Ilya sutskever | Humanity will eventually move towards AGI | The intelligent body will soon appear
Summary
TLDR在这段访谈中,Ilya Sutskever回顾了深度学习和神经网络在计算机视觉领域的重大突破。他分享了自己对于如何训练深度网络的见解,以及这些网络如何彻底改变了机器学习领域。Sutskever讨论了他在OpenAI的工作,包括开发GPT系列模型和CLIP模型,以及这些技术如何推动了AI的边界。他还探讨了AI的未来,包括对提高模型可靠性和效率的期望,以及AI如何可能改变我们的工作和生活方式。
Takeaways
- 📈 神经网络在计算机视觉领域的突破性进展,大幅超越了以往的方法。
- 🤖 深度学习的成功部分归功于James Martin等人的研究,他们证明了可以从监督数据中端到端训练深度网络。
- 🧠 人类视觉的快速反应意味着不需要太多层次的神经网络就能实现可观的视觉效果。
- 🚀 随着大型数据集和GPU的兴起,为训练大型神经网络提供了可能,从而推动了深度学习的发展。
- 🌐 深度学习的成功也改变了人们对机器学习模型的看法,从追求简单优雅的数学证明转向接受更强大的模型。
- 🔑 神经网络被视为一种编程工具,通过反向传播算法对这些“小计算机”进行编程。
- 🔑 神经网络的并行计算特性使其能够处理复杂的模式识别任务,如围棋和机器翻译。
- 🌟 深度学习在图像识别和语言处理等领域的成功,展示了其在解决人类能够快速解决的问题上的巨大潜力。
- 💡 深度学习的发展推动了对神经网络更深层次理解的探索,包括其在强化学习、自然语言处理和计算机视觉等领域的应用。
- 🔮 未来的AI研究可能会继续探索如何使神经网络更加可靠、高效,并在更广泛的任务中发挥作用。
Q & A
神经网络是如何在计算机视觉领域取得突破的?
-神经网络通过端到端的训练方法,利用大量标记数据进行学习,从而在计算机视觉领域取得了突破。这种训练方式最初由Hinton等人提出,并在ImageNet竞赛中得到验证,神经网络的表现大幅超越了以往的方法。
为什么深度学习在早期没有被广泛接受?
-早期深度学习没有被广泛接受的原因是存在一些普遍的误解,比如认为深度网络无法训练,因为梯度消失或梯度爆炸问题。此外,当时普遍认为需要复杂的数学证明来保证机器学习模型的有效性,而神经网络则没有这样的证明。
人类视觉的快速识别能力对神经网络设计有何启示?
-人类视觉系统能够在几百毫秒内识别物体,而我们的神经元反应速度相对较慢。这说明不需要太多的层次就能实现有效的视觉识别,这对神经网络设计提供了启示,即可以通过构建较大但不需要太多层的网络来实现高效的计算机视觉。
为什么选择使用GPU来训练神经网络?
-GPU提供了并行处理大量数据的能力,这对于训练大型神经网络至关重要。在Alex Krizhevsky开发出能够在GPU上高效训练的卷积神经网络(CNN)之后,这种方法开始变得可行,因为它大大缩短了训练时间,使得在ImageNet等大规模数据集上训练成为可能。
神经网络在语言处理方面的应用是如何实现的?
-神经网络在语言处理方面的应用是通过将语言视为一种可以通过模式识别来处理的连续信号来实现的。通过训练神经网络来预测文本序列中的下一个词,网络能够学习语言的结构和语义,从而在机器翻译等任务中取得显著效果。
为什么选择DOTA作为强化学习的研究项目?
-DOTA是一个复杂的实时策略游戏,具有高度的挑战性,它要求玩家具有快速反应、战略思维和直觉。选择DOTA作为研究项目是为了测试和推动强化学习技术的极限,特别是在处理多变量、多步骤决策和长期规划方面。
GPT模型是如何通过预测来实现语言理解的?
-GPT模型通过预测文本序列中的下一个词来实现语言理解。如果模型能够准确预测接下来的单词,这意味着它已经理解了前面的文本内容。随着预测精度的提高,模型能够捕捉到更复杂的语言特征,如词汇、语法和语义。
什么是CLIP模型,它如何将语言和视觉结合起来?
-CLIP模型是一个多模态神经网络,它通过大规模的自然语言监督学习视觉概念。CLIP能够将文本描述与图像内容关联起来,从而实现对图像的理解。这种结合语言和视觉的方法使得模型能够在没有大量标注数据的情况下,学习丰富的视觉概念。
为什么说神经网络是通用的计算设备?
-神经网络被视作通用的计算设备,因为它们可以接受任何形式的输入,通过学习内部表示来处理复杂的任务。无论是视觉识别、语言理解还是策略游戏,神经网络都可以通过适当的训练来适应这些任务,显示出它们的通用性和灵活性。
如何提高神经网络的可靠性和可控性?
-提高神经网络的可靠性和可控性可以通过多种方式实现,包括使用更大的数据集进行训练、设计更复杂的网络结构、以及通过强化学习从人类反馈中学习。此外,还可以通过精心设计的提示(prompting)来引导模型产生期望的输出。
Outlines
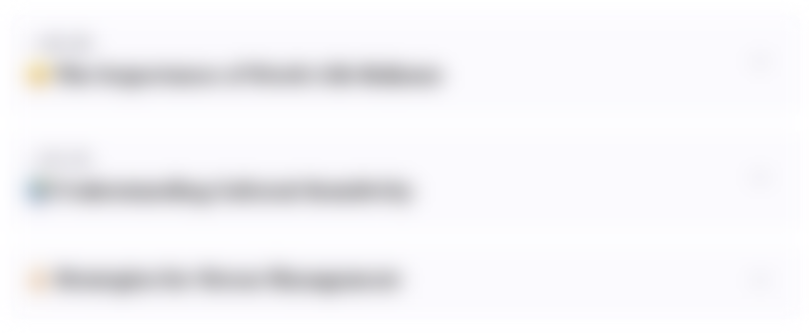
Cette section est réservée aux utilisateurs payants. Améliorez votre compte pour accéder à cette section.
Améliorer maintenantMindmap
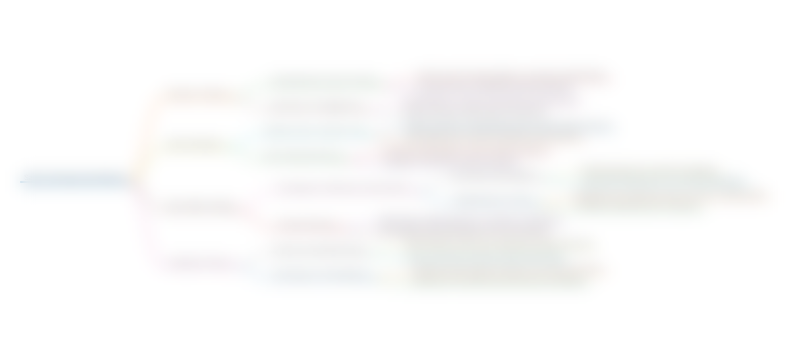
Cette section est réservée aux utilisateurs payants. Améliorez votre compte pour accéder à cette section.
Améliorer maintenantKeywords
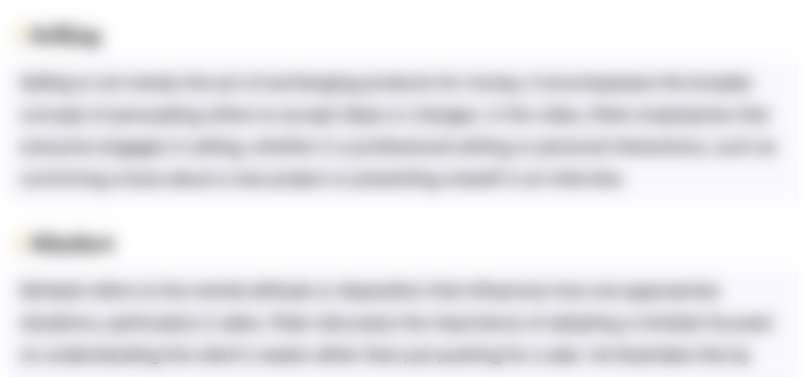
Cette section est réservée aux utilisateurs payants. Améliorez votre compte pour accéder à cette section.
Améliorer maintenantHighlights
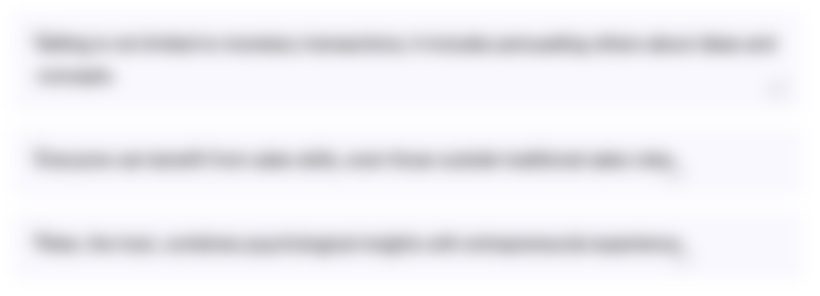
Cette section est réservée aux utilisateurs payants. Améliorez votre compte pour accéder à cette section.
Améliorer maintenantTranscripts
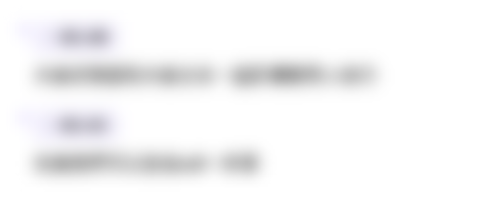
Cette section est réservée aux utilisateurs payants. Améliorez votre compte pour accéder à cette section.
Améliorer maintenantVoir Plus de Vidéos Connexes
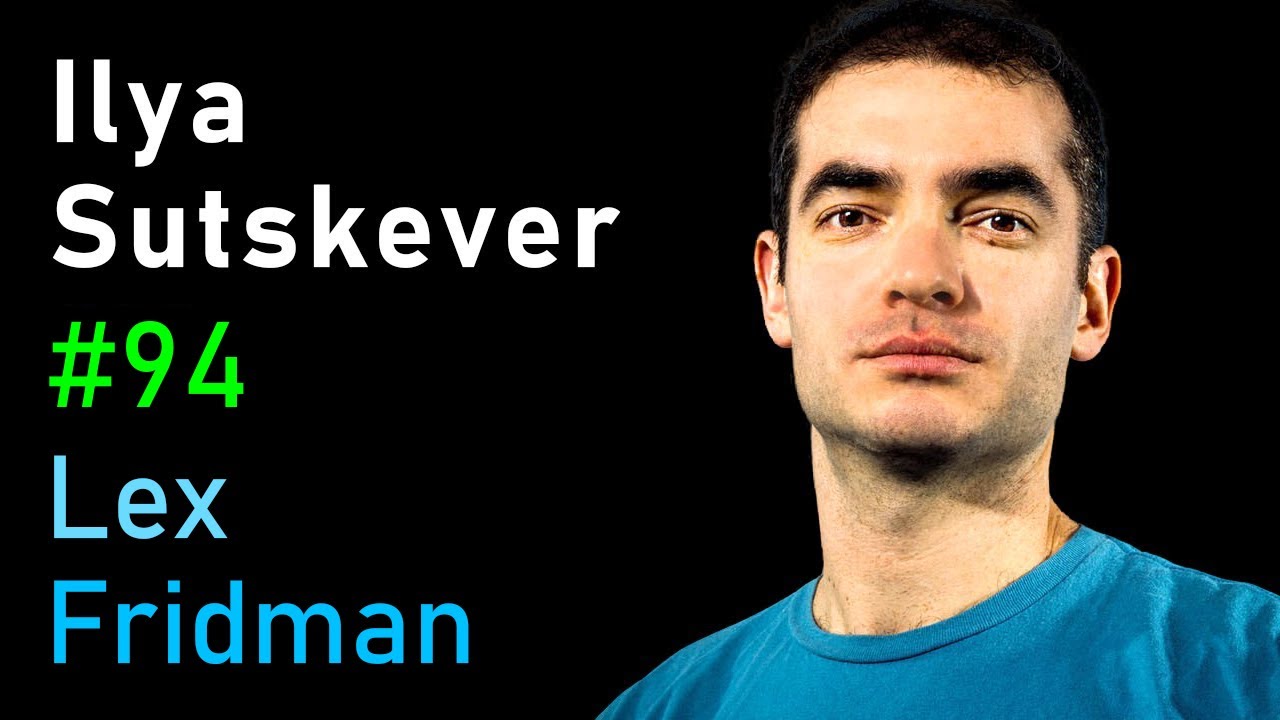
Ilya Sutskever: Deep Learning | Lex Fridman Podcast #94

Heroes of Deep Learning: Andrew Ng interviews Geoffrey Hinton
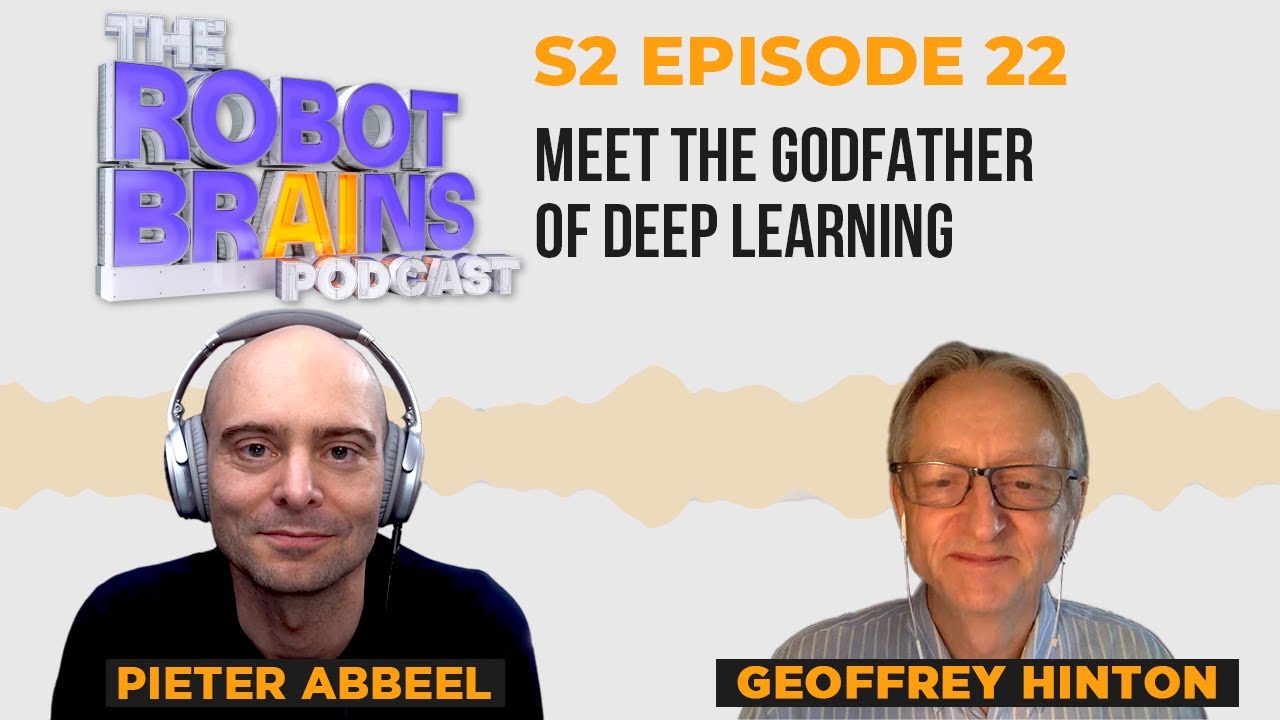
Season 2 Ep 22 Geoff Hinton on revolutionizing artificial intelligence... again
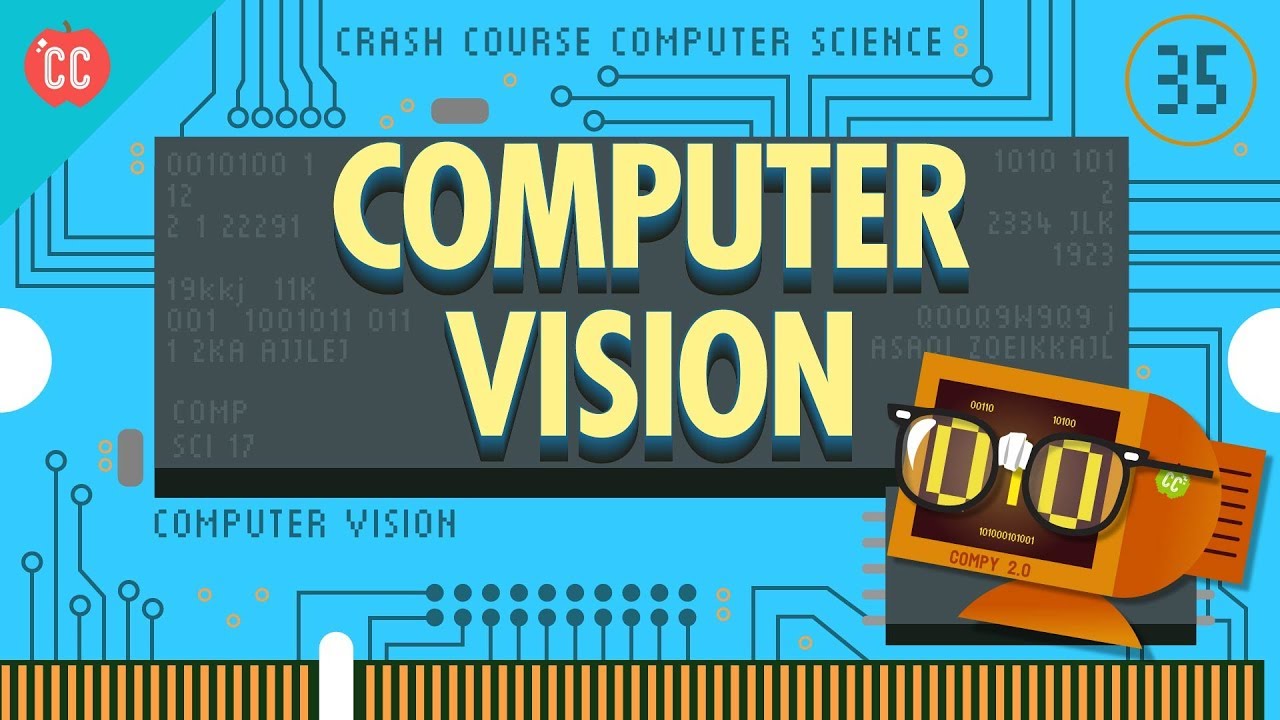
Computer Vision: Crash Course Computer Science #35
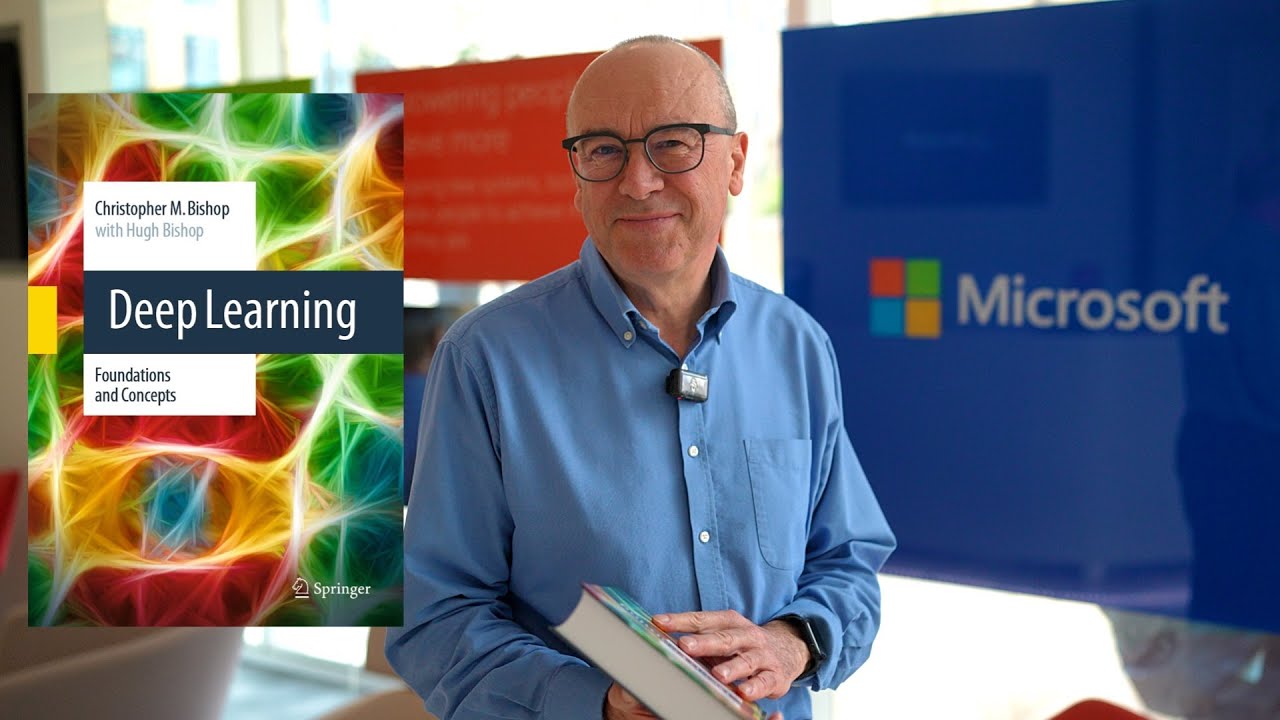
Prof. Chris Bishop's NEW Deep Learning Textbook!
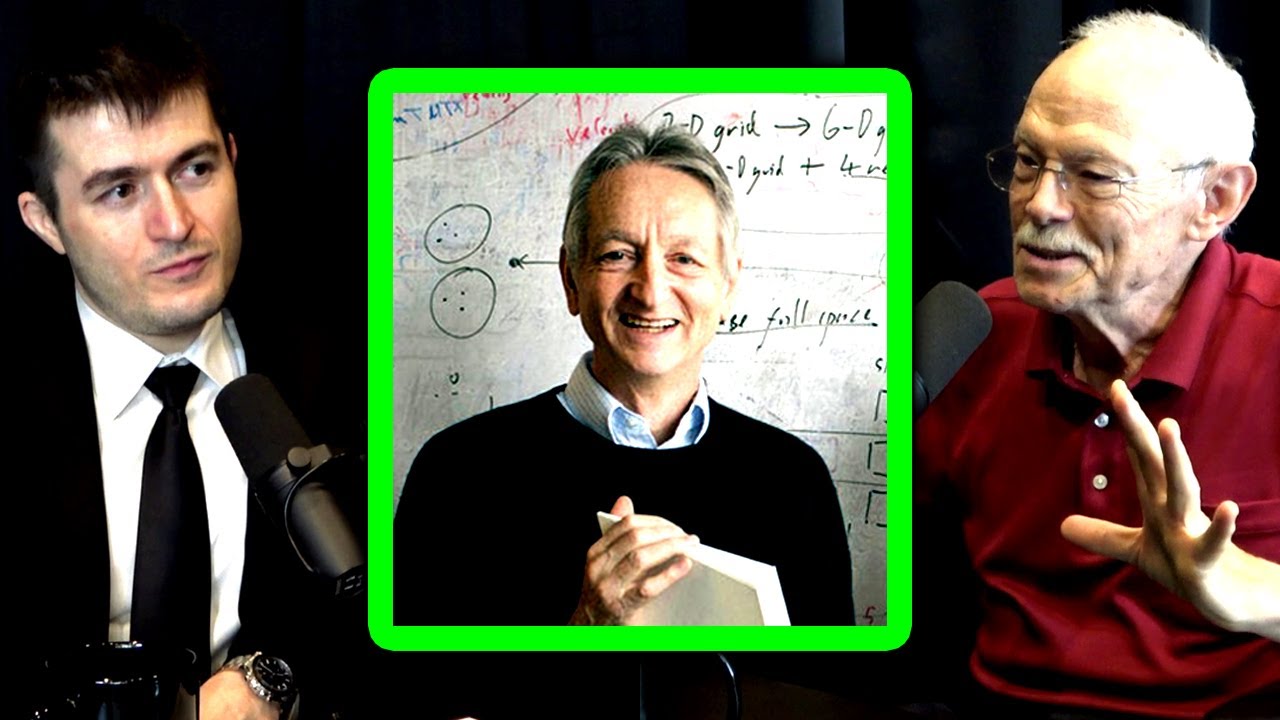
Geoffrey Hinton is a genius | Jay McClelland and Lex Fridman
5.0 / 5 (0 votes)