AI and Data Science in Earth Observation - Methodological Research
Summary
TLDRThis presentation delves into the challenges and advancements in AI for Earth Observation (EO) and climate modeling. Key topics include integrating physics-aware machine learning, enhancing AI with reasoning, ensuring model transferability across regions, and tackling uncertainties. The speaker discusses the importance of self-supervised learning, green AI, and foundation models, which reduce label dependence and support multiple downstream applications. Ethical considerations in AI, particularly related to privacy, bias, and fairness, are also highlighted. The session provides insights into cutting-edge AI techniques while addressing environmental and ethical concerns for a more sustainable future in AI-driven Earth Observation.
Takeaways
- 😀 AI for Earth Observation (EO) faces fundamental challenges like integrating physics-based domain knowledge with data-driven models.
- 😀 Reasoning in AI models remains a challenge; for example, understanding spatial-temporal relationships like cars driving on paved roads.
- 😀 Transferability of models is crucial, especially when applying trained models from one region to another region lacking data.
- 😀 Uncertainty quantification is important for AI models, helping to measure data and model uncertainties and ensure reliable output.
- 😀 Explainable AI is essential for remote sensing, especially when investigating extreme events like fires or floods, to understand their underlying causes.
- 😀 Green AI focuses on energy-efficient and label-efficient training methods, reducing environmental impact and resource usage.
- 😀 Self-supervised learning can significantly reduce the need for labeled data in Earth observation tasks, enabling more efficient models.
- 😀 Foundation models are a trending topic in AI, focusing on learning generic features from large data archives to support various downstream tasks.
- 😀 Future AI models may leverage quantum computing, opening new possibilities for complex applications in the coming decades.
- 😀 Ethical considerations are key in AI for EO, ensuring data fairness, privacy, and avoiding biases in AI systems, especially with open-source data.
- 😀 Human-in-the-loop methods, such as reasoning with natural language queries, are becoming more integrated in AI systems for Earth observation tasks.
Q & A
What is the key challenge discussed regarding the integration of physics and machine learning in Earth Observation?
-The key challenge is combining physics-based domain knowledge with data-driven AI, particularly in bringing together model-based knowledge with machine learning approaches to improve forecasting and model accuracy.
What is the role of reasoning models in AI for Earth Observation?
-Reasoning models help understand spatial and temporal relationships between different semantics in data. For example, recognizing that cars typically drive on paved roads is a trivial task for humans but challenging for AI systems to model.
Why is transferability an important issue in Earth Observation AI?
-Transferability is crucial because training data is often region-specific, and the goal is to apply models to regions where no training data exists, bridging the gap between regions with different data and sensors.
What are the key sources of uncertainty in Earth Observation AI models?
-Key sources of uncertainty include data uncertainty (e.g., due to clouds in satellite images), model uncertainty (errors from training and inference processes), and label uncertainty (ambiguity in classifying certain features, such as building types).
How does uncertainty quantification help in AI for Earth Observation?
-Uncertainty quantification allows AI models to assess the confidence of their predictions, helping users understand how reliable the model's outputs are, especially in cases of ambiguous or missing data.
What is the concept of green AI, and why is it important?
-Green AI focuses on making AI models more energy-efficient and label-efficient. This is important because AI model training often requires vast amounts of labeled data and computational power, and reducing these requirements can minimize environmental impact.
What are self-supervised learning and its benefits in Earth Observation?
-Self-supervised learning involves training models on large amounts of unlabeled data by defining pretext tasks. In Earth Observation, it can help reduce the need for labeled data, making it easier to apply AI to vast datasets like satellite imagery.
What is the difference between foundation models and traditional AI models?
-Foundation models are large-scale, pre-trained models that learn general features from massive datasets and can be adapted to a wide variety of downstream tasks. Traditional AI models are often trained specifically for a single task.
How could quantum machine learning impact Earth Observation in the future?
-Quantum machine learning may provide more computational power, enabling more efficient processing of complex Earth Observation data, which could lead to better models for tasks like climate forecasting and disaster prediction.
What ethical considerations are important when using AI in Earth Observation?
-Ethical considerations include avoiding bias in training data, ensuring data privacy, preventing social disadvantages, and addressing potential dual-use concerns. These are critical to ensure that AI applications benefit society equitably and responsibly.
Outlines
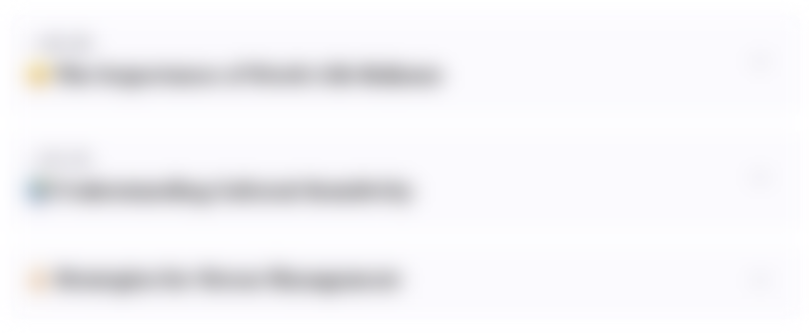
Cette section est réservée aux utilisateurs payants. Améliorez votre compte pour accéder à cette section.
Améliorer maintenantMindmap
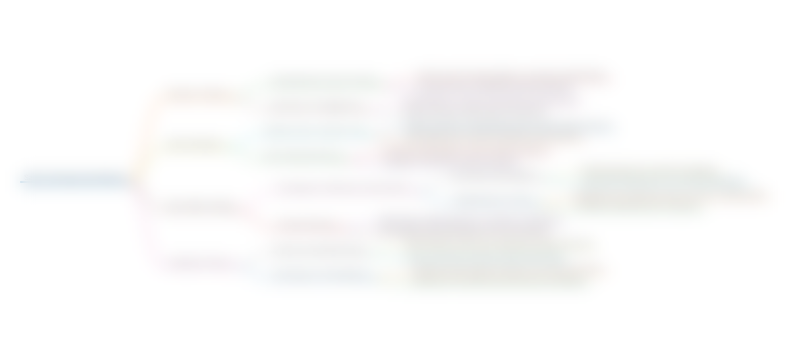
Cette section est réservée aux utilisateurs payants. Améliorez votre compte pour accéder à cette section.
Améliorer maintenantKeywords
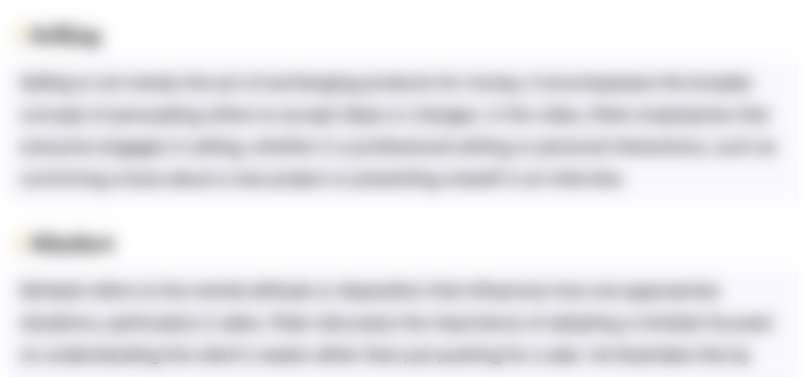
Cette section est réservée aux utilisateurs payants. Améliorez votre compte pour accéder à cette section.
Améliorer maintenantHighlights
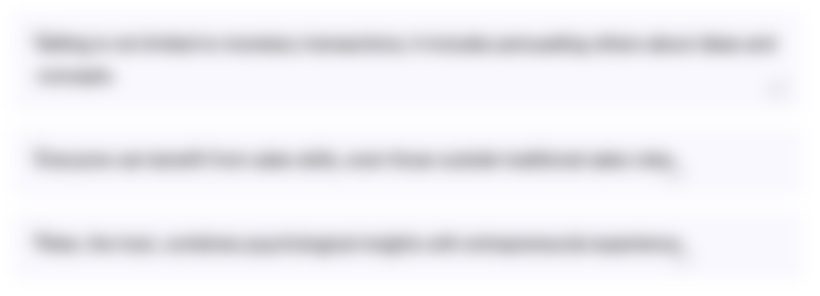
Cette section est réservée aux utilisateurs payants. Améliorez votre compte pour accéder à cette section.
Améliorer maintenantTranscripts
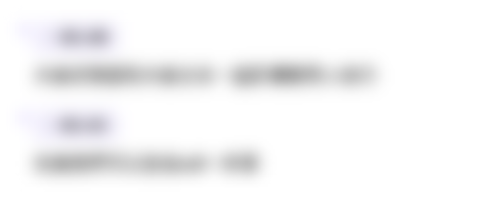
Cette section est réservée aux utilisateurs payants. Améliorez votre compte pour accéder à cette section.
Améliorer maintenantVoir Plus de Vidéos Connexes
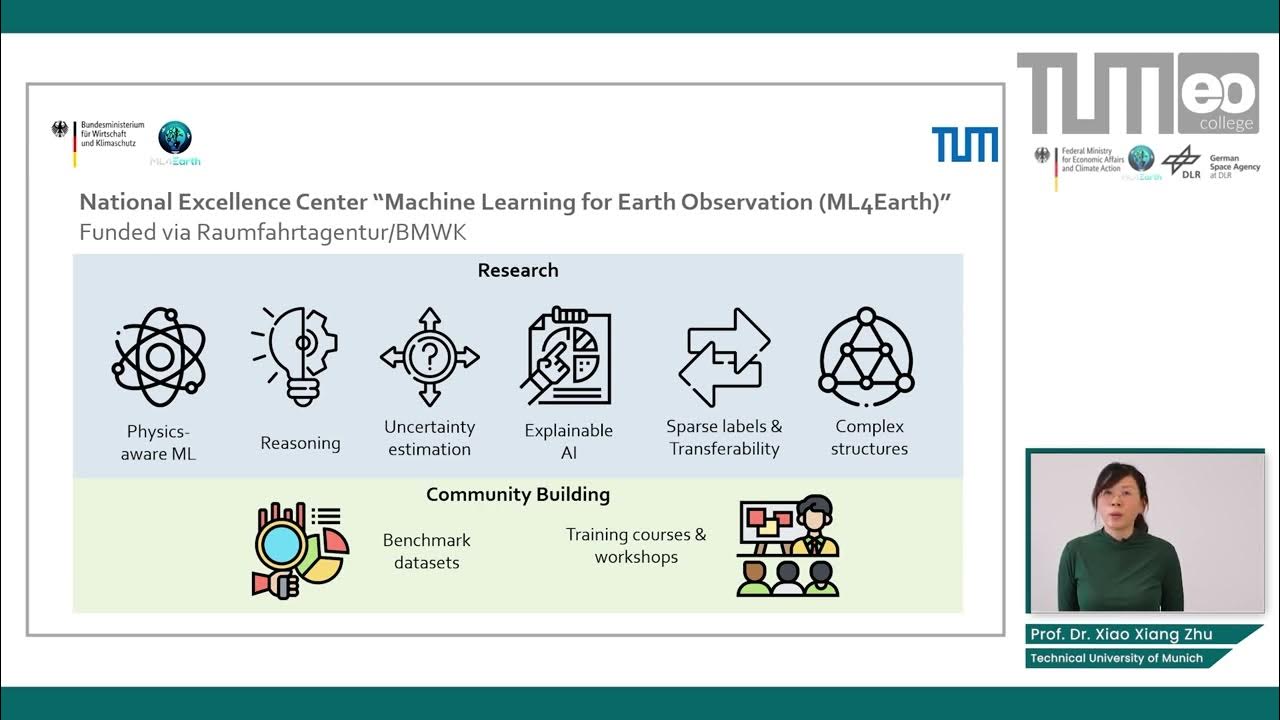
AI and Data Science in Earth Observation - Large Scale Representative Annotated Datasets
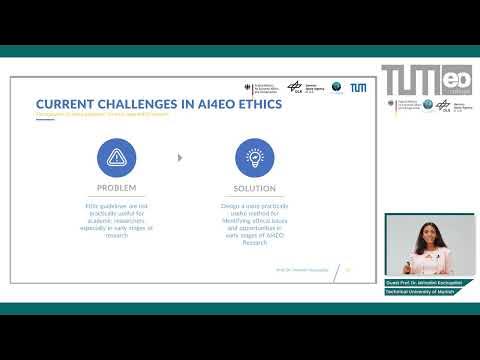
Ethics in AI4EO - Applying Ethics to AI4EO
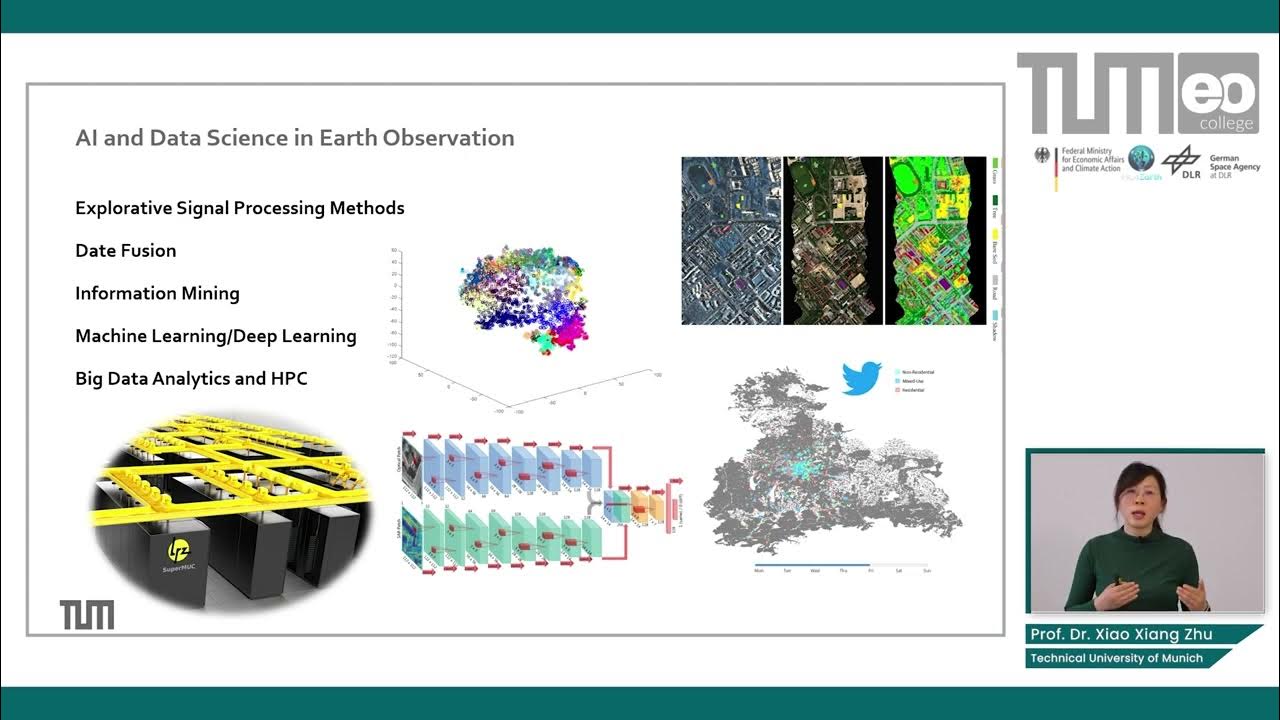
AI and Data Science in Earth Observation - Introduction

Ethics in AI4EO - Basic Concepts

Nvidia's 2024 Computex Keynote: Everything Revealed in 15 Minutes
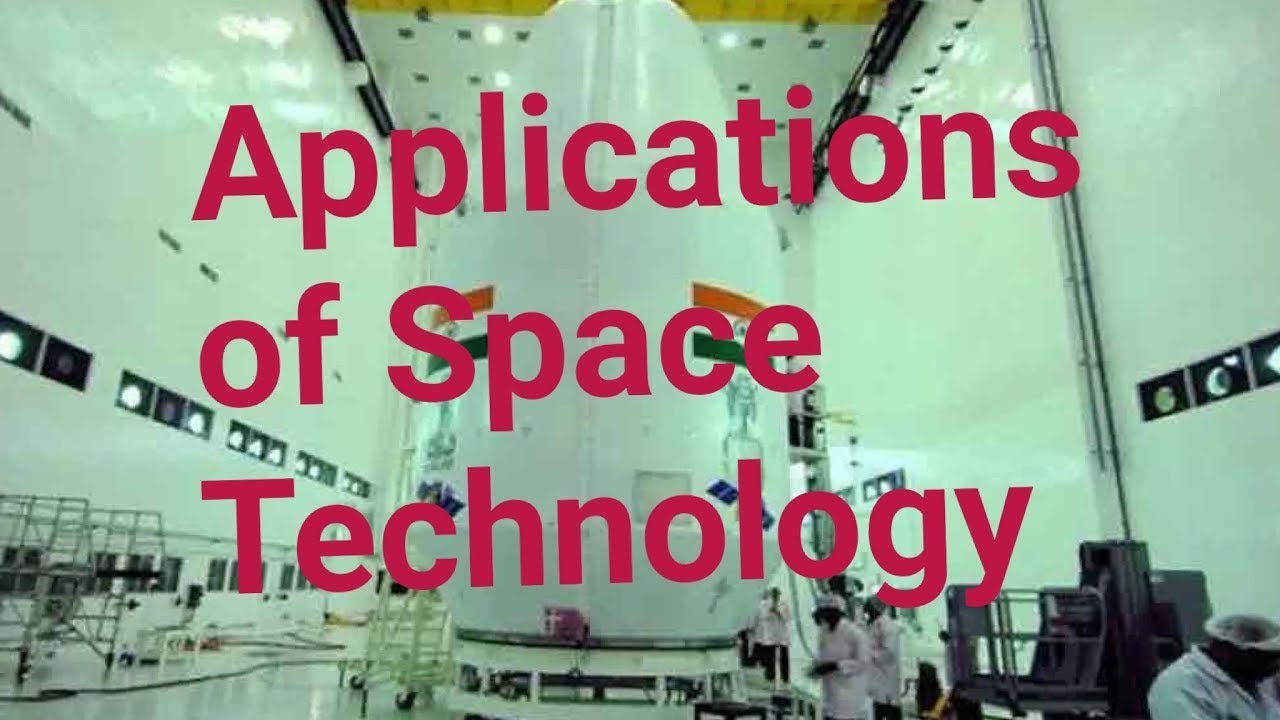
Applications of Space Technology (Space - S&T) | La Excellence || Best IAS Institute in Hyderabad
5.0 / 5 (0 votes)