Structural Equation Modeling SEM dengan Smart Partial Least Square (PLS)
Summary
TLDRThis video delves into the evolution of climate change activism, highlighting pivotal figures and global movements. It addresses the challenges activists face while showcasing innovative solutions they propose. Viewers will also learn practical steps to engage in the climate movement, empowering them to make a difference.
Takeaways
- 😀 Structural Equation Modeling (SEM) using Partial Least Squares (PLS) is a multivariate predictive statistical analysis technique.
- 😀 PLS allows for the analysis of multiple endogenous and exogenous variables simultaneously in structural models.
- 😀 One key advantage of PLS is that it does not require multivariate normal distribution of data, making it flexible for various datasets.
- 😀 PLS can be applied to different measurement scales, including nominal, ordinal, interval, and ratio scales.
- 😀 Small sample sizes can be analyzed with PLS, which is beneficial when collecting large datasets is challenging.
- 😀 PLS serves both as a confirmatory factor analysis and a multiple regression analysis method.
- 😀 The outer model in PLS connects indicators with latent variables, while the inner model connects the latent variables themselves.
- 😀 Reliability and validity tests, such as Composite Reliability and Cronbach's Alpha, are essential for assessing the quality of the measurement model.
- 😀 Goodness-of-fit measures like Q-squared relevance are used to evaluate the predictive relevance of the model.
- 😀 Hypothesis testing in PLS can be performed using t-tests, where significant relationships between variables are assessed through p-values.
Q & A
What is Partial Least Squares (PLS) used for in Structural Equation Modeling (SEM)?
-PLS is a multivariate predictive analysis technique that allows researchers to analyze multiple dependent and independent variables simultaneously, confirming theories and exploring new relationships.
What are the key advantages of using PLS?
-The key advantages include no requirement for multivariate normal distribution, flexibility with measurement scales, effectiveness with small sample sizes, capability for both theory confirmation and exploration, and handling of both reflective and formative constructs.
What is the difference between reflective and formative variables?
-Reflective variables share a common cause and are mutually exclusive, while formative variables contribute to the definition of a construct and do not necessarily have intercorrelation.
How does PLS handle data that does not meet normality assumptions?
-PLS does not require the assumption of normal distribution, making it suitable for datasets that may violate this assumption, allowing for more flexible analysis.
What software can be used for conducting PLS analysis?
-Software options for PLS analysis include SmartPLS, VisualPLS, PLS-Graph, and others, with SmartPLS being highlighted for its user-friendly interface.
What steps are involved in preparing data for PLS analysis?
-Data must be organized in a compatible format (like CSV), and variables should be clearly defined with their respective indicators before being imported into the PLS software.
What does a factor loading value above 0.5 indicate?
-A factor loading value above 0.5 indicates that the indicator is valid and strongly correlates with the latent variable it represents.
How is the reliability of a model assessed in PLS?
-Reliability is assessed using Cronbach's alpha and composite reliability. Values above 0.7 for these measures indicate a reliable model.
What is the significance of a p-value less than 0.05 in hypothesis testing?
-A p-value less than 0.05 indicates that the relationship being tested is statistically significant, leading to the acceptance of the hypothesis.
What are the implications of using PLS for exploratory research?
-Using PLS for exploratory research allows researchers to identify potential relationships between variables that may not have strong theoretical foundations, enabling new insights and hypotheses to be developed.
Outlines
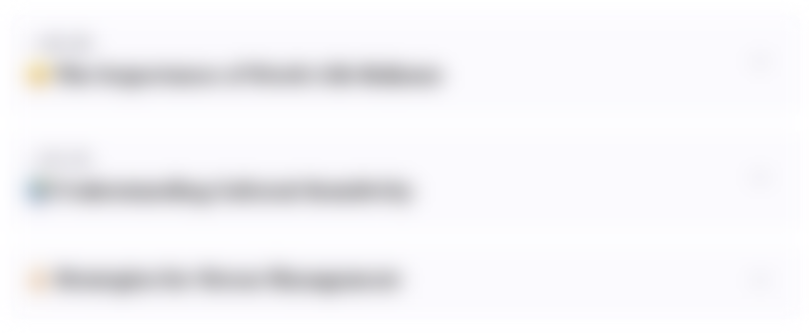
Этот раздел доступен только подписчикам платных тарифов. Пожалуйста, перейдите на платный тариф для доступа.
Перейти на платный тарифMindmap
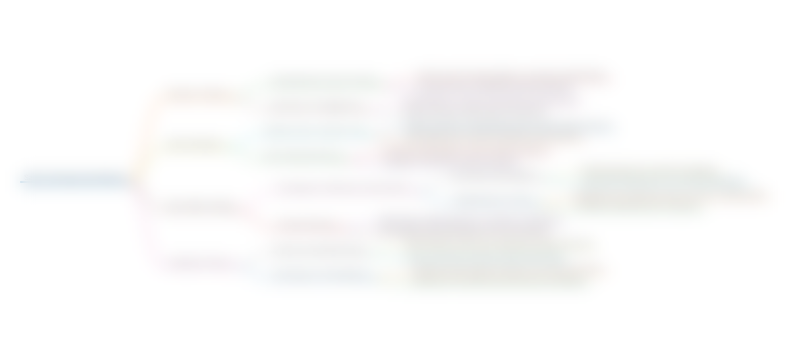
Этот раздел доступен только подписчикам платных тарифов. Пожалуйста, перейдите на платный тариф для доступа.
Перейти на платный тарифKeywords
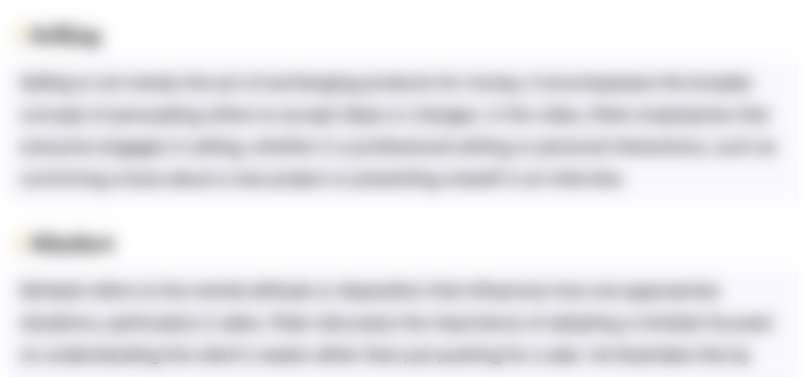
Этот раздел доступен только подписчикам платных тарифов. Пожалуйста, перейдите на платный тариф для доступа.
Перейти на платный тарифHighlights
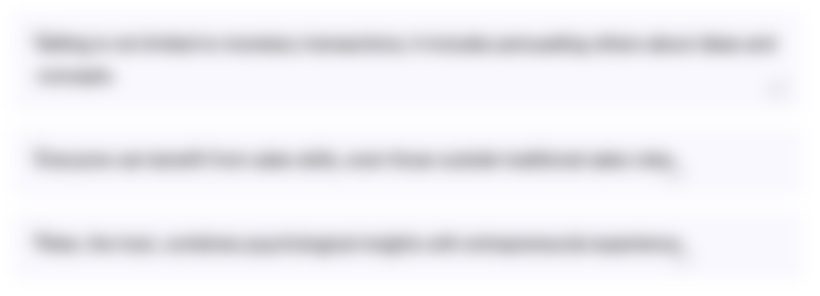
Этот раздел доступен только подписчикам платных тарифов. Пожалуйста, перейдите на платный тариф для доступа.
Перейти на платный тарифTranscripts
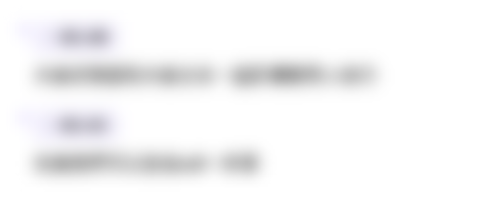
Этот раздел доступен только подписчикам платных тарифов. Пожалуйста, перейдите на платный тариф для доступа.
Перейти на платный тарифПосмотреть больше похожих видео
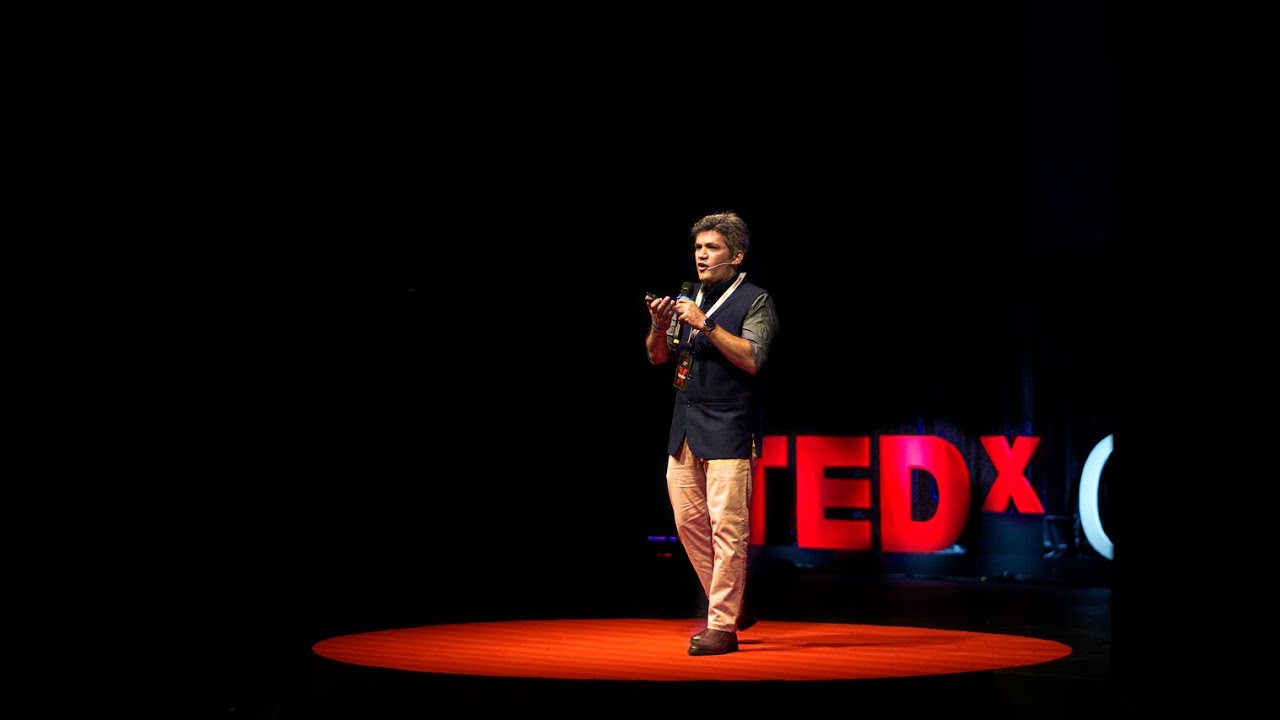
Social Media Driving Social Change | Md. Abdul Quayyum | TEDxGulshan
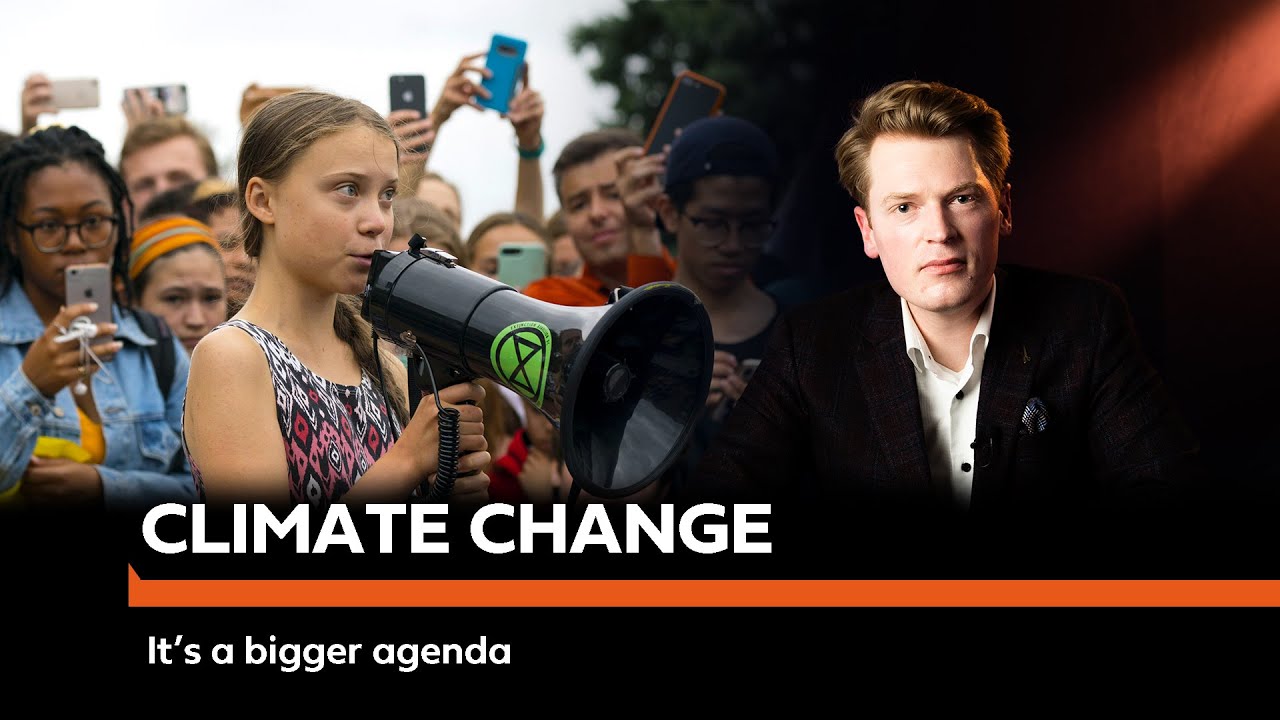
The Truth of It | What’s so wrong about it? | Climate Change Part I | Ep. 23
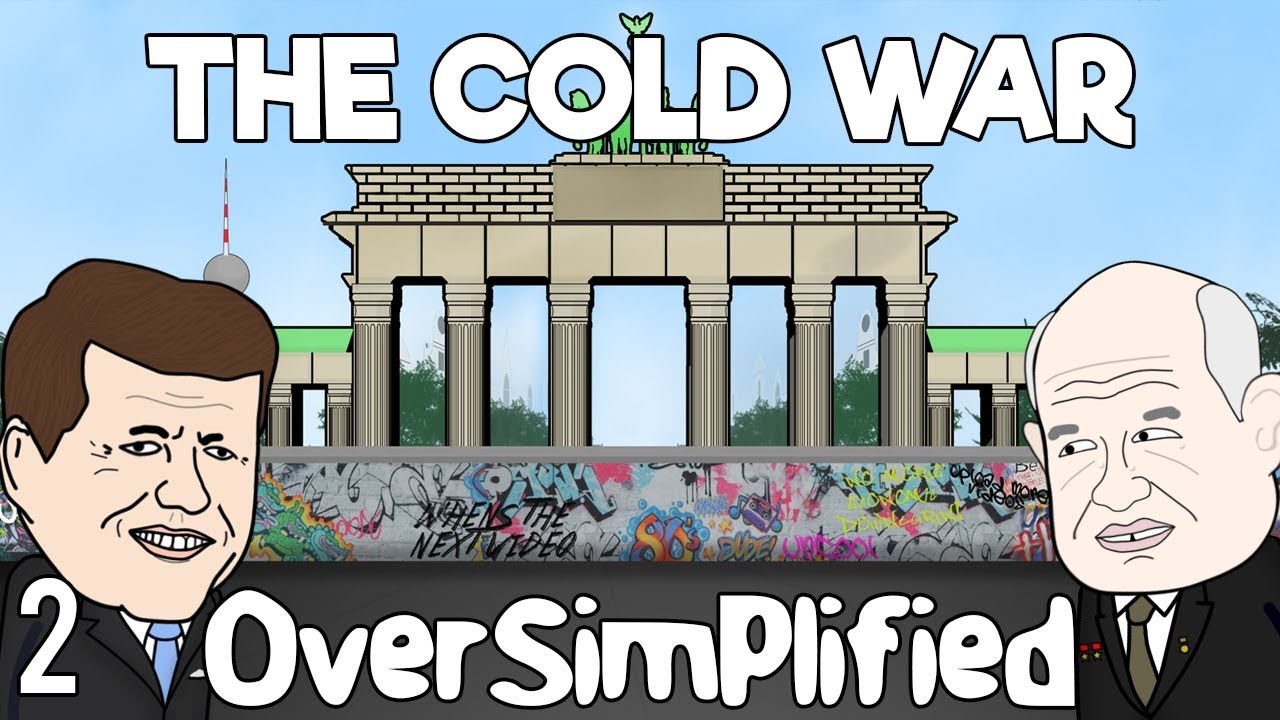
The Cold War - OverSimplified (Part 2)

Climate carnage: whose job is it to halt the climate crisis?

ridle pra smtr
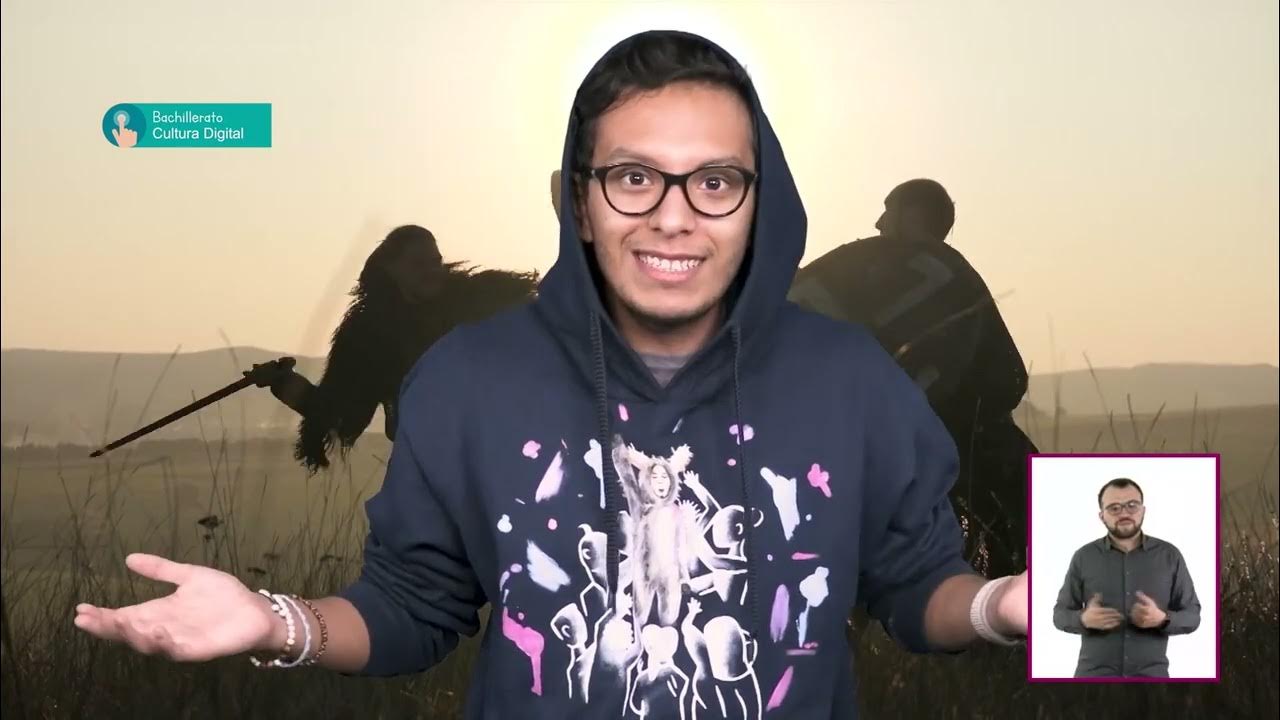
T3 » Bachillerato » Cultura Digital » No hay pretexto para dejar de participar
5.0 / 5 (0 votes)