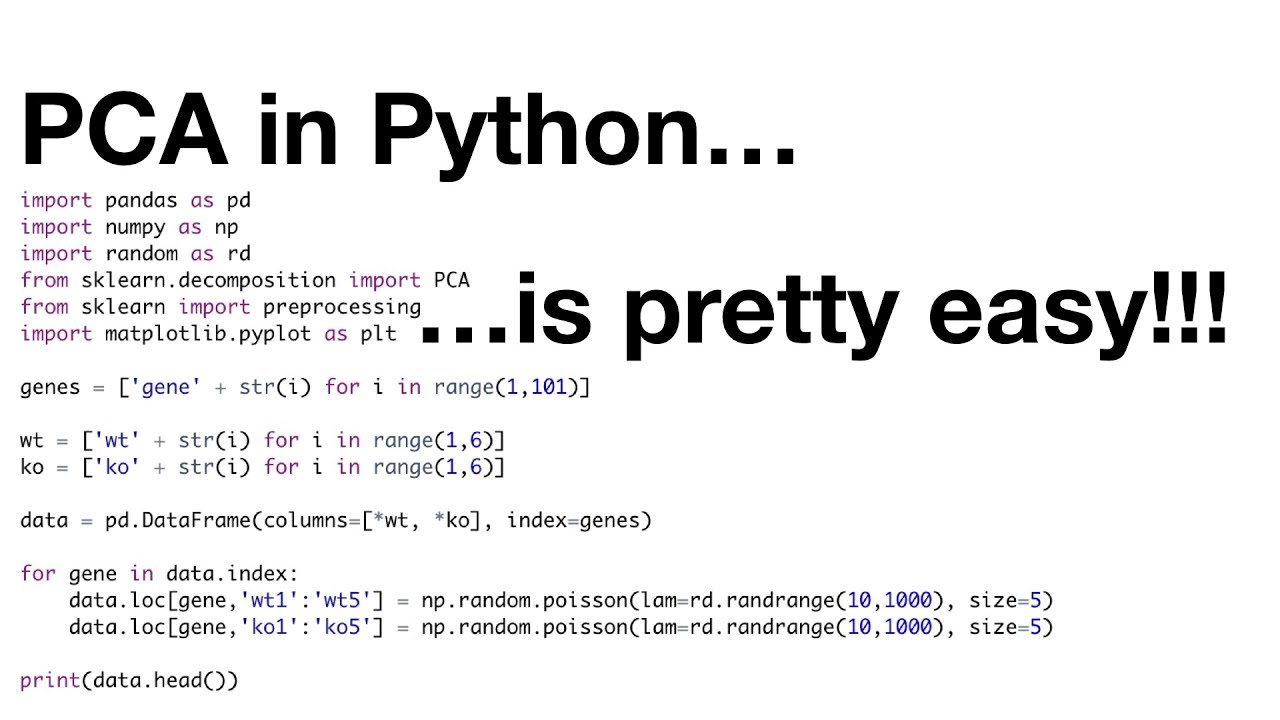
StatQuest: PCA in Python

Pembahasan Analisis Komponen Utama (Part 1) | Review Konsep
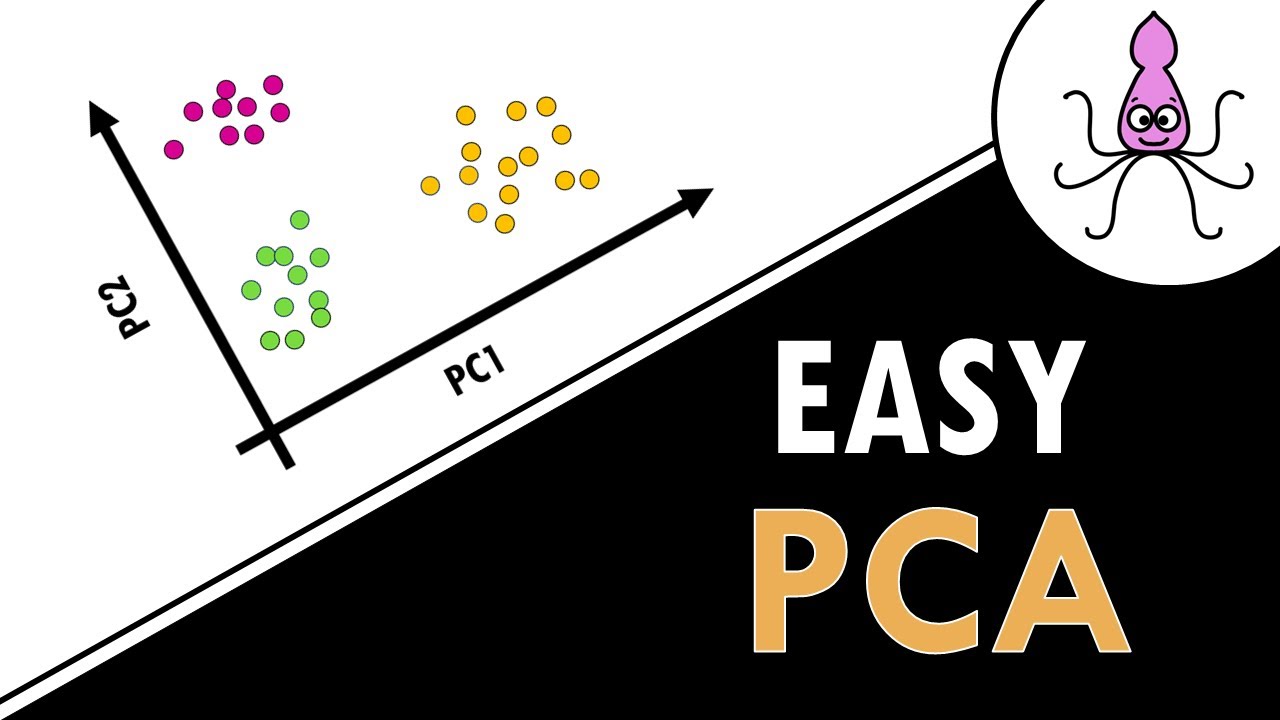
Principal Component Analysis (PCA) - easy and practical explanation
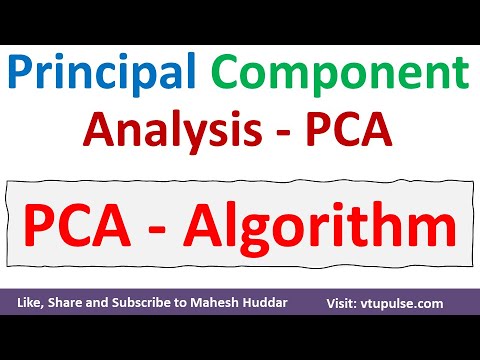
PCA Algorithm | Principal Component Analysis Algorithm | PCA in Machine Learning by Mahesh Huddar
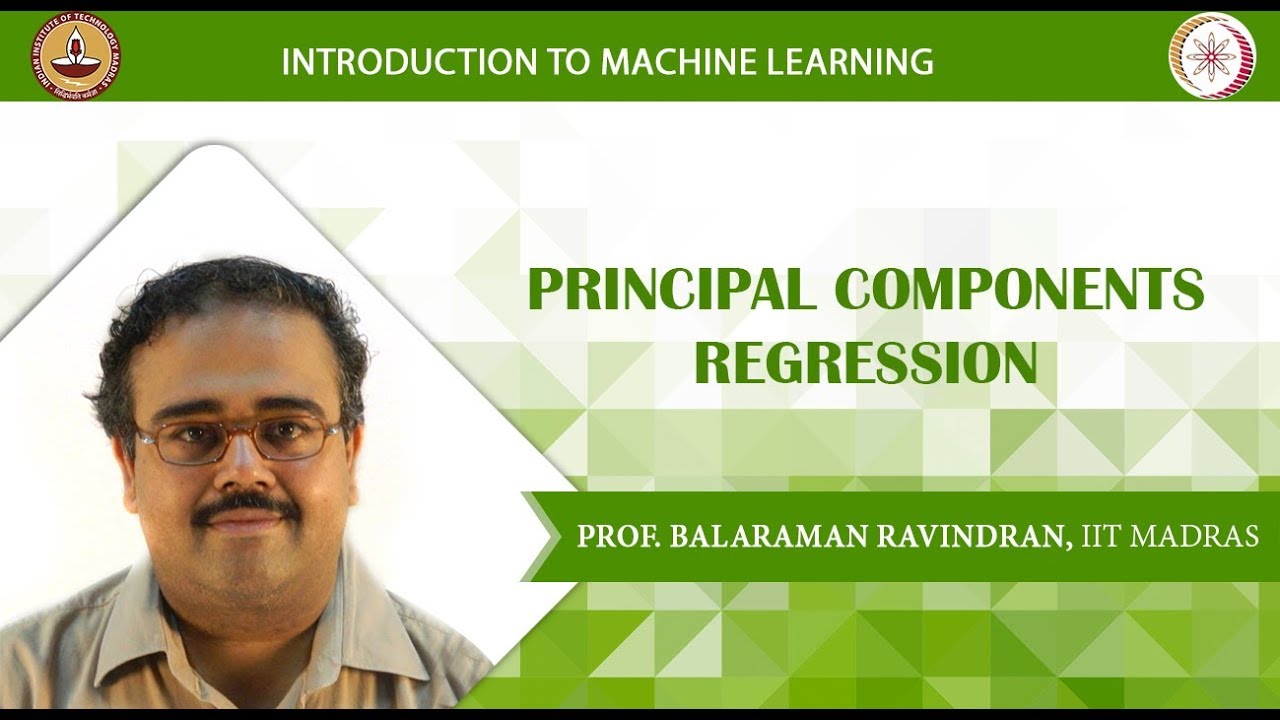
Week 3 Lecture 13 Principal Components Regression
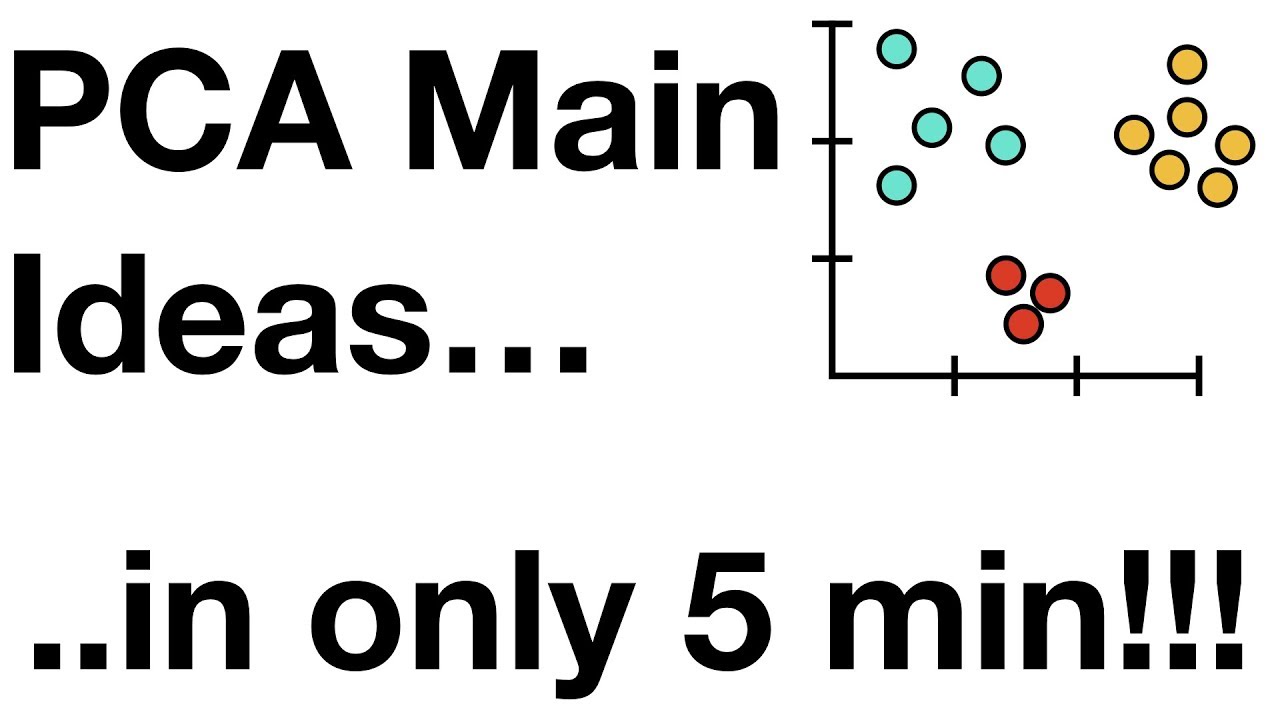
StatQuest: PCA main ideas in only 5 minutes!!!
5.0 / 5 (0 votes)