Geoffrey Hinton is a genius | Jay McClelland and Lex Fridman
Summary
TLDR视频脚本讲述了科学家杰夫·辛顿(Jeff Hinton)在神经网络和深度学习领域的贡献。辛顿与同事一起创建了PDP研究小组,推动了交互激活模型的研究。他们意识到,为了优化神经网络的学习,应该从生物学启发转向定义问题并调整连接权重来解决问题。这导致了梯度下降和反向传播算法的发展,后者通过从输出层向隐藏层反向传播错误信号来调整权重。辛顿的直觉式思考和对深度学习工作原理的直观解释,以及他对计算智能和认知机制的深刻理解,对视频讲述者产生了深远影响。视频还探讨了人类智能与计算智能的关系,以及如何通过构建超越生物限制的计算智能来扩展人类智能的可能性。
Takeaways
- 👨🔬 杰夫·辛顿(Jeff Hinton)是深度学习和神经网络领域的先驱,他对于反向传播算法(Backpropagation)的发展做出了重要贡献。
- 🔄 反向传播算法的核心思想是定义一个目标函数,并通过梯度下降(Gradient Descent)来调整连接权重,以最小化错误或损失。
- 📈 梯度下降的概念在工程领域已经为人所熟知,并且有一个称为delta规则的算法,由斯坦福大学的Woodrow和Hoff发明。
- 🧠 保罗·沃尔姆哈特(Paul Werbos)推广了delta规则,使其能够应用于多层神经网络,从而形成了广义delta规则,也就是后来的反向传播算法。
- 🤔 辛顿当时对反向传播算法的效果持怀疑态度,并提出了基于balsa机器概念的替代算法,但最终反向传播算法被证明更为有效。
- 🌟 辛顿在1981年发表了两篇开创性的论文,一篇关于transformers的概念,另一篇关于语义认知的早期研究,对认知科学产生了深远影响。
- 📚 辛顿在1977年未发表的一篇论文中描述了神经网络如何进行递归计算,这一想法他持续探索多年。
- 💡 辛顿的思考方式对理解人类数学认知产生了深远影响,他倾向于用直观的方式解释深度学习的工作原理,而不是使用复杂的数学方程。
- 🧵 辛顿的工作与布尔逻辑和理论物理相联系,特别是他在80年代中期关于Boltzmann机的研究,将布尔传统带入了更连续的概率和分级约束满足领域。
- 🧬 辛顿希望对推理本身做出贡献,不仅仅是人类的推理,而是更广泛地理解推理的机制。
- 🧠 人类智能只是智能的一种表现形式,辛顿和其他研究者正在探索的计算智能领域可能超越人类自身的生物学限制。
- 🚀 深度学习在谷歌大脑、OpenAI和DeepMind等项目中展现出巨大潜力,通过大规模计算解决了诸如围棋和蛋白质折叠等问题。
Q & A
杰弗里·辛顿(Jeff Hinton)在神经网络领域有哪些贡献?
-杰弗里·辛顿是深度学习和神经网络领域的先驱之一。他提出了反向传播(Backpropagation)算法,这是一种用于训练神经网络的监督学习技术。此外,他还对玻尔兹曼机(Boltzmann Machine)进行了研究,这是一种随机递归神经网络,能够学习数据的概率分布。辛顿的工作对理解人类认知和推理有深远的影响。
什么是反向传播算法,它在神经网络中的作用是什么?
-反向传播算法是一种用于训练多层神经网络的监督学习技术。它通过计算损失函数关于网络参数的梯度,并利用这些梯度来更新网络的权重。反向传播包括两个过程:正向传播和误差反向传播。在正向传播中,输入数据通过网络传播以产生输出;在误差反向传播中,计算输出和目标值之间的误差,并将这个误差信号反向传播回网络,以调整权重,减少误差。
玻尔兹曼机是如何与布尔逻辑和概率理论相结合的?
-玻尔兹曼机是一种随机神经网络,它结合了布尔逻辑和概率理论。布尔逻辑是关于推理和命题逻辑的数学理论,而概率理论处理不确定性和随机性。在玻尔兹曼机中,神经元的状态是随机确定的,反映了概率性,而网络的学习过程则涉及到逻辑和推理,因为它试图找到数据的概率分布。
杰弗里·辛顿在1981年的两篇论文中提出了哪些前瞻性的想法?
-在1981年,杰弗里·辛顿发表了两篇具有前瞻性的论文。一篇是关于变换器(transformers)的概念,这是一种在自然语言处理中非常重要的模型,用于处理序列数据。另一篇是关于语义认知的早期论文,这篇论文启发了对认知的语义方面的研究。
在深度学习中,为什么需要定义一个目标函数?
-在深度学习中,定义一个目标函数是为了量化模型的性能,即模型输出与实际值之间的差异。这个函数通常被称为损失函数或误差函数。通过最小化目标函数,可以调整网络的权重,使得模型的预测尽可能接近于真实数据,从而提高模型的准确性。
梯度下降法在神经网络训练中的作用是什么?
-梯度下降法是一种优化算法,用于最小化神经网络的损失函数。通过计算损失函数相对于网络权重的梯度,梯度下降法逐步调整权重,以减少预测误差。这是训练神经网络中的一个关键步骤,因为它指导了网络如何通过学习数据来改进其性能。
杰弗里·辛顿的研究对理解人类认知有哪些影响?
-杰弗里·辛顿的研究对理解人类认知有重要影响。他的工作不仅推动了神经网络和深度学习领域的发展,而且提供了关于大脑如何处理信息、如何进行推理和决策的新见解。特别是,他对玻尔兹曼机的研究,将逻辑和概率理论结合起来,为理解人类大脑的工作方式提供了新的视角。
为什么说反向传播算法是深度学习中的关键技术?
-反向传播算法是深度学习中的关键技术,因为它允许对多层神经网络进行有效的训练。在多层网络中,直接计算从输入到输出所有层的权重变化是非常复杂的。反向传播算法通过利用链式法则,从输出层开始,逐层反向计算误差梯度,从而简化了这一过程,使得训练复杂的深层网络成为可能。
在深度学习中,为什么需要考虑计算的规模?
-在深度学习中,计算的规模至关重要,因为模型的性能往往随着模型大小和训练数据量的增加而提高。较大的模型可以捕捉更复杂的特征,而更多的数据可以提供更丰富的信息,帮助模型更好地泛化。此外,大规模计算还可以利用并行处理和分布式计算的优势,加快训练过程。
杰弗里·辛顿的哪些思维方式对您产生了影响?
-杰弗里·辛顿的直观和视觉化的思维方式对提问者产生了深远的影响。辛顿倾向于通过图形和几何隐喻来解释深度学习的概念,而不是仅仅依赖于数学方程。这种直观的解释方式有助于更深刻地理解复杂的概念,并且使得这些概念更加易于理解和记忆。
如何理解杰弗里·辛顿在1977年未发表的论文中描述的神经网络进行递归计算的想法?
-在1977年的未发表的论文中,杰弗里·辛顿提出了一个关于神经网络进行递归计算的想法。这个想法涉及到在调用子程序时保存当前状态,并在子程序执行完毕后恢复这一状态,以便继续之前的计算。辛顿提出,可以通过快速调整连接权重来保存和恢复状态,这种方法后来成为了深度学习中处理序列数据的重要概念。
为什么说深度学习的发展与跨学科合作有关?
-深度学习的发展与跨学科合作密切相关,因为这个领域结合了计算机科学、神经科学、认知科学、数学和物理学等多个学科的理论和方法。跨学科合作促进了不同领域知识的融合,推动了深度学习算法和模型的创新。例如,玻尔兹曼机的提出就是逻辑、概率理论和物理学结合的产物。
Outlines
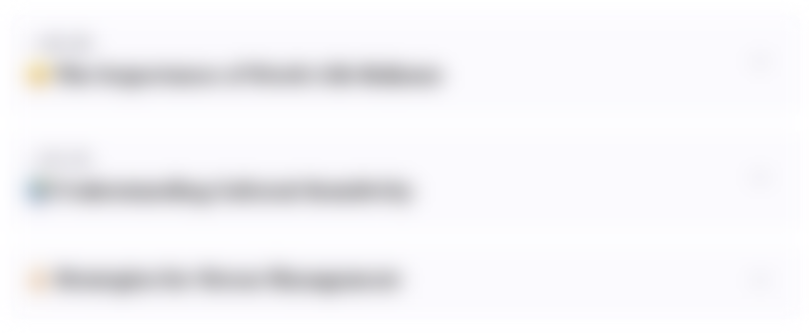
This section is available to paid users only. Please upgrade to access this part.
Upgrade NowMindmap
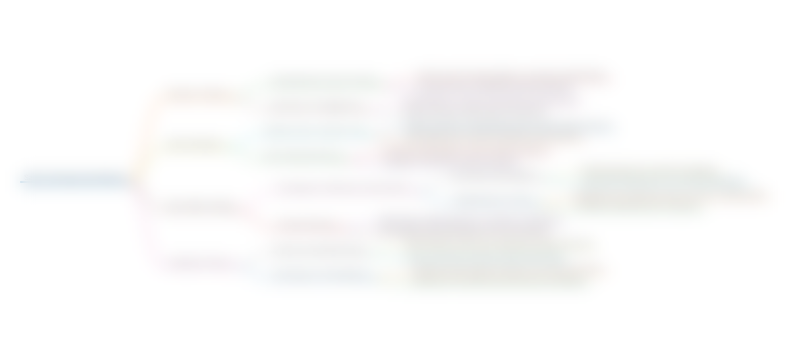
This section is available to paid users only. Please upgrade to access this part.
Upgrade NowKeywords
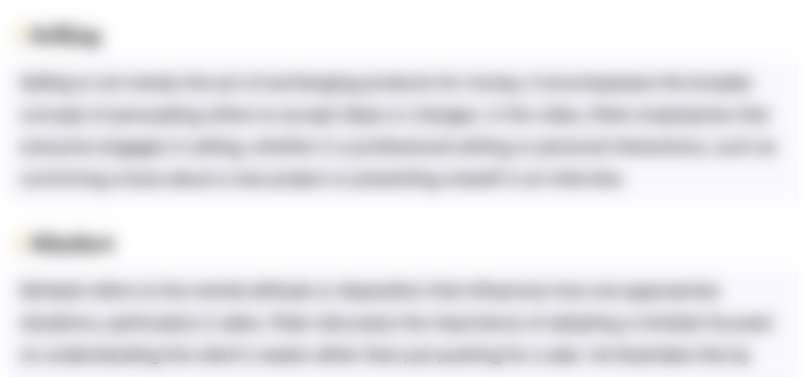
This section is available to paid users only. Please upgrade to access this part.
Upgrade NowHighlights
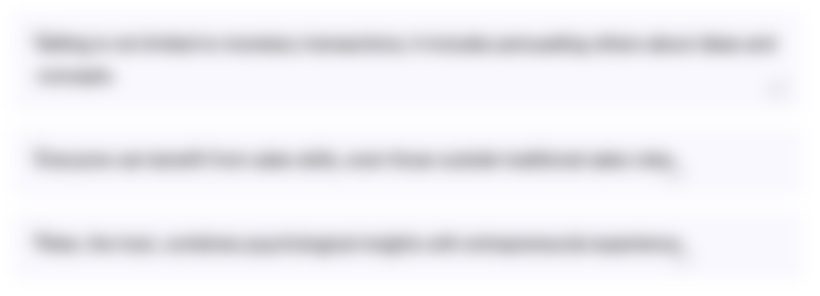
This section is available to paid users only. Please upgrade to access this part.
Upgrade NowTranscripts
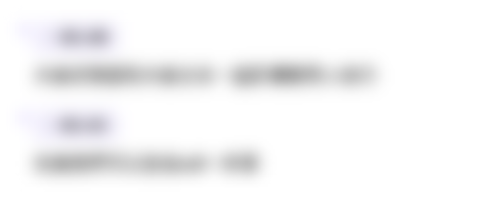
This section is available to paid users only. Please upgrade to access this part.
Upgrade NowBrowse More Related Video
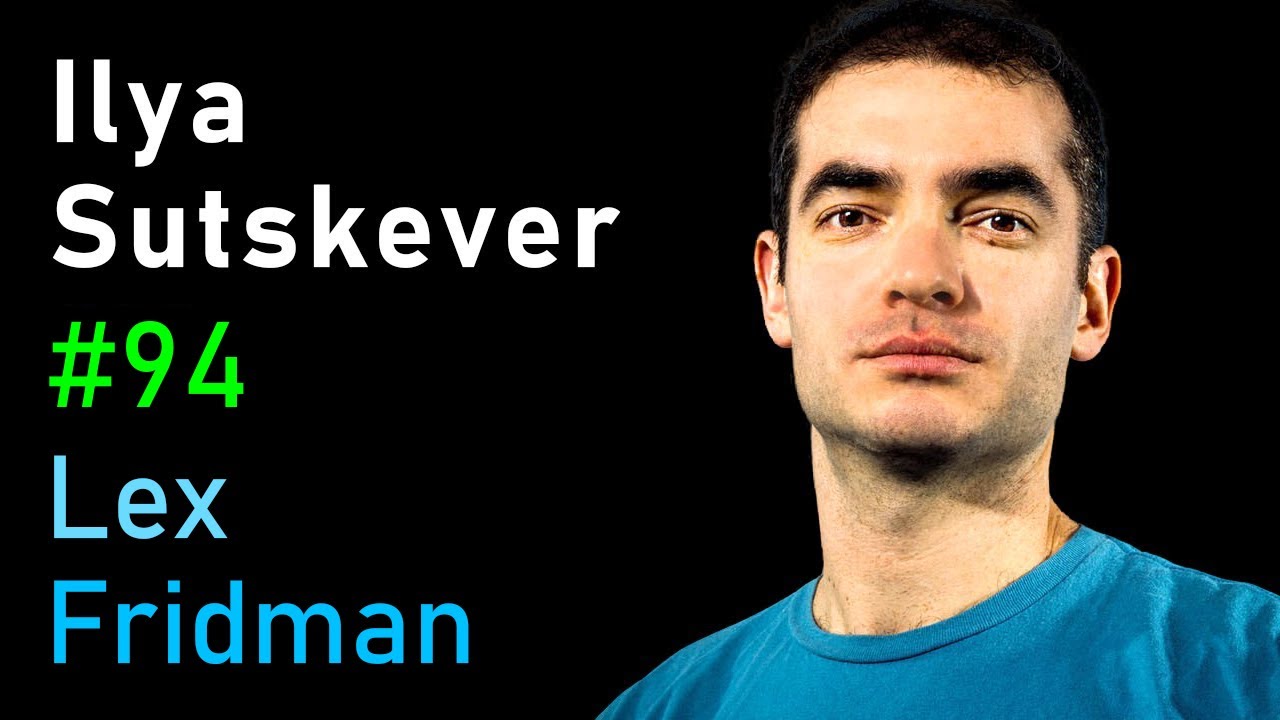
Ilya Sutskever: Deep Learning | Lex Fridman Podcast #94

Heroes of Deep Learning: Andrew Ng interviews Geoffrey Hinton
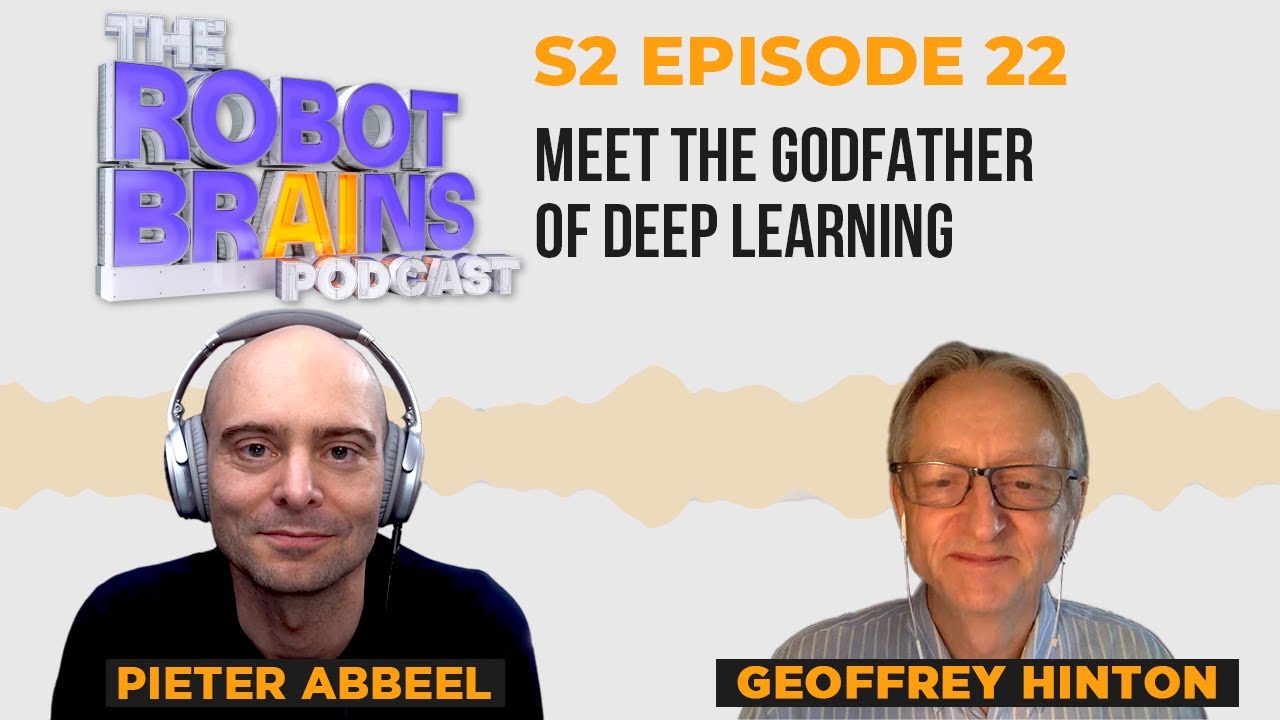
Season 2 Ep 22 Geoff Hinton on revolutionizing artificial intelligence... again
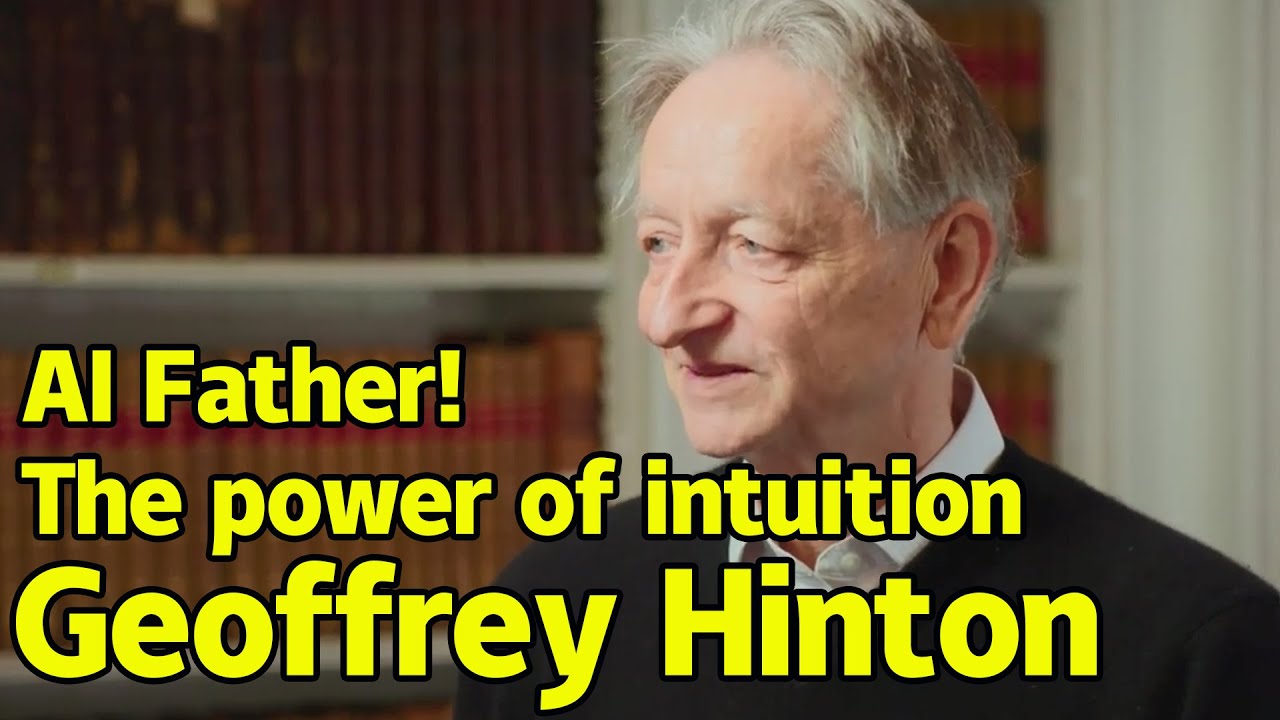
Geoffrey Hinton | Ilya's AI tutor Talent and intuition have already led to today’s large AI models
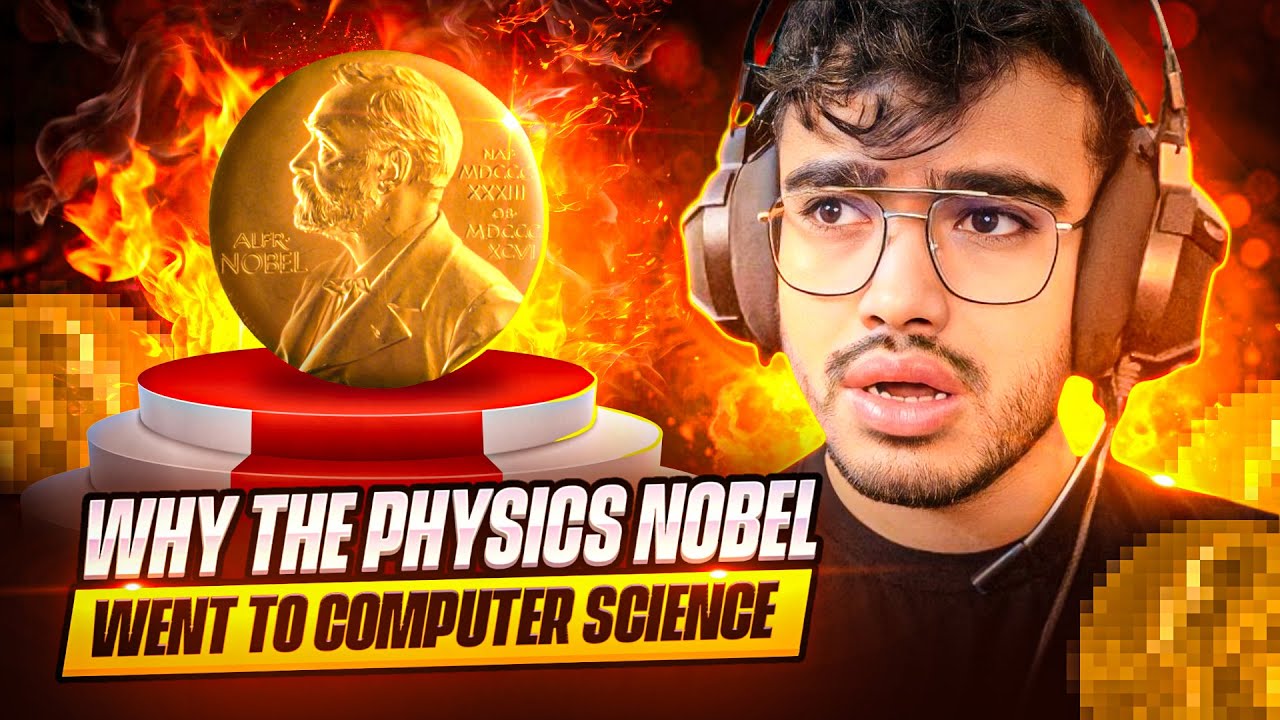
2024 Physics Nobel Prize Explained!
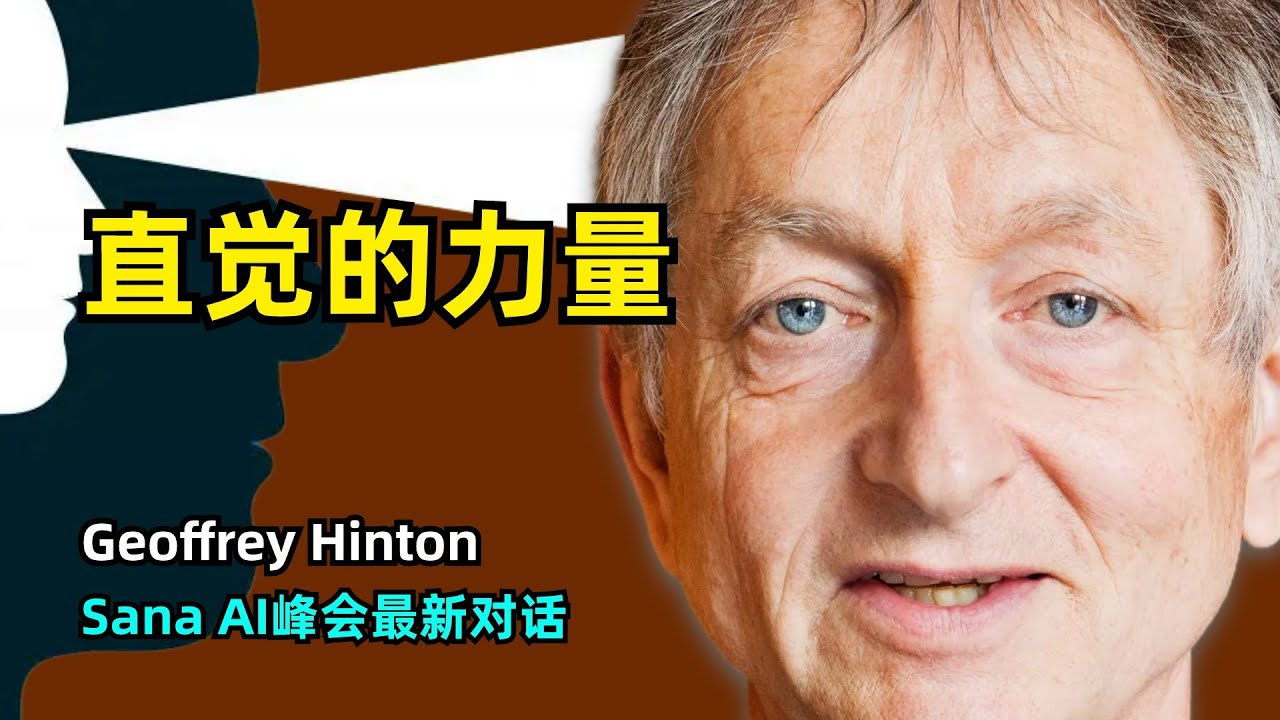
【人工智能】直觉的力量 | 杰弗里辛顿最新对话 | Sana AI峰会 | 回忆AI生涯 | Ilya的能力和直觉 | 缩放法则 | 多模态 | 语言与认知 | 神经网络 | AI情感 | 反向传播
5.0 / 5 (0 votes)