Inferential Statistics FULL Tutorial: T-Test, ANOVA, Chi-Square, Correlation & Regression Analysis
Summary
TLDRThis video demystifies inferential statistics, contrasting it with descriptive statistics. It explains how inferential stats help determine if observed patterns in sample data reflect broader population trends or are mere chance. Key inferential tests like T-tests, ANOVA, Chi-square, correlation, and regression are introduced, each serving distinct purposes—from comparing group means to analyzing relationships between variables. The video emphasizes the importance of representative sampling and cautions against assuming causation from correlation. Ideal for newcomers to statistics, it also promotes a free statistics cheat sheet for quick reference.
Takeaways
- 😀 Inferential statistics allow you to determine if patterns in a sample are representative of a larger population.
- 😀 Descriptive statistics summarize and organize existing data, while inferential statistics enable hypothesis testing and predictions.
- 😀 A T-test compares the means of two groups to assess if their differences are statistically significant.
- 😀 ANOVA (Analysis of Variance) allows for comparing the means of more than two groups simultaneously.
- 😀 The Chi-square test evaluates relationships between categorical variables, assessing if differences in proportions are significant.
- 😀 Correlation analysis examines the relationship between two numerical variables, identifying how they move together.
- 😀 A correlation coefficient (R value) indicates the strength and direction of a relationship, ranging from -1 to +1.
- 😀 Regression analysis predicts the value of a dependent variable based on one or more independent variables.
- 😀 It's crucial to understand that correlation does not imply causation; additional research is needed to establish cause-effect relationships.
- 😀 Utilizing a statistical cheat sheet can help simplify the selection and application of various statistical tests.
Q & A
What are inferential statistics?
-Inferential statistics are methods that allow researchers to make generalizations about a population based on a sample, testing hypotheses and determining if observed patterns are statistically significant.
How do inferential statistics differ from descriptive statistics?
-Descriptive statistics summarize and organize data from a sample, while inferential statistics assess whether the patterns seen in the sample reflect trends in the broader population and can make predictions.
What is statistical significance?
-Statistical significance refers to the likelihood that a relationship observed in data is not due to random chance, indicating that the findings may be representative of the broader population.
What is a T-test and when is it used?
-A T-test is a statistical test used to compare the means of two groups to determine if there is a significant difference between them, such as comparing exam scores of two classes.
What is ANOVA and how is it different from a T-test?
-ANOVA (Analysis of Variance) is used to compare the means of three or more groups, whereas a T-test is limited to comparing two groups.
What does a Chi-Square test assess?
-A Chi-Square test examines whether there is a statistically significant relationship between two categorical variables, such as gender and vehicle preference.
What is correlation analysis?
-Correlation analysis evaluates the relationship between two numerical variables to see how they move together, providing a correlation coefficient (R value) that indicates the strength and direction of the relationship.
What is regression analysis used for?
-Regression analysis is used to predict the value of a dependent variable based on one or more independent variables, allowing researchers to estimate outcomes, such as house prices based on various factors.
Why is it important to understand sampling strategies when using inferential statistics?
-Understanding sampling strategies is crucial because the reliability of inferential statistics depends on whether the sample accurately represents the population from which it is drawn.
What is the main limitation of correlation analysis?
-The main limitation of correlation analysis is that it does not prove causation; it only indicates that two variables are related, not that one causes the other.
Outlines
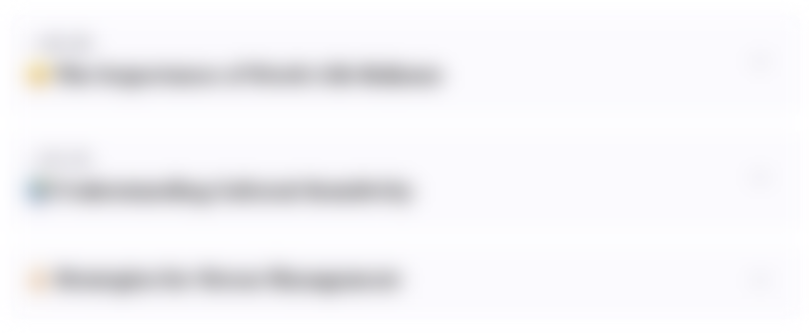
Esta sección está disponible solo para usuarios con suscripción. Por favor, mejora tu plan para acceder a esta parte.
Mejorar ahoraMindmap
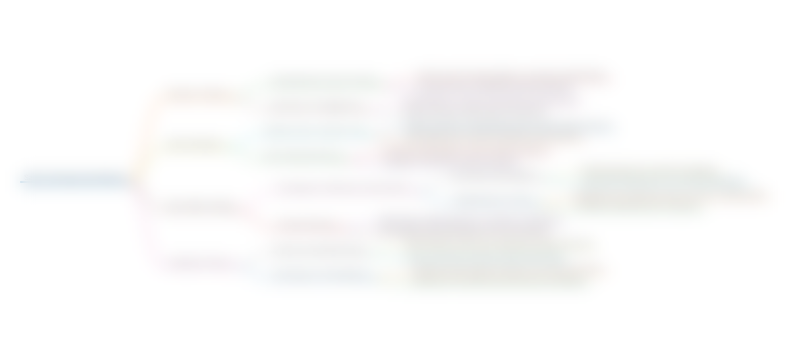
Esta sección está disponible solo para usuarios con suscripción. Por favor, mejora tu plan para acceder a esta parte.
Mejorar ahoraKeywords
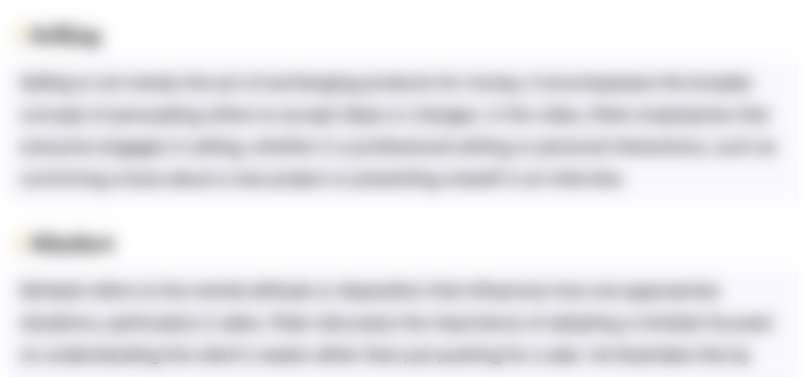
Esta sección está disponible solo para usuarios con suscripción. Por favor, mejora tu plan para acceder a esta parte.
Mejorar ahoraHighlights
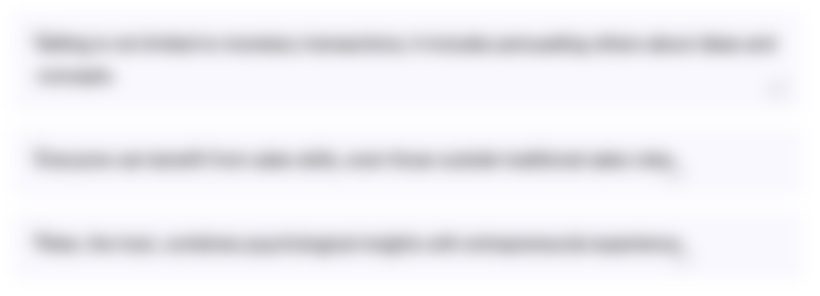
Esta sección está disponible solo para usuarios con suscripción. Por favor, mejora tu plan para acceder a esta parte.
Mejorar ahoraTranscripts
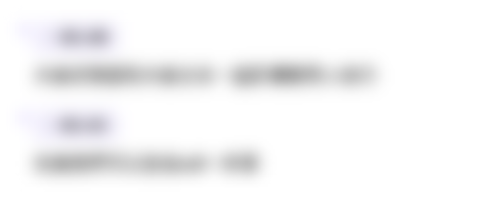
Esta sección está disponible solo para usuarios con suscripción. Por favor, mejora tu plan para acceder a esta parte.
Mejorar ahoraVer Más Videos Relacionados

KUPAS TUNTAS: Apakah Perbedaan Statistik Inferensial dengan Statistik Deskriptif ?
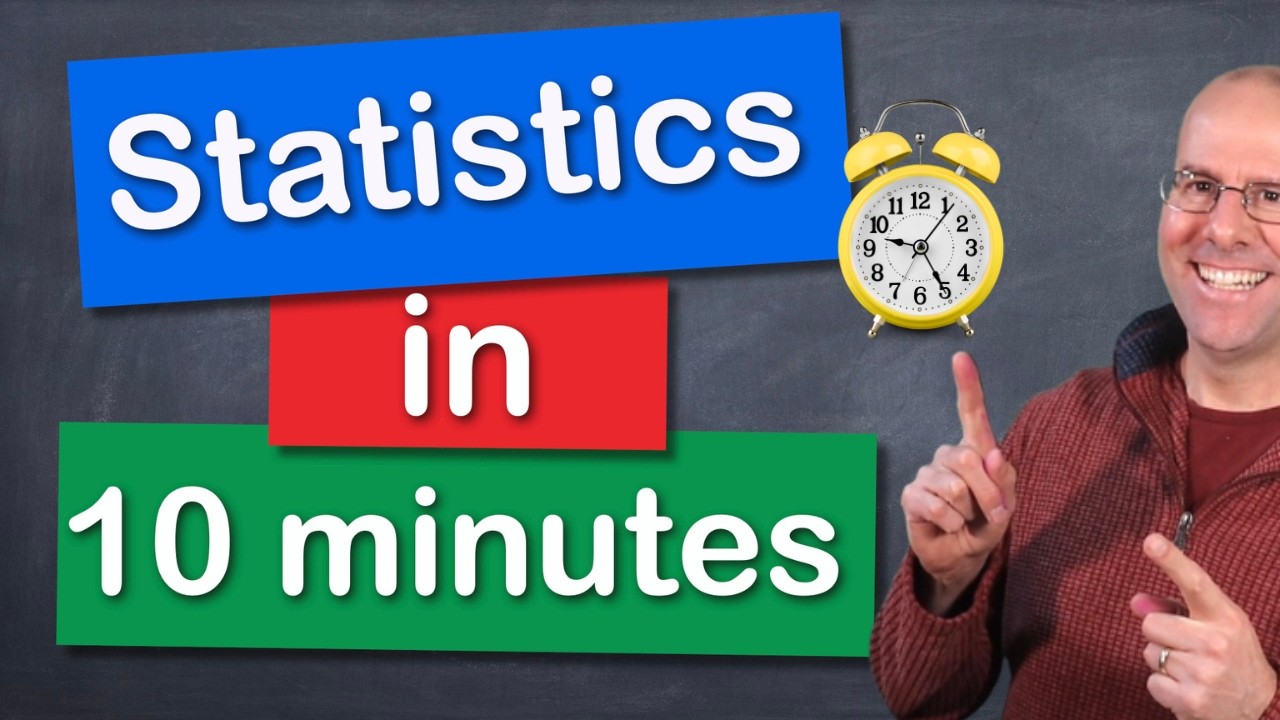
Statistics in 10 minutes. Hypothesis testing, the p value, t-test, chi squared, ANOVA and more
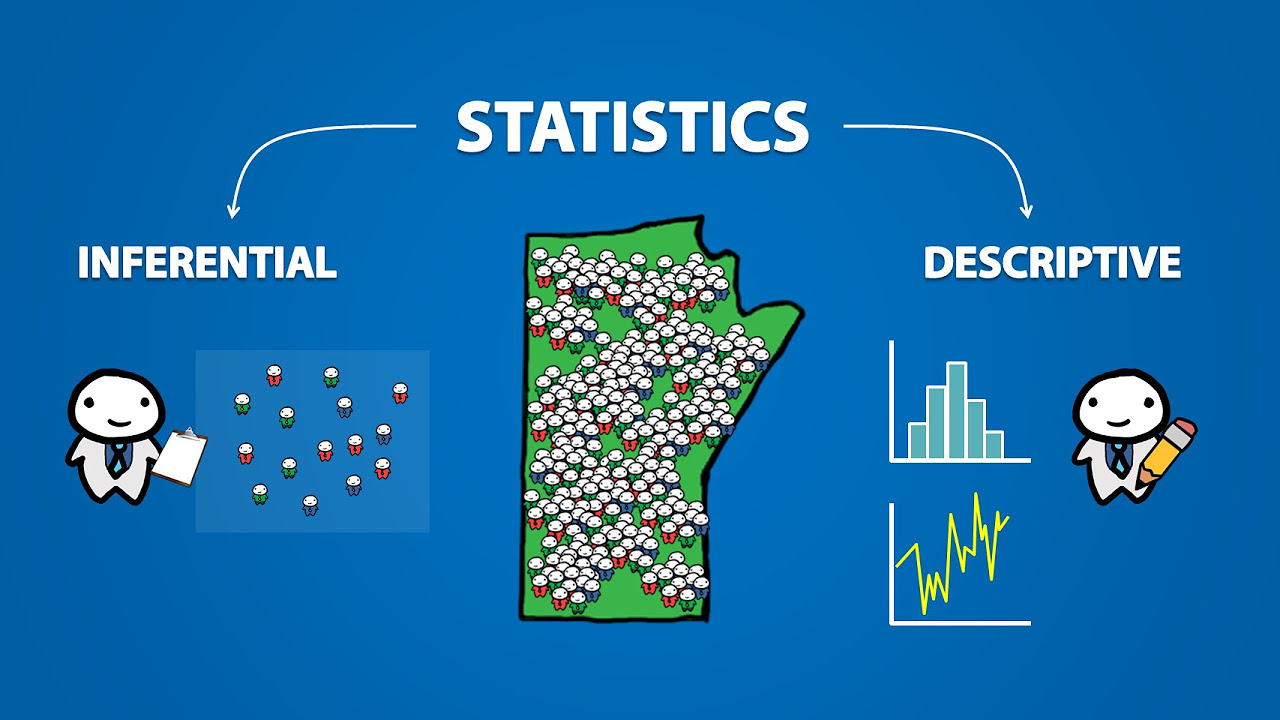
Introduction to Statistics (1.1)
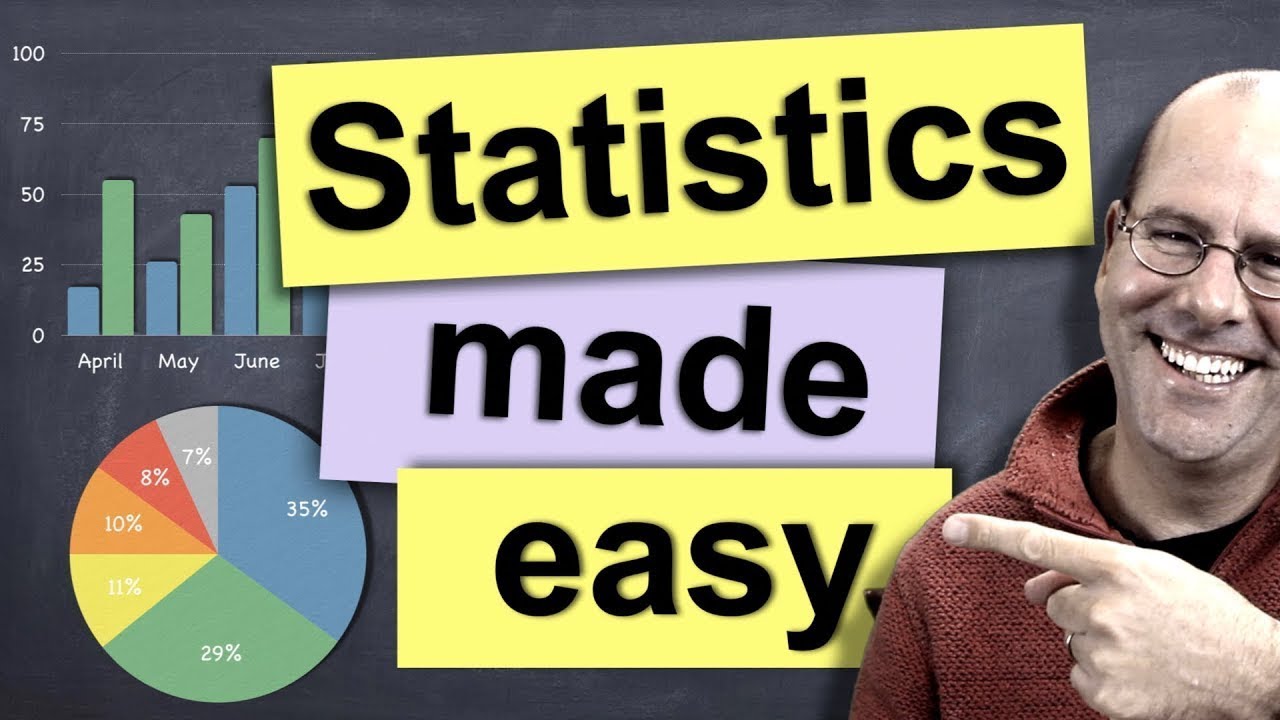
Statistics made easy ! ! ! Learn about the t-test, the chi square test, the p value and more
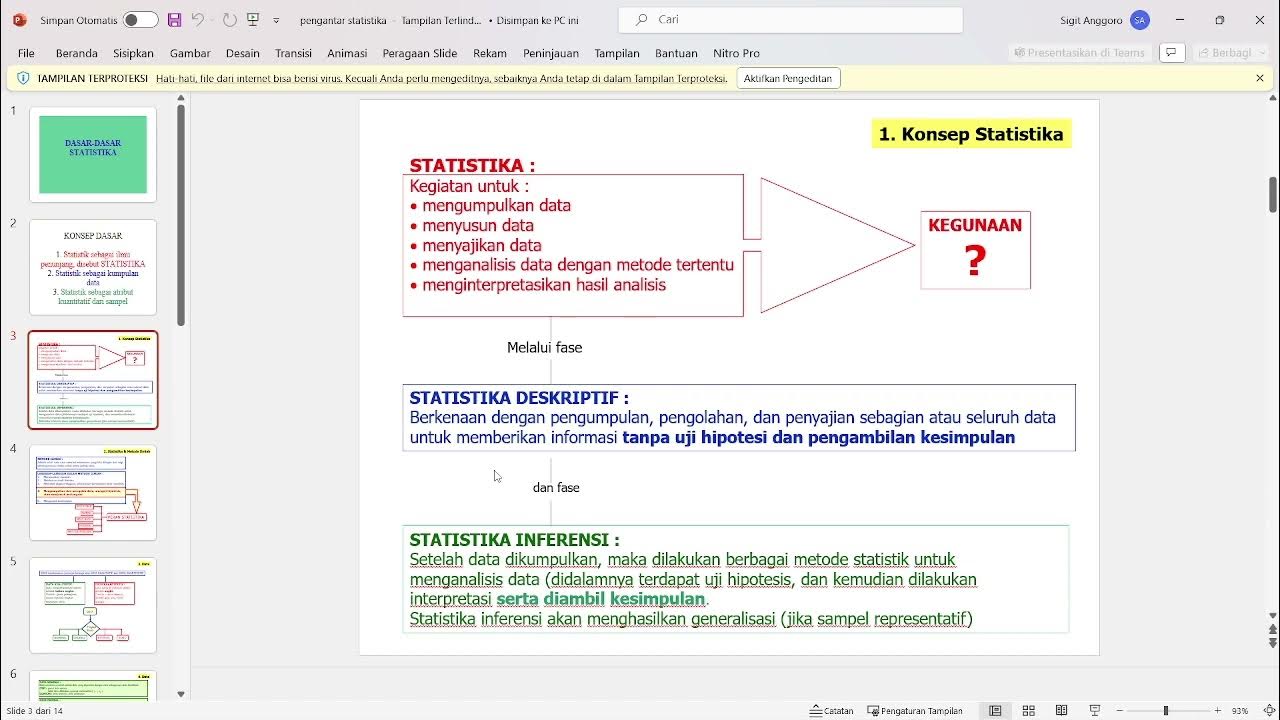
Statisitik ke 3-1
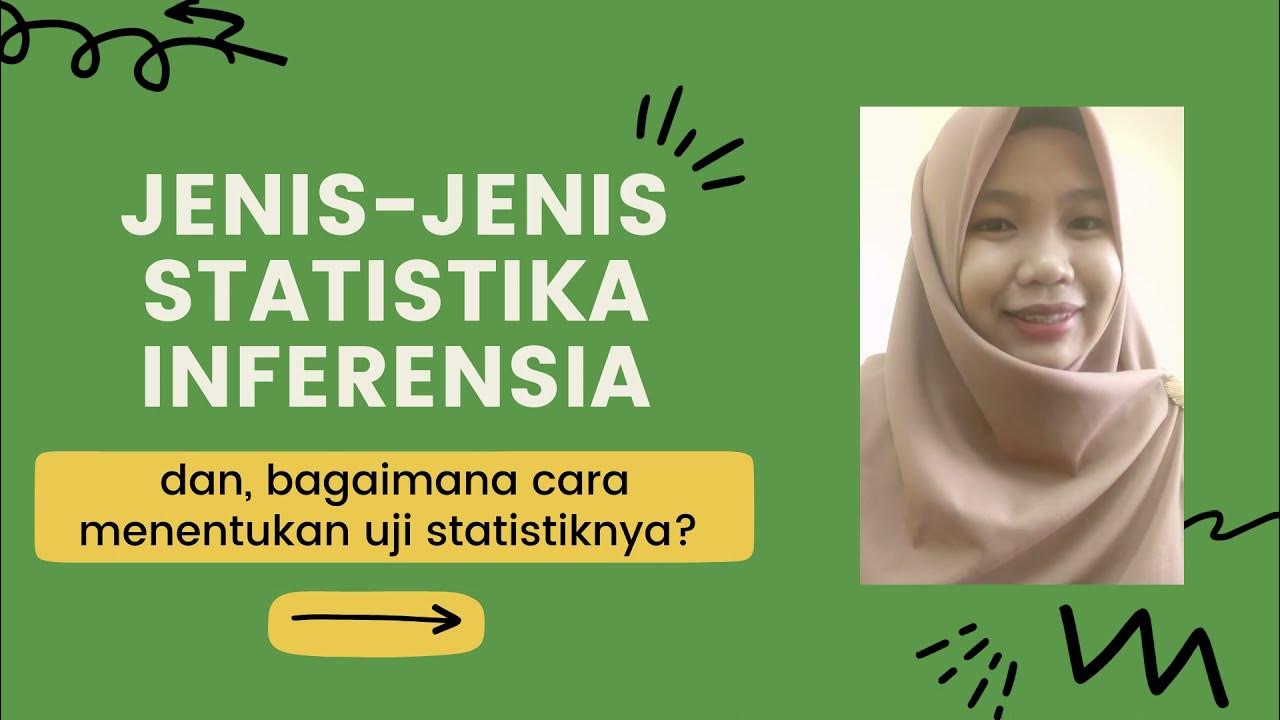
[PART 2] KONSEP DASAR STATISTIKA INFERENSIA
5.0 / 5 (0 votes)