Miles Cranmer - The Next Great Scientific Theory is Hiding Inside a Neural Network (April 3, 2024)
Summary
TLDRThe transcript discusses the emerging paradigm of interpreting neural networks for physical insights in scientific discovery. It highlights the potential of AI in learning complex models from limited data, exemplified by advances in fluid turbulence and planetary system instability prediction. The speaker emphasizes the importance of translating these models into interpretable mathematical language using symbolic regression. They also introduce the concept of polymathic AI, which involves creating large, flexible neural networks trained on diverse data to serve as foundational models for various scientific tasks, promoting a new approach to building theories in the physical sciences.
Takeaways
- đ§ The concept of interpreting neural networks for physical insights represents a new paradigm in scientific exploration.
- đ Success in using neural networks for scientific insights includes predicting instability in planetary systems and modeling fluid turbulence with high accuracy.
- đ Traditional scientific methods involve building theories from low-dimensional data, while modern AI-driven approaches use high-dimensional data and flexible functions.
- đ The speaker's motivation is to understand how neural networks achieve accuracy and to use these insights to advance scientific understanding.
- đ§Ź The potential of machine learning in science is highlighted by the ability of neural networks to learn from data and find patterns not previously recognized.
- đ Symbolic regression is a technique used to interpret neural networks by finding analytic expressions that optimize to fit data sets.
- đ§Ź The use of genetic algorithms in symbolic regression is akin to evolving equations to fit data, providing a bridge between machine learning models and mathematical language.
- đ Foundation models, like GPT for language, are proposed for science as a way to train on diverse data and then specialize for specific tasks, improving performance.
- đ The concept of 'polymathic AI' is introduced as a foundation model for science that can incorporate data across disciplines and be fine-tuned for particular problems.
- đ The importance of simplicity in scientific models is questioned, with the suggestion that what is considered simple may be based on familiarity and utility rather than inherent simplicity.
Q & A
What is the main motivation behind interpreting neural networks for physical insights?
-The main motivation is to extract valuable scientific insights from neural networks, which can potentially advance our understanding of various phenomena and contribute to the development of new theories in the physical sciences.
How does the traditional approach to science differ from the new paradigm of using neural networks?
-The traditional approach involves building theories based on low-dimensional data sets or summary statistics, whereas the new paradigm uses massive neural networks to find patterns and insights in large, complex data sets, and then builds theories around what the neural networks have learned.
Can you explain the concept of symbolic regression in the context of interpreting neural networks?
-Symbolic regression is a machine learning task that aims to find analytic expressions that optimize some objective by searching over all possible expression trees. It is used to build surrogate models of neural networks, translating the model into a mathematical language that is interpretable and familiar to scientists.
What is the significance of the universal approximation theorem in relation to neural networks?
-The universal approximation theorem states that a shallow neural network with a single layer of activations can approximate any 1D function to arbitrary accuracy. This highlights the power of neural networks in modeling complex relationships and functions in data.
How do foundation models like GPT differ from traditional machine learning models?
-Foundation models are trained on massive, diverse datasets and are flexible enough to serve as a basis for a wide range of tasks across different domains. They are first pre-trained on general data and then fine-tuned for specific tasks, whereas traditional models are often trained from scratch for a particular task.
What is the role of simplicity in the context of scientific discovery and interpretability?
-In the context of scientific discovery, simplicity often refers to the ability to describe complex phenomena with minimal assumptions or variables. It aids interpretability by providing clear, understandable explanations for observed data, which can lead to more effective models and theories.
How does the concept of pre-training neural networks relate to the development of polymathic AI?
-Pre-training neural networks on a broad range of data allows them to develop general priors for different types of problems, much like a well-rounded scientist. This approach is central to the development of polymathic AI, which aims to create models that can be fine-tuned for specific tasks across various scientific disciplines.
What are the potential challenges in training a foundation model for science, given the diversity of data types in different scientific fields?
-The main challenge lies in defining a general objective that can be applied to the diverse range of data types in science. The objective needs to be flexible enough to accommodate different data forms, such as sequences in molecular biology or images in astrophysics, while still enabling the model to learn broadly applicable concepts.
How does the concept of shared concepts across different physical systems relate to the training of foundation models?
-Shared concepts like causality and multiscale dynamics are common across various scientific disciplines. By training a foundation model on diverse datasets that encompass these shared concepts, the model can develop a general understanding of these principles, which can then be fine-tuned for specific tasks within particular fields.
What are the potential implications of polymathic AI for the future of scientific research?
-Polymathic AI has the potential to revolutionize scientific research by providing a generalizable foundation model that can quickly adapt to new tasks and problems. This could lead to faster discoveries, more efficient use of computational resources, and the development of new, broadly applicable scientific models.
Outlines
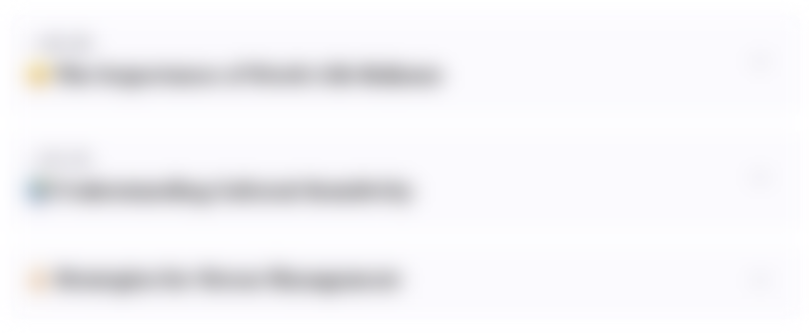
Cette section est réservée aux utilisateurs payants. Améliorez votre compte pour accéder à cette section.
Améliorer maintenantMindmap
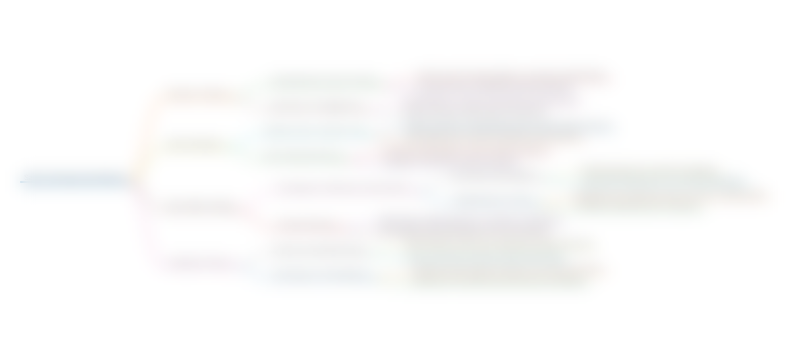
Cette section est réservée aux utilisateurs payants. Améliorez votre compte pour accéder à cette section.
Améliorer maintenantKeywords
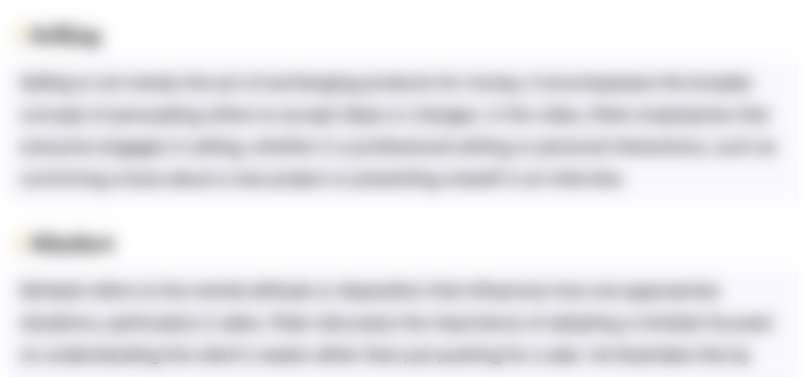
Cette section est réservée aux utilisateurs payants. Améliorez votre compte pour accéder à cette section.
Améliorer maintenantHighlights
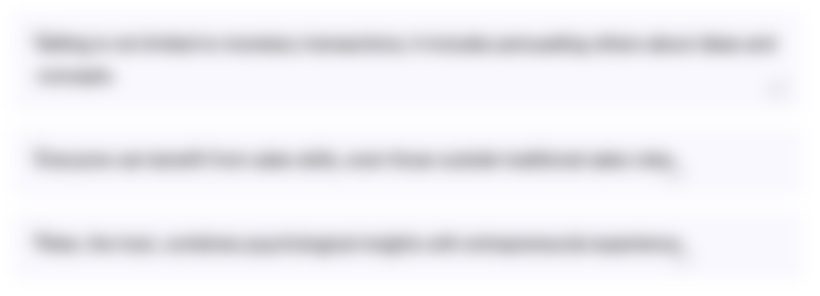
Cette section est réservée aux utilisateurs payants. Améliorez votre compte pour accéder à cette section.
Améliorer maintenantTranscripts
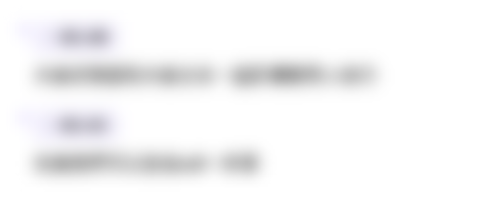
Cette section est réservée aux utilisateurs payants. Améliorez votre compte pour accéder à cette section.
Améliorer maintenant5.0 / 5 (0 votes)