AI and Data Science in Earth Observation - Large Scale Representative Annotated Datasets
Summary
TLDRThis video delves into the critical role of large-scale, annotated datasets in Earth Observation (EO) for AI applications. The speaker highlights the gap between annotated and unlabeled data, with only a small fraction of EO data currently annotated. They introduce ML for Earth, a project aimed at fostering AI development for Earth preservation, which offers valuable datasets, deep learning methods, and communication platforms to enhance collaboration. Despite progress, challenges remain, such as data uncertainty, explainability, and physics consistency. The video underscores the importance of community efforts to tackle these challenges and advance AI for EO solutions.
Takeaways
- ๐ The availability of large-scale, representative annotated datasets is crucial for the development of AI algorithms in Earth Observation (EO) and other fields.
- ๐ EO has a unique challenge compared to computer vision due to the lack of annotated data, making data collection and annotation an important focus.
- ๐ Deep learning algorithms in EO rely heavily on tailored architectures, loss functions, and data, with innovation increasingly driven by the availability and quality of datasets.
- ๐ Several benchmark datasets have been developed for specific EO tasks such as event recognition, image classification, change detection, and food security.
- ๐ The platform 'snuts' provides access to over 500 annotated datasets and over 50 state-of-the-art deep learning methods for EO tasks.
- ๐ 'Snuts' includes over 10 specialized libraries designed to support various EO applications, helping users easily access relevant datasets.
- ๐ Annotated datasets in EO are growing, with the number and size of datasets increasing over time, yet the amount of labeled data remains a small fraction of the total available data.
- ๐ Despite massive growth in EO and climate data (reaching 100 petabytes), less than 0.1% of it is annotated, highlighting a significant gap in labeled data.
- ๐ The 'ml for Earth' project, funded by the Federal Ministry for Economics and Climate Action, aims to build a community around AI for Earth Observation, both nationally and internationally.
- ๐ The 'ml for Earth' project includes research on machine learning challenges, along with efforts to provide benchmark datasets, courses, and workshops to foster collaboration within the EO community.
- ๐ The AI for EO field offers invaluable geo-information that can address global challenges, but key challenges such as uncertainty, physics consistency, explainability, and transferability still need to be tackled with community efforts.
Q & A
What is the main challenge in Earth Observation (EO) as discussed in the video?
-The main challenge in Earth Observation (EO) is the lack of large-scale, representative annotated data sets, which are crucial for training deep learning models. Despite the availability of vast amounts of EO data, only a small percentage of it is annotated, limiting its usability for AI-based applications.
How does the situation in Earth Observation differ from general computer vision?
-In Earth Observation, there is less annotated data compared to general computer vision. While computer vision often has large, labeled datasets, EO faces the challenge of a significant lack of annotations, which is critical for training deep learning models.
What is the importance of training data in AI development, particularly in EO?
-Training data is crucial in AI development because it directly influences the performance of deep learning algorithms. Even though there are many established AI architectures and methods, the dataโespecially labeled dataโremains the key factor in driving innovation and achieving successful AI applications in EO.
What kind of benchmark datasets have been produced for AI in EO?
-Various benchmark datasets have been developed for AI in EO, covering tasks like event recognition from videos, image classification, change detection, and food security. These datasets are meant to help researchers and practitioners get started with AI for Earth Observation.
What platform has been developed to facilitate access to AI for EO?
-A platform called Snuts has been developed to help people access AI for Earth Observation. It houses over 500 annotated datasets, more than 50 state-of-the-art deep learning methods, and over 10 specialized libraries to support different EO tasks.
What does the platform Snuts offer to its users?
-Snuts offers access to a wide range of annotated datasets, deep learning methods, and specialized libraries for Earth Observation tasks. It also provides attributes that help users search for suitable datasets, making it easier for them to start working on specific EO applications.
How has the number of annotated datasets in EO changed over time?
-The number of annotated datasets in EO has increased significantly over the years, from 2008 to the present. However, despite this growth, the percentage of labeled data remains small compared to the vast amounts of unlabeled data available in the field.
What gap still exists in EO data despite the increase in annotated datasets?
-Despite the increase in annotated datasets, there is still a large gap between the amount of labeled and unlabeled data. Less than 0.1% of the available EO data is annotated, while a vast amount of data remains without labels.
What is the purpose of the 'ML for Earth' project?
-The 'ML for Earth' project aims to build a community focused on AI for Earth Observation, both in Germany and internationally. It is funded by the Federal Ministry for Economics and Climate Action and managed by the German Space Agency. The project focuses on fundamental challenges in machine learning applied to Earth Observation and offers resources like benchmark datasets, courses, and workshops.
What is the goal of the 'Machine Learning for Earth Preservation' initiative?
-The goal of the 'Machine Learning for Earth Preservation' initiative is to develop machine learning solutions for Earth Observation applications, aiming to address global challenges. This includes offering tools, datasets, and fostering community engagement through courses, workshops, and communication channels.
Outlines
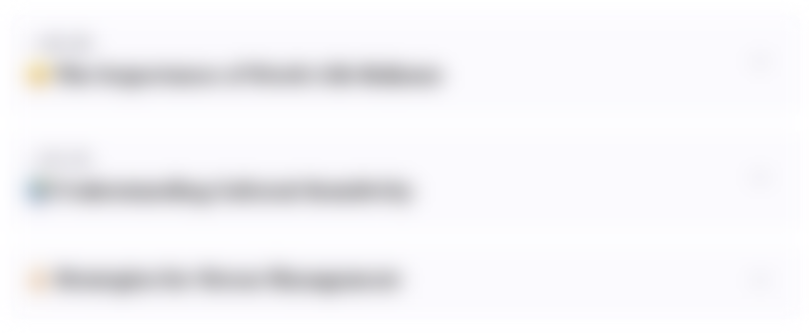
This section is available to paid users only. Please upgrade to access this part.
Upgrade NowMindmap
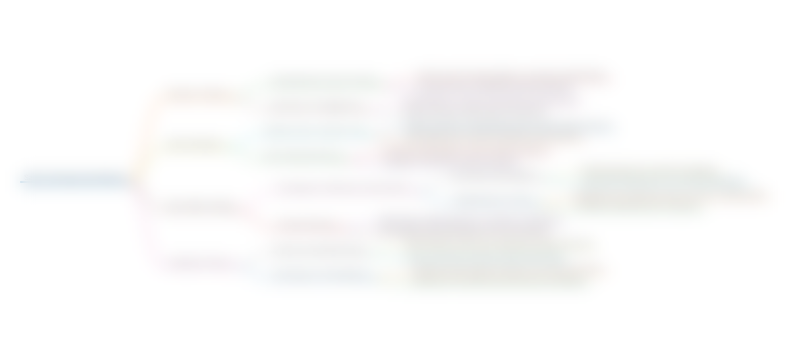
This section is available to paid users only. Please upgrade to access this part.
Upgrade NowKeywords
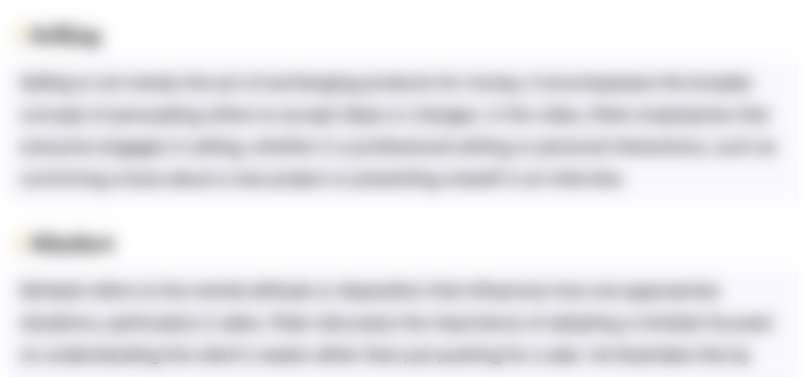
This section is available to paid users only. Please upgrade to access this part.
Upgrade NowHighlights
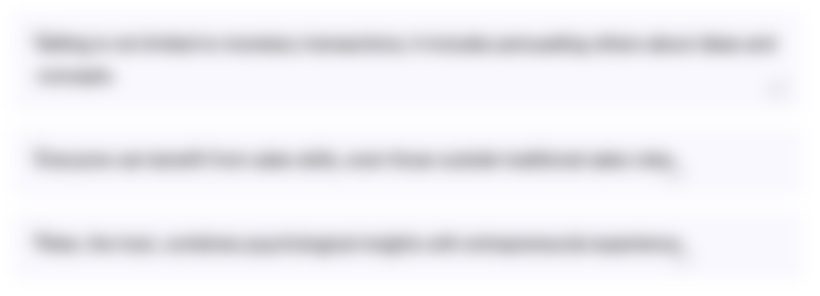
This section is available to paid users only. Please upgrade to access this part.
Upgrade NowTranscripts
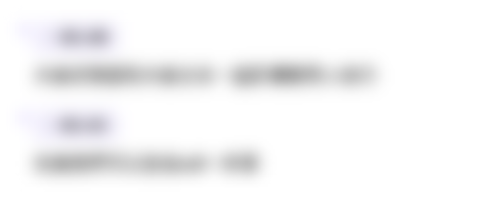
This section is available to paid users only. Please upgrade to access this part.
Upgrade NowBrowse More Related Video
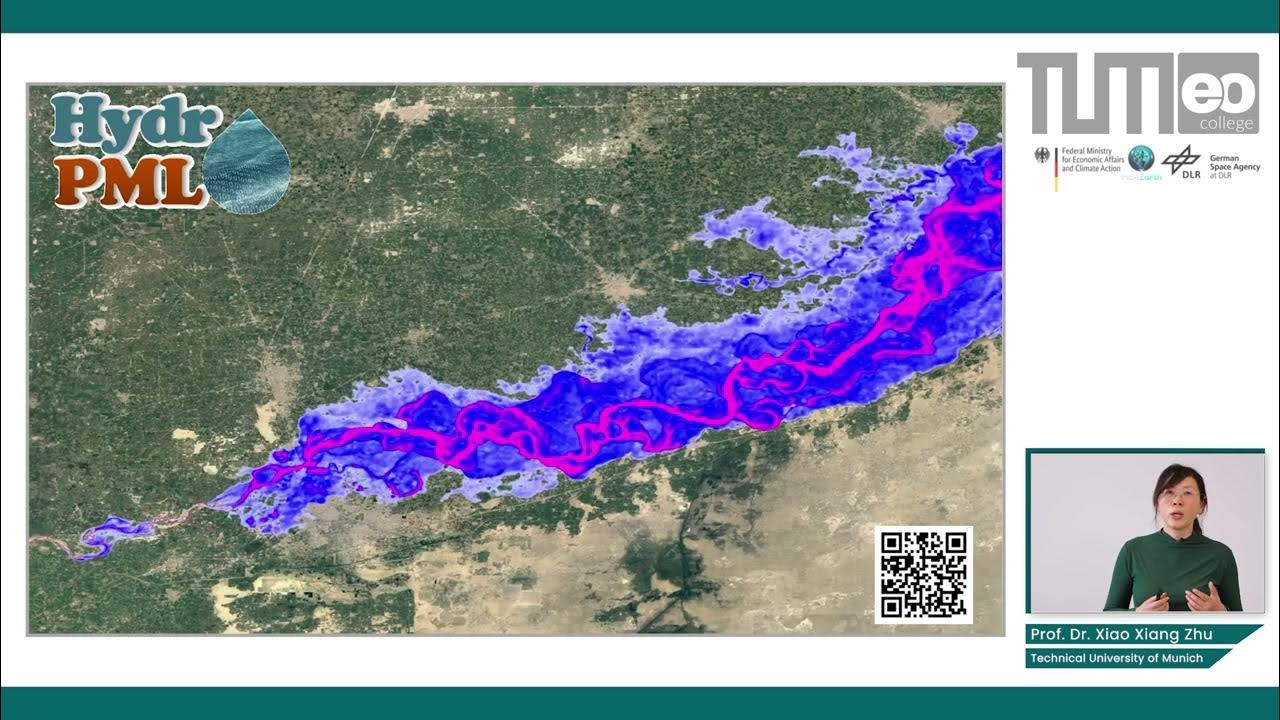
AI and Data Science in Earth Observation - Methodological Research
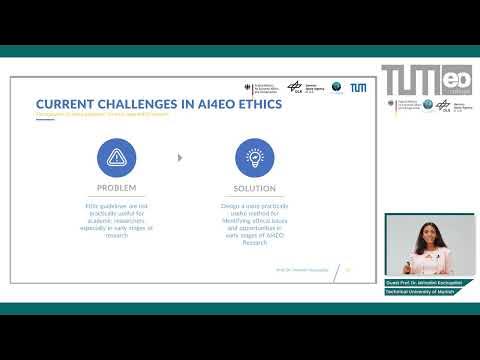
Ethics in AI4EO - Applying Ethics to AI4EO
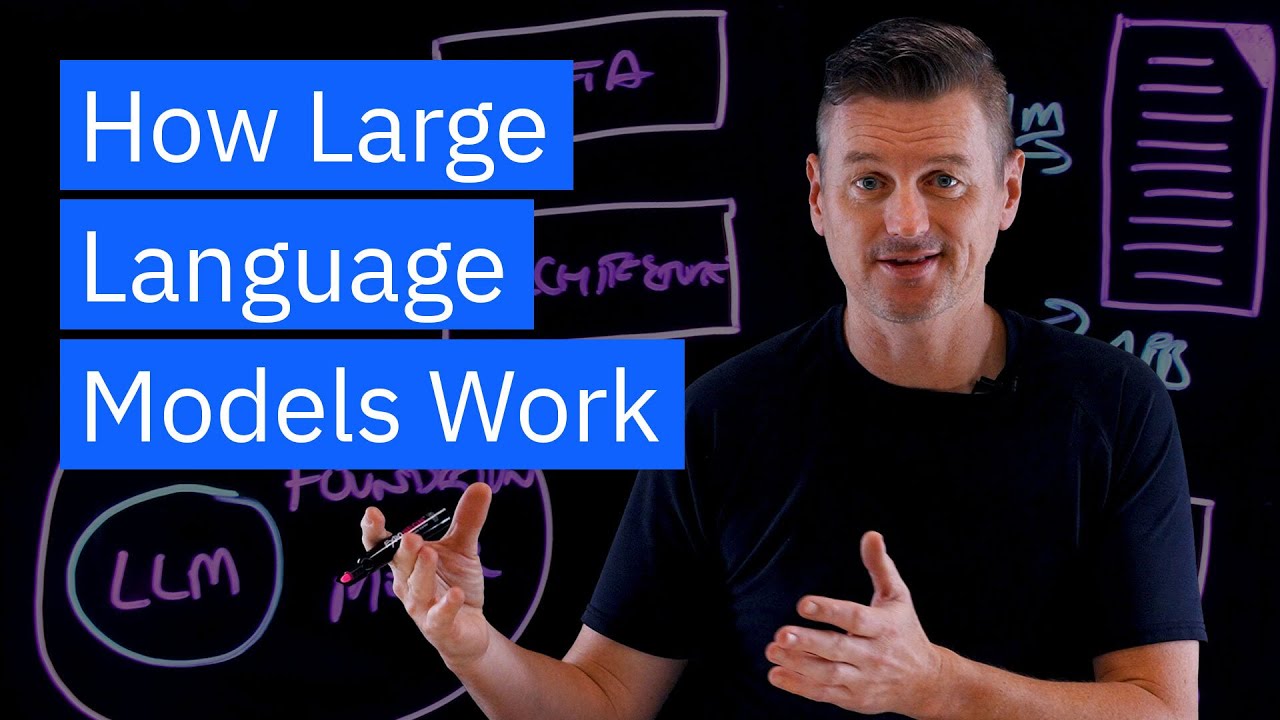
How Large Language Models Work

Processing Topologies|Introduction to IoT|On-Site|Off-Site|Remote& Collaborative processing|Critical

Ethics in AI4EO - Basic Concepts
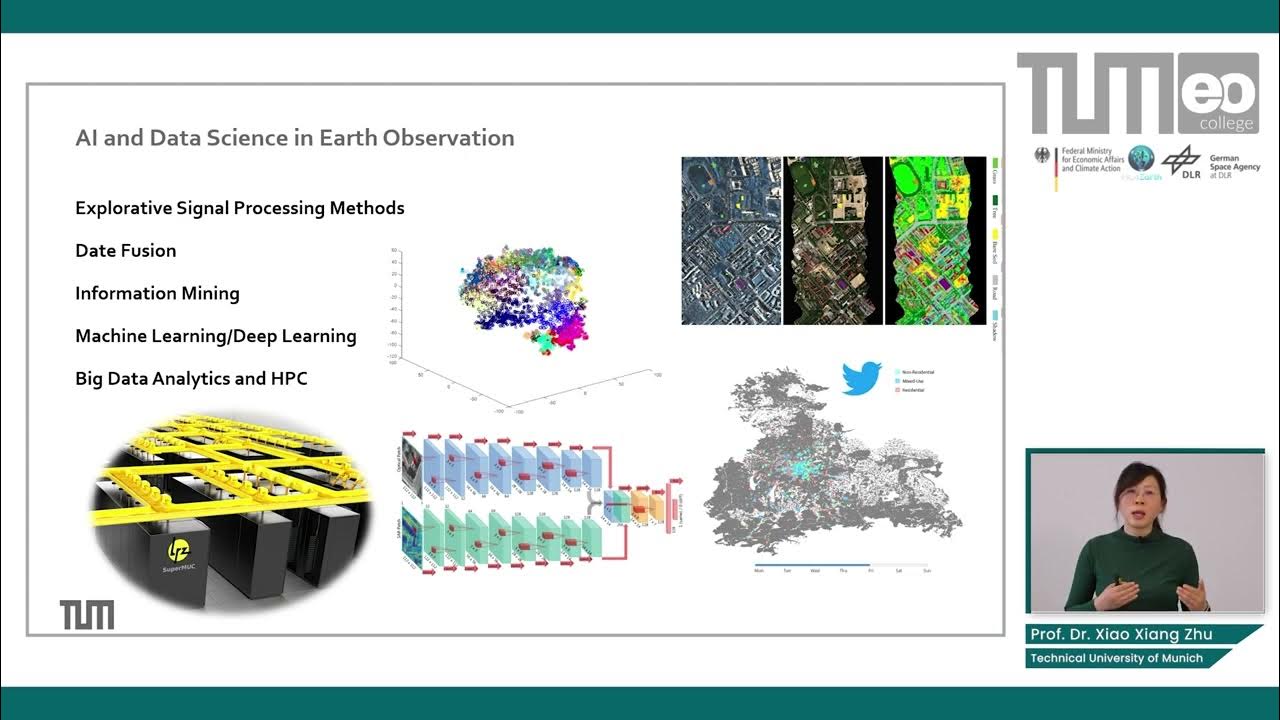
AI and Data Science in Earth Observation - Introduction
5.0 / 5 (0 votes)