Taxonomy of Neural Network
Summary
TLDRThis video script delves into the classification of natural networks, focusing on the taxonomy of neural networks. It explains various types such as perceptron neural networks, single-layer perceptrons, and multi-layer perceptrons, highlighting their structure and functionality. The script also touches on recurrent neural networks, their ability to remember and process sequential data, and long-term memory. It further discusses interconnected networks and their applications in image processing and classification, mentioning different types of neural networks like CNNs and their significance in feature extraction and categorization.
Takeaways
- π§ The video discusses the classification of neural networks in the context of natural language processing and science.
- π It explains the concept of perceptron neural networks, which are simple and do not have hidden layers.
- π The script touches on the limitations of single-layer perceptron neural networks in handling complex problems.
- π The video introduces the forward-propagation method used in neural networks, where input is given and output is produced.
- π‘ It mentions the development of multi-layer perceptron neural networks, which use multiple layers to process information.
- π The script highlights the use of recurrent neural networks (RNNs) for sequence data and their ability to maintain memory of previous inputs.
- π The video explains the concept of long short-term memory (LSTM) networks, which are a type of RNN designed to remember information for longer periods.
- π It discusses the role of convolutional neural networks (CNNs) in image processing and classification, emphasizing their use in feature extraction.
- π The script also covers the topic of fully connected networks, which are a type of artificial neural network where each neuron in one layer is connected to every neuron in the next layer.
- π The video concludes with a brief overview of different types of neural networks and their applications in various fields.
Q & A
What is the main topic of the video?
-The main topic of the video is the classification of neural networks, including various types such as perceptron neural networks, single-layer perceptrons, multi-layer perceptrons, recurrent neural networks, and convolutional neural networks.
What is a perceptron neural network?
-A perceptron neural network is a simple type of neural network that has input units and no hidden layer. It is used for binary classification tasks.
How does a single-layer perceptron work?
-A single-layer perceptron works by receiving input, processing it through a linear combination, and then applying a threshold function to produce an output.
What is a multi-layer perceptron?
-A multi-layer perceptron is a type of feedforward artificial neural network that has multiple layers of nodes, at least one hidden layer, and a non-linear activation function.
What is the role of the activation function in a neural network?
-The activation function in a neural network introduces non-linear properties to the model, allowing it to learn and model complex patterns in the data.
What is a recurrent neural network and how does it differ from other types of neural networks?
-A recurrent neural network is a type of neural network that uses loops or cycles in its structure, allowing it to maintain a form of internal memory. It differs from other types of neural networks by being capable of processing sequences of data and exhibiting dynamic temporal behavior.
What is the purpose of long-term memory in a neural network?
-The purpose of long-term memory in a neural network is to store information for extended periods, allowing the network to recall and use that information for future tasks.
How does a convolutional neural network process images?
-A convolutional neural network processes images by applying a series of filters to the input image, which allows it to extract features and perform classification tasks.
What is the significance of the term 'feedforward' in the context of neural networks?
-In the context of neural networks, 'feedforward' refers to the process where the input data is passed through the network in a forward direction, layer by layer, until an output is produced without any feedback loops.
What is a fully connected layer in a neural network?
-A fully connected layer in a neural network is a layer where every neuron is connected to every neuron in the subsequent layer, allowing for complex interactions and computations.
How does a neural network with feedback differ from a feedforward network?
-A neural network with feedback, such as a recurrent neural network, allows for connections that form cycles, enabling the network to use past information to influence current computations. In contrast, a feedforward network has a unidirectional flow of information without any cycles.
Outlines
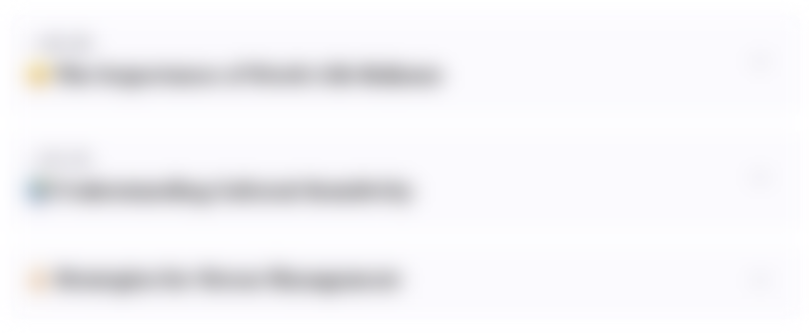
This section is available to paid users only. Please upgrade to access this part.
Upgrade NowMindmap
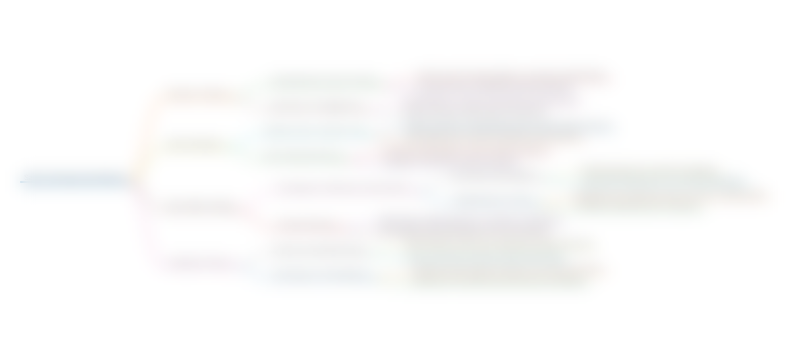
This section is available to paid users only. Please upgrade to access this part.
Upgrade NowKeywords
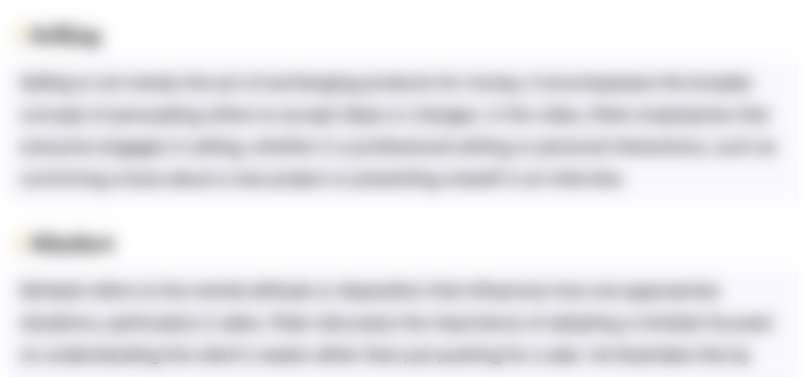
This section is available to paid users only. Please upgrade to access this part.
Upgrade NowHighlights
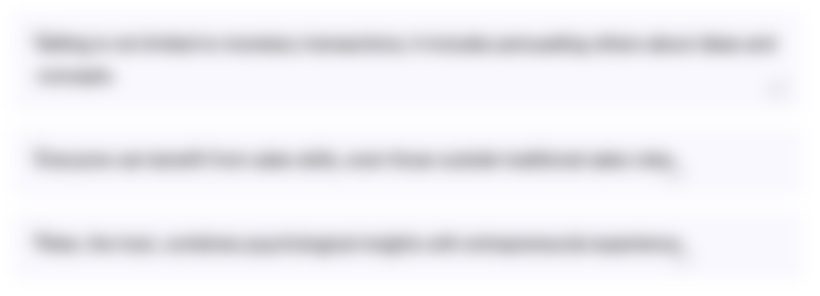
This section is available to paid users only. Please upgrade to access this part.
Upgrade NowTranscripts
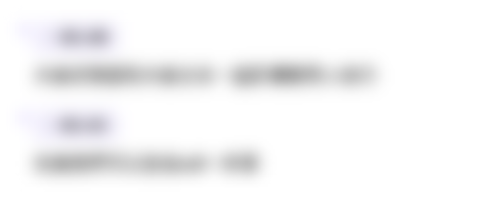
This section is available to paid users only. Please upgrade to access this part.
Upgrade NowBrowse More Related Video
5.0 / 5 (0 votes)