Debunking Devin: "First AI Software Engineer" Upwork lie exposed!
Summary
TLDRThe video script discusses the hype around AI, specifically focusing on the claims made about an AI named Devin, which was introduced as the 'world's first AI software engineer.' The speaker, a software professional with 35 years of experience, critiques the exaggeration and misinformation surrounding AI capabilities, using Devin as a case study. He argues that while AI can perform impressive tasks, it is not capable of fully replacing human software engineers, especially in understanding and communicating with clients. The speaker emphasizes the importance of truthful representation of AI's abilities and the potential harm caused by overhyping its capabilities.
Takeaways
- 😶 The video script critiques the hype around AI, specifically calling out the exaggerated claims made about an AI named Devin.
- 🚫 The main claim that Devin can 'make money taking on messy Upwork tasks' is disputed as false and misleading.
- 🙅♂️ The speaker emphasizes that they are not anti-AI, but rather against the hype and misinformation surrounding AI capabilities.
- 🎥 The video description and company tweets are highlighted as sources of the misleading claims about Devin's capabilities.
- 🤖 The speaker acknowledges that generative AI tools like GitHub Copilot and ChatGPT are impressive and useful, but stresses the importance of honesty in their representation.
- 🛠️ The actual task that Devin was supposed to accomplish is discussed, noting that it was a cherry-picked, specific task rather than a general capability.
- 🔍 The speaker conducted their own research and attempted to replicate Devin's work, finding that the AI's output was not as groundbreaking as claimed.
- ⏳ The time it took for the speaker to replicate Devin's work was significantly less than what was shown in the video, questioning the efficiency of the AI's process.
- 💻 The speaker points out that Devin generated errors and then attempted to fix them, which does not align with the narrative of fixing existing code in the repository.
- 🔗 The speaker encourages viewers to check the original sources of information and to be skeptical of headlines and claims made about AI.
- 📢 The video ends with a call for transparency, honesty, and skepticism in the face of AI hype and the potential for misinformation.
Q & A
What is the main claim presented in the video description that the speaker argues is a lie?
-The main claim presented in the video description that the speaker argues is a lie is that Devin, an AI software engineer, can make money by taking on messy Upwork tasks. The speaker asserts that this does not happen in the video and that it is misleading.
What is the speaker's professional background and stance on AI?
-The speaker has been a software professional for 35 years and is not anti-AI. However, the speaker is anti-hype and believes that lying about the capabilities of AI tools like Devin does a disservice to everyone.
How does the speaker describe the impact of hype and misinformation around AI capabilities?
-The speaker suggests that the hype and misinformation around AI capabilities can lead non-technical people to overestimate the current capabilities of AI. This can result in less skepticism towards AI outputs, leading to potential problems such as increased bugs, exploits, and hacks in the software ecosystem.
What was the specific task that Devin was supposed to perform on Upwork?
-Devin was supposed to provide detailed instructions on how to make inferences with a specific model in a repository on AWS EC2. However, the speaker argues that Devin did not fulfill this task as it did not generate the required detailed instructions.
What does the speaker criticize about the way Devin was presented and the hype around it?
-The speaker criticizes the hype around Devin for being exaggerated and misleading. The speaker points out that the claims about Devin's capabilities were not truthful and that the company behind Devin should have been more honest about what it could actually do.
What is the speaker's opinion on the importance of communication in software engineering?
-The speaker believes that communication is a crucial part of software engineering, involving understanding the needs of the customer, stakeholders, and team members. The speaker argues that AI is currently not capable of handling these communication aspects, which are some of the most important tasks in software engineering.
What did the speaker find when replicating Devin's work?
-The speaker found that Devin did not fix any actual errors from the repository provided by the Upwork client. Instead, Devin generated its own code with errors and then attempted to debug and fix those self-generated issues.
How long did it take the speaker to replicate Devin's results?
-It took the speaker approximately 36 minutes and 55 seconds to replicate what Devin did, which was significantly less time than the six hours and 20 minutes that Devin supposedly took.
What is the speaker's advice for AI product creators and those who report on AI?
-The speaker advises AI product creators to be truthful about their products' capabilities and not to exaggerate. For journalists, bloggers, and influencers, the speaker urges them to verify the claims they read on the Internet and not to blindly amplify unverified information.
What does the speaker suggest is the current state of generative AI in terms of coding?
-The speaker suggests that the current state of generative AI in coding often produces complicated, convoluted, and sometimes nonsensical code. It may work, but it is not efficient and can create more work for maintenance, bug fixing, or updates in the future.
What is the speaker's final message to the audience regarding skepticism and the internet?
-The speaker's final message is a call for skepticism towards everything seen on the Internet or news, especially when it comes to AI-related content. The speaker emphasizes the importance of not taking information at face value and verifying the truthfulness of claims before accepting them as facts.
Outlines
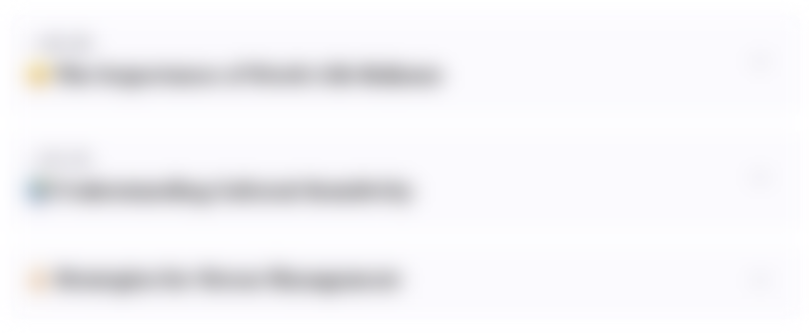
このセクションは有料ユーザー限定です。 アクセスするには、アップグレードをお願いします。
今すぐアップグレードMindmap
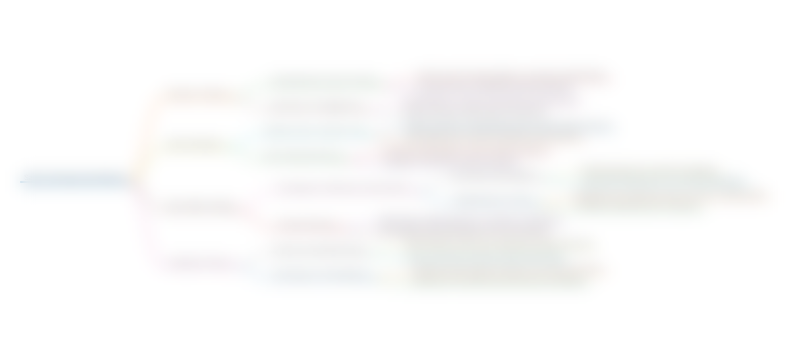
このセクションは有料ユーザー限定です。 アクセスするには、アップグレードをお願いします。
今すぐアップグレードKeywords
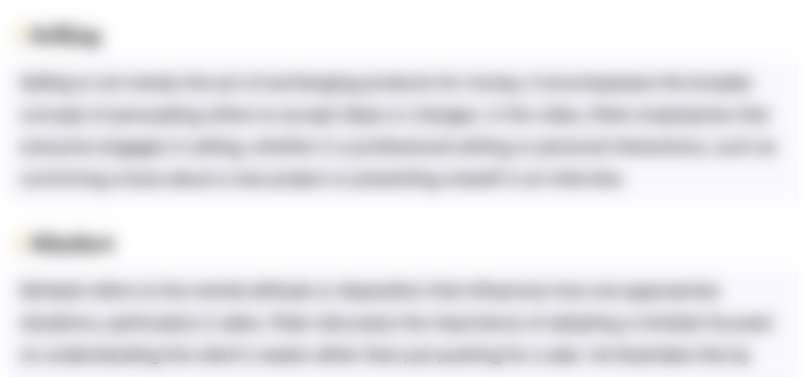
このセクションは有料ユーザー限定です。 アクセスするには、アップグレードをお願いします。
今すぐアップグレードHighlights
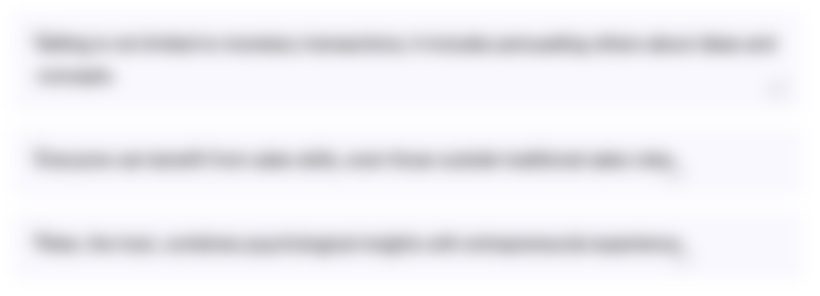
このセクションは有料ユーザー限定です。 アクセスするには、アップグレードをお願いします。
今すぐアップグレードTranscripts
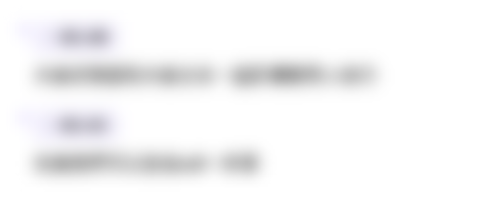
このセクションは有料ユーザー限定です。 アクセスするには、アップグレードをお願いします。
今すぐアップグレード関連動画をさらに表示
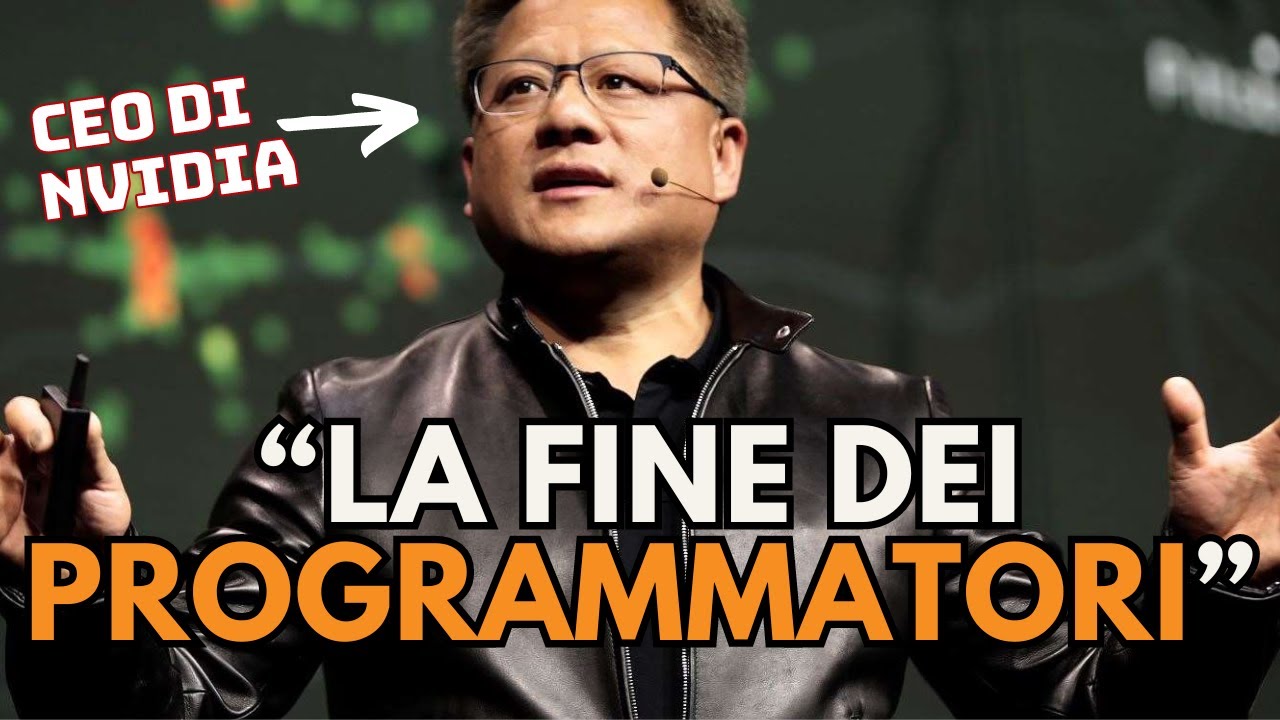
Devin: l'AI che sostituirà i programmatori [Reaction]
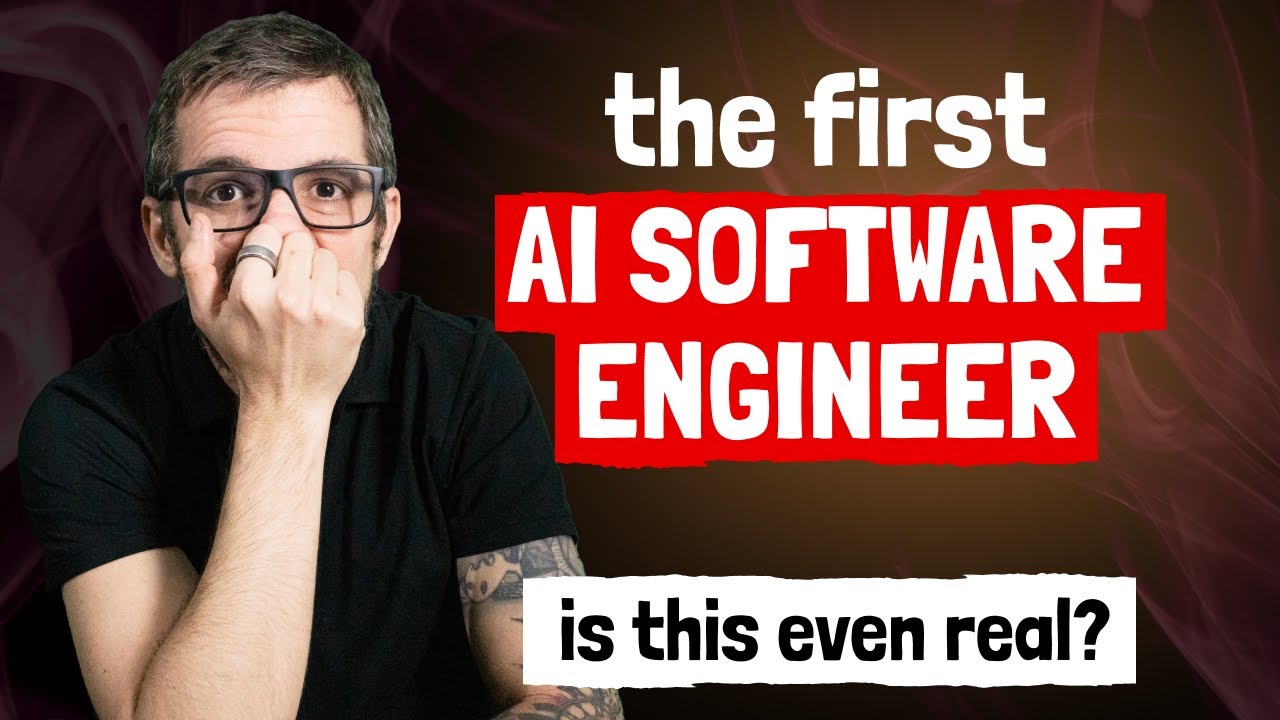
I used the first AI Software Engineer for a week. This is happening.

Devin Was a Lie! - The Big Expose (AI Scam) 😡
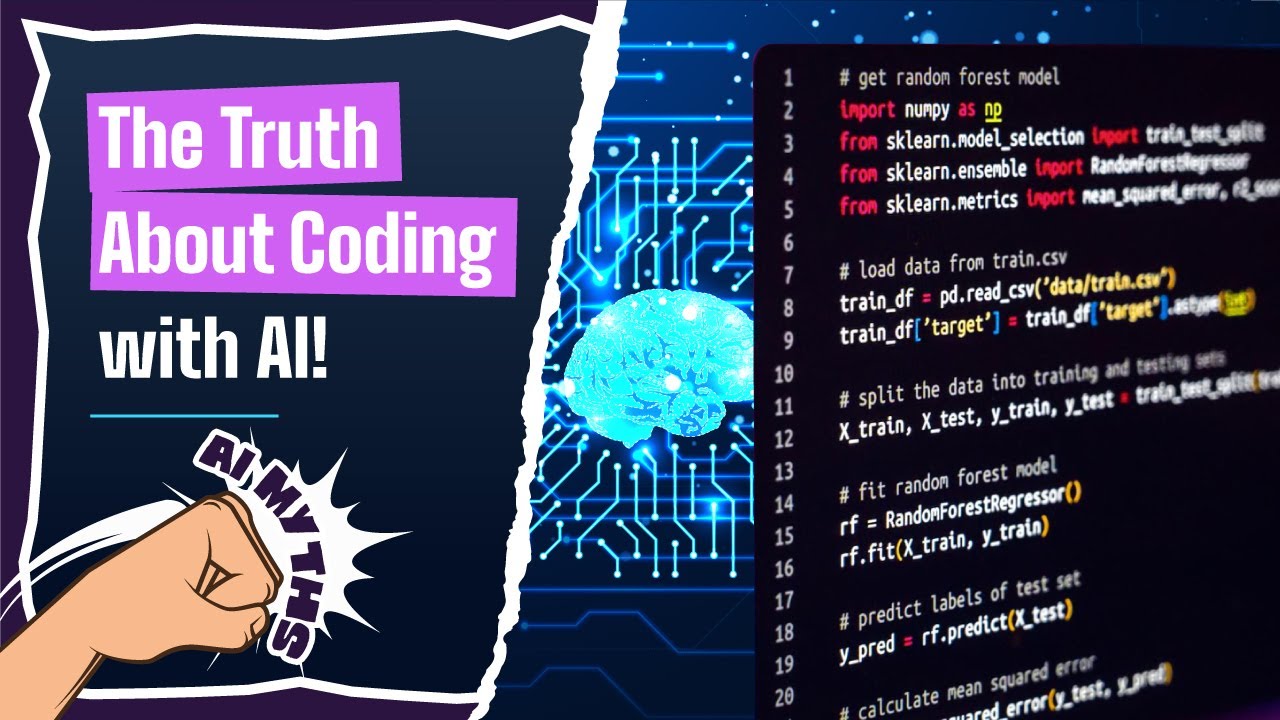
Software Engineers and IT Leaders are Dead Wrong about AI
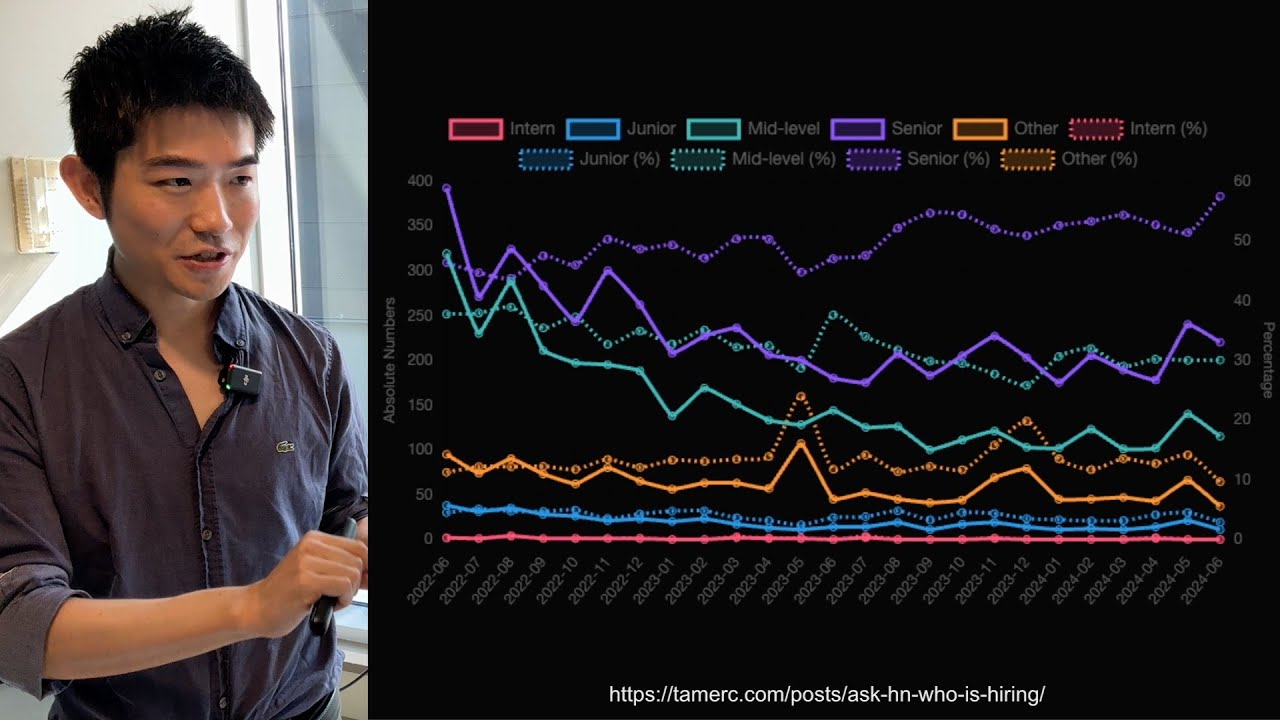
Is AI Replacing Software Engineering?
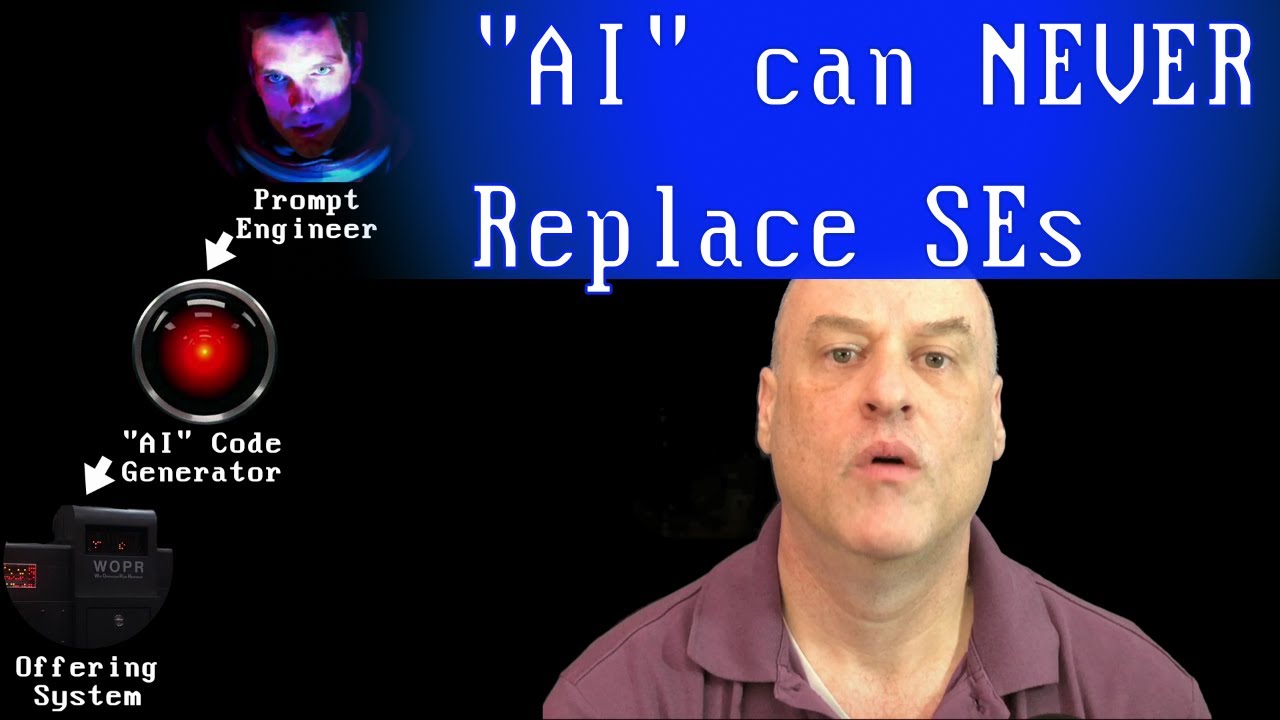
Software Engineer jobs (Mid-Senior) can NEVER be replaced by a Coding "AI" like Devin
5.0 / 5 (0 votes)