The carbon footprint of Transformers
Summary
TLDRThe video discusses the carbon footprint of training AI models like transformers, highlighting key factors such as energy source, training time, and hardware efficiency. Renewable energy sources like solar or wind significantly reduce emissions, while non-renewable sources, such as coal, increase them. Additionally, choosing low-carbon cloud instances, using pre-trained models, and optimizing hyperparameters can mitigate carbon emissions. Tools like 'machine learning submissions calculator' and 'codecarbon' help track emissions during AI model training, encouraging conscious and eco-friendly practices in AI development.
Takeaways
- 🌍 The carbon footprint of training AI models depends largely on the type of energy used. Renewable sources like solar or wind generate very little carbon, while non-renewable sources like coal have a much higher carbon impact.
- ⏳ Longer training times result in higher energy consumption, which increases carbon emissions, especially for large models trained over extended periods.
- ⚙️ Efficient hardware usage, particularly GPUs, can significantly reduce energy consumption and lower the carbon footprint during model training.
- 📊 Location matters: For example, cloud computing in Mumbai emits 920g of CO2 per kilowatt-hour, while in Montreal, it's only 20g, making location a critical factor in emissions.
- 🔄 Using pre-trained models is akin to recycling in machine learning, reducing the need for extensive retraining and lowering carbon emissions.
- 🎯 Fine-tuning pre-trained models instead of training from scratch can help minimize both training time and energy consumption, further reducing emissions.
- 🛠️ Start with small experiments and ensure stable code to avoid wasting resources on debugging later in large-scale training.
- 🎲 Random search for hyperparameter tuning can be as effective as grid search, while using fewer resources and lowering the overall carbon footprint.
- 💡 Strubell et al.'s 2019 paper highlights that most emissions come from exhaustive neural architecture searches and hyperparameter tuning, not from training a single model.
- 📈 Tools like the 'machine learning submissions calculator' and 'codecarbon' can help track and estimate the carbon footprint of model training, making it easier to manage and reduce emissions.
Q & A
What factors contribute to the carbon footprint of training AI models?
-The carbon footprint of training AI models depends on the type of energy used, the duration of training, and the hardware efficiency. Renewable energy sources produce significantly less carbon than non-renewable sources, and longer training times or inefficient hardware increase energy consumption and carbon emissions.
How does the energy source impact the carbon emissions during AI training?
-The energy source greatly impacts carbon emissions. For example, using renewable energy like solar or wind results in very low emissions, while using coal or other fossil fuels significantly increases the carbon footprint.
What role does training duration play in the carbon emissions of AI models?
-The longer an AI model is trained, the more energy is consumed, which in turn increases carbon emissions. This effect is amplified if large models are trained for extended periods, such as weeks or months.
How does hardware efficiency affect the carbon footprint of training AI models?
-Hardware efficiency is crucial for reducing energy consumption. Efficient GPUs can process data faster and more effectively, lowering the overall energy required for training and, subsequently, the carbon emissions.
Why is the location of cloud instances important for the carbon footprint?
-The location of cloud instances affects the carbon footprint due to regional differences in carbon intensity. For example, Mumbai's cloud instances emit 920 grams of CO2 per kilowatt-hour, while Montreal's emit only 20 grams per kilowatt-hour, resulting in a 40-fold difference in emissions for the same workload.
How can using pre-trained models help reduce the carbon footprint of AI training?
-Using pre-trained models, rather than training from scratch, reduces the need for extensive computational resources. Fine-tuning pre-trained models is akin to recycling, as it minimizes additional energy consumption and carbon emissions.
What is the importance of starting with small experiments in AI training?
-Starting with small experiments helps in debugging and ensuring that the code is stable before scaling up. This approach prevents wasting energy on long, flawed training sessions, thus reducing unnecessary carbon emissions.
What are the benefits of random search for hyperparameters over grid search in AI training?
-Random search can be just as effective as grid search in finding the optimal hyperparameter configuration, while testing fewer combinations. This reduces computational costs and the associated carbon emissions by narrowing down the number of trials.
How significant are the carbon emissions of training a transformer model with 200 million parameters?
-Training a transformer model with 200 million parameters produces around 87 kg (200 pounds) of CO2. While substantial, this is far from the emissions equivalent to five cars over their lifetime or even a single transatlantic flight, as some earlier reports suggested.
What tools can help estimate the carbon footprint of AI training?
-There are tools like the 'Machine Learning Emissions Calculator,' where users input details like hardware type, location, and duration to estimate emissions. Additionally, 'codecarbon' can track emissions programmatically by running alongside the training process and providing a CSV report of CO2 emissions.
Outlines
🌍 Understanding the Carbon Footprint of AI Models
This paragraph delves into the carbon footprint generated by training AI models, specifically transformers. It mentions that some reports suggest training a single AI model could produce emissions equivalent to five cars over their lifetime. The truth depends on various factors, with the type of energy used being a key determinant. Renewable energy sources such as solar, wind, and hydropower emit little to no carbon, while non-renewable sources like coal have a much larger carbon footprint. The paragraph also highlights the impact of training duration, energy efficiency of hardware like GPUs, and the importance of running models efficiently to minimize emissions.
⚡ Regional Carbon Intensity of Cloud Computing
Here, the focus is on the carbon intensity of cloud computing, using Mumbai, India, and Montreal, Canada, as examples. A cloud instance in Mumbai emits 920 grams of CO2 per kilowatt-hour, compared to only 20 grams in Montreal, making a significant difference in carbon emissions depending on location. The paragraph emphasizes the importance of choosing a low-carbon cloud instance, especially for intensive, long-duration model training, which can multiply the emissions drastically if conducted in high-carbon-intensity regions.
🔄 Benefits of Using Pre-Trained Models and Fine-Tuning
This section compares using pre-trained models to recycling in machine learning. Instead of training a model from scratch, fine-tuning an existing model can greatly reduce carbon emissions. It also discusses starting small when experimenting with models to avoid unnecessary energy consumption. Ensuring the stability of code before long training sessions helps avoid bugs and wasted energy. Random search for hyperparameter tuning is recommended over grid search as it’s equally effective and more energy-efficient.
🚗 Carbon Impact of Model Architecture Search
This paragraph revisits the original 2019 paper by Strubell et al. that compared training a large transformer model to the carbon footprint of five cars. It clarifies that a 200-million-parameter transformer emits about 200 pounds of CO2, which is far less than the emissions equivalent to five cars or even a transatlantic flight. The real concern arises during model architecture search and extensive hyperparameter tuning, where emissions can escalate significantly. Conscious decisions and efficient practices can help mitigate this impact.
🛠 Tools to Measure and Reduce Your Carbon Emissions
This section introduces two tools for tracking carbon emissions: the 'Machine Learning Submissions Calculator' and 'CodeCarbon.' These tools allow researchers to estimate their emissions based on hardware usage, duration, and location. CodeCarbon, in particular, runs alongside code execution and provides CSV reports to help users measure their carbon footprint. It’s integrated into platforms like AutoNLP, making it easier for users to monitor emissions during model training and deployment.
📊 Visualizing and Tracking Your Carbon Emissions
This paragraph expands on the capabilities of CodeCarbon, describing how it offers a visual interface to compare emissions with common activities like driving a car or watching TV. It emphasizes the utility of this tool in helping users gauge the scale of their emissions and its integration into machine learning platforms like AutoNLP to facilitate emission tracking throughout model training and deployment.
Mindmap
Keywords
💡Carbon Footprint
💡Renewable Energy
💡GPU Efficiency
💡Training Duration
💡Pre-trained Models
💡Hyperparameter Tuning
💡Location-based Emissions
💡Machine Learning Emissions Calculator
💡CodeCarbon
💡Neural Architecture Search
Highlights
Training an AI model can emit as much CO2 as five cars over their lifetime, depending on the type of energy used.
The carbon footprint depends on the energy source: renewable energy like solar, wind, and hydroelectric power emits very little carbon.
Non-renewable energy sources, such as coal, produce a much higher carbon footprint due to greenhouse gas emissions.
Training time matters: longer training means more energy consumption, which increases carbon emissions.
The hardware used is also important, as some GPUs are more energy-efficient than others, reducing carbon emissions.
Choosing a cloud computing instance with low-carbon emissions is crucial, as regions vary significantly in their carbon intensity.
Cloud instances in regions like Montreal, with only 20 grams of CO2 per kilowatt-hour, produce 40 times less carbon than regions like Mumbai.
Using pre-trained models is like recycling in machine learning—it saves energy and reduces emissions compared to training from scratch.
Fine-tuning the last few layers of a model instead of training a large model from scratch can help reduce carbon emissions.
Starting with small experiments to debug and ensure code stability helps avoid unnecessary long training periods, which consume more energy.
Random search for hyperparameters is as effective as grid search but consumes less energy by testing fewer combinations.
Training a transformer with 200 million parameters emits about 87 kg of CO2, which is far from the emissions equivalent to five cars.
Massive CO2 emissions occur during neural architecture searches and hyperparameter tuning, which can lead to 272 metric tons of CO2.
The 'Machine Learning Submissions Calculator' allows manual tracking of hardware usage and carbon emissions during AI training.
CodeCarbon is a programmatic tool that runs alongside code to estimate and track CO2 emissions, and is integrated into platforms like AutoNLP.
Transcripts
Parlons donc de l'empreinte carbone des transformers.
Vous avez peut-être vu des titres tels que celui-ci
indiquant qu'entraîner un seul modèle d'IA peut entraîner autant d'émissions de CO2
que cinq voitures dans leur vie.
Alors quand est-ce vrai et est-ce toujours vrai ?
En fait, cela dépend de plusieurs choses.
Le plus important, c'est que cela dépend 8 00:00:23,430 --> 00:00:24,960 sur le type d'énergie que vous utilisez.
Si vous utilisez une énergie renouvelable telle que le
solaire, l'éolien, l'hydroélectrique, vous n'éméttez
pas vraiment de carbone du tout. Très, très peu.
Si vous utilisez des sources d'énergie non renouvelables telles que le charbon
alors l'empreinte carbone est beaucoup plus élevée
parce qu'en fait, vous émettez beaucoup de gaz à effet de serre.
Un autre aspect est le temps d'entraînement.
Donc, plus vous entraînez longtemps, plus vous dépensez d'énergie.
Plus vous utilisez d'énergie, plus vous émettez de carbone.
Donc, cela s'additionne.
Surtout si vous entraînez de grands modèles
pendant des heures, des jours et des semaines.
Le matériel que vous utilisez a également son importance
car certains GPU, par exemple, sont plus efficaces
que d'autres et donc utiliser des GPU
efficiement, correctement, à 100% tout le temps,
peut vraiment réduire la consommation d'énergie que vous avez.
Et encore une fois, réduire votre empreinte carbone.
Il y a aussi d'autres aspects comme l'IO
comme les données, etc., etc.
Mais ce sont les trois principaux sur lesquels vous devez vous concentrer.
Donc quand je parle de sources d'énergie et d'intensité de carbone
Qu'est-ce que cela signifie vraiment ?
Donc si vous regardez en haut de l'écran
vous avez une empreinte carbone
d'une instance de cloud computing à Mumbai en Inde
qui émet 920 grammes de CO2 par kilowattheure.
C'est presque un kilo
de CO2 par kilowattheure d'électricité utilisé.
Si vous comparez cela avec Montréal au Canada,
où je suis en ce moment, 20 grammes de CO2 par kilo heure.
C'est donc une très, très grande différence.
Près de 40 fois plus de carbone émis
à Mumbai qu'à Montréal.
Et donc cela peut vraiment, vraiment s'accumuler.
Si vous entraînez un modèle pendant plusieurs semaines, par exemple
vous multipliez par 40
le carbone que vous émettez.
Donc, choisir la bonne instance,
choisir une instance de calcul à faible émission de carbone,
est vraiment la chose la plus importante que vous puissiez faire.
Et c'est là que cela peut vraiment s'accumuler
si vous vous entrainez de manière très intensive
dans une région à forte intensité de carbone.
D'autres éléments à prendre en compte, par exemple
utiliser des modèles pré-entraînés.
C'est l'équivalent du recyclage pour l'apprentissage automatique.
Lorsque vous disposez de modèles pré-entraînés, vous pouvez les utiliser.
Vous n'émettez pas de carbone du tout.
Vous ne ré-entraînez rien.
Donc c'est aussi faire ses devoirs
de regarder ce qui existe déjà.
Finetuner au lieu d'entraîner à partir de zéro.
Donc, une fois de plus,
si vous trouvez un modèle qui correspond presque à ce dont vous avez besoin
mais pas tout à fait, finetunez les dernières couches
pour qu'il corresponde vraiment à votre objectif.
au lieu d'entrâiner un gros transformer
à partir de zéro. Cela peut vraiment aider.
Commencer par de petites expériences
et déboguer au fur et à mesure.
Cela signifie, par exemple,
comprendre l'encodage des données,
s'assurer qu'il n'y a pas de petits bugs, que vous allez
réalisez, après 16 heures d'entraînement.
Commencer petit et vraiment s'assurer
de ce que vous faites, que votre code est stable.
Et enfin, faire une revue de la littérature pour
choisir des plages d'hyperparamètres pour ensuite poursuivre
avec une recherche aléatoire au lieu d'une recherche par grille.
Les recherches de combinaisons d'hyperparamètres
aléatoires se sont avérés être aussi efficaces
pour trouver la configuration optimale, que la recherche par grille.
Mais évidemment, vous n'essayez pas toutes les combinaisons possibles,
vous n'en essayez qu'un sous-ensemble.
Cela peut donc être très utile.
Donc maintenant, nous revenons
à l'article original de Strubell et al. de 2019,
l'infâme papier sur la vie des cinq voitures.
Si vous regardez
un transformer de 200 millions de paramètres,
son empreinte carbone est d'environ 200 livres [87 kg] de CO2,
ce qui est important.
Mais c'est loin des cinq voitures.
Ce n'est même pas un vol transatlantique.
Ce qui compte vraiment, c'est lorsque vous faites
vos recherches d'architectures neuronales,
quand vous faites le réglage des hyperparamétres,
en essayant toutes les combinaisons possibles,
etc., etc.
Et c'est là que
d'où proviennent les 600 000 livres [272,16 t] de CO2.
C'est donc là que les choses s'additionnent.
Mais si vous faites les choses consciemment et consciencieusement,
alors votre empreinte carbone ne sera pas aussi importante,
comme le laissait entendre le papier. Quelques outils
pour savoir combien de CO2 exactement vous émettez.
Il y a un outil en ligne appelé « machine
learning submissions calculator » qui vous permet
de saisir manuellement, par exemple, le matériel que vous avez utilisé,
le nombre d'heures pendant lesquelles vous l'avez utilisé,
où il était situé : localement ou dans le cloud.
Et puis il va vous donner une estimation
de la quantité de CO2 que vous avez émise.
Un autre outil qui fait cela de manière programmatique,
s'appelle codecarbon.
Vous pouvez l'installer avec pip, vous pouvez aller sur leur GitHub,
et essentiellement il s'exécute en parallèle de votre code.
Donc, vous l'appelez
et ensuite vous faites tous vos entraînements.
Et puis à la fin, il va vous donner une estimation :
un fichier CSV contenant une estimation de vos émissions.
Et ça va vous permettre de faire des comparaisons.
Il y a une interface visuelle où vous pouvez
comparer avec la conduite d'une voiture ou la télévision.
Ainsi, cela peut vous donner une idée
de la portée de vos émissions également.
Et en fait, codecarbon est déjà intégré dans AutoNLP.
et j'espère que les gens l'utiliseront
pour suivre facilement leurs émissions tout au long
de l'entraînement et le déploiement des transformers.
Посмотреть больше похожих видео

Como o resultado é apresentado na PEC Calc? (Parte 5 da série)
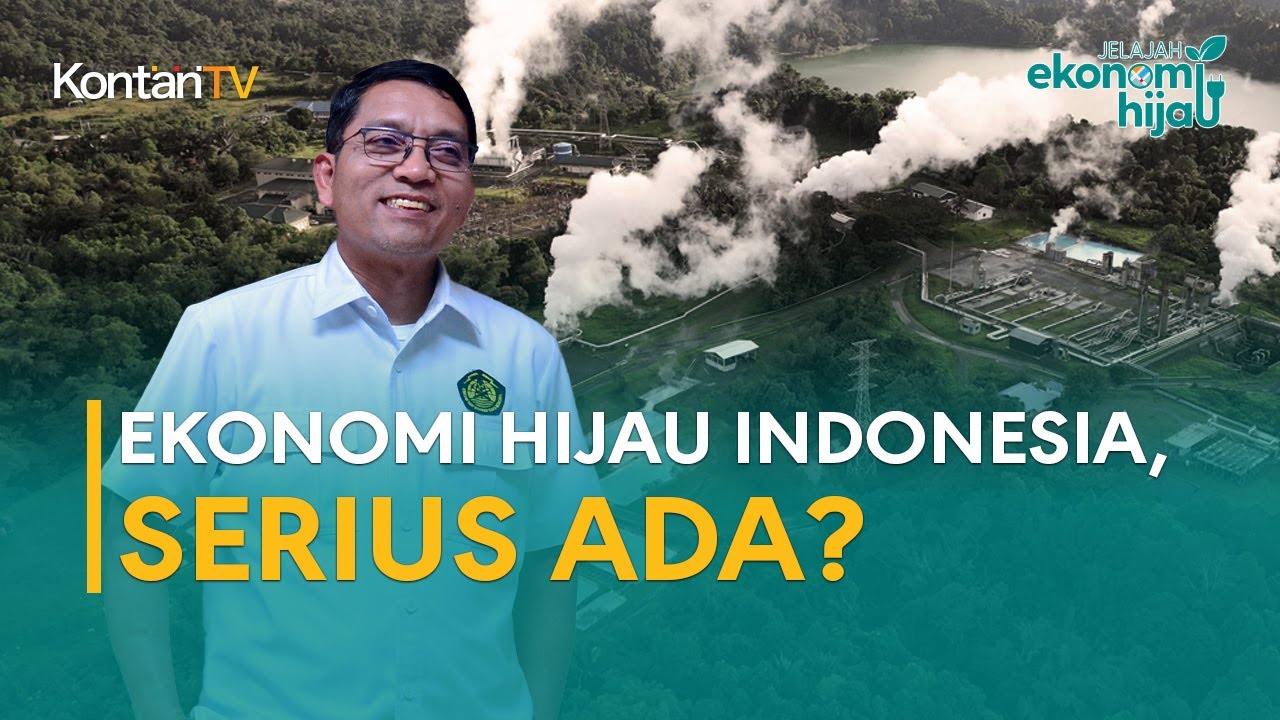
Ekonomi Hijau Indonesia, Serius Ada?
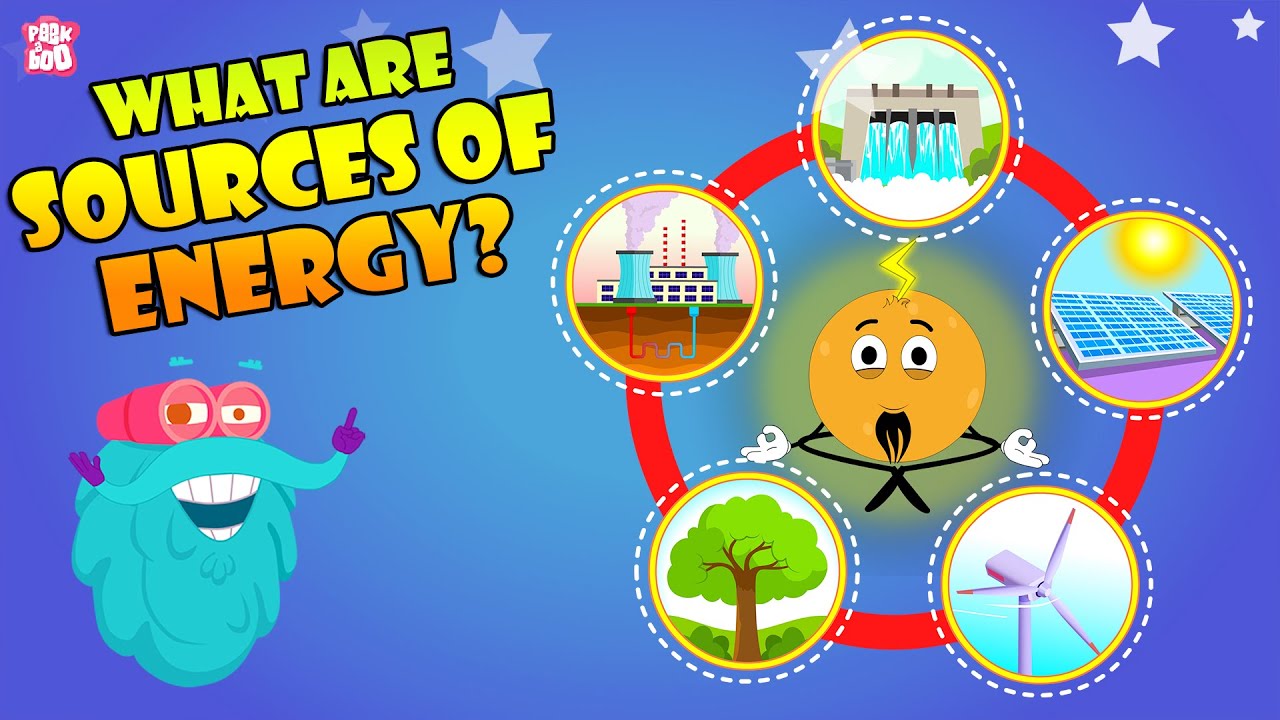
What Are Sources of Energy? | Energy Explained | The Dr Binocs Show | Peekaboo Kidz

Innovative energy technology | Microsoft #TechTalk

L'Intelligenza Artificiale inquina?
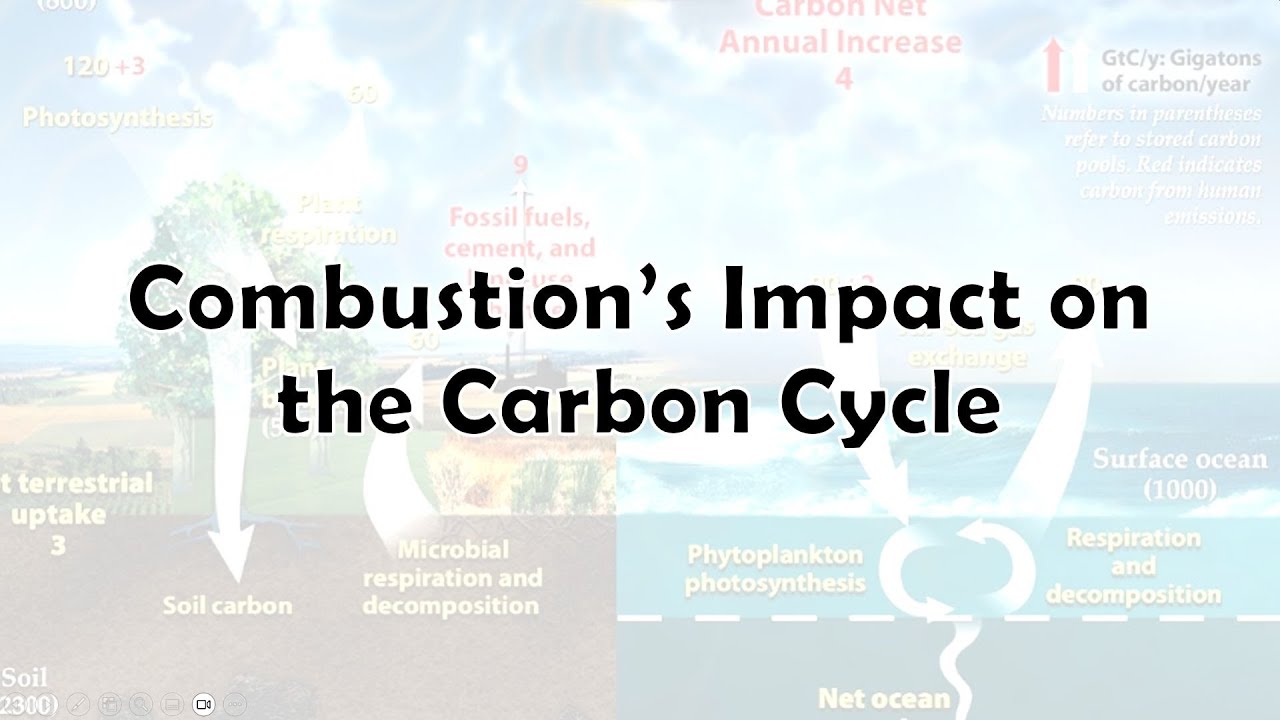
Combustion's Impact on the Carbon Cycle
5.0 / 5 (0 votes)