Liquid Neural Networks | Ramin Hasani | TEDxMIT
Summary
TLDRThe transcript explores the evolving landscape of AI, particularly focusing on deep learning and neural networks. It discusses how larger models improve accuracy but also face challenges like robustness and bias. The speaker contrasts traditional statistics with modern AI, introducing liquid neural networks as an innovative solution. These smaller, adaptable systems outperform traditional models in certain tasks like autonomous driving and object detection. The talk emphasizes the need for smarter AI design and accountability while highlighting the potential for smaller networks with greater generalization and adaptability in real-world applications.
Takeaways
- 😀 Deep learning can handle complex tasks, such as converting textual input into images, with large models having millions or even billions of parameters.
- 😀 As model size increases, the accuracy of AI models improves, but there's a point where more parameters don't result in further accuracy gains and new behaviors emerge in 'over-parameterized' models.
- 😀 Larger AI models show improved generalization and robustness in decision-making, but they may struggle with underrepresented samples and reasoning tasks.
- 😀 The ability of large AI models to reason logically stays the same unless they're provided with a physical simulation engine to enhance their intuitive physics understanding.
- 😀 Deep learning models can achieve impressive results, but they also come with challenges such as high carbon footprints, biases, and fairness issues.
- 😀 Liquid neural networks, inspired by brain science, offer a new approach with fewer parameters while maintaining the desirable characteristics of larger models.
- 😀 Liquid neural networks are adaptable even after training, allowing them to respond to incoming inputs and exhibit robustness.
- 😀 In autonomous driving, liquid neural networks, with just 19 neurons, can efficiently make decisions and maintain focus despite input noise and environmental changes.
- 😀 Liquid neural networks show superior decision-making compared to traditional AI systems, with clear attention maps focused on relevant features in the environment.
- 😀 In scenarios like drone navigation, liquid neural networks show the ability to focus on the correct task despite environmental challenges, outperforming standard deep learning models.
- 😀 Smarter design in AI, such as the development of liquid neural networks, may offer solutions to challenges faced by large-scale AI models, such as accountability, bias, and energy consumption.
Q & A
What is the main concept discussed in the transcript regarding AI models?
-The main concept discussed is how AI models, specifically deep learning models, behave as their size increases. The speaker explains how traditional statistical models suggest diminishing returns beyond a certain size, but in deep learning, larger models continue to show improvements, entering an 'over-parameterized regime' where new behaviors emerge.
What is the 'over-parameterized regime' in deep learning?
-The 'over-parameterized regime' refers to a stage where the size of neural networks exceeds that of the training data, yet the model still performs well in terms of accuracy. In this regime, models exhibit better generalization and robustness, but reasoning abilities do not improve unless supported by a physical simulator.
How do large-scale AI models improve robustness?
-Large-scale AI models improve robustness by becoming less sensitive to input perturbations, such as environmental changes or noise. As the model size increases, the system is better able to maintain performance and adapt to variations in the data.
What is the challenge with underrepresented samples in large models?
-While large models show general improvements in many areas, they tend to perform poorly on underrepresented or minority samples. This can lead to biases in decision-making and underperformance for certain segments of the data.
What role does reasoning play in large-scale models?
-Reasoning capabilities in large-scale models do not improve just by increasing the model size. The ability to understand and reason logically from a task remains unchanged unless the model is provided with additional tools, like a physical simulation engine, to mimic intuitive reasoning.
What are the concerns related to large-scale AI models?
-Concerns include the energy consumption and carbon footprint of training large models, as well as issues of bias, fairness, and the accountability of AI systems in decision-making processes, particularly in their deployment within society.
What is the concept of liquid neural networks?
-Liquid neural networks are inspired by brain science and consist of small, adaptable systems that can continue to change after training. These networks have fewer parameters but still exhibit high performance and can adapt to new inputs, which is crucial for building responsible, efficient AI systems.
How do liquid neural networks differ from traditional deep learning models?
-Unlike traditional deep learning models, liquid neural networks can adapt after training based on incoming data, making them more efficient and adaptable. They also have a much smaller number of parameters while still achieving similar performance, especially in real-world applications like autonomous driving.
What are the advantages of liquid neural networks in autonomous driving?
-In autonomous driving, liquid neural networks can drive a car with only 19 neurons while maintaining the ability to focus on relevant areas (like edges and the road horizon) even when noisy inputs are added. This is a significant advantage over traditional systems, which may be less focused or more affected by environmental perturbations.
What challenges did the drone task reveal in the comparison between traditional models and liquid neural networks?
-In the drone task, traditional models showed scattered and ineffective attention, focusing more on irrelevant factors like lighting conditions rather than the objective. In contrast, liquid neural networks demonstrated more focused attention on the target object, enabling the drone to perform the task effectively even in unstructured environments.
Outlines
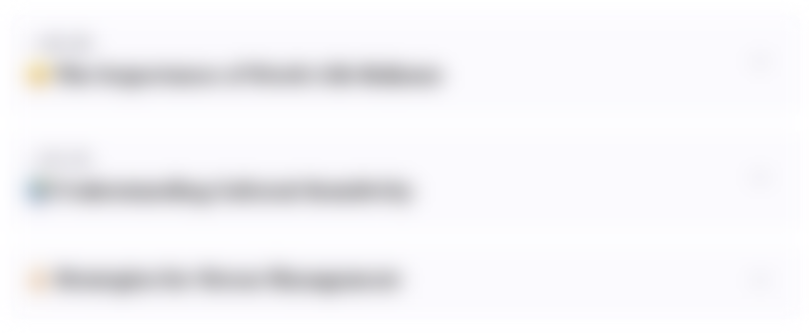
This section is available to paid users only. Please upgrade to access this part.
Upgrade NowMindmap
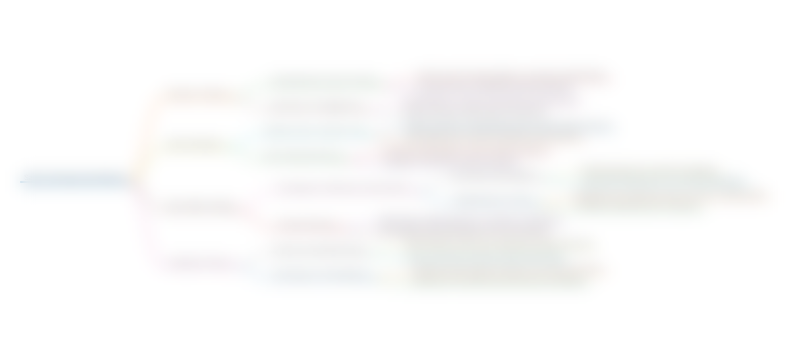
This section is available to paid users only. Please upgrade to access this part.
Upgrade NowKeywords
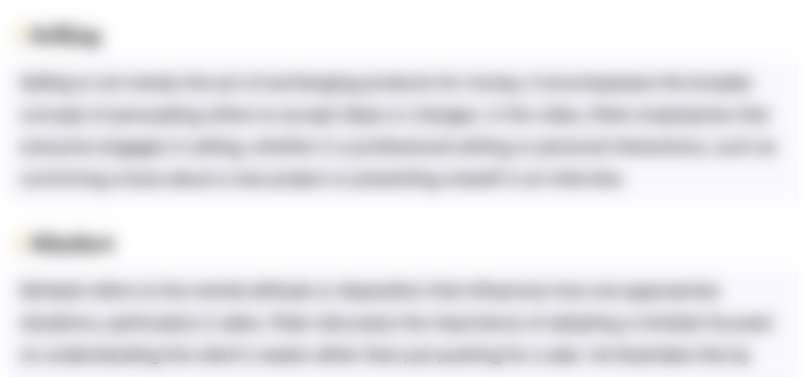
This section is available to paid users only. Please upgrade to access this part.
Upgrade NowHighlights
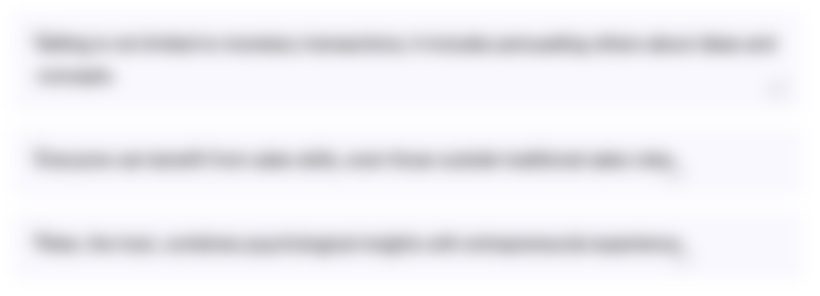
This section is available to paid users only. Please upgrade to access this part.
Upgrade NowTranscripts
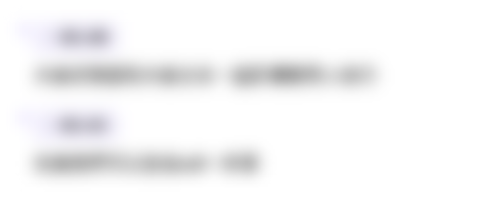
This section is available to paid users only. Please upgrade to access this part.
Upgrade NowBrowse More Related Video
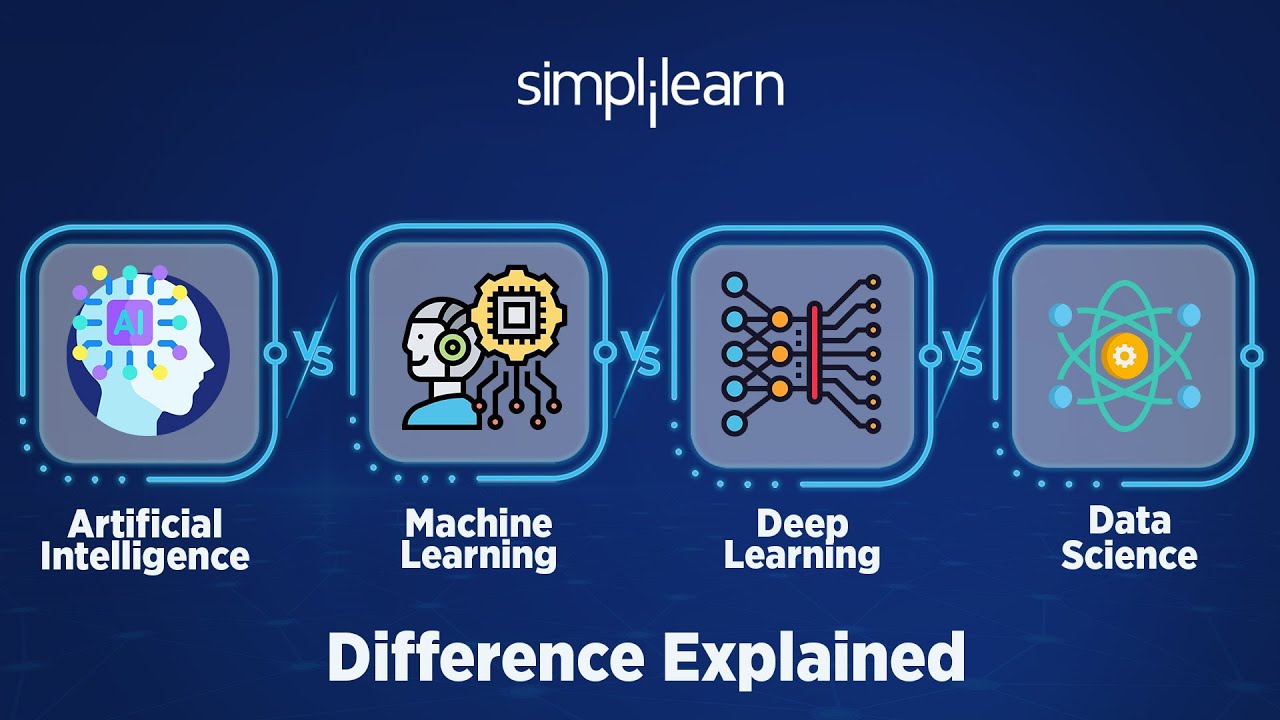
AI vs ML vs DL vs Data Science - Difference Explained | Simplilearn
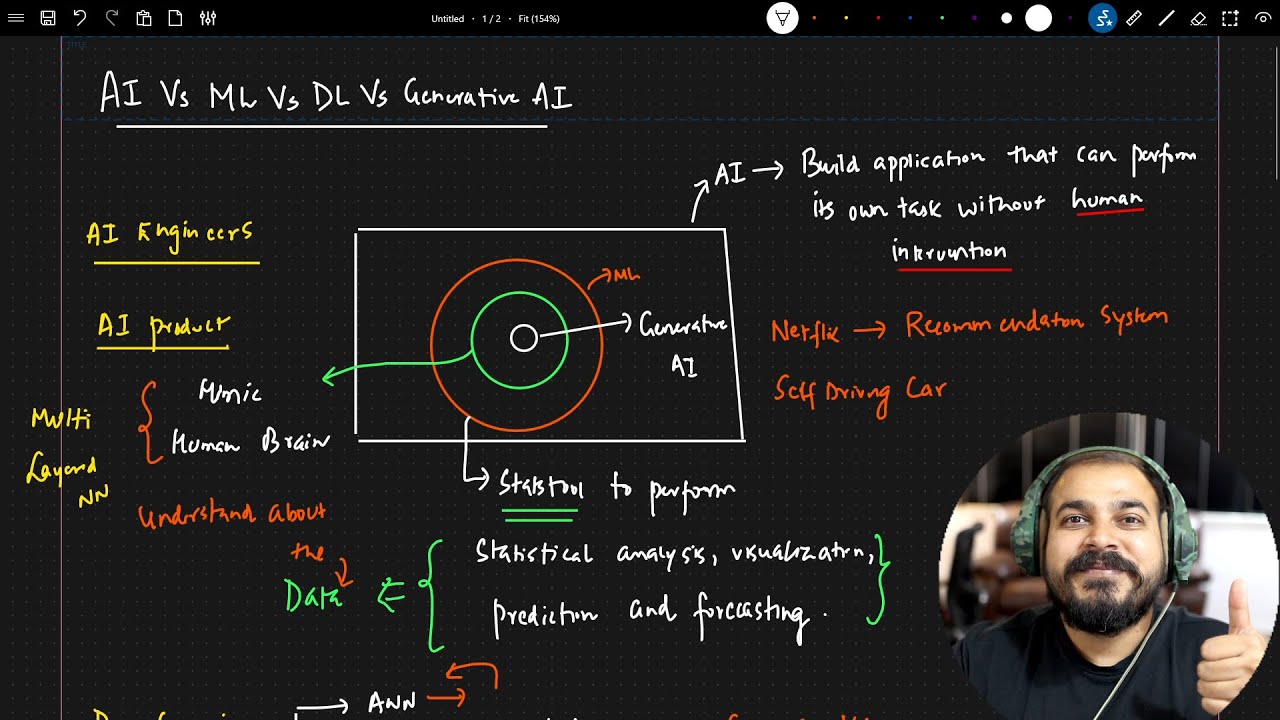
AI vs ML vs DL vs Generative Ai

L'intelligence artificielle, comment ça marche ? Extrait de conférence, Nov 2024
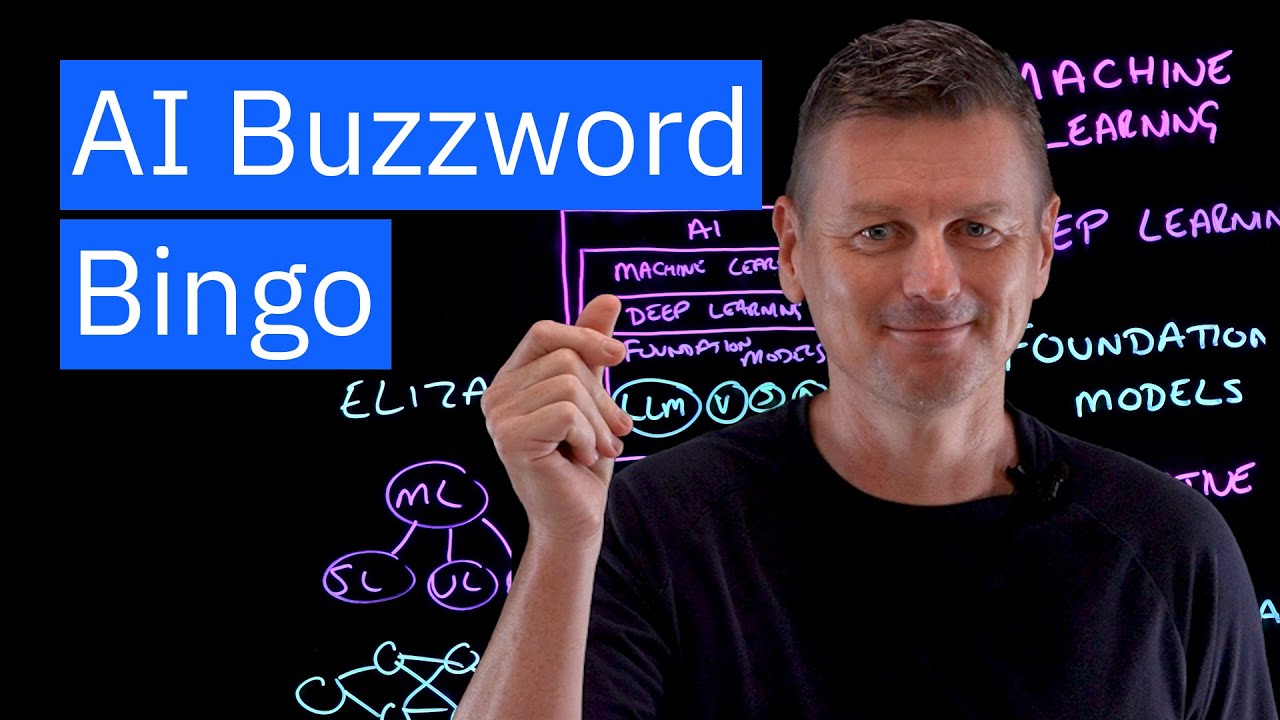
Machine Learning vs. Deep Learning vs. Foundation Models
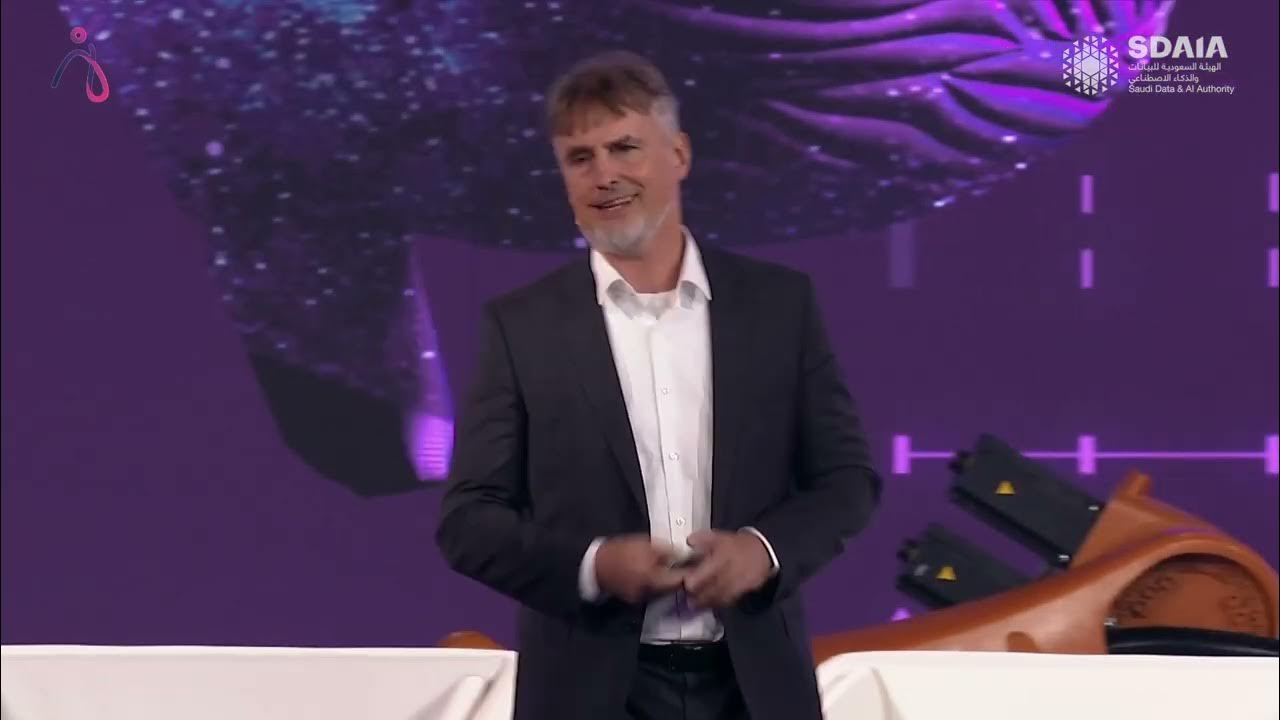
Dr. Jüergen Schmidhuber Keynote - Global AI Summit 2022
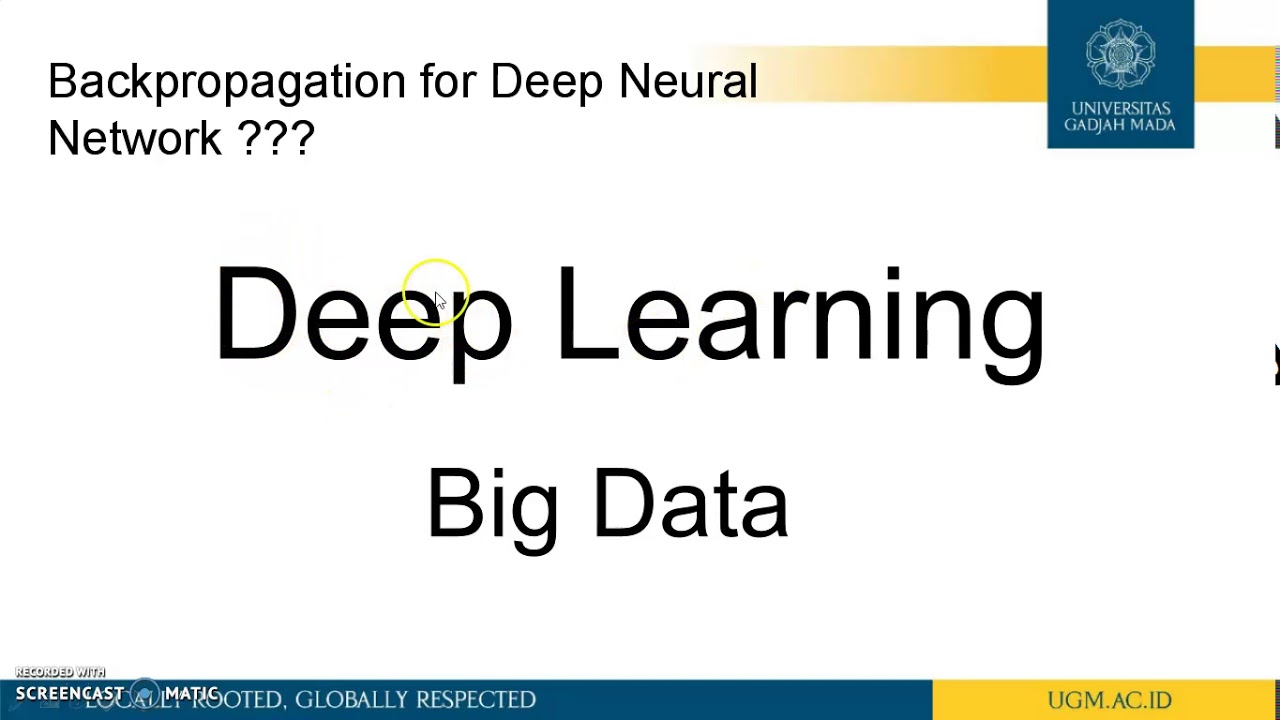
Introduction to Deep Learning - Part 2
5.0 / 5 (0 votes)