Operations & Supply Chain Management: Forecasting & Demand Planning
Summary
TLDRThe video covers key concepts in forecasting and demand planning, explaining how organizations project future values to guide decisions. It highlights the importance of good forecasting in operations management, addressing time horizons, patterns like trends and seasonality, and statistical methods such as moving averages and regression. It also emphasizes the blend of science and art in forecasting, combining quantitative models with human judgment. Forecast accuracy and methods like mean squared error and tracking signals are discussed, underscoring forecasting's role in predicting demand and guiding decisions, despite its inherent imperfections.
Takeaways
- đ Forecasting involves projecting future values for variables and is crucial for decision-making in operations management.
- âïž Good forecasting leads to effective inventory and staffing decisions, while poor forecasting can result in shortages and poor customer service.
- âł Forecasting can be short-term (up to 3 months), intermediate (3 to 12 months), or long-range (10 years or more). Different operations decisions are made in each horizon.
- đ A time bucket is the unit of measure for a forecast period, such as a year, month, week, or minute.
- đ Time series forecasting relies on historical data to predict future outcomes, often exhibiting trends that can be linear or non-linear.
- đ Seasonal and cyclical patterns in time series data help with forecasting, while random and irregular variations make it more challenging.
- âïž Forecast errors are measured using metrics like Mean Squared Error (MSE), Mean Absolute Deviation (MAD), and Mean Absolute Percentage Error (MAPE) to select the best model.
- đ Simple statistical methods include moving averages and exponential smoothing, while regression models establish relationships between variables for more complex forecasting.
- đšâđ« Judgmental forecasting uses expert opinions when quantitative data is insufficient, employing methods like grassroots forecasting and the Delphi method.
- đŻ Combining different forecasting models and adjusting for future events can improve accuracy, and using tracking signals helps monitor forecast bias.
Q & A
What is the definition of forecasting in the context of operations management?
-Forecasting is the process of projecting the values of one or more variables into the future to aid in decision-making related to operations.
Why is forecasting important for organizations?
-Good forecasts help organizations make informed decisions about operations, such as inventory and staffing, whereas poor forecasts can lead to shortages, inadequate customer service, and complaints.
What are the three types of planning horizons in forecasting?
-The three planning horizons are short-term (up to 3 months), intermediate (3 to 12 months), and long-range (up to 10 years or more). Different types of operational decisions are made in each horizon.
What is the difference between linear and non-linear trends in forecasting?
-A linear trend implies constant growth or decline over time, represented by a straight line, while a non-linear trend implies varying growth or decline, represented by a curve.
What is the significance of time series data in forecasting?
-Time series data, which is a set of observations measured over time, is important for forecasting as it relies on historical data to predict future outcomes.
What are some common statistical forecasting methods mentioned in the video?
-Common methods include simple moving averages, simple exponential smoothing (SES), and regression analysis, which help predict future values based on historical data.
What are the key differences between mean squared error (MSE), mean absolute deviation (MAD), and mean absolute percentage error (MAPE)?
-MSE squares individual forecast errors and averages them, MAD averages absolute deviations, and MAPE measures the average percentage error between forecasted and actual values.
What is the role of human judgment in forecasting?
-Human judgment is used when quantitative data is insufficient or unreliable. It helps refine forecasts, especially through methods like grassroots forecasting and the Delphi method.
What is grassroots forecasting and how does it differ from the Delphi method?
-Grassroots forecasting relies on input from people close to the customer, such as salespeople, whereas the Delphi method gathers expert opinions from knowledgeable personnel to develop forecasts.
What is a tracking signal, and how is it used in forecasting?
-A tracking signal monitors forecast accuracy by quantifying bias, which is the tendency for forecasts to consistently differ from actual values. It helps organizations detect and correct forecast errors.
Outlines
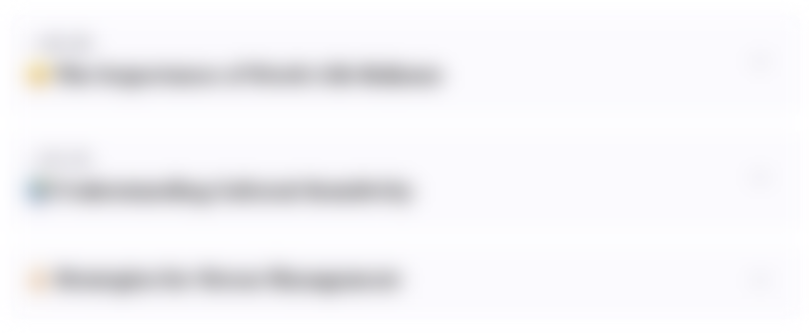
Cette section est réservée aux utilisateurs payants. Améliorez votre compte pour accéder à cette section.
Améliorer maintenantMindmap
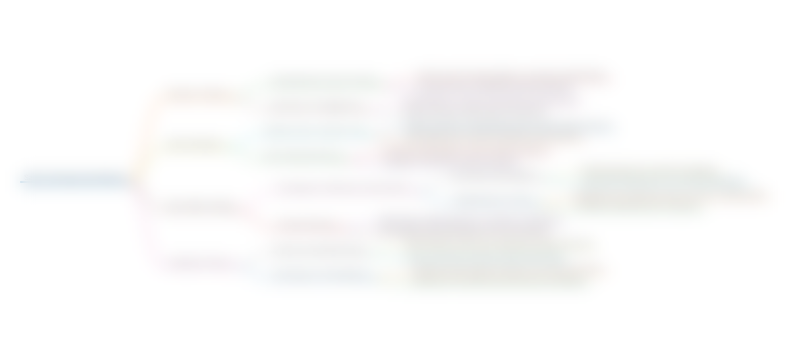
Cette section est réservée aux utilisateurs payants. Améliorez votre compte pour accéder à cette section.
Améliorer maintenantKeywords
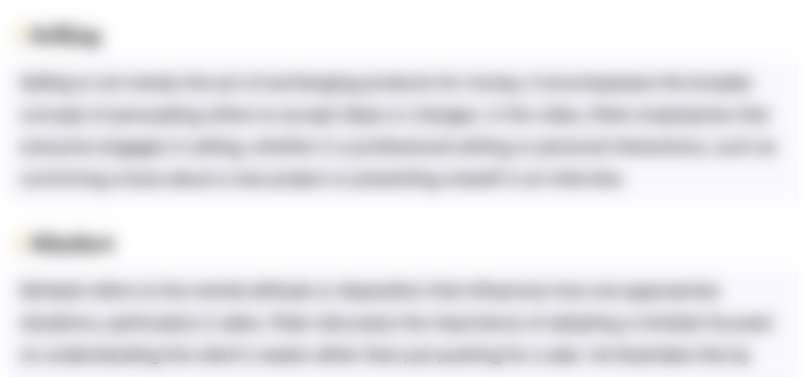
Cette section est réservée aux utilisateurs payants. Améliorez votre compte pour accéder à cette section.
Améliorer maintenantHighlights
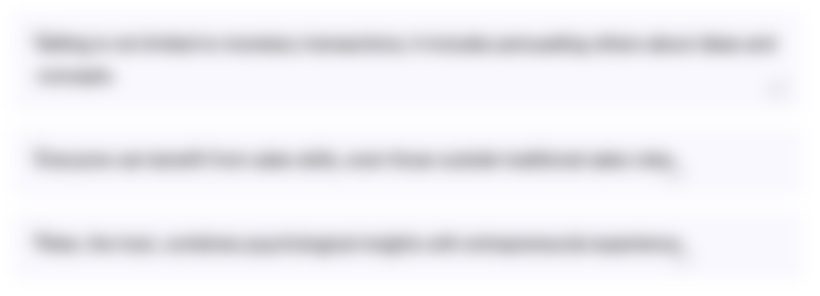
Cette section est réservée aux utilisateurs payants. Améliorez votre compte pour accéder à cette section.
Améliorer maintenantTranscripts
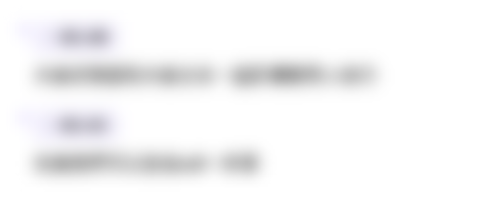
Cette section est réservée aux utilisateurs payants. Améliorez votre compte pour accéder à cette section.
Améliorer maintenantVoir Plus de Vidéos Connexes
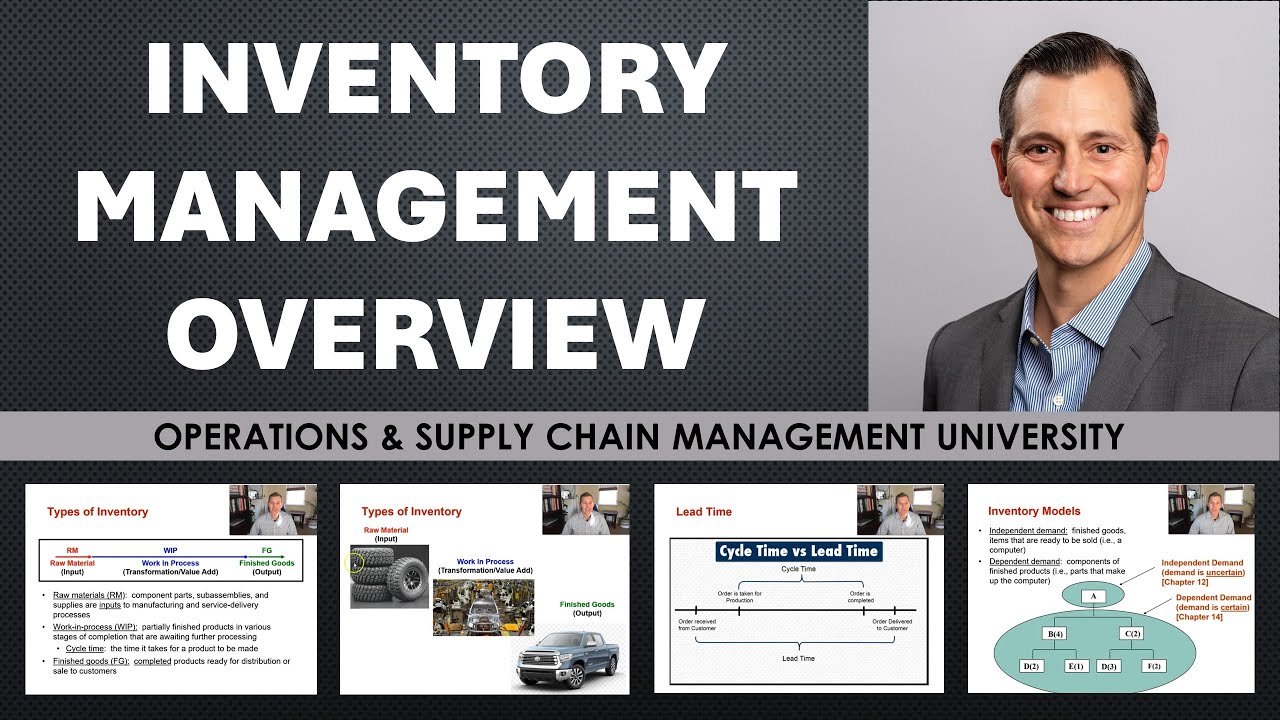
Inventory Management overview in Operations & Supply Chain Management
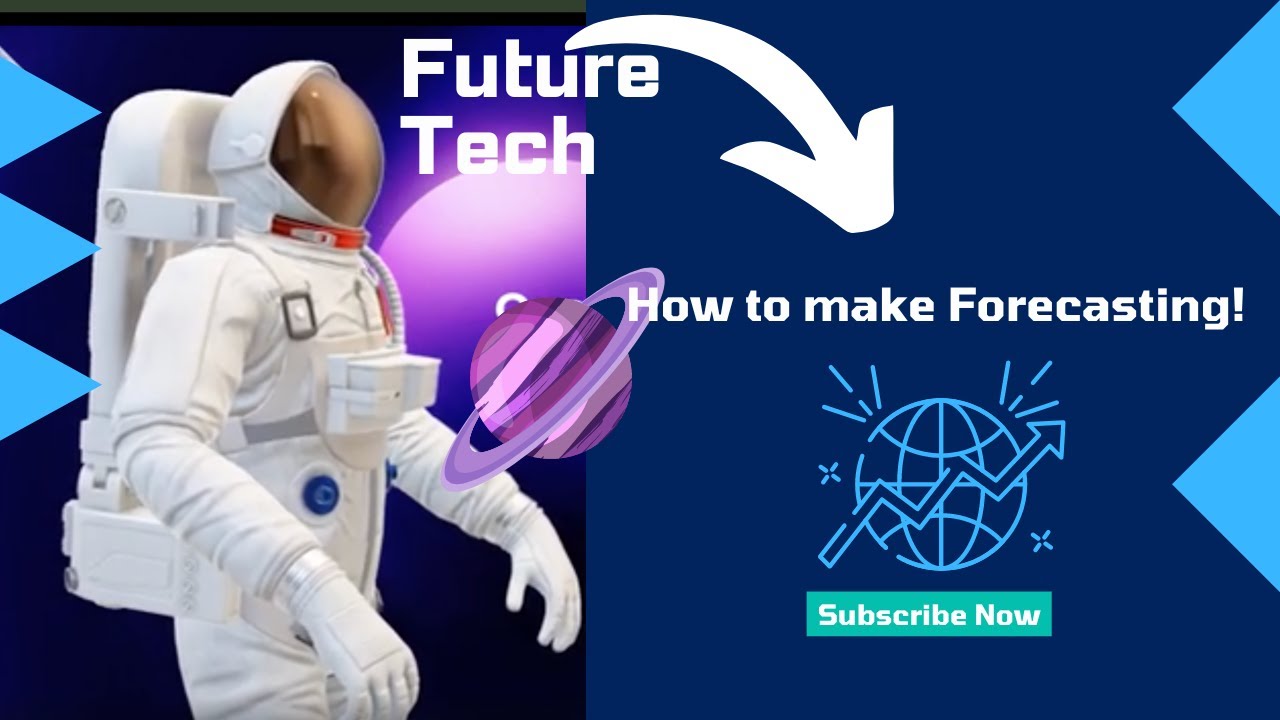
How to make Forecasting! | Quantitative Forecasting with Least Squares Method | Quickly & easily

MATERI KULIAH MANAJEMEN OPERASIONAL - PERENCANAAN KAPASITAS
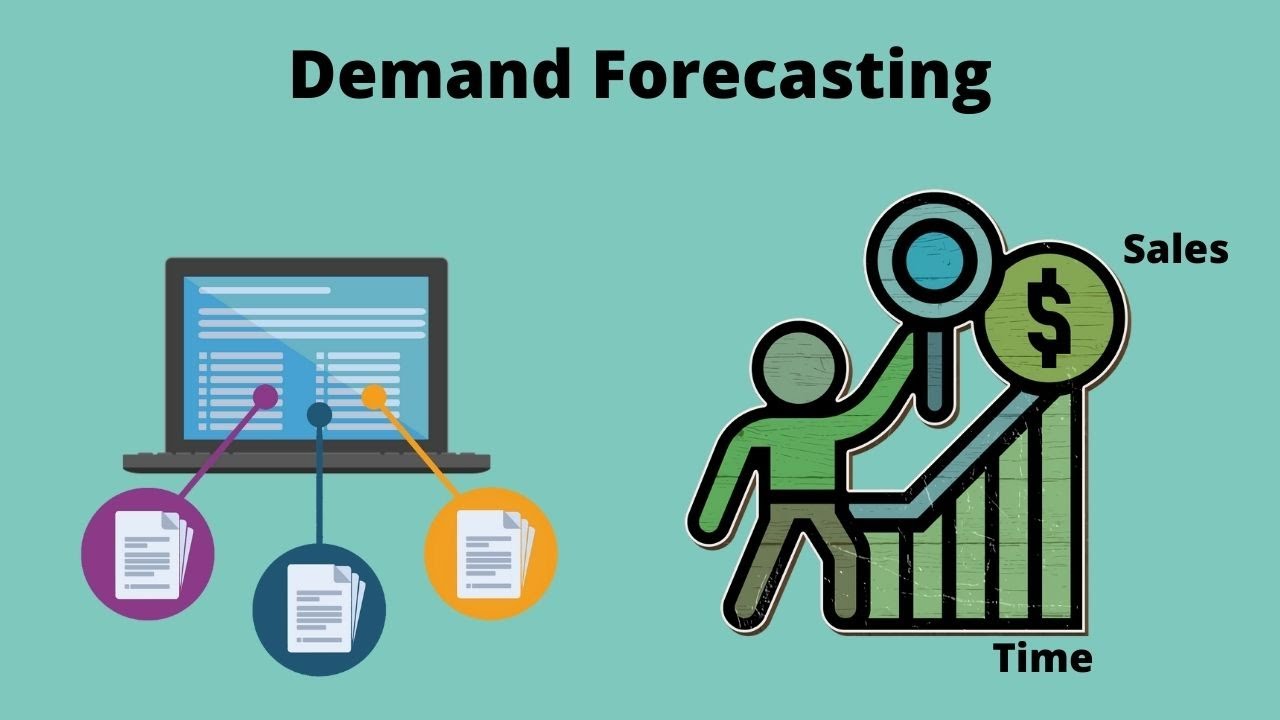
What is Demand Forecasting?
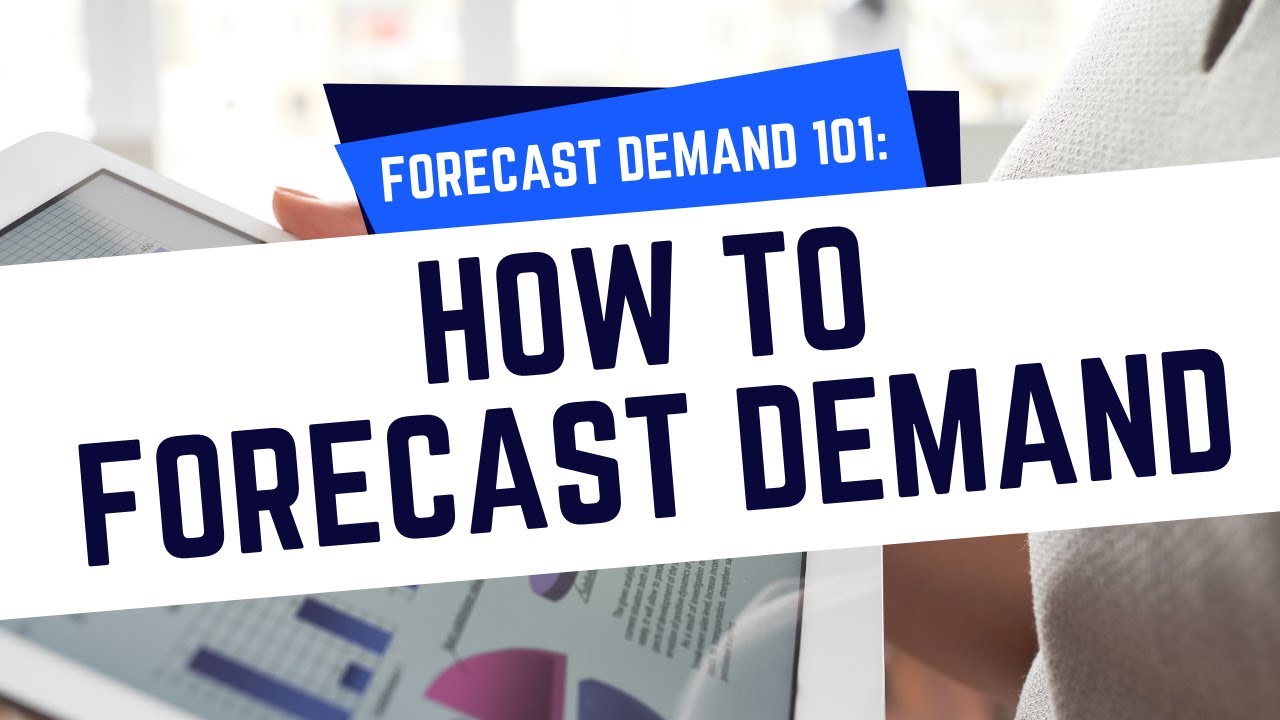
Demand Forecasting 101: What Is It & Why It's Important

Peramalan Permintaan Part 1 (Moving Average and Weighted Moving Average)
5.0 / 5 (0 votes)