Book Recommendation System in Python with LLMs
Summary
TLDRIn this informative video, the host guides viewers through the process of coding a book recommendation system using Python and large language models. The system involves creating a vector store to hold vector representations of books, transforming textual data into 4,096-dimensional vectors with the help of LLMs like Llama 2. The video demonstrates how to use a dataset from Kaggle, craft textual representations, and perform similarity searches to recommend the most relevant books. The host also highlights the importance of maintaining consistent data structures for accurate recommendations.
Takeaways
- 📚 The video is about creating a book recommendation system using large language models in Python.
- 🔍 The goal is to build a vector store (Vector Store Service, VSS) that contains vector representations of various books.
- 📈 The books' attributes like title, description, author, and publishing date will be transformed into a textual representation and then into a high-dimensional vector.
- 📈📈 These vectors will be 4,096-dimensional, intelligently derived from the text to represent each book uniquely.
- 🔎 The system performs a similarity search to find the closest vector in the vector space to a newly input book's vector, suggesting the most similar books.
- 🤖 Large language models (LLMs) are used for the embedding process, which is crucial for intelligently converting text into meaningful vectors.
- 🛠️ The video mentions using 'ollama' for convenience, a tool that allows running models locally to get text embeddings.
- 🗃️ A 'faiss' vector store from Facebook is used to store and search through the vectors.
- 📊 The data set used is the 7K books data set from Kaggle, chosen for its inclusion of book descriptions, which are essential for accurate representation.
- 📝 A textual representation function is created to structure the book data in a way that is useful for the LLM.
- 🔑 The video emphasizes the importance of maintaining consistent data structure when building and querying the vector store to ensure accurate recommendations.
Q & A
What is the main topic of the video?
-The main topic of the video is how to code a book recommendation system using large language models in Python.
What is the purpose of building a vector store for the book recommendation system?
-The purpose of building a vector store is to contain vector representations of different books, which will be used for similarity search to recommend books.
What attributes of books are mentioned in the script as being used for the recommendation system?
-The attributes mentioned include title, description, author, publishing date, categories, average rating, and number of pages.
Why are vector representations used instead of raw text for the book recommendation system?
-Vector representations are used because they intelligently encode the text into a high-dimensional space where similarity can be measured numerically, which is not possible with raw text.
What is the dimensionality of the vectors that represent the books in the system?
-The dimensionality of the vectors is 4096, meaning each vector has 4096 numerical values.
Which model is used for text embedding in the video?
-The video uses 'llama 2' for text embedding, which is a large language model that can convert text into a meaningful vector representation.
What is the role of the 'requests' package in the video script?
-The 'requests' package is used to send a request to the 'llama 2' model's API to get the embedding for a given text representation of a book.
What is the data set used in the video for building the book recommendation system?
-The data set used is the '7K books data set' from Kaggle, which includes descriptions along with other attributes of the books.
How does the script handle the process of finding similar books once the vector store is built?
-The script performs a similarity search in the vector store by finding the vector that is closest to the vector representation of a new book, and then recommends the books associated with the closest vectors.
What is the importance of keeping the textual representation structure consistent when building the vector store?
-Keeping the textual representation structure consistent is important because it ensures that the vector store accurately reflects the text and maintains the integrity of the similarity search results.
How does the video demonstrate the effectiveness of the book recommendation system?
-The video demonstrates the effectiveness by showing the process of finding similar books to a given book and displaying the recommended books that are indeed similar in genre or theme.
Outlines
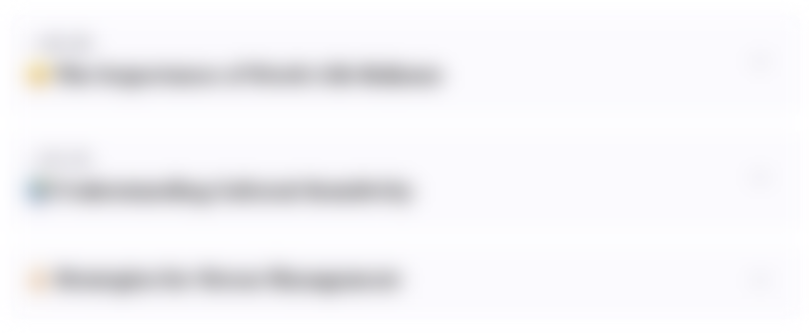
このセクションは有料ユーザー限定です。 アクセスするには、アップグレードをお願いします。
今すぐアップグレードMindmap
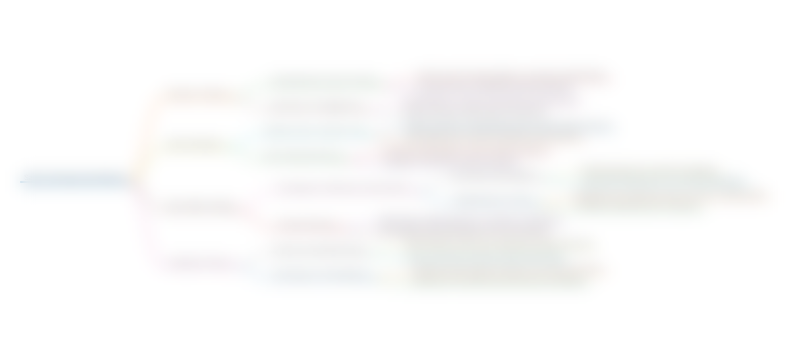
このセクションは有料ユーザー限定です。 アクセスするには、アップグレードをお願いします。
今すぐアップグレードKeywords
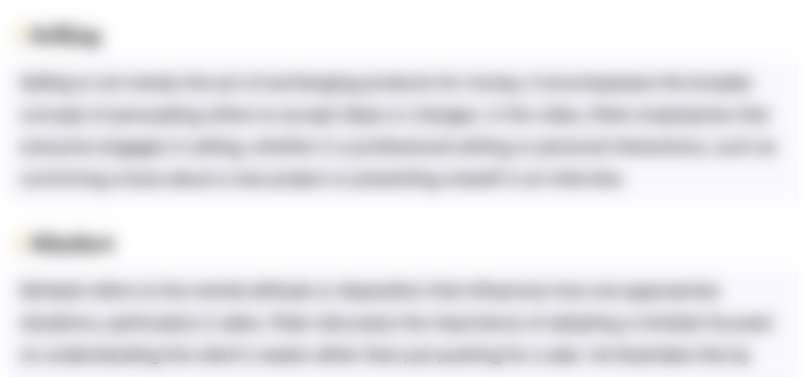
このセクションは有料ユーザー限定です。 アクセスするには、アップグレードをお願いします。
今すぐアップグレードHighlights
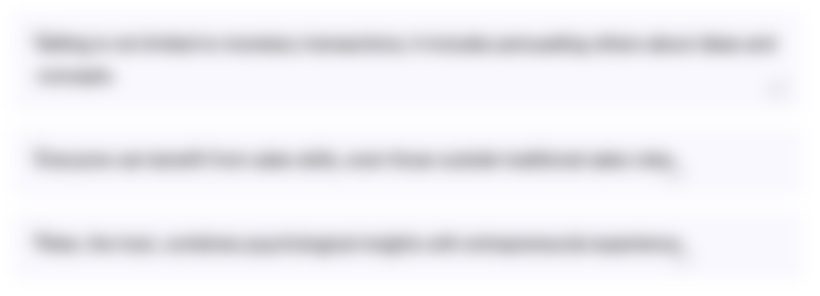
このセクションは有料ユーザー限定です。 アクセスするには、アップグレードをお願いします。
今すぐアップグレードTranscripts
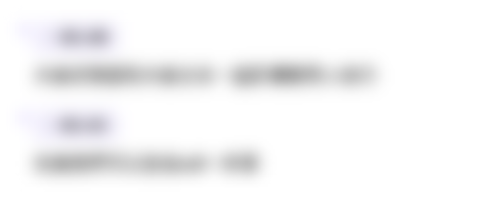
このセクションは有料ユーザー限定です。 アクセスするには、アップグレードをお願いします。
今すぐアップグレード関連動画をさらに表示
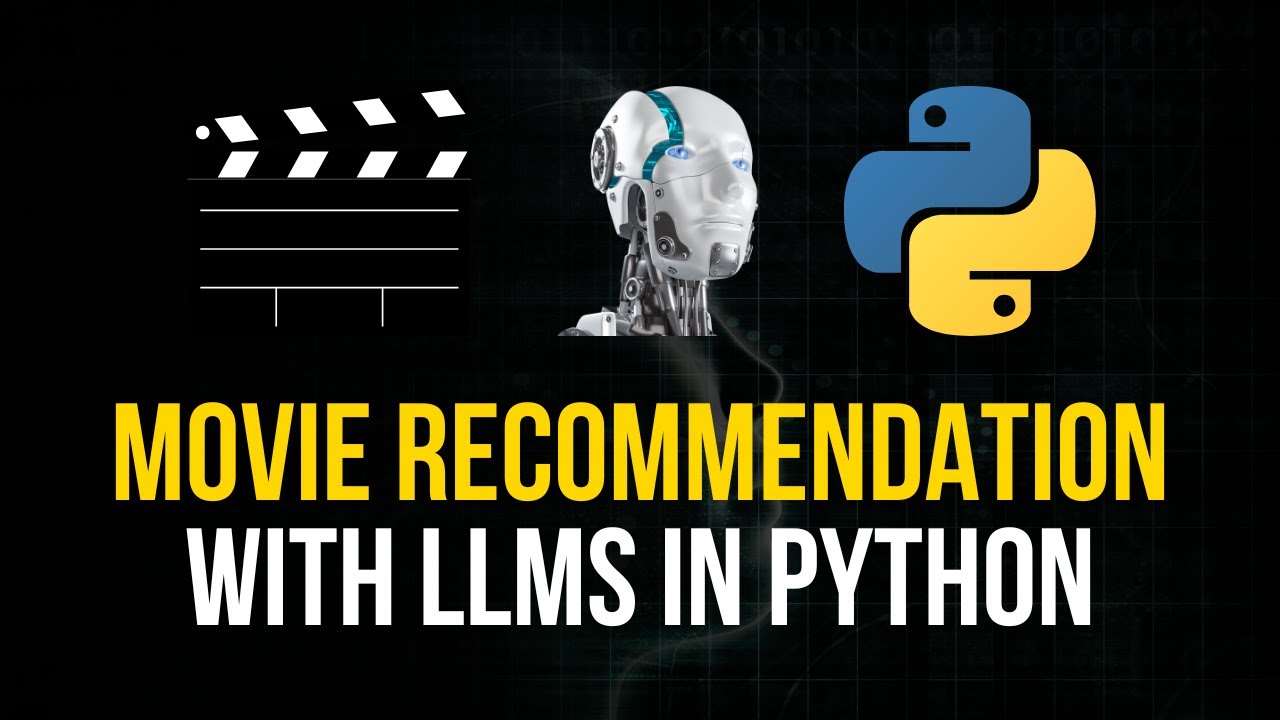
Movie Recommender System in Python with LLMs
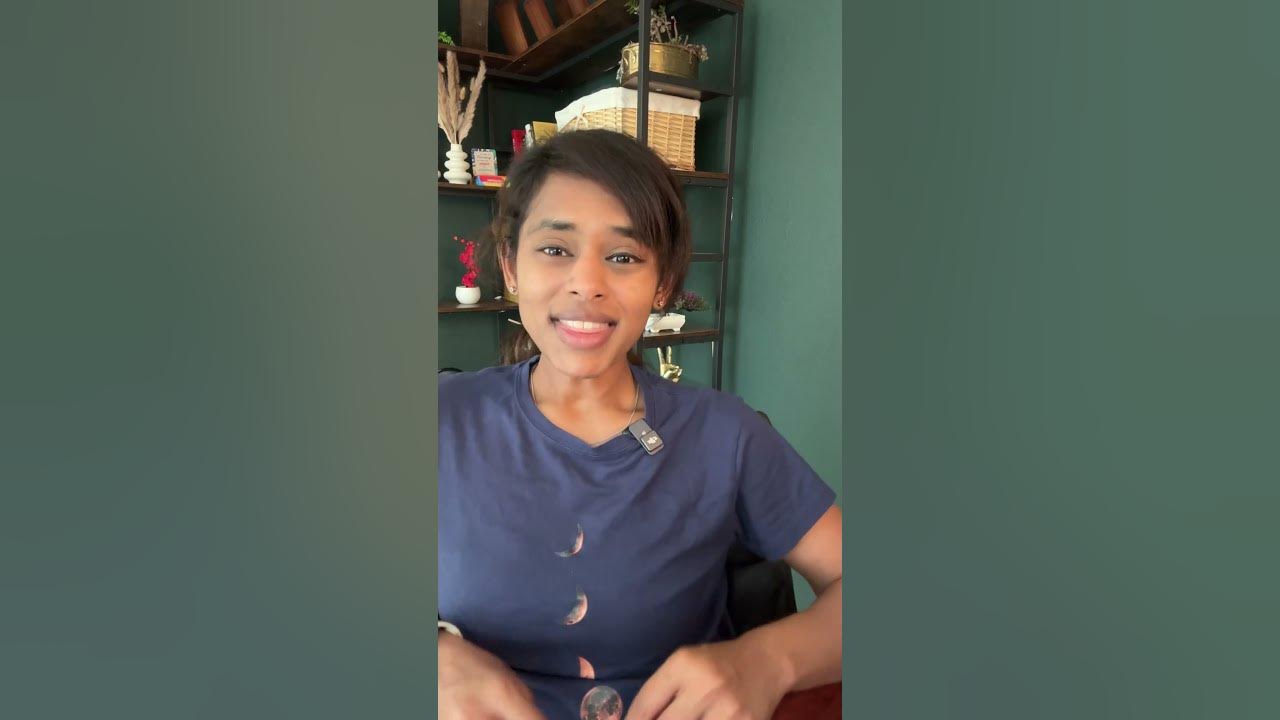
Introduction to Generative AI (Day 10/20) What are vector databases?
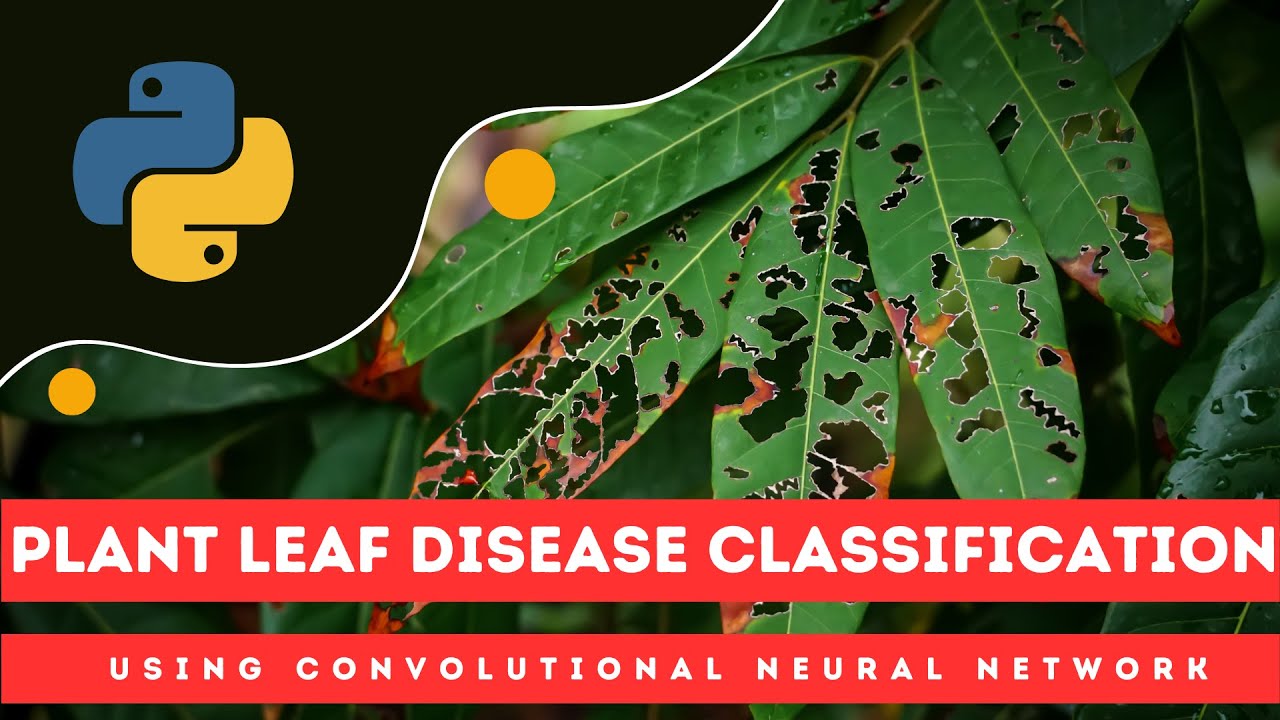
Plant Leaf Disease Detection Using CNN | Python
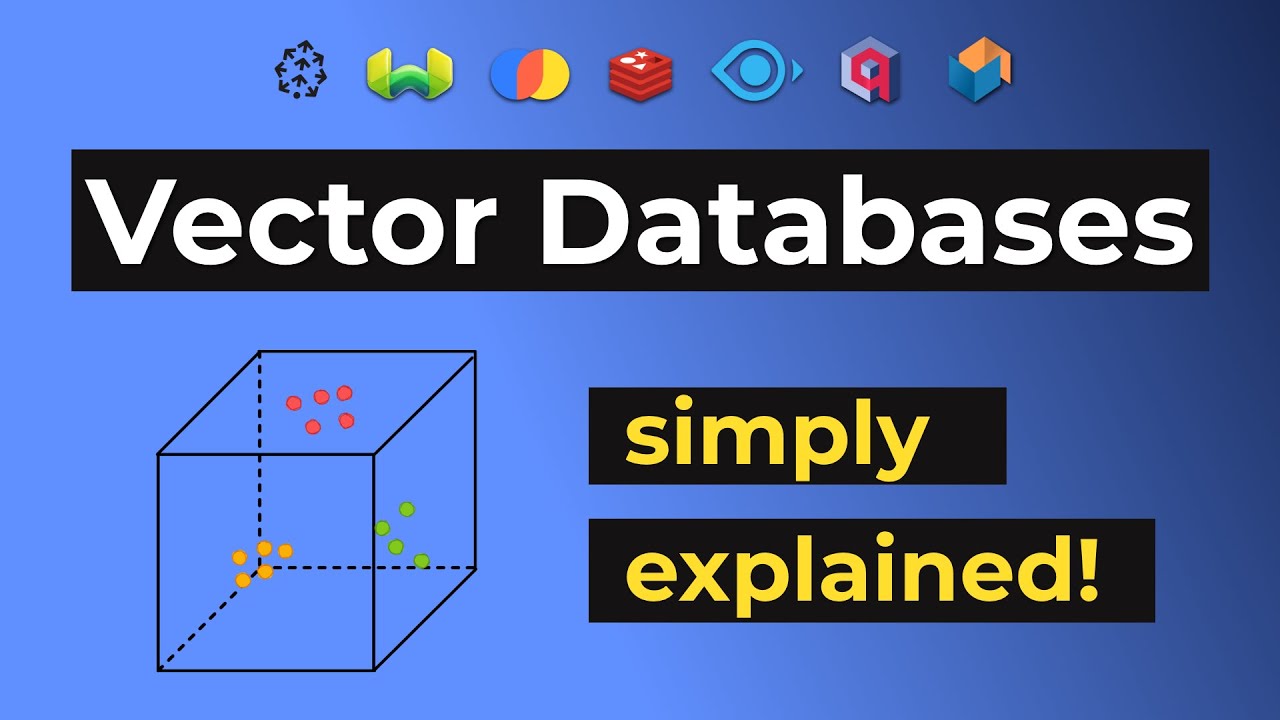
Vector Databases simply explained! (Embeddings & Indexes)
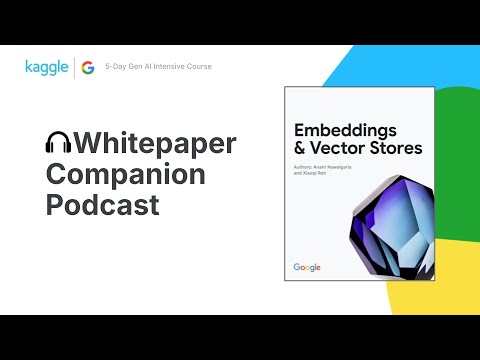
Whitepaper Companion Podcast - Embeddings & Vector Stores

Llama Index ( GPT Index) step by step introduction
5.0 / 5 (0 votes)