Sampling Distributions: Introduction to the Concept
Summary
TLDRThis video script introduces the concept of sampling distributions, essential for statistical inference. It explains that the sampling distribution of a statistic, such as the sample mean, is the probability distribution of that statistic if samples were drawn repeatedly from a population. Using a university class example, the script illustrates how the sample mean varies across different samples and how this variation can be visualized through a histogram, approximating the true sampling distribution. The significance of understanding sampling distributions is highlighted for making statistical inferences about population parameters.
Takeaways
- 📚 The concept of sampling distributions is fundamental to statistical inference techniques.
- 🔍 A sampling distribution represents the probability distribution of a statistic based on repeated sampling from a population.
- 👨🏫 The example of a university class with 16 students illustrates the concept, where the average age is the population parameter.
- 🔢 The true population mean (mu) is an unknown quantity to the professor and is calculated as 239.8125 in the example.
- 🎯 The professor uses a random sample of three students' ages to estimate the unknown population mean (mu).
- 📉 The sample mean is calculated by averaging the ages of the sampled students, providing a point estimate for mu.
- ⚖️ The uncertainty of the sample mean as an estimate for mu is addressed using the sampling distribution of the sample mean.
- 📈 The histogram of sample means, obtained from repeated sampling, closely resembles the true sampling distribution of the sample mean.
- 📊 The sample mean is often distributed approximately normally, which is a common assumption in many statistical analyses.
- 🤔 The sampling distribution helps in understanding the variability of a statistic and its potential closeness to the true population parameter.
- 📝 Mathematical arguments based on the sampling distribution are used to make inferences about population parameters, such as confidence intervals.
Q & A
What is the concept of a sampling distribution?
-A sampling distribution is the probability distribution of a given statistic, showing how that statistic would vary if numerous samples of the same size were drawn from the population.
Why is the concept of a sampling distribution important in statistical inference?
-The concept of a sampling distribution is crucial in statistical inference because it allows us to make inferences about population parameters based on the distribution of a statistic from multiple samples.
What is the difference between a population parameter and a sample statistic?
-A population parameter is a numerical characteristic of the entire population, such as the population mean (mu). A sample statistic is an estimate of the population parameter derived from a sample, like the sample mean (X bar).
In the script, what is the example used to illustrate the concept of a sampling distribution?
-The script uses the example of a university class with 16 students where the professor wants to know the average age of the students. The professor can only access the ages of a random sample of three students at a time.
How is the true population mean calculated in the script's example?
-The true population mean (mu) is calculated by taking the average of the ages of all 16 students, which is given as 239.8125 in the script.
What is the purpose of drawing multiple samples in the script's example?
-Drawing multiple samples serves to illustrate that the sample mean (X bar) will vary from sample to sample, highlighting the concept of the sampling distribution of the sample mean.
How is the sample mean calculated from a sample of students' ages?
-The sample mean is calculated by summing the ages of the students in the sample and then dividing by the number of students in that sample.
What does the script suggest about the distribution of the sample mean in many situations?
-The script suggests that in many situations, the distribution of the sample mean is approximately normal, even though the example provided does not show this.
How many possible samples are there in the script's example if the sample size is 3 and the population size is 16?
-There are 560 possible samples when the sample size is 3 and the population size is 16, calculated using the combination formula 'n choose k' (16 choose 3).
What is the significance of the histogram of sample means in the script's repeated sampling argument?
-The histogram of sample means represents the distribution of the sample mean across many repeated samples, providing an approximation of the true sampling distribution of the sample mean.
How does the concept of a sampling distribution help in making statements about population parameters?
-The concept of a sampling distribution allows us to make probabilistic statements about population parameters, such as expressing confidence intervals for estimates of the population mean.
Outlines
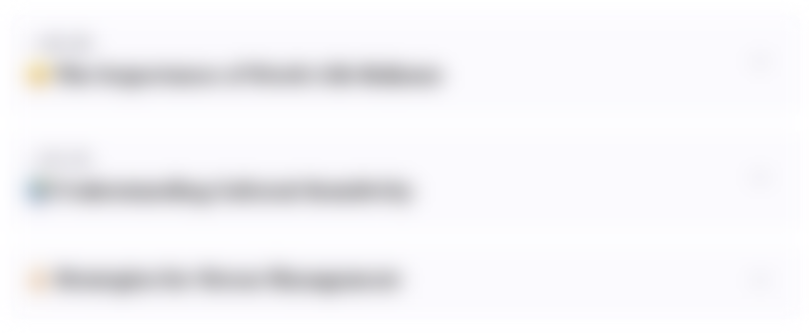
このセクションは有料ユーザー限定です。 アクセスするには、アップグレードをお願いします。
今すぐアップグレードMindmap
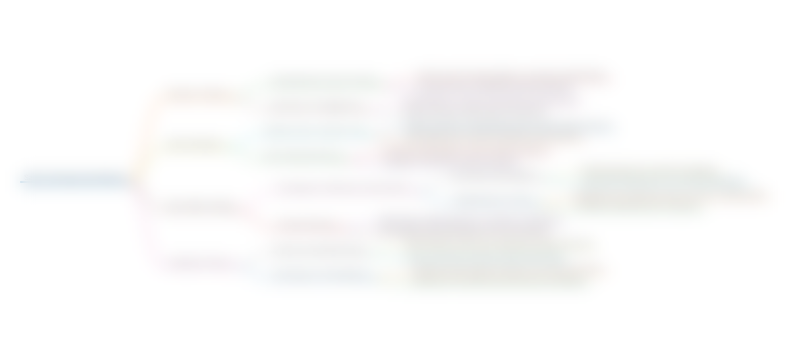
このセクションは有料ユーザー限定です。 アクセスするには、アップグレードをお願いします。
今すぐアップグレードKeywords
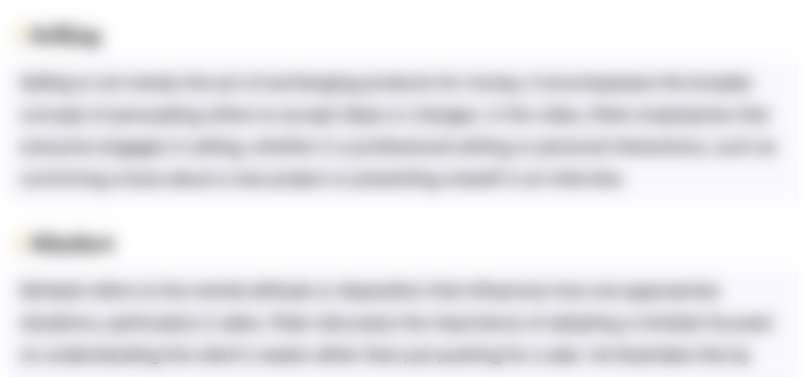
このセクションは有料ユーザー限定です。 アクセスするには、アップグレードをお願いします。
今すぐアップグレードHighlights
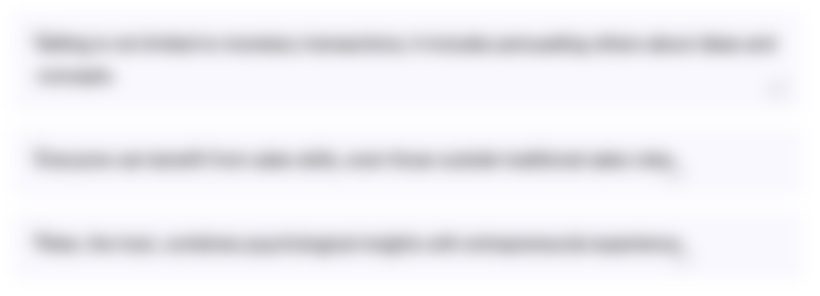
このセクションは有料ユーザー限定です。 アクセスするには、アップグレードをお願いします。
今すぐアップグレードTranscripts
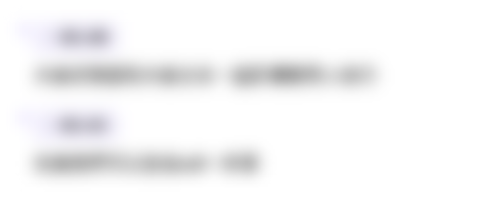
このセクションは有料ユーザー限定です。 アクセスするには、アップグレードをお願いします。
今すぐアップグレード5.0 / 5 (0 votes)