AI and Data Science in Earth Observation - Introduction
Summary
TLDRIn this introductory course on Earth observation, Professor Shaanu outlines the role of artificial intelligence and data science in transforming satellite-based Earth data into valuable insights. The course highlights the rapid advancement of Earth observation technologies, from Sentinel missions to commercial satellite innovations. With vast data sources, the integration of AI is essential for effective analysis and information retrieval. Emphasizing the importance of high-quality, geodetic measurements, the course explores the challenges and opportunities in applying machine learning, deep learning, and data fusion to address pressing global issues like climate change and sustainable development goals.
Takeaways
- 😀 Earth observation relies heavily on satellites in low Earth orbit, enabling the measurement of Earth's surface from various locations and angles.
- 😀 AI and data science are transforming Earth observation by handling vast amounts of satellite data and providing solutions for global challenges like climate change and urbanization.
- 😀 The Golden Era of Earth observation is driven by advanced satellite infrastructure, with programs like ESA's Sentinel missions offering continuous, high-quality data.
- 😀 Commercial satellite companies, such as Planet Labs, are complementing space agency missions by offering high-frequency, albeit lower-resolution, Earth coverage.
- 😀 AI methods in Earth observation need to be tailored specifically for this domain rather than borrowing from fields like computer vision, as EO data has unique characteristics.
- 😀 The rapid growth of Earth observation data—more than 150 petabytes daily—demands new AI techniques for efficient information retrieval and mining from large datasets.
- 😀 Deep learning has become a powerful tool in remote sensing, with a focus on designing custom models for EO problems, such as high-accuracy physical and biochemical variable retrieval.
- 😀 Unlike internet images, EO data requires careful consideration of its high quality, including radiometric and geometric calibration, as well as its inherent geodetic measurements.
- 😀 The diversity of EO data—spanning optical, hyperspectral, microwave, and time-series data—makes it more complex than typical computer vision data, requiring innovative AI solutions.
- 😀 AI in Earth observation should focus on high-societal-relevance problems, such as monitoring sustainable development goals (SDGs), rather than more general tasks like image classification.
- 😀 The course emphasizes AI’s role in addressing real-world global problems by leveraging satellite data to develop actionable insights for pressing environmental and societal issues.
Q & A
What is the main focus of the course 'ML for Earth'?
-The main focus of the course is to introduce artificial intelligence and data science in Earth observation, with a particular emphasis on the use of satellite data for various applications in global change, environmental sciences, and sustainability.
How do satellites in low Earth orbit assist in Earth observation?
-Satellites in low Earth orbit provide global coverage by continuously orbiting the Earth, collecting measurements of the Earth's surface. This allows for both broad monitoring and high-resolution data for specific locations when sensors are directed at them.
What types of applications benefit from Earth observation data?
-Earth observation data is used in a wide range of applications including global change research, environmental sciences, meteorology, sustainability monitoring, resource management, and urban planning.
Why is Earth observation considered to be in a 'Golden Era'?
-Earth observation is considered to be in a 'Golden Era' because of the availability of high-quality, continuous, and open satellite data, particularly from the Sentinel missions and other space programs. This infrastructure provides more reliable data than ever before, with long-term accessibility.
How does the private sector contribute to Earth observation?
-The private sector, with companies like Planet Labs, contributes to Earth observation by launching small satellites in large numbers. These satellites provide high-frequency, albeit lower resolution, data with daily global coverage, complementing the data from space agencies.
What are the major challenges posed by big data in Earth observation?
-The major challenges include the sheer volume of data (e.g., 150 petabytes daily), which makes it impractical to process all of it. Therefore, efficient methods to mine relevant data, as well as the application of AI and machine learning, are necessary to extract useful information.
Why is artificial intelligence crucial for Earth observation?
-AI is crucial in Earth observation due to the need for specialized methods to handle big data and high-resolution data. Traditional analytic methods are no longer sufficient, and AI helps in efficiently extracting, analyzing, and processing large datasets.
What is the role of deep learning in Earth observation?
-Deep learning plays a significant role in Earth observation by enabling the extraction of complex patterns and features from large datasets. It allows for the development of tailored models that are more accurate and efficient in handling the diverse types of data from Earth observation satellites.
What are the key phases in the development of deep learning in remote sensing?
-The development of deep learning in remote sensing has gone through three phases: the initial phase where models were borrowed from AI and tested with limited success, a second phase where domain-specific architectures were developed, and the current phase where domain knowledge and physical principles are being integrated into deep learning models.
How does Earth observation data differ from standard computer vision data?
-Earth observation data differs from standard computer vision data in several ways: it includes geodetic measurements, it spans across multiple dimensions (location, time, and sensor modality), and the data is highly controlled and calibrated. Additionally, Earth observation often lacks large labeled datasets, unlike typical computer vision tasks.
What are the five dimensions of Earth observation data?
-The five dimensions of Earth observation data are: 1) location (x, y, z), 2) time (T), 3) sensor modality (Lambda), which includes different types of sensors like optical, hyperspectral, and microwave, 4) geospatial data from unconventional sources like social media, and 5) temporal data that provides time series for continuous monitoring.
Outlines
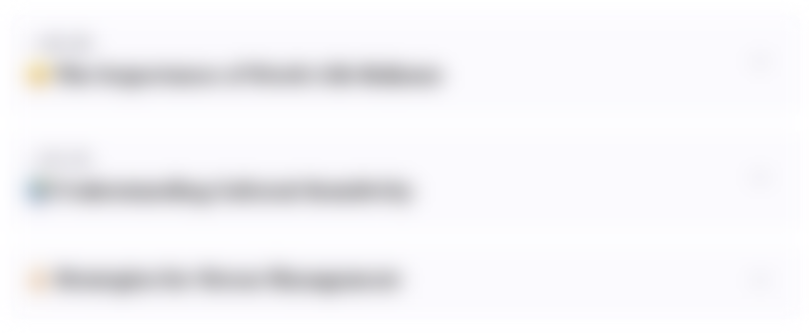
This section is available to paid users only. Please upgrade to access this part.
Upgrade NowMindmap
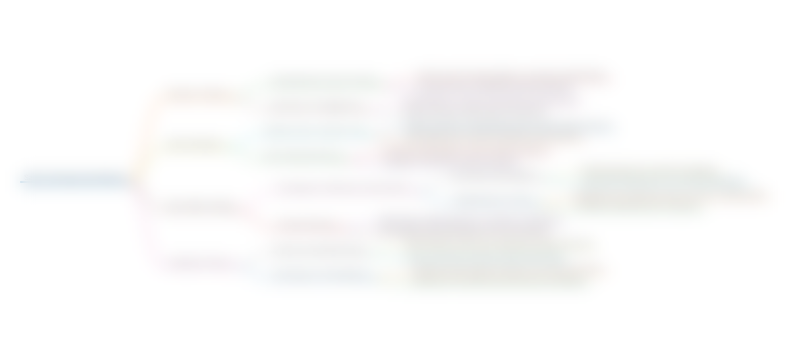
This section is available to paid users only. Please upgrade to access this part.
Upgrade NowKeywords
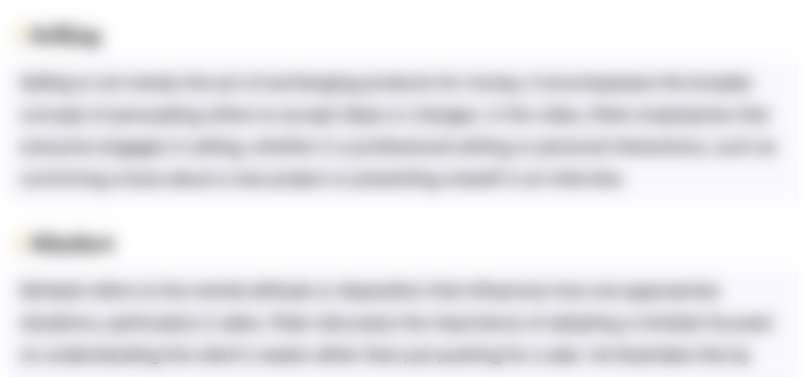
This section is available to paid users only. Please upgrade to access this part.
Upgrade NowHighlights
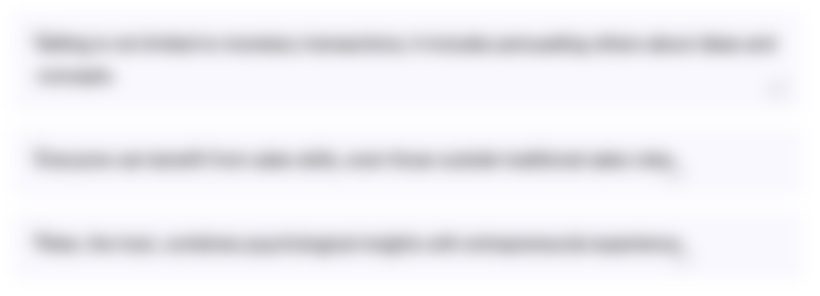
This section is available to paid users only. Please upgrade to access this part.
Upgrade NowTranscripts
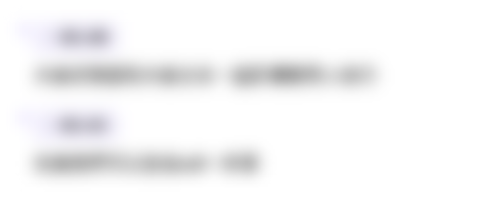
This section is available to paid users only. Please upgrade to access this part.
Upgrade NowBrowse More Related Video
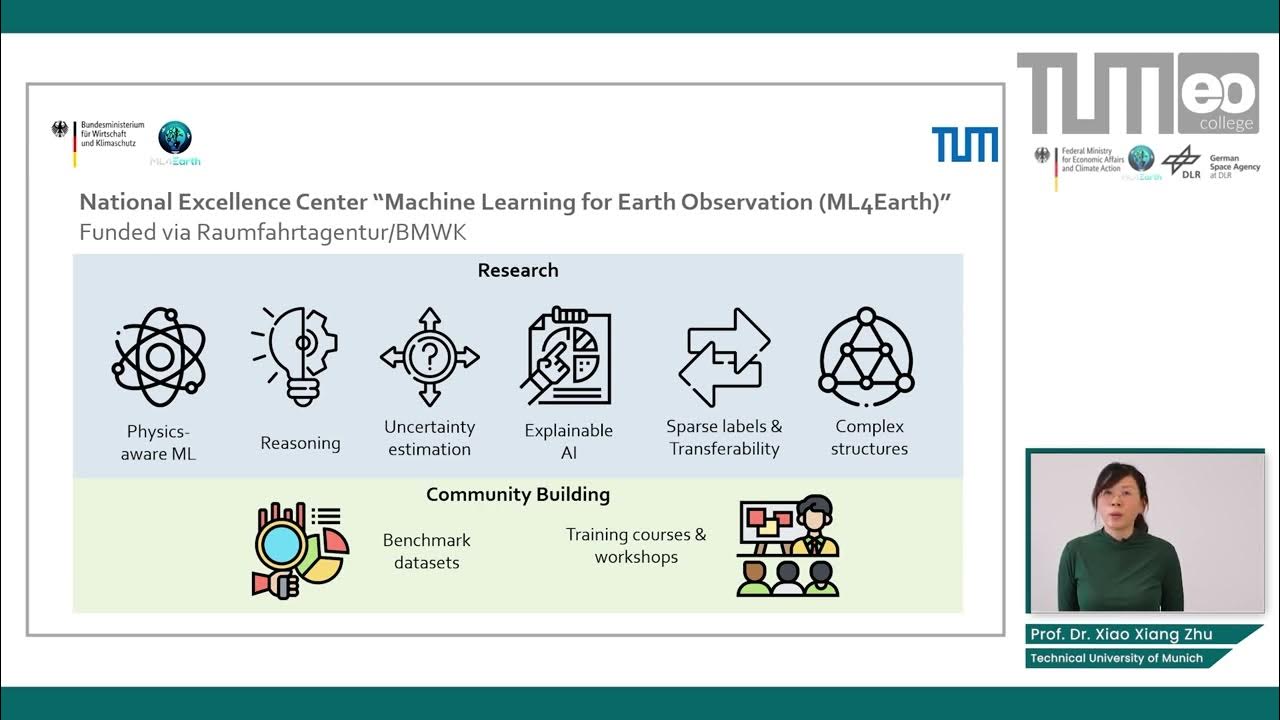
AI and Data Science in Earth Observation - Large Scale Representative Annotated Datasets
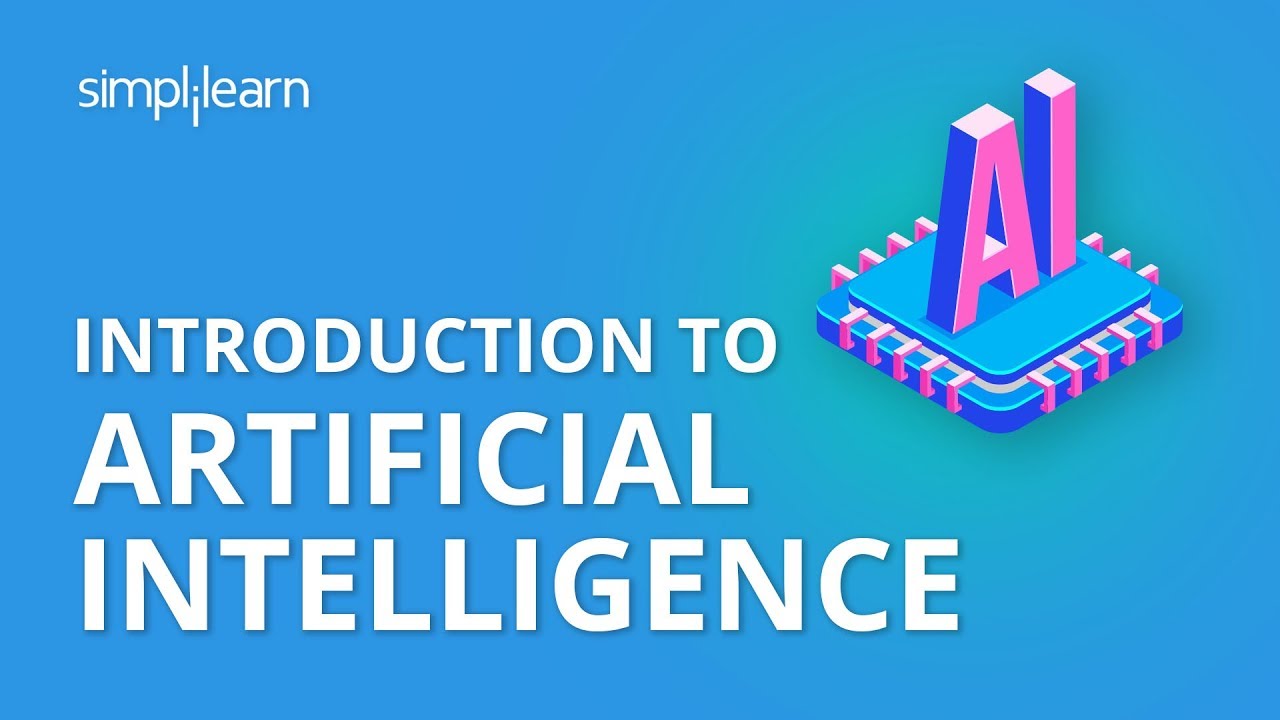
Introduction To Artificial Intelligence | What Is AI?| Artificial Intelligence Tutorial |Simplilearn
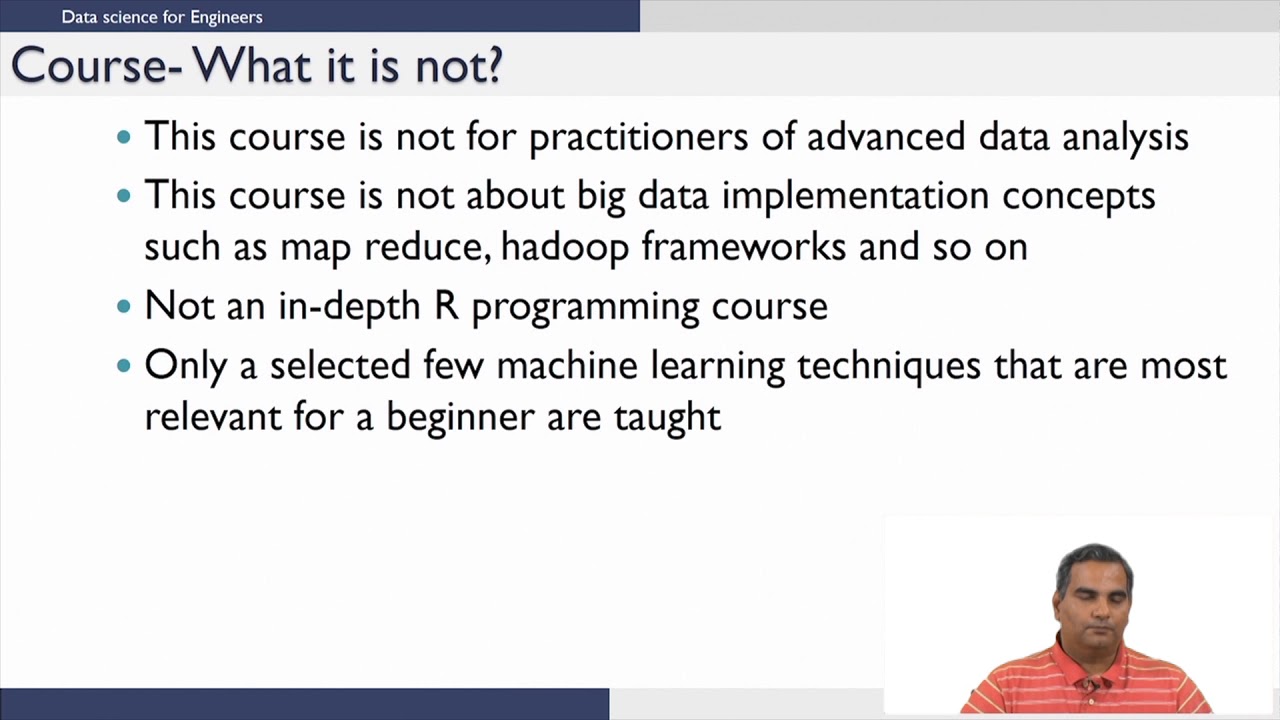
Data science for engineers Course philosophy and expectation
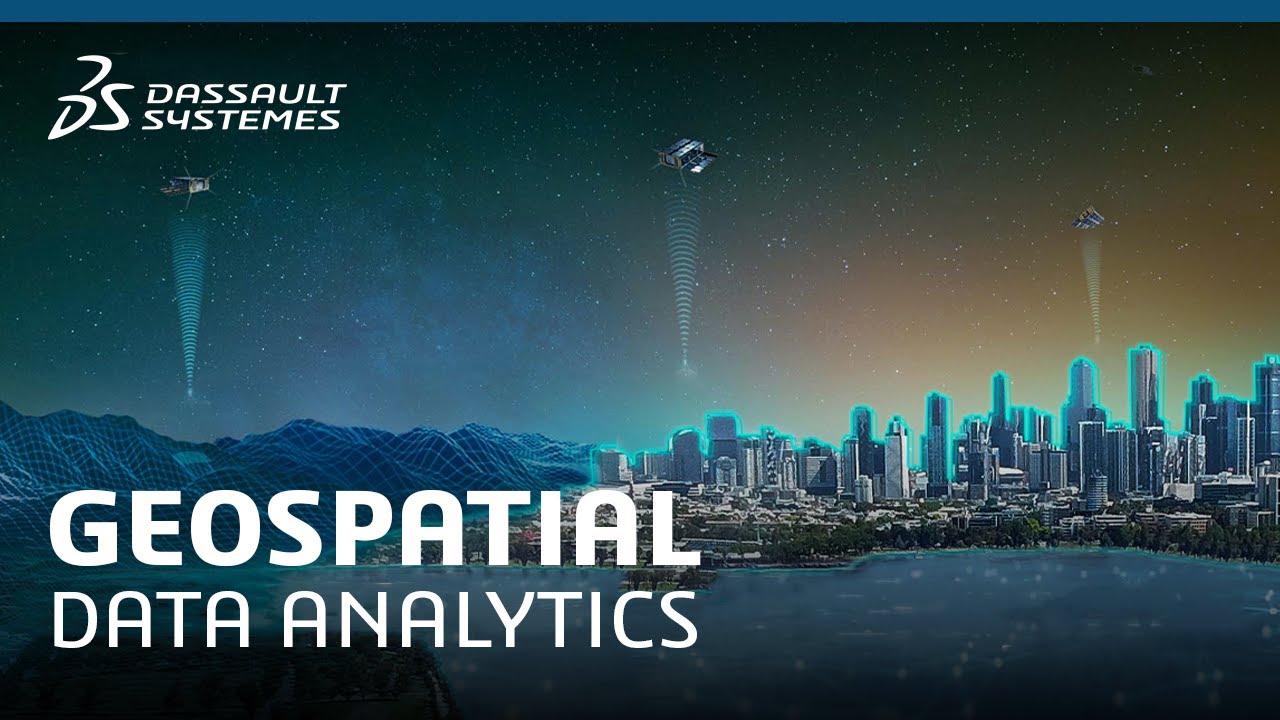
The Power of Geospatial Data Analytics – Dassault Systèmes

1-Course Introduction
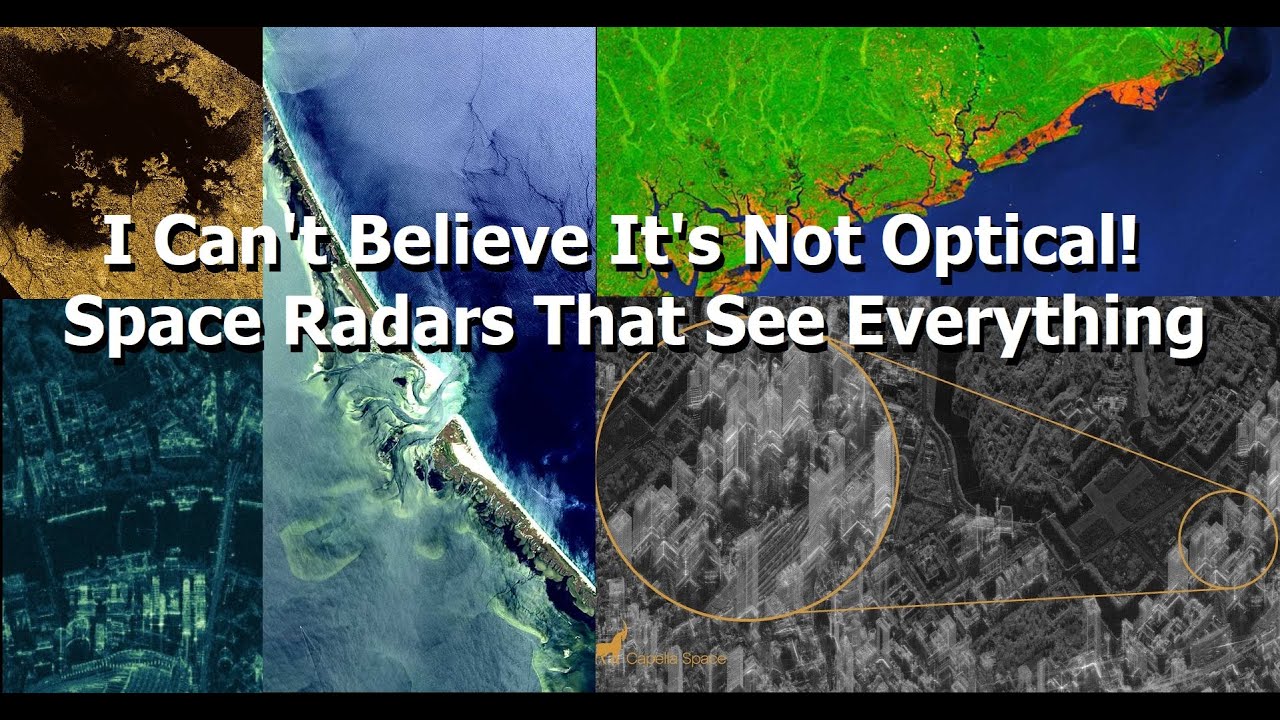
Satellites Use 'This Weird Trick' To See More Than They Should - Synthetic Aperture Radar Explained.
5.0 / 5 (0 votes)